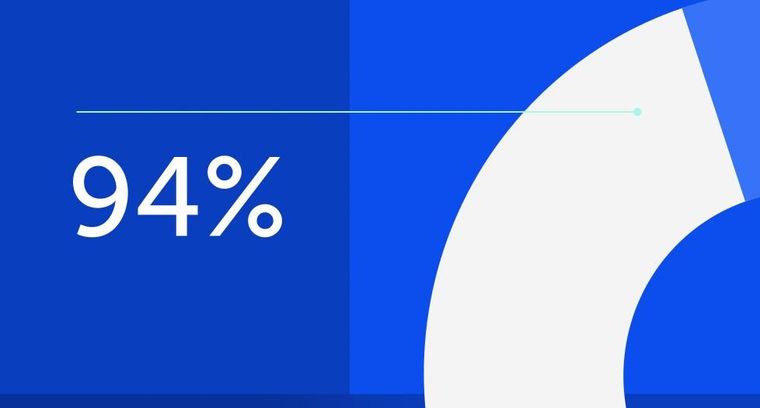
94% of researchers rate our articles as excellent or good
Learn more about the work of our research integrity team to safeguard the quality of each article we publish.
Find out more
ORIGINAL RESEARCH article
Front. Public Health, 10 April 2025
Sec. Aging and Public Health
Volume 13 - 2025 | https://doi.org/10.3389/fpubh.2025.1475951
This article is part of the Research TopicAnalyses on Health Status and Care Needs among Older AdultsView all 40 articles
Introduction: In the context of global aging, it is crucial to understand the heterogeneity, changing trends, and influencing factors of social isolation in older people.
Methods: 7198 older people over 60 in China Longitudinal Aging Social Survey in 2016 and 2018 were analyzed, using Latent Profile Analysis, Latent Transition Analysis, and multiple logistic regression analysis.
Results: The study identified five categories of social isolation among older people; the rural, widowed, with low daily living activity ability, and with low cognitive ability were more likely to belong to the social isolation category; the stability of various profiles of social isolation was strong, but the high-level social network category had the danger of changing to the medium-level social network category and the social isolation risk category; over time, those with high daily living activity ability moved into worse social isolation categories, while those with high cognitive ability moved into better categories.
Discussion: The social isolation status of the older adult had group heterogeneity and transitioned over time. The findings provide empirical evidence for taking targeted measures based on the influencing factors in older people to achieve successful aging.
1/6 people in the world will be over 60 years old by 2030 according to the World Health Organization (1). From 2020 to 2030, the number of people over 60 will increase to 1.4 billion from 1 billion. China also faces the major challenge of aging, because China has the largest older adult population in the world (2, 3). Factors such as declining fertility rates, longer lifespans, and the aging of the baby boom generation have led to an aging society (4, 5), while unprepared infrastructure such as inadequate medical facilities, poor transportation, and inadequate housing have exacerbated the challenges posed by aging (6). Although everyone is going to die, successful aging is important for alleviating the social and economic pressures of an aging population (7). Social relationships are important for both physical health (8, 9) and mental health (10, 11). They are the key factors affecting successful aging (12). Social isolation, an objective and quantifiable reflection of poor social relationships, means the reduction in social networks and lacking social connections, such as living alone, having few friends or family, and limited contact with people (13). It affects physical function, cognitive function, and mental health in older adults (14) and is associated with increased mortality (15). A study on the aged population in urban and rural China, which included 220,506 participants, found a prevalence of 36.6% of loneliness among older Chinese individuals (16). Therefore, it is important to pay attention to the change in the social isolation status of older people and then adopt targeted prevention and intervention measures to improve the quality of life and promote the successful aging of older people (17).
Magnusson and Mahoney (18) emphasize the Holistic-interaction Perspective and think that the existence of individual differences does not mean that development is disordered, and individuals with differences will partly move in different directions in the following development. Over time, more stable “types” will eventually emerge, that is, in the multi-dimensional space of psychological and behavioral development. Individuals who are brought together by a similar combination of psychological and behavioral characteristics show clearer homogeneity within the individual categories and clearer differentiation between the individual categories. Some studies have found that many interventions designed to solve the problem of social isolation in older people are not effective; an important reason is the heterogeneity of the older people with social isolation (19). Fortunately, the categorization of social isolation in the older adults has received increasing attention in Western nations. Previously, most studies classified social isolation status based on the total scores or dimension- specific scores from standardized scales, overlooking individual item responses and failing to dig into the data (20).
According to the developmental situation theory, individual development has plasticity, and individuals are affected by situational events constantly and keep continuous development and change (21). The social relationship network of older people may change with time. These observations suggest that older adults who previously belonged to one subgroup of social networks may shift to another subgroup because of changes in certain indicators. Studies have found that older people in different social relationship networks or loneliness subgroups have different transformation or stability patterns within a certain time (22, 23).
Moreover, factors such as internet penetration rate (24), social and cultural background (e.g., collectivism vs. individualism) (25), and other regional influences contribute to differences in social isolation status among older people across countries and regions (26, 27). However, research on these dynamics in China is lacking. The Chinese senior population is a unique situation that needs more research because of its particular cultural and social environment, quickly shifting demography, and rapid use of technology. Furthermore, even though many studies have explored the effects of social isolation of the older adults on depression, mortality, physical health status, and other factors (8, 28, 29), in-depth studies on the heterogeneity of social isolation of the older adults are rare, especially the large sample longitudinal tracking literature on the heterogeneity of social isolation of the older adults in China. Critical questions remain unanswered, including whether the patterns of social isolation among Chinese seniors are stable, whether they follow a unique development trajectory, and what factors affect these trajectories.
Results from cross-sectional data show that social isolation has significant differences in demographic variables (30, 31). Both males and females are strongly influenced by structural systems with different opportunities, demands, and constraints, which shape different social relationships (32). However, the effects of gender on social isolation are inconsistent (32–34); socioeconomic status (SES) determines the opportunities and ability of older persons to maintain and utilize non-family relationships (35). The influence of residence and spouse status on social isolation is more consistent: rural older people and older people without spouses are more at risk of social isolation (23, 36, 37). Recently, Sung et al. (23) explored the factors related to the change in social network types of older people and found that older, less educated, and worsening functional and mental health older people were more likely to transition into less diverse types compared with remaining in the same type. In addition, cognitive ability, and daily living activity ability in older people are closely related to social isolation (12, 38), but their influence on the transition trend of social isolation status is still lacking.
To explore the categories of social isolation among the Chinese older adults and the transition trends between these categories over time, we adopted person-centered approaches utilizing Latent Profile Analysis (LPA) and Latent Transition Analysis (LTA). In recent years, person-centered research methodologies have gained prominence by identifying similar individuals with similar feature patterns to capture diverse behavioral patterns. The aim is to trace group heterogeneity and elucidate the association between explicit variables through independent latent variables categorized by type, thereby revealing differences among individuals or developmental stages (39). Compared to traditional mean segmentation or cluster analysis, LPA offers greater accuracy, objectivity, and well-fitted models (40). LTA has advantages in capturing dynamic changes, estimating transition probabilities, flexible modeling, and supporting evaluation of intervention effects, and so on (41, 42). Given these benefits, LPA and LTA are well-suited for testing our research hypotheses.
Based on Holistic interaction Perspective, developmental situation theory and previous studies, this study proposed the following research hypotheses:
H1: There are different latent profiles of social isolation among the older adults.
H2: Individuals in the social isolation categories of the older adults will transition to other categories over time.
H3: The categories of social isolation of the older adults and the transition among categories are affected by various factors.
The data of this study was secondary data from the China Longitudinal Aging Social Survey (CLASS), which adopted a stratified and multi-stage probability sampling method. County-level regions (including counties, county-level cities, and districts) were selected as the primary sampling units, and then village/neighborhood committees were selected as the secondary sampling units. The respondents were Chinese citizens over the age of 60 from these units. The project covers 476 villages/neighborhood committees in 30 provinces/autonomous regions/municipalities across China. The survey began in 2011, followed by the first nationwide baseline survey in 2014 and two follow-up surveys in 2016 and 2018. This study selected the data from the survey in 2016 and 2018 for several reasons: first, the data in 2016 and 2018 were more contemporary than the data in 2014; second, Latent Transition Analysis (LTA) could be completed by using two waves of data; third, after matching the three waves of data, only 3,852 cases could be retained, resulting in data loss; fourth, the follow-up survey in 2016 and 2018 had further adjusted and enriched the questionnaire content on the basis of 2014, which meant that the survey content in 2016 and 2018 was more stable and consistent. A total of 11,471 data were collected in 2016, and 11,419 data were collected in 2018. Each participant was given a unique ID, which was used to match data from 2016 and 2018. We removed cases in which participants only participated in 2016 or 2018: 1,830 cases in 2016 and 1,778 cases in 2018. Also, 2,443 cases were excluded due to too many missing values on key variables, regular responses, or logical errors. Finally, the data of 7,198 older people over 60 years old was kept. Descriptive statistics for the sample are presented in Table 1.
The simplified Lubben Social Network Scale (LSNS-6) revised by Chang et al. was utilized (43–45). There were six items on the scale, divided into two dimensions: family network (3 questions) and friend network (3 questions) and were scored for six points (0 = none, 1 = 1, 2 = 2 and more, 3 = 3–4, 4 = 5–8, 5 = 9 and more). The scale has been widely used with good reliability and validity (46, 47). In CLASS, the Cronbach’s α coefficient of this scale in 2016 and 2018 were 0.871 and 0.870, respectively.
The scale developed by Lawton et al. (48) was utilized, including two subscales of basic life activity ability and instrumental daily activity ability. The basic activities included dressing, bathing, eating, taking medicine, incontinence, going to the toilet, and walking indoors; instrumental daily activities included making phone calls, taking medicine, taking transportation, shopping, cooking, and doing housework. The scale was scored on three points (1 = needn’t help from others, 2 = need some help, 3 = no ability at all). All items were scored in reverse. Cronbach’s α coefficient of this scale in 2016 and 2018 in CLASS was 0.889 and 0.918, respectively.
Referring to the Minimum Mental State Examination, MMSE (49), 16 items of the scale were divided into 4 dimensions: orientation (five questions), memory ability (3 questions), attention and calculation ability (five questions), and recall ability (3 questions). Wrong answers were worth 0 points, and correct answers were worth 1 point. The total score was 0–16 points. The scale was widely used in testing global cognitive function in research settings and clinical screening (50). Cronbach’s α coefficient of this scale in 2016 and 2018 in CLASS was 0.822 and 0.817, respectively.
SPSS 26.0 was used for data entry and descriptive statistical analysis of sociodemographic characteristics, including the mean, standard deviation, and percentages. Mplus 7 was used for latent profile analysis (LPA), latent transition analysis (LTA), and multiple logistic regression analysis. We used LPA to classify social isolation at two time points, evaluating fit using AIC, BIC, sample size-adjusted BIC (aBIC), Entropy index, likelihood ratio test index LMR-LRT (p-value), and Bootstrap-based likelihood ratio test BLRT (p-value) index. The smaller the three information evaluation indicators (AIC, BIC, and aBIC), the better the model fit. The value range of the Entropy index was 0 to 1, and the closer it was to 1, the more accurate the classification was. When the Entropy index was less than 0.60, it meant that more than 20% of individuals had a classification error. When the Entropy index reached 0.8, it indicated that the classification accuracy exceeded 90% (51). When the p-values of LMR-LRT and BLRT reached a significant level, it indicated that the model with k categories was significantly better than that with k-1 categories (52, 53).
LTA was used to calculate the probability of change for different latent profiles over time. Based on LPA, LTA without covariates was used to investigate the changing relationship between various social insolation categories at two time points. The classification results of the two-time points obtained by the LTA were not the same as the classification results of the LPA exactly at two-time points. In the LPA, the classification criteria of the two-time points were determined separately to make the fitting function of the respective model reach the minimum value. However, the category means, and variance of each dimension must be set equal across time points in LTA, so that the classes of the two-time points are comparable (53). To ensure that the five groups at the two-time points maintain the original LPA classification characteristics when running LTA, we took the LPA analysis results as the parameter setting values for LTA.
Multiple logistic regression analysis was used to explore the impact of demographic variables, daily living activity ability, and cognitive ability on the grouping of social isolation of older people. The results were dominated by the value of OR (Odd Ratio), which referred to the probability of cases belonging to the other group compared with the reference group when two groups were compared under the influence of covariates (20).
The cases that remained in the original category from T1 to T2 were taken as the reference category. The OR referred to the change ratio between the probability of the cases changing to other categories and the probability of staying in the same category now. If the OR was greater than 1, it meant that under the influence of covariates, the probability of the transition increased, and vice versa, it decreased (54, 55). We included all relevant demographic characteristics as covariates in the same model to simultaneously adjust for potential confounding effects of demographic variables.
We independently performed exploratory cross-sectional LPA with 1 to 6 profile solutions for two time points. At T1 and T2, the fit indices AIC, BIC, and aBIC for the classification of social isolation of the older people were monotonically decreasing, and the values of Entropy were all greater than 0.8. LMR-LRT and BLRT were also significant. According to these indicators synthetically, social isolation was best divided into five categories. First, Entropy was the largest value when divided into five categories. Secondly, although AIC, BIC, and aBIC have been monotonically decreasing, their speed slowed down significantly in the five categories of models. Finally, when the older people were divided into five categories, the category of friend isolation features appeared. It conformed to the classification of social isolation experience because friend isolation was a particularly important subtype of social isolation for older people (36, 56).
We named the five categories according to their characteristics in the item scores of social isolations. As shown in Figures 1, 2, the horizontal axis represented the 6 items of the Social Network Scale, and the vertical axis represented the scores on 6 items. There were four lines with roughly the same trend of balanced scores in all items from bottom to top and a special line with unbalanced scores. The first one was the bottom line, which represented the first category. In this line, the score of each item was less than 1 point, indicating that older people in this category have almost no family or friends to interact with in contacting, talking about personal affairs, or seeking help. So, we named it the social isolation category (SIC, 6.56 and 9.45% at two time points). The second line from the bottom up represented the second category. The score of each item was around 2 points, indicating that older people in this category have only about 2 family members or friends to interact with. We named it the social isolation risk category (SIRC, 30.45 and 33.57% at two time points). The scores of the third and fifth categories, represented by the top two lines, fluctuated around 3 and 4 points respectively, indicating that they have 3–4 or 5–8 family members or friends to interact with. We named them the medium-level social network category (MSNC, 49.18 and 45.32% at two time points) and the high-level social network category (HSNC, 8.91 and 7.10% at two time points), respectively. The fourth category was special; the average scores of items 1, 2, and 3 were close to 3 points, while the average scores of items 4, 5, and 6 were less than 1 point, indicating that older people in this category can interact with more family members in contacting, talking about personal matters, or seeking help, and almost no friends to interact with. We named it the friend-type social isolation category (FSIC, 4.90 and 4.57% at two time points; Table 2).
Figure 1. LPA results at T1 (2016). i1-i3 were the three items of the family network dimension of the social network scale, and i4-i6 are the three items of the friend network dimension of the social network scale. The percentage represents the proportion of different categories of older adult in all participants.
Figure 2. LPA results at T2 (2018). i1-i3 were the three items of the family network dimension of the social network scale, and i4-i6 are the three items of the friend network dimension of the social network scale. The percentage represents the proportion of different categories of older adult in all participants.
Without adding any covariates, the latent transition model was used to analyze the changes of five social isolation profiles from T1 to T2. The results are shown in Table 3. The diagonal of the transition matrix represents the probability that the subject will remain in the original potential state at two time points. The Entropy value of the model was 0.866, which demonstrated that the classification accuracy was good (57). The first 4 categories had high probability of keeping in the original groups from 2016 to 2018: 58.8, 68.8, 61.7, and 63.1%, respectively. The probability of keeping in HSNC was lower, 34.7%. As time passes, the older people of HSNC may translate into MSNC and SIRC, with 39.7 and 22.3% probability, respectively. The older people in FSIC may translate into MSNC with a 22.7% probability. The older people in MSNC may translate into SIRC with a 24.1% probability. The older people in SIRC may translate into MSNC with a 21.8% probability. The older people in SIC may translate into SIRC and MSNC with 15.3 and 19.2% probability, respectively.
Table 3. Latent transition probabilities from T1 (2016) to T2 (2018) based on the estimated latent transition model.
To explore the effects of gender, marital status, and household registration location on social isolation grouping of the older adults, multiple logistic regression analysis was carried out with SIC as the reference group, and the change ratio of the probability (Odds Ratio, OR) of the older adults belonging to other groups under the influence of covariates was obtained. In this study, gender (0 = male, 1 = female), household registration location (0 = rural, 1 = urban), and marital status (0 = widowed, 1 = non-widowed) were all classified variables. Male, agricultural household registration, and widowed older people were taken as reference groups, respectively, and which group female, urban household registration, and non-widowed older people were more likely to belong to was analyzed.
The results at T1 were shown in Table 4. Compared with males, females were more likely to be in SIRC than in SIC (OR = 1.292). Compared with the rural older people, the probabilities of the urban older people in SIRC (OR = 1.654) and in MSNC (OR = 1.339) were higher than that in SIC. Compared with widowed older people, non-widowed older people were more likely to be in SIC (OR = 0.576) than in FSIC.
The results at T2 were shown in Table 5. There was no significant difference in grouping possibilities between males and females. Compared with the rural older people, the probabilities of the urban older people in SIRC (OR = 1.448), MSNC (OR = 1.716), and HSNC (OR = 1.464) were higher than that in SIC. Compared with widowed older people, non-widowed older people were more likely to be in HSNC than in SIC (OR = 1.443).
We adopted multiple logistic regression analysis to explore the influence of aging factors on social isolation grouping, including daily living activity ability and cognitive ability.
The results on T1 were shown in Table 4. Older people with high daily living activity ability were more likely to be in SIRC (OR = 1.171), MSNC (OR = 1.250), and HSNC (OR = 1.127), and were less likely to be in FSIC (OR = 0.913), compared with in SIC.
Compared with belonging to SIC, older people with high cognitive ability were more likely to belong to SIRC (OR = 1.219), MSNC (OR = 1.204), and HSNC (OR = 1.158).
The results at T2 were shown in Table 5. Compared with belonging to SIC, the older people with high daily living activity ability were more likely to belong to SIRC (OR = 1.131), MSNC (OR = 1.153), FSIC (OR = 0.911), and HSNC (OR = 1.164).
Compared with belonging to SIC, the older people with high cognitive ability were more likely to belong to HSNC (OR = 1.210) and less likely to belong to FSIC (OR = 0.731).
The results of multiple regression analysis showed that gender, residence registration, marital status, daily living ability and cognitive ability had different effects on the classification and transformation of social isolation in the older adults.
Based on exploring the influence of various factors on the classification of social isolation of the older adults, we further explored the influence of various factors on the transition of social isolation categories of the older adults. The analysis results of the transition trend were shown in Table 6. From T1 to T2, for the older people with high daily living activity ability: compared with staying in SIC, the older people were less likely to transfer to MSNC (OR = 0.899) and FSIC (OR = 0.824); compared with staying in SIRC, the older people were less likely to change to FSIC (OR = 0.846); compared with staying in MSNC, the older people were more likely to transfer to SIC (OR = 1.113); compared with staying in FSIC, the older people were more likely to change to SIC (OR = 1.213) and SIRC (OR = 1.182).
From T1 to T2, for the older people with high cognitive ability: compared with staying in SIC, the older people were more likely to transfer to SIRC (OR = 1.176), the MSNC (OR = 1.402), and HSNC (OR = 1.317); compared with staying in SIRC, the older people were more likely to transfer to MSNC (OR = 1.192) and less likely to transfer to SIC (OR = 0.850); compared with staying in MSNC, the older people were less likely to change to SIC (OR = 0.713), SIRC (OR = 0.839), and FSIC (OR = 0.791); compared with staying in FSIC, the older people were more likely to transfer to MSNC (OR = 1.264); compared with staying in HSNC, the older people were less likely to change to SIC (OR = 0.759).
In this study, latent profile analysis and latent transition analysis were used to analyze the latent categories of social isolation of older people and their change patterns over time. Logistic regression analysis was used to explore the effects of demographic variables, daily living ability, and cognitive ability on the classification of social isolation, as well as the effects of daily living ability and cognitive ability on the transformation of social isolation of the Chinese older people.
Our study results proved that there was great heterogeneity in the social isolation states among Chinese older people, which verified hypothesis 1. The medium-level social network category had the largest population, and the next was the social isolation risk category. The population of the social isolation category and the high-level social network category were slightly different at the two time points. There was a special category for older people—the friend-type social isolation category compared with some studies (58, 59). The difference may be attributed to the measurement tools used. Another study, comparing average scores, finds that older people are more isolated from friends than from family (56), supporting the validity of this classification to some extent. Their scores on the two dimensions of family network and friend network were significantly different. It indicated that family and friends had different effects on the social isolation of older people. China’s traditional culture attaches special importance to the family concept. The Confucian socio-ethical vision “Filial piety is the first” made family support still the main way for older people in China (60). A survey finds that 85.4% of older people are willing to select home-based old-age care (61). When older people are ill or incapacitated, they are more likely to seek help from family members and decrease participation in social activities, increasing the risk of friend isolation. So, the social aspect should build perfect social places to increase social chances for older people. The family aspect should encourage and support older people to engage in social activities with friends.
Developmental situation theory suggests that individual development and change are unique, influenced by historical time, life stages, and critical events (21). Although for SIC, SIRC and MSNC categories, the probabilities of remaining in the original categories were relatively large, there were still about 40% of the older adults will change to other categories. More than 60% of those in the HSNC category moved toward worse social isolation categories. These findings support hypothesis 2.
Although the social isolation status of the older adults changed between the two time points, the MSNC and SIRC categories were the largest at both time points. At the same time, the stability of remaining in the MSNC and SIRC categories over time was relatively high, except that the high-level social network category was more likely to change to the medium-level social network category and the social isolation risk category. It suggested that once social isolation in older people was formed, it was difficult to alleviate by itself, and there was a risk of deterioration. Therefore, it was crucial to carry out targeted identification and intervention in the early stage when older people enter different degrees of social isolation. Although the transition from social isolation to a high level of social network status was rare, we found that a small number of older people made a transition to a relatively good social network status. This illustrated the dynamic nature of social isolation and once again suggests that we must take targeted measures based on the heterogeneity of social isolation in older people (19). Previous studies have found patterns of growth or stability of social networks in older people (62, 63), which may be the focus of future work of community workers and family caregivers.
The results of multiple regression analysis showed that gender, household registration, marital status, daily living ability and cognitive ability had varying effects on the classification and transition of social isolation status in the older adults, which verified research hypothesis 3. Influences of the demographic variables on latent profiles of social isolation were slightly different, but the overall trend was similar at T1 and T2. There was almost no significant difference in the likelihood between male and female older people belonging to the social isolation category and other categories. The previous contradictory research results may be due to the sample size or geographical reasons. For example, a study in Nigeria finds that widowed women are more likely to experience loneliness in later life, which is associated with local polygamy (64). Compared with the rural older people and the widowed older people, the urban older people and the non-widowed older people, respectively, showed a lower possibility of belonging to the social isolation category. It meant that the rural older people and the widowed older people have a greater risk of social isolation (65, 66).
There was an overall trend: the older people with high daily living activity ability and cognitive ability were less likely to belong to the social isolation category at T1 and T2. Older people might withdraw from social life passively because of their deteriorating health (67). So, we should pay more attention to the rural, widowed, low daily living activity ability, and low cognitive ability older people and increase their social chances and social convenience.
Both the daily living activity ability and cognitive ability are important indicators of measuring the function of older people (68, 69). They are closely related to social isolation, too. We found that they affect the transition of social isolation in diverse ways. Compared with remaining in the original category, the older people with high daily living activity ability were more likely to change to the more serious social isolation category and less likely to move to the better social network category from T1 to T2. This seemed contrary to the previous conclusion that the greater the daily living activity ability, the less likely social isolation was. However, this shifting trend illustrates the likelihood of changes in the social isolation category over time, not the relationship between the two at a time point. Maybe the reason was that older people with high daily living activity ability were less likely to need active care and help, leading to the neglect of their social communication needs by family and friends. So, older people with high daily living activity ability had a greater possibility of changing into a more serious social isolation category as time went on. It indicated that we should also pay attention to changes in the social interaction status of older people with high daily living activity ability; otherwise, it would be possible to change to a more serious social isolation group.
The older people with high cognitive ability showed an overall trend toward better social network categories compared with remaining in the original category from T1 to T2. Older people with strong cognitive ability could fully understand and use social network relationships, maintain a good social network state, and even change to a better direction. This also suggested that people should pay special attention to the protection and strengthening of older people’s cognitive ability when taking care of older people, such as carrying out effective ability training. If the older adults were physically allowed, they should insist on exercise, undertake life affairs within their capacity, use their brains appropriately, and maintain good cognitive function.
Social isolation of older people in China could be divided into five latent profiles: social isolation category, social isolation risk category, medium-level social network category, friend-type social isolation category, and high-level social network category. Rural older people and widowed older people were more likely to belong to the social isolation category compared with urban older people and non-widowed older people. Older people with high daily living activity ability and cognitive ability were less likely to belong to the social isolation category. As time went on, the social isolation of older people had a strong stability, but older people in the high-level social network category had the risk of changing to the medium-level social network category and social isolation risk category. With the development of time, older people with high daily living activity ability were more likely to change to the more serious social isolation categories, and older people with strong cognitive ability were more likely to change to the better social isolation categories.
First, only 2 waves of data separated by 2 years were used for the transition analysis of social isolation. Future studies need to explore the shifting patterns of social isolation with more wave data collection over a longer period to draw more stable and reliable conclusions. Second, the measurement indexes in this study were not rich enough: the number of family members or friends to contact, talk about personal matters, or seek help. Future studies may consider including the social networks between older people and community workers, work partners, community health care workers, etc., and consider the quality indicators of social networks. Third, the data we used was from CLASS, but it was gathered before the COVID-19 pandemic, and the pandemic changed people’s lifestyles and social networks. Therefore, further investigation of changes in the social isolation of older people during and after the pandemic will be necessary.
The data of this study came from the China Longitudinal Aging Social Survey, CLASS (http://class.ruc.edu.cn/). The data are available from the corresponding author upon reasonable request.
Ethical review and approval were not required for the study on human participants in accordance with the local legislation and institutional requirements. Written informed consent from the participants or the participants’ legal guardian/next of kin was not required to participate in this study in accordance with the national legislation and the institutional requirements. The dataset used in this study, China Longitudinal Aging Social Survey (CLASS), is publicly available (http://class.ruc.edu.cn/). The data was released with the official permission of the National Survey Research Center at Renmin University of China (NSRC).
XL: Data curation, Validation, Writing – review & editing, Writing – original draft, Resources. YzZ: Conceptualization, Data curation, Methodology, Writing – original draft, Writing – review & editing. JW: Formal analysis, Resources, Writing – review & editing. YZ: Formal analysis, Resources, Writing – review & editing. LG: Formal analysis, Resources, Writing – review & editing. BY: Conceptualization, Supervision, Validation, Writing – original draft, Writing – review & editing.
The author(s) declare that financial support was received for the research and/or publication of this article. This study was supported by National Natural Science Foundation (72164018), The Humanities and Social Sciences Program of the Ministry of Education (22YJA190012), and the Jiangxi Provincial University Humanities and Social Sciences Research Project for the Year 2024 (SZZX24192).
Special thanks to all the members of the research team, execution team, field supervisor team, sampling team, and the survey team of the China Longitudinal Aging Social Survey, CLASS. Thank them for their effective work and for providing the data. At the same time, thanks to the older people who participated in the survey.
The authors declare that the research was conducted in the absence of any commercial or financial relationships that could be construed as a potential conflict of interest.
All claims expressed in this article are solely those of the authors and do not necessarily represent those of their affiliated organizations, or those of the publisher, the editors and the reviewers. Any product that may be evaluated in this article, or claim that may be made by its manufacturer, is not guaranteed or endorsed by the publisher.
1. World Health Organization. Aging and health. (2018). Available online at: https://www.who.int/news-room/fact-sheets/detail/ageing-and-health [Accessed October 1, 2018].
2. Jiang, Q, and Feng, Q. Editorial: aging and health in China. Front Public Health. (2022) 10:998769. doi: 10.3389/fpubh.2022.998769
3. Xu, X, Zhao, Y, Zhang, X, and Xia, S. Identifying the impacts of social, economic, and environmental factors on population aging in the Yangtze River Delta using the geographical detector technique. Sustain For. (2018) 10:1528. doi: 10.3390/su10051528
4. United Nations, Department of Economic and Social Affairs, Population Division. World population ageing 2019: Highlights. New York: United Nations (2019).
5. Lee, R, and Mason, A. Fertility, human capital, and economic growth over the demographic transition. Eur J Popul. (2010) 26:159–82. doi: 10.1007/s10680-009-9186-x
6. Phillipson, C. Developing age-friendly communities: new approaches to growing old in urban environments In: Settersten R and Angel J, editors. Handbook of sociology of aging. New York, NY: Springer (2011). p. 279–93.
7. Rowe, JW, and Kahn, RL. Successful aging 2.0: conceptual expansions for the 21st century. J Gerontol: Series B. (2015) 70:593–6. doi: 10.1093/geronb/gbv025
8. Bartlo, P, and Karnes, M. Promoting physical health: older adults. In: Pizzi MA and Amir M, editors. Interprofessional perspectives for community practice. New York, NY: Routledge (2024). p. 97–120.
9. Xu, Y. Role of social relationship in predicting health in China. Soc Indic Res. (2019) 141:669–84. doi: 10.1007/s11205-017-1822-y
10. Fekete, C, Tough, H, Arora, M, Hasnan, N, Joseph, C, Popa, D, et al. Are social relationships an underestimated resource for mental health in persons experiencing physical disability? Observational evidence from 22 countries. Int J Public Health. (2021) 66:619823. doi: 10.3389/ijph.2021.619823
11. Jenkins, GT, Janich, N, Wu, S, and Shafer, M. Social isolation and mental health: evidence from adults with serious mental illness. Psychiatr Rehabil J. (2023) 46:148–55. doi: 10.1037/prj0000554
12. Miura, KW, Sekiguchi, T, Otake-Matsuura, M, Sawada, Y, Tanaka, E, Watanabe, T, et al. Patterns of social relationships among community-dwelling older adults in Japan: latent class analysis. BMC Geriatr. (2022) 22:75. doi: 10.1186/s12877-022-02748-7
13. Shankar, A, McMunn, A, Banks, J, and Steptoe, A. Loneliness, social isolation, and behavioral and biological health indicators in older adults. Health Psychol. (2011) 30:377–85. doi: 10.1037/a0022826
14. Del Pozo, CB, Perales, F, Alfonso-Rosa, RM, and Del Pozo-Cruz, J. Impact of social isolation on physical functioning among older adults: a 9-year longitudinal study of a U.S.-representative sample. Am J Prev Med. (2021) 61:158–64. doi: 10.1016/j.amepre.2021.02.003
15. Pantell, M, Rehkopf, D, Jutte, D, Syme, SL, Balmes, J, and Adler, N. Social isolation: a predictor of mortality comparable to traditional clinical risk factors. Am J Public Health. (2013) 103:2056–62. doi: 10.2105/AJPH.2013.301261
16. Wang, J, Zhou, Y, Zhang, Q, Li, J, Zhai, D, Li, J, et al. Loneliness among older Chinese individuals: the status quo and relationships with activity-related factors. BMC Geriatr. (2024) 24:42. doi: 10.1186/s12877-023-04611-9
17. Ho, M, Pullenayegum, E, and Fuller-Thomson, E. Is social participation associated with successful aging among older Canadians? Findings from the Canadian longitudinal study on aging (CLSA). IJERPH. (2023) 20:6058. doi: 10.3390/ijerph20126058
18. Magnusson, D. The holistic-interactionistic paradigm: some directions for empirical developmental research. Eur Psychol. (2001) 6:153–62. doi: 10.1027/1016-9040.6.3.153
19. Machielse, A. The heterogeneity of socially isolated older adults: a social isolation typology. J Gerontol Soc Work. (2015) 58:338–56. doi: 10.1080/01634372.2015.1007258
20. Wu, G, Zhang, M, Ni, Y, Yang, Y, Qi, C, and Wu, J. Changes in loneliness among elderly people and its effect factors: a latent transition analysis. Acta Psychol Sin. (2018) 50:1061–70. doi: 10.3724/SP.J.1041.2018.01061
21. Bühler, JL, and Nikitin, J. Sociohistorical context and adult social development: new directions for 21st century research. Am Psychol. (2020) 75:457–69. doi: 10.1037/amp0000611
22. Cheng, GH-L, Sung, P, Chan, A, Ma, S, and Malhotra, R. Transitions between social network profiles and their relation with all-cause mortality among older adults. Soc Sci Med. (2022) 292:114617. doi: 10.1016/j.socscimed.2021.114617
23. Sung, P, Malhotra, R, Cheng, GH-L, and Chan, AW-M. Transitions in social network types over time among older adults. Gerontology. (2022) 68:817–28. doi: 10.1159/000521213
24. Lin, Y, Zhu, T, Zhang, X, and Zeng, Z. Trends in the prevalence of social isolation among middle and older adults in China from 2011 to 2018: the China health and retirement longitudinal study. BMC Public Health. (2024) 24:339. doi: 10.1186/s12889-024-17734-4
25. Lykes, VA, and Kemmelmeier, M. What predicts loneliness? Cultural difference between individualistic and collectivistic societies in europe. J Cross-Cult Psychol. (2014) 45:468–90. doi: 10.1177/0022022113509881
26. Teo, RH, Cheng, WH, Cheng, LJ, Lau, Y, and Lau, ST. Global prevalence of social isolation among community-dwelling older adults: a systematic review and meta-analysis. Arch Gerontol Geriatr. (2023) 107:104904. doi: 10.1016/j.archger.2022.104904
27. Taylor, RJ, Chatters, LM, and Taylor, HO. Race and objective social isolation: older african Americans, black caribbeans, and non-hispanic whites. J Gerontol: Series B. (2019) 74:1429–40. doi: 10.1093/geronb/gby114
28. Wang, J, Zhang, WS, Jiang, CQ, Zhu, F, Jin, YL, Thomas, GN, et al. Persistence of social isolation and mortality: 10-year follow-up of the Guangzhou biobank cohort study. Psychiatry Res. (2023) 322:115110. doi: 10.1016/j.psychres.2023.115110
29. Wolters, NE, Mobach, L, Wuthrich, VM, Vonk, P, Van Der Heijde, CM, Wiers, RW, et al. Emotional and social loneliness and their unique links with social isolation, depression and anxiety. J Affect Disord. (2023) 329:207–17. doi: 10.1016/j.jad.2023.02.096
30. Cassie, KM, Miller-Cribbs, J, and Smith, A. An exploratory study of factors associated with social isolation and loneliness in a community sample. Soc Work Health Care. (2020) 59:485–98. doi: 10.1080/00981389.2020.1795780
31. Takahashi, T, Nonaka, K, Matsunaga, H, Hasebe, M, Murayama, H, Koike, T, et al. Factors relating to social isolation in urban Japanese older people: a 2-year prospective cohort study. Arch Gerontol Geriatr. (2020) 86:103936. doi: 10.1016/j.archger.2019.103936
32. Umberson, D, Lin, Z, and Cha, H. Gender and social isolation across the life course. J Health Soc Behav. (2022) 63:319–35. doi: 10.1177/00221465221109634
33. Kim, J, and Park, G-R. Cumulative exposure to social isolation and longitudinal changes in life satisfaction among older adults. Society Mental Health. (2024) 14:113–28. doi: 10.1177/21568693241232410
34. Silberzan, L, Martin, C, and Bajos, N EpiCov Study Group. Social isolation among older adults in the time of COVID-19: a gender perspective. Front Public Health. (2022) 10:840940. doi: 10.3389/fpubh.2022.840940
35. McMaughan, DJ, Oloruntoba, O, and Smith, ML. Socioeconomic status and access to healthcare: interrelated drivers for healthy aging. Front Public Health. (2020) 8:231. doi: 10.3389/fpubh.2020.00231
36. Guadalupe, S, and Vicente, HT. Types of personal social networks of older adults in Portugal. Soc Indic Res. (2022) 160:445–66. doi: 10.1007/s11205-019-02252-3
37. Henning-Smith, C, Tuttle, M, Tanem, J, Jantzi, K, Kelly, E, and Florence, LC. Social isolation and safety issues among rural older adults living alone: perspectives of meals on wheels programs. J Aging Soc Policy. (2024) 36:282–301. doi: 10.1080/08959420.2022.2081025
38. Bland, AR, Roiser, JP, Mehta, MA, Sahakian, BJ, Robbins, TW, and Elliott, R. COVID-19 induced social isolation; implications for understanding social cognition in mental health. Psychol Med. (2022) 52:3748–9. doi: 10.1017/S0033291720004006
39. Hickendorff, M, Edelsbrunner, PA, McMullen, J, Schneider, M, and Trezise, K. Informative tools for characterizing individual differences in learning: latent class, latent profile, and latent transition analysis. Learn Individ Differ. (2018) 66:4–15. doi: 10.1016/j.lindif.2017.11.001
40. Magidson, J, and Vermunt, J. Latent class models for clustering: a comparison with K-means. Canadian J Market Res. (2002) 20:36–43.
41. Collins, LM, and Lanza, ST. Latent class and latent transition analysis: With applications in the social, behavioral, and health sciences. Hoboken: John Wiley & Sons (2010).
42. Nylund-Gibson, K, and Masyn, KE. Covariates and mixture modeling: results of a simulation study exploring the impact of misspecified effects on class enumeration. Struct Equ Model Multidiscip J. (2016) 23:782–97. doi: 10.1080/10705511.2016.1221313
43. Lubben, J, Blozik, E, Gillmann, G, Iliffe, S, Von Renteln, KW, Beck, JC, et al. Performance of an abbreviated version of the Lubben social network scale among three European community-dwelling older adult populations. The Gerontologist. (2006) 46:503–13. doi: 10.1093/geront/46.4.503
44. Gray, J, Kim, J, Ciesla, JR, and Yao, P. Rasch analysis of the Lubben social network Scale-6 (LSNS-6). J Appl Gerontol. (2016) 35:508–28. doi: 10.1177/0733464814560468
45. Chang, Q, Sha, F, Chan, CH, and Yip, PSF. Validation of an abbreviated version of the Lubben social network scale (“LSNS-6”) and its associations with suicidality among older adults in China. PLoS One. (2018) 13:e0201612. doi: 10.1371/journal.pone.0201612
46. Chan, SCY, Wong, CC, Huang, QL, and Fung, CK. The psychometric properties of the Lubben social network scale (LSNS-6) and its associations with well-being indicators in Hong Kong older adults. Australas J Ageing. (2023) 42:683–9. doi: 10.1111/ajag.13214
47. Jang, Y, Powers, DA, Park, NS, Chiriboga, DA, Chi, I, and Lubben, J. Performance of an abbreviated Lubben social network scale (LSNS-6) in three ethnic groups of older Asian Americans. The Gerontologist. (2022) 62:e73–81. doi: 10.1093/geront/gnaa156
48. Lawton, M, and Brody, EM. Assessment of older people: self-maintaining and instrumental activities of daily living. Gerontologist. (1969) 9:179–86. doi: 10.1093/geront/9.3_Part_1.179
49. Folstein, MF, Folstein, SE, and McHugh, PR. “Mini-mental state” a practical method for grading the cognitive state of patients for the clinician. J Psychiatr Res. (1975) 12:189–98. doi: 10.1016/0022-3956(75)90026-6
50. Bukhbinder, AS, Hinojosa, M, Harris, K, Li, X, Farrell, CM, Shyer, M, et al. Population-based mini-mental state examination norms in adults of Mexican heritage in the Cameron County Hispanic cohort. J Alzheimers Dis. (2023) 92:1323–39. doi: 10.3233/JAD-220934
51. Lubke, G, and Muthén, BO. Performance of factor mixture models as a function of model size, covariate effects, and class-specific parameters. Struct Equ Model Multidiscip J. (2007) 14:26–47. doi: 10.1080/10705510709336735
52. Nylund, KL, Asparouhov, T, and Muthén, BO. Deciding on the number of classes in latent class analysis and growth mixture modeling: a Monte Carlo simulation study. Struct Equ Model Multidiscip J. (2007) 14:535–69. doi: 10.1080/10705510701575396
53. Wen, ZL, Xie, JY, and Wang, HH. Principles, procedures and programs of latent class models. J East China Normal University (Educ Sci). (2023) 41:1–15. doi: 10.16382/j.cnki.1000-5560.2023.01.001
54. Sorgente, A, Lanz, M, Serido, J, Tagliabue, S, and Shim, S. Latent transition analysis: guidelines and an application to emerging adults’ social development. TPM - Test Psychometrics, Methodol App Psychol. (2019) 26:39–72. doi: 10.4473/TPM26.1.3
55. Wang, JC, and Wang, XQ. Structural equation modeling: Applications using Mplus. Hoboken: John Wiley & Sons (2019). 375 p.
56. Moormann, KI, Pabst, A, Bleck, F, Löbner, M, Kaduszkiewicz, H, Van Der Leeden, C, et al. Social isolation in the oldest-old: determinants and the differential role of family and friends. Soc Psychiatry Psychiatr Epidemiol. (2024) 59:979–88. doi: 10.1007/s00127-023-02524-x
57. Wang, H, He, Y, Bian, Y, Wang, Y, and Wen, Z. Longitudinal changes in adolescent adjustment: a latent transition analysis. Curr Psychol. (2023) 42:9708–19. doi: 10.1007/s12144-021-02261-x
58. Gale, CR, Westbury, L, and Cooper, C. Social isolation and loneliness as risk factors for the progression of frailty: the English longitudinal study of ageing. Age Ageing. (2018) 47:392–7. doi: 10.1093/ageing/afx188
59. Hammond, MD, Goulden, LE, Sibley, CG, and Milfont, TL. Latent transition analysis indicates four relatively stable profiles of loneliness in New Zealand. Aust N Z J Public Health. (2022) 46:654–60. doi: 10.1111/1753-6405.13262
60. Nie, J-B. The summit of a moral pilgrimage: Confucianism on healthy ageing and social eldercare. Nurs Ethics. (2021) 28:316–26. doi: 10.1177/0969733020944446
61. Zhao, R, Zhou, H, and Zhu, J. Factors associated with willingness to choose the way for old-age care: a population-based cross-sectional study in Chongqing. China INQUIRY. (2021) 58:004695802110201. doi: 10.1177/00469580211020196
62. Cornwell, B, Goldman, A, and Laumann, EO. Homeostasis revisited: patterns of stability and rebalancing in older adults’ social lives. J Gerontol: Series B. (2021) 76:778–89. doi: 10.1093/geronb/gbaa026
63. Litwin, H, and Levinsky, M. Always alone? Network transitions among detached older Europeans and their effects. Ageing Soc. (2021) 41:2299–313. doi: 10.1017/S0144686X20000240
64. Olawa, BD, Omolayo, BO, and Azikiwe, JC. Gender influence on loneliness and family and nonfamily support among older adults: the confounding role of widowhood. J Women Aging. (2021) 33:268–87. doi: 10.1080/08952841.2019.1690370
65. Kotian, DB, Mathews, M, Parsekar, SS, Nair, S, Binu, VS, and Subba, SH. Factors associated with social isolation among the older people in India. J Geriatr Psychiatry Neurol. (2018) 31:271–8. doi: 10.1177/0891988718796338
66. Pollak, C, Verghese, J, Buchman, AS, Jin, Y, and Blumen, HM. Loneliness predicts progression of frailty in married and widowed, but not unmarried community dwelling older adults. J Frailty Aging. (2024) 13:163–71. doi: 10.14283/jfa.2024.27
67. Li, T, and Zhang, Y. Social network types and the health of older adults: exploring reciprocal associations. Soc Sci Med. (2015) 130:59–68. doi: 10.1016/j.socscimed.2015.02.007
68. Bezdicek, O, Ferreira, J, Fellows, R, and Liepelt-Scarfone, I. Editorial: activities of daily living and everyday functioning: from normal aging to neurodegenerative diseases. Front Aging Neurosci. (2023) 15:1161736. doi: 10.3389/fnagi.2023.1161736
Keywords: older people, social isolation, latent profile analysis, latent transition analysis, longitudinal study
Citation: Liu X, Zhang Y, Wu J, Zeng Y, Guo L and Ye B (2025) Latent profiles and transitions of social isolation among older adults and their influencing factors: a person-centered approach. Front. Public Health. 13:1475951. doi: 10.3389/fpubh.2025.1475951
Received: 04 August 2024; Accepted: 14 March 2025;
Published: 10 April 2025.
Edited by:
Jian Sun, Nanjing Agricultural University, ChinaReviewed by:
Sarah Dys, Portland State University, United StatesCopyright © 2025 Liu, Zhang, Wu, Zeng, Guo and Ye. This is an open-access article distributed under the terms of the Creative Commons Attribution License (CC BY). The use, distribution or reproduction in other forums is permitted, provided the original author(s) and the copyright owner(s) are credited and that the original publication in this journal is cited, in accordance with accepted academic practice. No use, distribution or reproduction is permitted which does not comply with these terms.
*Correspondence: Yanzhen Zhang, enl6MTIyQGdtYWlsLmNvbQ==; eWFuemhlekB1Y2kuZWR1; Baojuan Ye, eWViYW9qdWFuMDgwNkAxNjMuY29t
†ORCID: Xuezhi Liu, http://orcid.org/0009-0002-5572-7293
Yanzhen Zhang, http://orcid.org/0000-0001-9919-309X
Lingjing Guo, http://orcid.org/0009-0007-6079-5131
Baojuan Ye, http://orcid.org/0000-0002-1776-5742
Disclaimer: All claims expressed in this article are solely those of the authors and do not necessarily represent those of their affiliated organizations, or those of the publisher, the editors and the reviewers. Any product that may be evaluated in this article or claim that may be made by its manufacturer is not guaranteed or endorsed by the publisher.
Research integrity at Frontiers
Learn more about the work of our research integrity team to safeguard the quality of each article we publish.