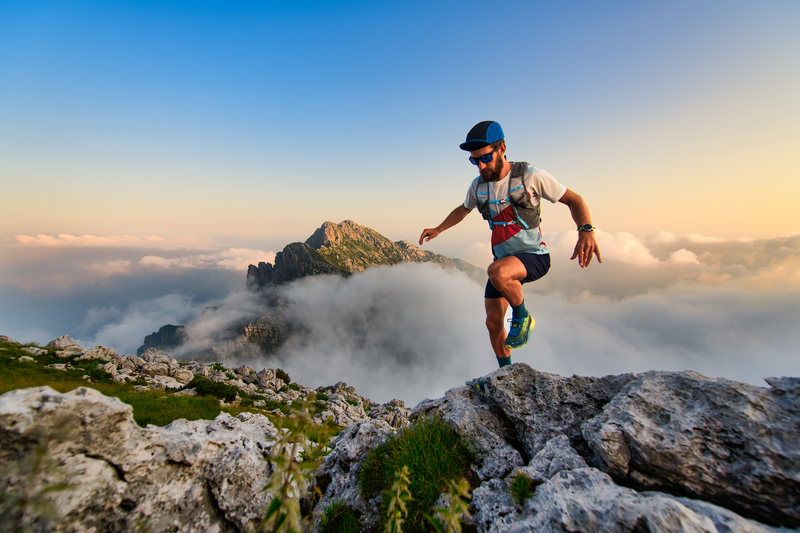
94% of researchers rate our articles as excellent or good
Learn more about the work of our research integrity team to safeguard the quality of each article we publish.
Find out more
ORIGINAL RESEARCH article
Front. Public Health
Sec. Environmental Health and Exposome
Volume 13 - 2025 | doi: 10.3389/fpubh.2025.1503522
The final, formatted version of the article will be published soon.
You have multiple emails registered with Frontiers:
Please enter your email address:
If you already have an account, please login
You don't have a Frontiers account ? You can register here
The health status of low-income rural residents is intricately linked to social equity and justice and the realization of the goal of common prosperity. Based on the CFPS data from 2010 to 2020 and the list of "Broadband China" demonstration cities, this paper employs a multi-period Difference-in-Differences (DID) approach to empirically analyze the impact of digital infrastructure construction on the health of low-income rural residents and its mechanism. The results indicate that: (1) Digital infrastructure construction had a significant positive impact on the health of rural low-income residents. After adjusting the sample period, changing the policy implementation time point, excluding other policy interference, and Propensity Score Matching-Difference-in-Differences (PSM-DID), the model was still robust. (2) The mechanism test found that digital infrastructure would improves the health of rural low-income residents through three mechanisms: the adoption of new agricultural technology, land transfer, and leisure time. (3) In terms of heterogeneity analysis, based on region type, education level, marital status and public supporting system, digital infrastructure construction can enhance the health status of low-income rural residents in the western region, those with low education level, married people and those with more public supporting system. This study suggests the continued promotion of the construction of digital infrastructure, with a focus on the construction level of the western and western regions, narrow the "digital divide" between different groups, and improve public supporting facilities in backward rural areas, so as to further enhance the health status of low-income groups.
Keywords: digital infrastructure construction1, Low-income rural residents2, Broadband China, Multiple stage DID4, China Household Tracking Survey5
Received: 05 Oct 2024; Accepted: 24 Feb 2025.
Copyright: © 2025 Wang, Ning and Tan. This is an open-access article distributed under the terms of the Creative Commons Attribution License (CC BY). The use, distribution or reproduction in other forums is permitted, provided the original author(s) or licensor are credited and that the original publication in this journal is cited, in accordance with accepted academic practice. No use, distribution or reproduction is permitted which does not comply with these terms.
* Correspondence:
Qian Wang, School of Nursing, Jiangxi University of Science and Technology, Nanchang, China
Disclaimer: All claims expressed in this article are solely those of the authors and do not necessarily represent those of their affiliated organizations, or those of the publisher, the editors and the reviewers. Any product that may be evaluated in this article or claim that may be made by its manufacturer is not guaranteed or endorsed by the publisher.
Research integrity at Frontiers
Learn more about the work of our research integrity team to safeguard the quality of each article we publish.