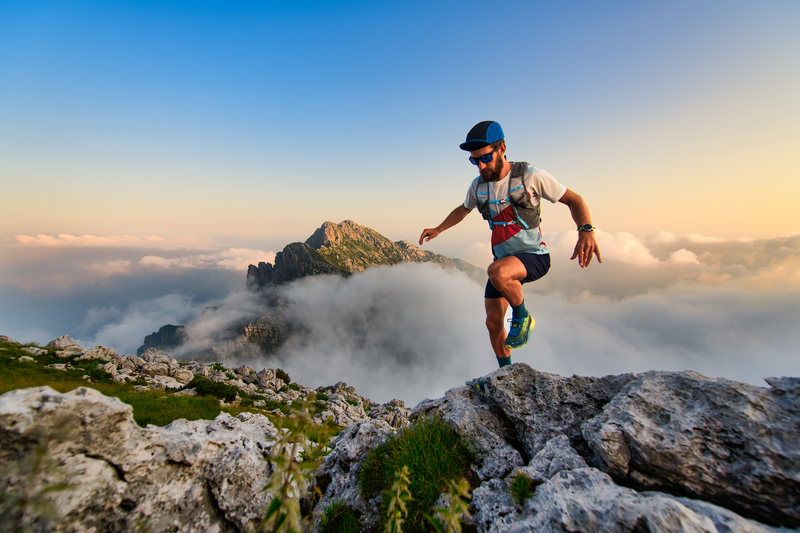
94% of researchers rate our articles as excellent or good
Learn more about the work of our research integrity team to safeguard the quality of each article we publish.
Find out more
ORIGINAL RESEARCH article
Front. Public Health
Sec. Children and Health
Volume 13 - 2025 | doi: 10.3389/fpubh.2025.1513567
The final, formatted version of the article will be published soon.
You have multiple emails registered with Frontiers:
Please enter your email address:
If you already have an account, please login
You don't have a Frontiers account ? You can register here
Background: Despite their utility for program planning, acute malnutrition treatment coverage estimates at the national and sub-national levels are rarely available. Prior work has identified methodological concerns with current approaches.We estimated the point prevalence and treatment coverage of acute malnutrition in 11 districts (or similar subnational areas) across four high-burden countries in Africa using representative cluster-based population survey methods and compared these estimates to those derived from administrative data and other direct methods where available. We also aimed to assess information about risk factors for malnourished children by coverage status.The point estimate of coverage suggests that <20% of eligible children with severe acute malnutrition (SAM) were enrolled in treatment in nine administrative areas. We found that in some contexts, coverage estimates derived using administrative data are useful, while in others, they are not -and that their accuracy can vary by month and year. By comparison, coverage estimates from other direct methods were overestimated and/or outdated, and practitioners tended to overestimate coverage. Coverage did not differ significantly by sex or age of the child but did vary by mid-upper arm circumference (MUAC) at assessment. Measured SAM coverage did not correlate either with measured SAM prevalence or with expected coverage estimated a priori by program staff.Our findings suggest that in the assessed high-burden countries, many more children are eligible for treatment than are enrolled. We present this methodology as an alternative to existing primary methods and a complement to coverage estimates from routine program and population data.
Keywords: Cluster survey, coverage, SQUEAC, Severe acute malnutrition, Therapeutic feeding program
Received: 18 Oct 2024; Accepted: 28 Feb 2025.
Copyright: © 2025 Heymsfield, Radin, Biotteau, Kangas, Tausanovitch, Tesfai, Kiema, OUEDRAOGO, Mamoudou, Issa, Bangali, Wa Ngboloko, Chaïbou, Maman, Leidman and Bilukha. This is an open-access article distributed under the terms of the Creative Commons Attribution License (CC BY). The use, distribution or reproduction in other forums is permitted, provided the original author(s) or licensor are credited and that the original publication in this journal is cited, in accordance with accepted academic practice. No use, distribution or reproduction is permitted which does not comply with these terms.
* Correspondence:
Grace Heymsfield, International Rescue Committee, New York, United States
Disclaimer: All claims expressed in this article are solely those of the authors and do not necessarily represent those of their affiliated organizations, or those of the publisher, the editors and the reviewers. Any product that may be evaluated in this article or claim that may be made by its manufacturer is not guaranteed or endorsed by the publisher.
Research integrity at Frontiers
Learn more about the work of our research integrity team to safeguard the quality of each article we publish.