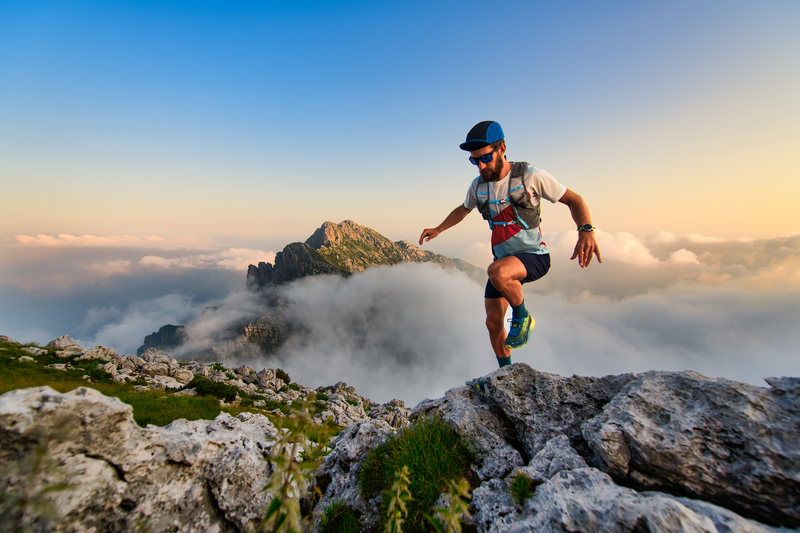
95% of researchers rate our articles as excellent or good
Learn more about the work of our research integrity team to safeguard the quality of each article we publish.
Find out more
ORIGINAL RESEARCH article
Front. Public Health
Sec. Environmental Health and Exposome
Volume 13 - 2025 | doi: 10.3389/fpubh.2025.1471490
The final, formatted version of the article will be published soon.
You have multiple emails registered with Frontiers:
Please enter your email address:
If you already have an account, please login
You don't have a Frontiers account ? You can register here
Background: Exposure to heavy metals has been implicated in adverse auditory health outcomes, yet the precise relationships between heavy metal biomarkers and hearing status remain underexplored. This study leverages a machine learning framework to investigate these associations, offering a novel approach to understanding the interplay between environmental exposures and hearing loss.Methods: We conducted a retrospective cross-sectional analysis using data from the 2012–2018 National Health and Nutrition Examination Survey (NHANES), encompassing 2,772 participants after applying exclusion criteria. Demographic, clinical, and heavy metal biomarker data (e.g., blood lead and cadmium levels) were analyzed as features, with hearing loss status—defined as a pure-tone average threshold exceeding 25 dB HL across 500, 1000, 2000, and 4000 Hz in the better ear—serving as the binary outcome. Multiple machine learning algorithms, including Random Forest, XGBoost, Gradient Boosting, Logistic Regression, CatBoost, and MLP, were optimized and evaluated. Model performance was assessed using accuracy, area under the curve (AUC), sensitivity, and specificity, while SHAP (SHapley Additive exPlanations) elucidated feature contributions.Results: The CatBoost model demonstrated the strongest performance, achieving an accuracy of 74.9% and an AUC of 0.792 on test data. Age, education level, gender, and blood levels of lead and cadmium emerged as the most significant features associated with hearing loss, as determined by SHAP analysis. These findings highlight key correlates of hearing impairment within the study population.Conclusion: This study underscores the utility of a machine learning framework in identifying associations between heavy metal biomarkers and hearing loss in a nationally representative sample. While not designed to forecast hearing loss over time, our findings suggest potential clinical relevance for identifying individuals with elevated heavy metal exposure who may warrant further audiometric evaluation. This work lays a foundation for future longitudinal studies to explore these relationships more comprehensively.
Keywords: Hearing Loss, heavy metals, machine learning, Association, risk
Received: 27 Jul 2024; Accepted: 31 Mar 2025.
Copyright: © 2025 Nabavi, Kashkooli, Nabavizadeh and Safari. This is an open-access article distributed under the terms of the Creative Commons Attribution License (CC BY). The use, distribution or reproduction in other forums is permitted, provided the original author(s) or licensor are credited and that the original publication in this journal is cited, in accordance with accepted academic practice. No use, distribution or reproduction is permitted which does not comply with these terms.
* Correspondence:
Farimah Safari, Student Research Committee, Shiraz University of Medical Sciences, Shiraz, Fars, Iran
Disclaimer: All claims expressed in this article are solely those of the authors and do not necessarily represent those of their affiliated organizations, or those of the publisher, the editors and the reviewers. Any product that may be evaluated in this article or claim that may be made by its manufacturer is not guaranteed or endorsed by the publisher.
Research integrity at Frontiers
Learn more about the work of our research integrity team to safeguard the quality of each article we publish.