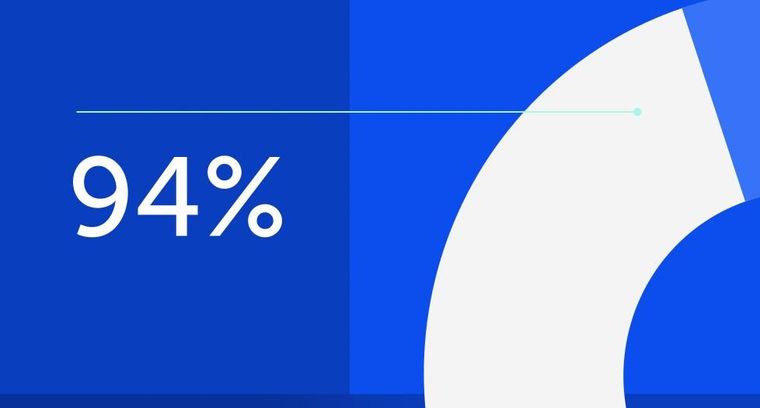
94% of researchers rate our articles as excellent or good
Learn more about the work of our research integrity team to safeguard the quality of each article we publish.
Find out more
ORIGINAL RESEARCH article
Front. Public Health
Sec. Environmental Health and Exposome
Volume 13 - 2025 | doi: 10.3389/fpubh.2025.1472398
The final, formatted version of the article will be published soon.
Select one of your emails
You have multiple emails registered with Frontiers:
Notify me on publication
Please enter your email address:
If you already have an account, please login
You don't have a Frontiers account ? You can register here
Introduction: Public health data analysis is critical to understanding disease trends. Existing analysis methods struggle with the complexity of public health data, which includes both location and time factors. Machine learning offers powerful tools but can be computationally expensive and require specialized knowledge. Dynamic mode decomposition (DMD) is an alternative that offers efficient analysis with fewer resources. This study explores applying DMD in public health using lung cancer data and compares it with other machine learning models. Methods: We analyzed lung cancer incidence data (2000-2021) from 1,013 US counties. Machine learning models (random forest, gradient boosting machine, support vector machine) were trained and optimized on the training data. We also employed time series, a linear regression model, and DMD for comparison. All models were evaluated based on their ability to predict 2021 lung cancer incidence rates. Results: The time series model achieved the lowest root mean squared error, followed by random forest. Meanwhile, DMD had an RMSE similar to that of Random Forest. Nearly all counties in Kentucky had higher lung cancer incidence rates, while states like California, New Mexico, Utah, and Idaho showed lower trends. Conclusion: In summary, DMD offers a promising alternative for public health professionals to capture underlying trends and potentially have lower computational demands compared to other machine learning models.
Keywords: machine learning, Dynamic mode decomposition, lung cancer, Public health data, random forest, gradient boosting machine
Received: 29 Jul 2024; Accepted: 09 Apr 2025.
Copyright: © 2025 Guo, Tan and Hughes. This is an open-access article distributed under the terms of the Creative Commons Attribution License (CC BY). The use, distribution or reproduction in other forums is permitted, provided the original author(s) or licensor are credited and that the original publication in this journal is cited, in accordance with accepted academic practice. No use, distribution or reproduction is permitted which does not comply with these terms.
* Correspondence: M. Courtney Hughes, Northern Illinois University, DeKalb, United States
Disclaimer: All claims expressed in this article are solely those of the authors and do not necessarily represent those of their affiliated organizations, or those of the publisher, the editors and the reviewers. Any product that may be evaluated in this article or claim that may be made by its manufacturer is not guaranteed or endorsed by the publisher.
Supplementary Material
Research integrity at Frontiers
Learn more about the work of our research integrity team to safeguard the quality of each article we publish.