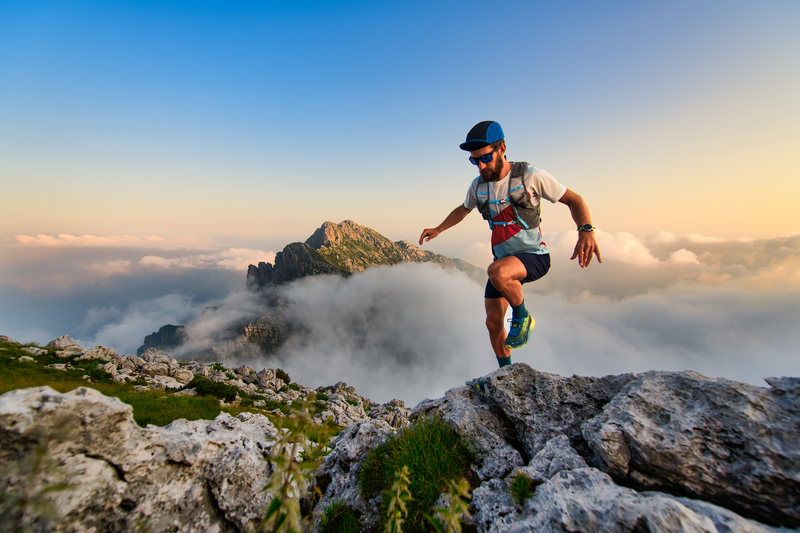
94% of researchers rate our articles as excellent or good
Learn more about the work of our research integrity team to safeguard the quality of each article we publish.
Find out more
ORIGINAL RESEARCH article
Front. Public Health
Sec. Health Economics
Volume 13 - 2025 | doi: 10.3389/fpubh.2025.1518327
This article is part of the Research Topic Sustainable and Mission-oriented Innovation in Economic Systems and Governance for Equitable Global Health and Wellbeing View all 23 articles
The final, formatted version of the article will be published soon.
You have multiple emails registered with Frontiers:
Please enter your email address:
If you already have an account, please login
You don't have a Frontiers account ? You can register here
Investigating the network of firms in a specific industry helps explain industrial location and urban functions and provides guidelines for promoting industrial restructuring and high-quality development. This study develops a network model for the relationship between firms and cities based on the data of listed Specialized, Refined, Distinctive, and Innovative (SRDI) medical device manufacturing firms in China to identify the spatial distribution and influencing factors of the urban network of such firms using network analysis and GeoDetector. Three conclusions are obtained from the study. First, the urban network of listed SRDI medical device manufacturing firms exhibits a sparse structure, with the density decreasing from east to west, and the out-degree presenting significant spatial concentration. Suzhou, Shanghai, and Shenzhen are the core of the network power. The in-degree presents low spatial concentration. Clearly differentiated network functions are observed. Second, significant spatial differences are noted between high-and low-level linkage networks from the perspective of corporate governance structure. Third, economic level, labour costs, level of opening-up, talent base, and technological innovation capability have significant effects on the urban network of listed SRDI medical device manufacturing firms.
Keywords: specialized and sophisticated firms, Urban network, spatial organization, Listed firms, Medical device industry
Received: 28 Oct 2024; Accepted: 21 Feb 2025.
Copyright: © 2025 Hu, Yang, Qiu, Wei, Hu and Zhou. This is an open-access article distributed under the terms of the Creative Commons Attribution License (CC BY). The use, distribution or reproduction in other forums is permitted, provided the original author(s) or licensor are credited and that the original publication in this journal is cited, in accordance with accepted academic practice. No use, distribution or reproduction is permitted which does not comply with these terms.
* Correspondence:
Hao Hu, Shanghai University, Shanghai, 200444, Shanghai Municipality, China
Haiyan Zhou, Nueva Ecija University of Science and Technology, Cabanatuan, 3100, Philippines
Disclaimer: All claims expressed in this article are solely those of the authors and do not necessarily represent those of their affiliated organizations, or those of the publisher, the editors and the reviewers. Any product that may be evaluated in this article or claim that may be made by its manufacturer is not guaranteed or endorsed by the publisher.
Research integrity at Frontiers
Learn more about the work of our research integrity team to safeguard the quality of each article we publish.