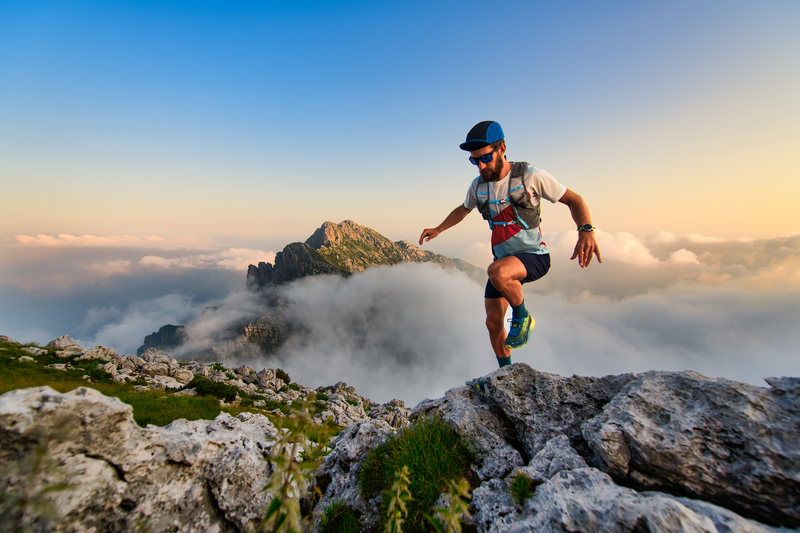
94% of researchers rate our articles as excellent or good
Learn more about the work of our research integrity team to safeguard the quality of each article we publish.
Find out more
ORIGINAL RESEARCH article
Front. Public Health
Sec. Infectious Diseases: Epidemiology and Prevention
Volume 13 - 2025 | doi: 10.3389/fpubh.2025.1478736
This article is part of the Research Topic Diagnosis and Treatment Strategies of Tick-borne Diseases View all 8 articles
The final, formatted version of the article will be published soon.
You have multiple emails registered with Frontiers:
Please enter your email address:
If you already have an account, please login
You don't have a Frontiers account ? You can register here
Objective: To determine the current and future suitable areas of Rickettsia japonica, and to provide a reference for preventing its outbreak and spread.Methods: Based on the geographic distribution of R. japonica and H. longicornis overlapping data points and information on 56 climatic factors, we utilized the Maxent model to estimate suitable areas for R. japonica in Asian adjacent Regions and China. Model parameter adjustments and the construction of receiver operating characteristic curves were conducted using R 4.3.0 software.Average precipitation in June (prec6, 28.2%), Temperature Seasonality (bio4, 9.8%) and the minimum temperature in August (tmin8, 9.2%) contributed most to the distribution of R. japonica. The performance metrics for the Maxent model in predicting the distribution of R. japonica are as follows: the Area Under the Curve (AUC) is 0.990, the True Skill Statistic (TSS) is 0.857, and the Kappa statistic is 0.763. Under current climatic conditions, the Asian and adjacent space medium and highly suitable areas for R. japonica are estimated to be 176.78×10 4 km 2 and 95.13×10 4 km 2 , respectively. The highly suitable areas for R. japonica were mainly distributed in east and south Asia. In China, the high suitability areas are mainly distributed in the southeast coastal areas and the Qinling Mountains and Huai River cities. Under future climatic conditions, the Asian and adjacent regions maximum area change rate of R. Japonica increased by 118.65%, and that of China increased by 50.42%. Meanwhile, the suitable areas of R. japonica gradually expanding northward in China.Under global climate change, the suitable area of R. japonica is generally increasing, with a northward shift observed in China. Governments should strengthen monitoring, risk assessment, and response strategies in highly suitable regions, while also preventing the invasion of R. japonica from external source.
Keywords: Rickettsia japonica, Japanese spotted fever, Potential distribution, Maxent, Climate Change
Received: 10 Aug 2024; Accepted: 24 Feb 2025.
Copyright: © 2025 Wang, Shang, Wang, Ji, Wang and Liu. This is an open-access article distributed under the terms of the Creative Commons Attribution License (CC BY). The use, distribution or reproduction in other forums is permitted, provided the original author(s) or licensor are credited and that the original publication in this journal is cited, in accordance with accepted academic practice. No use, distribution or reproduction is permitted which does not comply with these terms.
* Correspondence:
Qiyong Liu, Chinese Center For Disease Control and Prevention, Beijing, 102206, China
Disclaimer: All claims expressed in this article are solely those of the authors and do not necessarily represent those of their affiliated organizations, or those of the publisher, the editors and the reviewers. Any product that may be evaluated in this article or claim that may be made by its manufacturer is not guaranteed or endorsed by the publisher.
Research integrity at Frontiers
Learn more about the work of our research integrity team to safeguard the quality of each article we publish.