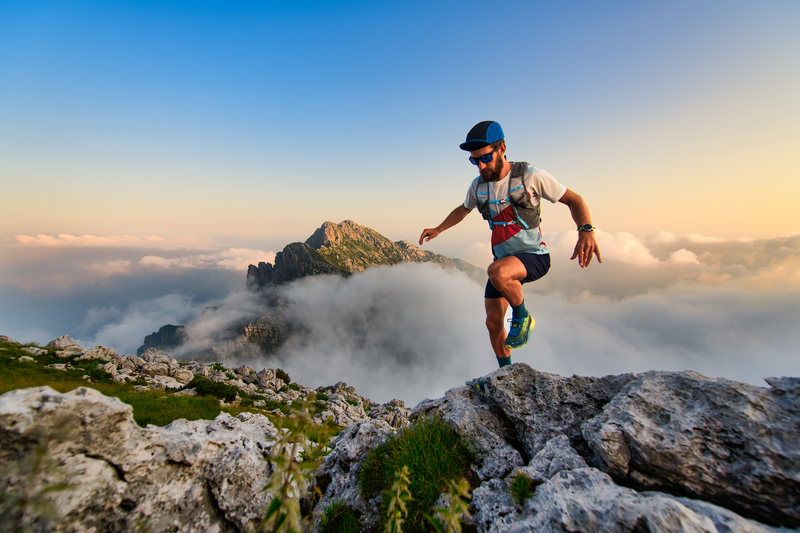
95% of researchers rate our articles as excellent or good
Learn more about the work of our research integrity team to safeguard the quality of each article we publish.
Find out more
ORIGINAL RESEARCH article
Front. Public Health
Sec. Aging and Public Health
Volume 13 - 2025 | doi: 10.3389/fpubh.2025.1437213
This article is part of the Research Topic Machine Learning and Deep Learning in Data Analytics and Predictive Analytics of Physiological Data View all 4 articles
The final, formatted version of the article will be published soon.
You have multiple emails registered with Frontiers:
Please enter your email address:
If you already have an account, please login
You don't have a Frontiers account ? You can register here
Bayesian networks (BNs) are an excellent machine learning algorithm for extensively exploring the influencing factors associated with many diseases. However, few researchers have used BNs to examine the influencing factors associated with arthritis in elderly people in the Chinese community. Our aim has been to use BNs to construct a complex network of relationships between arthritis and its related influencing factors and to predict arthritis through Bayesian inference, thereby providing scientific references for its control and prevention. Data were downloaded from the 2015 China Health and Retirement Longitudinal Study (CHARLS) online database, a longitudinal survey of the middle-aged and elderly population in China. Twenty-two variables such as smoking, depressive symptoms, age, and joint pain were included in this study. First, Elastic Networks (EN) were used to screen for features closely associated with arthritis, and we subsequently incorporated these features into the construction of the BN model. We performed structural learning of the BN based on the taboo algorithm and used the maximum likelihood method for parameter learning of the BN. In total, 15764 participants were enrolled in this study, which included 5076 patients with arthritis. EN identified 13 factors strongly associated with arthritis. The BN consisted of 14 nodes and 24 directed edges. Among them, depressive symptoms and age were direct influences on arthritis, whereas gender was an indirect influence on the diseases. BN graphically visualized the complex network of relationships between arthritis and its influences and predicted the development of arthritis through Bayesian inference. These results were in line with clinical practice. BNs thus have a wide range of application prospects.
Keywords: bayesian networks, Elastic networks, Arthritis, prediction, Influencing factors
Received: 23 May 2024; Accepted: 17 Mar 2025.
Copyright: © 2025 Zhong, Li, Hu, Hu, Jin, Xie, Hu and Ma. This is an open-access article distributed under the terms of the Creative Commons Attribution License (CC BY). The use, distribution or reproduction in other forums is permitted, provided the original author(s) or licensor are credited and that the original publication in this journal is cited, in accordance with accepted academic practice. No use, distribution or reproduction is permitted which does not comply with these terms.
* Correspondence:
Dailun Hu, Hebei Medical University, Shijiazhuang, China
Bin Ma, Murdoch University, Perth, 6150, Western Australia, Australia
Disclaimer: All claims expressed in this article are solely those of the authors and do not necessarily represent those of their affiliated organizations, or those of the publisher, the editors and the reviewers. Any product that may be evaluated in this article or claim that may be made by its manufacturer is not guaranteed or endorsed by the publisher.
Research integrity at Frontiers
Learn more about the work of our research integrity team to safeguard the quality of each article we publish.