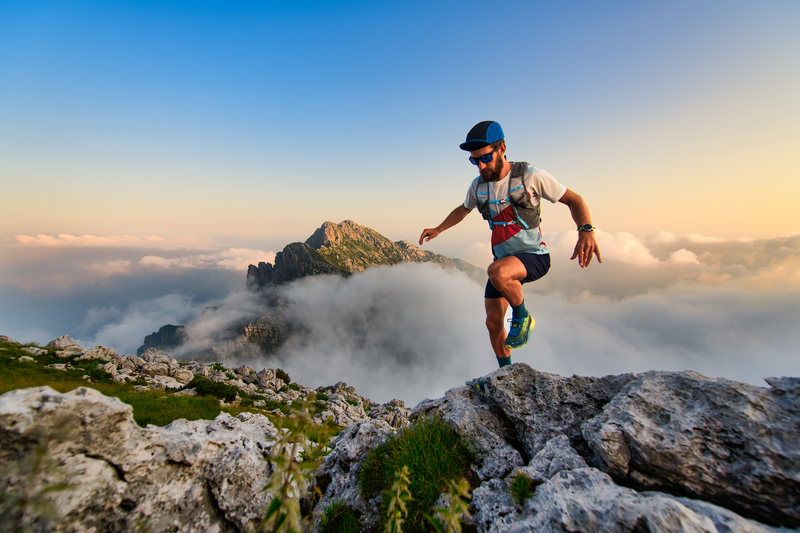
94% of researchers rate our articles as excellent or good
Learn more about the work of our research integrity team to safeguard the quality of each article we publish.
Find out more
ORIGINAL RESEARCH article
Front. Public Health
Sec. Public Mental Health
Volume 13 - 2025 | doi: 10.3389/fpubh.2025.1406062
This article is part of the Research Topic Cognitive and Mental Health Improvement Under- and Post-COVID-19 - Volume III View all 11 articles
The final, formatted version of the article will be published soon.
You have multiple emails registered with Frontiers:
Please enter your email address:
If you already have an account, please login
You don't have a Frontiers account ? You can register here
The factors associated with sleep quality among medical personnel providing support on the frontline during the height of the COVID-19 pandemic remain unclear, and appropriate predictive and screening tools are lacking.This study was designed and conducted to investigate whether factors such as weight change, job title, and tea consumption influence the sleep quality of these workers.Additionally,The study aims to develop predictive models to analyze the sleep problems experienced by healthcare workers during periods of epidemic instability, and to provide relevant data and tools to support effective intervention and prevention strategies. A cross-sectional study was conducted from June 25 to July 14, 2022, using a self-administered general information questionnaire and the PSQI to investigate the sleep quality of medical personnel providing aid in Shanghai. The relevant influencing factors were obtained via univariate analysis and multivariate stepwise logistic regression analysis, and 80% of the data were used in the training-test set (n=1,060) and 20% were used in the independent validation set (n=266). We used snowball sampling to establish the six models of logistics (LG), deep learning (DL), naïve Bayes (NB), artificial neural networks (ANN), random forest (RF), and gradient-boosted trees (GBT) and perform model testing. Among the participants, 75.8% were female. Those under 35 years of age comprised 53.7% of the medical staff, while those over 35 years accounted for 46.3%. The educational background of the participants included 402 individuals with an associate degree (30.3%), 713 with a bachelor's degree (53.8%), and 211 with a master's degree or higher (15.9%).Weight, job title, and tea consumption during the aid period were the main factors influencing the sleep quality of medical personnel during the aid period. The areas under the curve (AUC) of LG, DL, NB, ANN, RF, and GBT were 0.645, 0.656, 0.626, 0.640, 0.551, and 0.582, respectively. The DL model has the best prediction performance (specificity = 86.1%, sensitivity= 45.5%) of all the models. During the height of the COVID-19 pandemic, the sleep quality of frontline medical personnel providing aid in Shanghai was influenced by multiple factors,and the DL model was found to have the strongest overall predictive efficacy for sleep quality.
Keywords: sleep quality, medical personnel, COVID-19, machine learning, Deep Learning Sleep quality, deep learning
Received: 24 Mar 2024; Accepted: 05 Mar 2025.
Copyright: © 2025 Huang, Chen, Qiu, Dai, Yao, Zhuang, Wu, Zeng, Fan and Zhang. This is an open-access article distributed under the terms of the Creative Commons Attribution License (CC BY). The use, distribution or reproduction in other forums is permitted, provided the original author(s) or licensor are credited and that the original publication in this journal is cited, in accordance with accepted academic practice. No use, distribution or reproduction is permitted which does not comply with these terms.
* Correspondence:
Yixiang Zhang, The Second Affiliated Hospital of Fujian Medical University, Quanzhou, 362000, Fujian Province, China
Disclaimer: All claims expressed in this article are solely those of the authors and do not necessarily represent those of their affiliated organizations, or those of the publisher, the editors and the reviewers. Any product that may be evaluated in this article or claim that may be made by its manufacturer is not guaranteed or endorsed by the publisher.
Research integrity at Frontiers
Learn more about the work of our research integrity team to safeguard the quality of each article we publish.