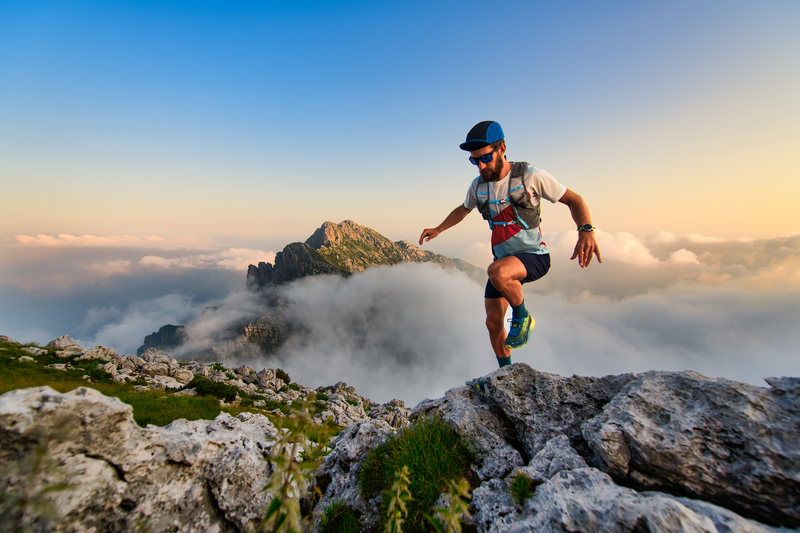
94% of researchers rate our articles as excellent or good
Learn more about the work of our research integrity team to safeguard the quality of each article we publish.
Find out more
ORIGINAL RESEARCH article
Front. Public Health
Sec. Environmental Health and Exposome
Volume 13 - 2025 | doi: 10.3389/fpubh.2025.1520354
The final, formatted version of the article will be published soon.
You have multiple emails registered with Frontiers:
Please enter your email address:
If you already have an account, please login
You don't have a Frontiers account ? You can register here
In the context of insufficient water resources and deteriorating water quality in China, this study investigates the impact of water quality on residents' self-assessed health using data from the 2016 China Genuine Progress indicator Survey. Employing an ordered probit (oprobit) model, the analysis focuses on household cooking water sources (river/lake, well, tap, mineral/purified/filtered) and water pollution exposure in living or working environments. Results indicate that transitioning from river/lake water to safer sources-well, tap, and mineral/purified/filtered water-increases the probability of residents' reporting self-assessed health ratings as "very good" by 7.9%, 10.4% and 12.9%, while reducing that of "very bad or not very good" by 7.2%, 9.4% and 11.7%, respectively. Conversely, exposure to water pollution in living or working place decreases the likelihood of self-assessed health ratings as "very good" by 2.4% and raises that of "very bad or not very good" by 2.1%. The impact of cooking water quality on health outcome is confirmed for the subsample with lower socioeconomic status, while the impact of water pollution exposure on health outcome is more significant for the subsample with higher socioeconomic status. Robustness checks using hospitalization days as an alternative dependent variable and replacing oprobit with ologit/OLS models confirm these findings. The study underscores the critical role of safe water access and ecological protection in enhancing public health. Policy recommendations include using and managing water resources strictly for holistic water security, maximizing the potential of China's revised Environmental Protection Laws and establishing a cross-agency coordination mechanism to tackle pollution sources, and improving medical services and fitness facilities to advance the "Healthy China" initiative.
Keywords: Water Quality, Water ecological pollution, Self-assessed health rating, Oprobit model, Robustness check
Received: 31 Oct 2024; Accepted: 28 Feb 2025.
Copyright: © 2025 Yan and Cui. This is an open-access article distributed under the terms of the Creative Commons Attribution License (CC BY). The use, distribution or reproduction in other forums is permitted, provided the original author(s) or licensor are credited and that the original publication in this journal is cited, in accordance with accepted academic practice. No use, distribution or reproduction is permitted which does not comply with these terms.
* Correspondence:
Jing Yan, Shanxi Academy of Governance, Taiyuan, China
Disclaimer: All claims expressed in this article are solely those of the authors and do not necessarily represent those of their affiliated organizations, or those of the publisher, the editors and the reviewers. Any product that may be evaluated in this article or claim that may be made by its manufacturer is not guaranteed or endorsed by the publisher.
Research integrity at Frontiers
Learn more about the work of our research integrity team to safeguard the quality of each article we publish.