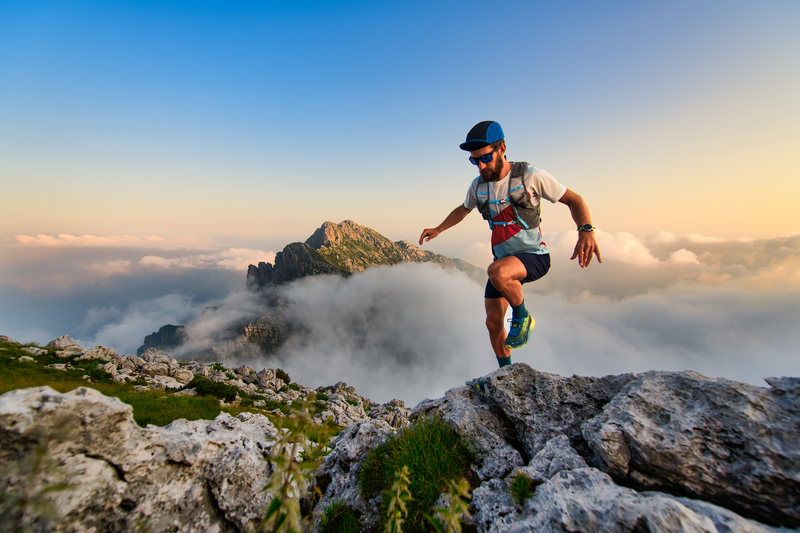
94% of researchers rate our articles as excellent or good
Learn more about the work of our research integrity team to safeguard the quality of each article we publish.
Find out more
PERSPECTIVE article
Front. Public Health
Sec. Life-Course Epidemiology and Social Inequalities in Health
Volume 13 - 2025 | doi: 10.3389/fpubh.2025.1499468
This article is part of the Research Topic Advancing Equity in Maternal Health: Addressing the Care Needs of Underserved Women View all 6 articles
The final, formatted version of the article will be published soon.
You have multiple emails registered with Frontiers:
Please enter your email address:
If you already have an account, please login
You don't have a Frontiers account ? You can register here
Maternal morbidity and mortality (MMM) rates in the U.S. are high and increasing, and are disproportionately experienced by understudied, underrepresented, and underreported (U3) populations, especially Black, Indigenous, and/or rural women. Decreasing MMM among U3 women would substantially improve maternal health equity and health outcomes, yet current data limitations inhibit our ability to fully understand the reasons underlying the disparities or regional nuances. This article calls for leveraging diverse, publicly available data such as deidentified health system utilization data; geocoded locations of hospitals providing multiple levels of maternal care services; and social determinants and demographic data into a series of linked datasets to enable county-level investigations of maternal health equity, healthcare utilization, and health outcomes.
Keywords: Maternal health, linked data, Health Equity (MeSH), BIRTH OUTCOMES, Health Policy
Received: 20 Sep 2024; Accepted: 13 Feb 2025.
Copyright: © 2025 Colf and Shreffler. This is an open-access article distributed under the terms of the Creative Commons Attribution License (CC BY). The use, distribution or reproduction in other forums is permitted, provided the original author(s) or licensor are credited and that the original publication in this journal is cited, in accordance with accepted academic practice. No use, distribution or reproduction is permitted which does not comply with these terms.
* Correspondence:
Karina M. Shreffler, University of Oklahoma Health Sciences Center, Oklahoma City, United States
Disclaimer: All claims expressed in this article are solely those of the authors and do not necessarily represent those of their affiliated organizations, or those of the publisher, the editors and the reviewers. Any product that may be evaluated in this article or claim that may be made by its manufacturer is not guaranteed or endorsed by the publisher.
Research integrity at Frontiers
Learn more about the work of our research integrity team to safeguard the quality of each article we publish.