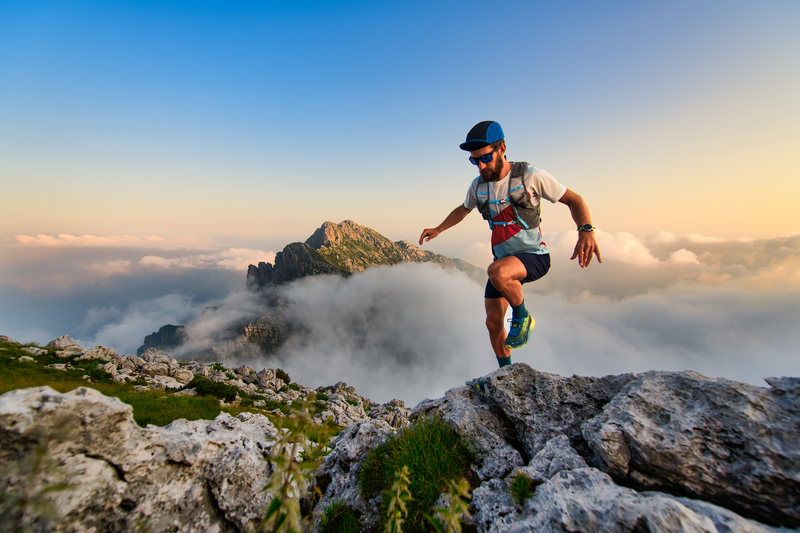
94% of researchers rate our articles as excellent or good
Learn more about the work of our research integrity team to safeguard the quality of each article we publish.
Find out more
ORIGINAL RESEARCH article
Front. Public Health
Sec. Environmental Health and Exposome
Volume 13 - 2025 | doi: 10.3389/fpubh.2025.1505170
The final, formatted version of the article will be published soon.
You have multiple emails registered with Frontiers:
Please enter your email address:
If you already have an account, please login
You don't have a Frontiers account ? You can register here
Background:Long-term exposure to air pollution is associated with a higher incidence of various non-infectious diseases.However,not only air pollution,but also other risk factors,such as lifestyle, can play a role in the occurrence of these diseases or premature deaths from them. The study aimed to compare the lifestyle of residents of two differently air polluted regions and to determine how lifestyle is affected by socioeconomic variables.Methods:In the framework of the project Healthy Ageing in Industrial Environments,two cohorts of persons from an industrial area and a control area were established.The cohorts consisted of individuals aged 35 to 65 years.Lifestyle factors included diet,BMI,alcohol and cigarette consumption,duration of sleep,physical activity,and time spent doing hobbies.Influencing factors included region, sex, age,education,family status,and economic situation.Fully adjusted binary and ordinal logistic regression models were used for evaluation,and the output was the odds ratio (OR) with 95% confidence intervals (CI).Results:The effect of more air polluted industrial region was related to higher BMI (OR = 1.23; 95% CI: 1.08-1.4) and physical activity (OR = 1.31; 95% CI: 1.13-1.51) and surprisingly to lower smoking level (OR = 0.84; 95% CI: 0.74-0.99).Conclusion: The results of our study are useful in targeting public health strategies and intervention programs to specific populations, and the results will be share with public awareness groups that focus on prevention and the physiological aspects of physical activity.
Keywords: Air Pollution, lifestyle, Industrial region, Public Health, environmental
Received: 02 Oct 2024; Accepted: 22 Jan 2025.
Copyright: © 2025 Riedlova, Tomaskova, Slachtova, Babjakova and Jirik. This is an open-access article distributed under the terms of the Creative Commons Attribution License (CC BY). The use, distribution or reproduction in other forums is permitted, provided the original author(s) or licensor are credited and that the original publication in this journal is cited, in accordance with accepted academic practice. No use, distribution or reproduction is permitted which does not comply with these terms.
* Correspondence:
Petra Riedlova, University of Ostrava, Ostrava, Czechia
Disclaimer: All claims expressed in this article are solely those of the authors and do not necessarily represent those of their affiliated organizations, or those of the publisher, the editors and the reviewers. Any product that may be evaluated in this article or claim that may be made by its manufacturer is not guaranteed or endorsed by the publisher.
Research integrity at Frontiers
Learn more about the work of our research integrity team to safeguard the quality of each article we publish.