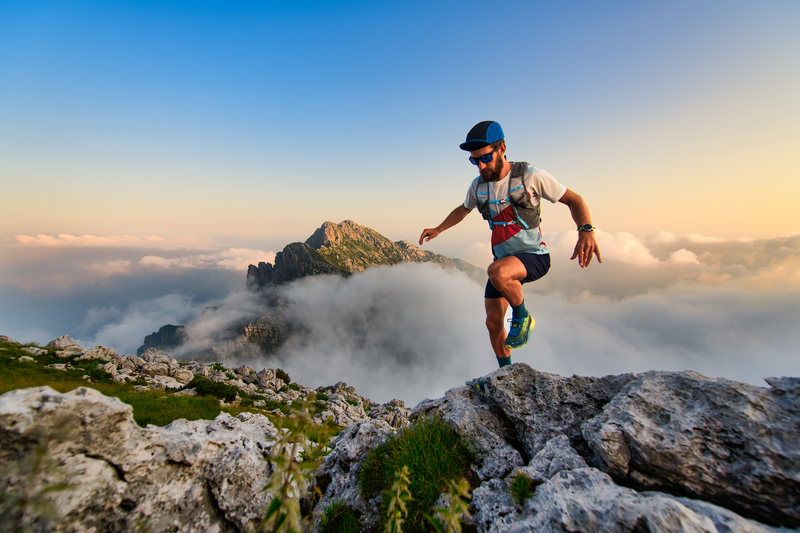
95% of researchers rate our articles as excellent or good
Learn more about the work of our research integrity team to safeguard the quality of each article we publish.
Find out more
ORIGINAL RESEARCH article
Front. Public Health , 16 January 2025
Sec. Infectious Diseases: Epidemiology and Prevention
Volume 12 - 2024 | https://doi.org/10.3389/fpubh.2024.1515871
Introduction: Public health messaging is crucial for promoting beneficial health outcomes, and the latest advancements in artificial intelligence offer new opportunities in this field. This study aimed to evaluate the effectiveness of ChatGPT-4 in generating pro-vaccine messages on different topics for Human Papillomavirus (HPV) vaccination.
Methods: In this study (N = 60), we examined the persuasive effect of pro-vaccine messages generated by GPT-4 and humans, which were constructed based on 17 factors impacting HPV vaccination. Paired-samples t-tests were used to compare the persuasiveness of these messages.
Results: GPT-generated messages were reported as more persuasive than human-generated messages on some influencing factors (e.g., untoward effect, stigmatized perception). Human-generated messages performed better on the message regarding convenience of vaccination.
Discussion: This study provides evidence for the viability of ChatGPT, in generating persuasive pro-vaccine messages to influence people’s vaccine attitudes. It is indicated that the feasibility and efficiency of using AI for public health communication.
Public health messaging is critical in promoting beneficial public health outcomes. Effective public health campaigns hold significant societal importance, as they nudge people to make better decisions for themselves and their communities (1, 2). Public health messaging research focuses on composing messages that deliver crucial information to remind individuals to adopt behaviors conducive to health (3).
In recent years, Artificial Intelligence (AI) has made significant achievement for generating information. Previous research has explored AI’s impact on health information-seeking behaviors (4), as well as the ethical implications of AI and data in public health communication and persuasion (5, 6). With the development of Large Language Models (LLMs), it has demonstrated powerful generative capabilities, and has achieved notable success in content creation across various contexts, including the public health domain (7, 8). ChatGPT developed by OpenAI is one of such LLMs. Within just two months of its launch, it surpassed 100 million users and reached over 1.5 billion monthly visits. It is necessary to explore the applications of ChatGPT in public health messaging.
Currently, the potential benefit to use LLMs in vaccination has been reported. Deiana et al. (9) found that ChatGPT can provide accurate information in a conversational way, addressing common myths and misconceptions about vaccination (9). Karinshak et al. (8) conducted a systematic evaluation of GPT-3’s ability to generate COVID-19 pro-vaccine messages, found that AI can create effective public health messages under human supervision (8). In addition, Kim et al. (10) found that ChatGPT can utilize content from social media to analyze public opinions on vaccination (10).
Cervical cancer, as one of the major global health concerns, poses a significant threat to the public health (11). Despite getting vaccinated against HPV (Human Papillomavirus) is an important method to prevent cervical cancer (12), HPV vaccination hesitancy and low vaccination rates still persist. A systematic review suggests that only 15–31% of respondents have even heard of HPV, while only 0.6–11% know that HPV is a risk factor for cervical cancer (13). Moreover, the abundance of misinformation about vaccination on the internet has led to a decline in public acceptance and trust in vaccinations, presenting significant challenges for the promotion of HPV vaccination (14, 15).
Currently, less researches have been conducted for evaluating GPT-4’s performance to generate pro-vaccine messages for HPV. Although prior studies have shown that AI can support human decision-making and persuasion (16, 17), including in communication tasks within high risk areas such as public health (18, 19), the generation capability of pro-vaccine messages on different topics remains unclear. Previous research has identified 17 influencing factors that individuals may take into account when deciding whether to get HPV vaccine (20), providing an ideal framework for generating HPV vaccination information. In this study, we use this framework to construct pro-vaccine messages according to these influencing factors, and explore the differences in persuasiveness between ChatGPT and human generated HPV pro-vaccine messages. We propose the following hypothesis:
H: ChatGPT generated HPV pro-vaccine messages are more persuasive than human on some influencing factors.
According to G⁎Power, we would need 45 participants when conducting paired samples t-test. In this study, a total of 60 undergraduate and graduate students were finally recruited, with an average age of 23.03 (SD ± 2.22). Our study has received ethical approval from the Institutional Review Board of the Institute of Psychology, Chinese Academy of Sciences with the ethics approval number H23089. All participants in the study were adults and signed a consent form after being fully informed.
A questionnaire was developed in this study to measure the persuasiveness of messages generated according to 17 influencing factors. These factors were derived from a study by Song and colleagues on determinants of HPV vaccination decision among young Chinese women (20). Through semi-structured interviews, they identified 17 key factors influencing audiences’ vaccination decisions (e.g., vaccine safety, untoward effect) (see Table 1). For each message, we added the item measuring the perceived persuasiveness, “I think this message is persuasive in promoting the HPV vaccination” (1 = Strongly disagree, 7 = Strongly agree). The whole procedure is depicted in Figure 1.
Figure 1. The process of questionnaire development. *The prompt used in this study: “Please craft a persuasive vaccine message, based on the following influencing factor and its operational definitions, to encourage individuals to get the HPV vaccine, in no more than 100 words”.
All experimental procedures were conducted with the informed consent of the participants. Participants read the pro-vaccine messages at first, which were presented in a randomized order, and reported their perceived persuasiveness. Then, to examine differences in the persuasiveness of human-authored, GPT-generated pro-vaccine messages and baseline messages, we used paired-samples t-tests. All of these analyses were conducted using SPSS 27.
The analysis of the persuasiveness reported in Table 2 shows that differences between the GPT-generated, human-generated and baseline messages are significant on several influencing factors. Specifically, the persuasiveness of GPT-generated messages was significantly higher for the messages about negative cue (e.g., untoward effect, stigmatized perception) and group behavior (e.g., high standard group, conformity) compared to human-authored. This indicates that GPT-generated messages are more persuasive compared to human-authored ones. The persuasiveness of the practical information (e.g., convenience of vaccination) messages generated by GPT-4 was significantly lower than human-authored. It suggests that the messages constructed based on convenience of vaccination, human-generated messages are more persuasive than GPT-generated. In addition, the results of comparing of the GPT-generated and human-generated messages with baseline messages shows that both GPT and human generated messages were more persuasive than the baseline messages on most influencing factors. However, for messages related to the objective (e.g., unofficial position, scientific principle and dual role persuasion), the baseline messages were significantly more persuasive than both GPT and human generated.
Table 2. The persuasiveness comparison of human-generated messages, GPT-generated messages and baseline messages.
This study compared the performance of creating persuasive pro-vaccine messages by 17 influencing factors between GPT-4 and human-authored. The results showed that GPT-generated messages regarding untoward effect, stigmatizing perception, high-standard group, and conformity were significantly more persuasive. This study highlights the potential value of using LLMs in public health communication and demonstrates that models such as GPT-4 can be useful tools for augmenting content generation.
GPT-4 outperformed humans in decreasing public concern about vaccine side effects and correcting misconceptions about HPV vaccines. Vaccine side effects are always a crucial issue of public concern (21), while stigmatized views of HPV also contribute to vaccine hesitancy (22). Prior study showed that ChatGPT generates longer and more diverse responses when given prompts containing negative content (23). For HPV vaccination, The negative cues (e.g., untoward effect, stigmatized perception) prompt GPT generate more detailed explanations, enhancing the messages’ persuasiveness. Although GPT has exhibited limitations concerning the interpretation of cultural context (24), since GPT-4 was trained on trillions of words from the internet, it can capture the characteristics of public language and generate messages that align with social norms and public perceptions (7, 25). As a result, GPT-generated messages showed greater persuasiveness regarding the messages about group behavior (e.g., high standard group, conformity) too.
In addition, GPT-generated convenience of vaccination messages showed lower persuasive than human generated. Since ChatGPT uses different linguistic devices than humans (26),and AI’s language strategies may not align with human preferences in specific areas. Readers may expect concise and relatable language when discussing the convenience of vaccination. GPT-4 too formal or lack contextual adaptability (27), which diminishes the emotional connection and persuasiveness of the messages.
However, the persuasiveness of pro-vaccine messages may be reduced when they have an obvious persuasive intent on certain topics. In our study, some messages (e.g., unofficial position, dual role persuasion and scientific principle) require authenticity and objectivity of viewpoint. If we construct such messages around a specific purpose, they may appear too deliberate and official, which diminishes public trust.
Our findings could bring some inspirations to the persuading practices in vaccination promotion or even broader field. The present results provide evidence for the viability of LLMs, particularly ChatGPT, in generating persuasive pro-vaccine messages to influence people’s vaccine attitudes. GPT-4’s performance in comparison to human-authored content reveals that LLMs have the potential to support public health program. With the unique ability to draw upon massive amounts of textual data, LLMs are able to synthesize extensive, diverse sources in content generation almost instantly in contrast to existing workflows, which require extensive, expensive consumer research processes. GPT-generated messages will lead to higher levels of persuasiveness and better express the benefits of vaccination on certain topics (e.g., untoward effect); thus, they will promote the intention to get the COVID-19 vaccine. Although prompt development can take a significant amount of time, once effective prompts have been identified, GPT-4 can easily generate a large number of unique messages. Our results suggests that AI has the potential to contribute to public health communication workflows to effectively and efficiently develop strategic communication campaigns. In addition, we intentionally used structured prompts, such structured prompts can still serve as a useful reference for human experts, helping them generate more effective messages.
The current study also has several limitations. First, our study was conducted in China, and the results might be applicable in China only. People should use the results of this study with caution in the context of different cultural backgrounds. Our study focused on the perspective of undergraduate and graduate. Little is known about whether these results found in this study would work well for the other groups. Future study should further investigate on difference population. In addition, the primary focus of this experiment was to examine the persuasiveness of the messages, but one single question may not be sufficient to fully assess their impact, and it is ideal to examine the audiences’ behavioral changes (e.g., willingness to vaccinate) after exposure to specific types of information.
The results of this study suggest that GPT-4 has the potential to effectively generate persuasive pro-vaccine messages, such as HPV vaccination. GPT-generated pro-vaccine messages constructed around untoward effect, stigmatizing perception, high-standard group, and conformity showed significantly higher persuasiveness than human generated. But the convenience of vaccination message was lower. It suggests that GPT-4 can be adopted to mediate public health messaging by quickly generating message drafts and the appropriate call to action, with human oversight. This study demonstrates the feasibility and efficiency of using LLMs for public health communication, contributing to the field of AI application.
The original contributions presented in the study are included in the article/supplementary material, further inquiries can be directed to the corresponding author.
The studies involving humans were approved by the Institutional Review Board of the Institute of Psychology, Chinese Academy of Sciences. The studies were conducted in accordance with the local legislation and institutional requirements. The participants provided their written informed consent to participate in this study. Written informed consent was obtained from the individual(s) for the publication of any potentially identifiable images or data included in this article.
DX: Writing – original draft, Conceptualization, Data curation, Methodology, Writing – review & editing. MS: Conceptualization, Methodology, Writing – review & editing. TZ: Conceptualization, Funding acquisition, Methodology, Project administration, Supervision, Writing – review & editing.
The author(s) declare that financial support was received for the research, authorship, and/or publication of this article. We are grateful for the financial support from the Beijing Natural Science Foundation [No. IS23088].
The authors declare that the research was conducted in the absence of any commercial or financial relationships that could be construed as a potential conflict of interest.
The authors declare that no Generative AI was used in the creation of this manuscript.
All claims expressed in this article are solely those of the authors and do not necessarily represent those of their affiliated organizations, or those of the publisher, the editors and the reviewers. Any product that may be evaluated in this article, or claim that may be made by its manufacturer, is not guaranteed or endorsed by the publisher.
1. Farrelly, MC, Nonnemaker, J, Davis, KC, and Hussin, A. The influence of the national truth campaign on smoking initiation. Am J Prev Med. (2009) 36:379–84. doi: 10.1016/j.amepre.2009.01.019
2. Pirkis, J, Rossetto, A, Nicholas, A, Ftanou, M, Robinson, J, and Reavley, N. Suicide prevention media campaigns: a systematic literature review. Health Commun. (2019) 34:402–14. doi: 10.1080/10410236.2017.1405484
3. Maibach, E., and Parrott, R. (1995). Designing health messages: Approaches from communication theory and public health practice.
4. Ankenbauer, S. A., and Lu, AJ. Navigating the “glimmer of Hope”: Challenges and resilience among U.S. older adults in seeking COVID-19 vaccination. CSCW’21 Companion: Companion Publication of the 2021 Conference on Computer Supported Cooperative Work and Social Computing, (2021) 10–13. doi: 10.1145/3462204.3481768
5. Diaz, M. Algorithmic technologies and underrepresented populations Companion Publication of the 2019 Conference on Computer Supported Cooperative Work and Social Computing. Association for Computing Machinery. (2019) 47–51. doi: 10.1145/3311957.3361857
6. Nova, F. F., Saha, P., Shafi, M. S. R., and Guha, S. (2019). Sharing of public harassment experiences on social Media in Bangladesh.
7. Brown, TB, Mann, B, Ryder, N, Subbiah, M, Kaplan, J, Dhariwal, P, et al. Language models are few-shot learners. NIPS20: Proceedings of the 34th International Conference on Neural Information Processing Systems. (2020) 159:1877–901. doi: 10.48550/arXiv.2005.14165
8. Karinshak, E, Liu, SX, Park, JS, and Hancock, JT. Working with AI to persuade: examining a large language Model's ability to generate pro-vaccination messages. Proceedings of the ACM on Human-Computer Interaction. 7:1–29. doi: 10.1145/3579592
9. Deiana, G, Dettori, M, Arghittu, A, Azara, A, Gabutti, G, and Castiglia, P. Artificial intelligence and public health. Evaluating ChatGPT Responses to Vaccination Myths and Misconceptions. (2023) 11:1217. doi: 10.3390/vaccines11071217
10. Kim, S, Kim, K, and Wonjeong Jo, C. Accuracy of a large language model in distinguishing anti- and pro-vaccination messages on social media: the case of human papillomavirus vaccination. Prev Med Rep. (2024) 42:102723. doi: 10.1016/j.pmedr.2024.102723
11. Bray, F, Ferlay, J, Soerjomataram, I, Siegel, RL, Torre, LA, and Jemal, A. Global cancer statistics 2018: GLOBOCAN estimates of incidence and mortality worldwide for 36 cancers in 185 countries. CA Cancer J Clin. (2018) 68:394–424. doi: 10.3322/caac.21492
12. Lei, J, Ploner, A, Elfström, KM, Wang, J, Roth, A, Fang, F, et al. HPV vaccination and the risk of invasive cervical Cancer. New England Journal of Medicine. (2020) 383:1340–8. doi: 10.1056/NEJMoa1917338
13. Klug, SJ, Hukelmann, M, and Blettner, M. Knowledge about infection with human papillomavirus: a systematic review. Prev Med. (2008) 46:87–98. doi: 10.1016/j.ypmed.2007.09.003
14. Nguyen, HB, Nguyen, THM, Vo, THN, Vo, TCN, Nguyen, DNQ, Nguyen, H-T, et al. Post-traumatic stress disorder, anxiety, depression and related factors among COVID-19 patients during the fourth wave of the pandemic in Vietnam. Int Health. (2023) 15:365–75. doi: 10.1093/inthealth/ihac040
15. Wong, LP, Wong, P-F, Megat Hashim, MMAA, Han, L, Lin, Y, Hu, Z, et al. Multidimensional social and cultural norms influencing HPV vaccine hesitancy in Asia. Hum Vaccin Immunother. (2020) 16:1611–22. doi: 10.1080/21645515.2020.1756670
16. Jakesch, M., French, M., Ma, X., Hancock, J. T., and Naaman, M. (2019). AI-mediated communication: How the perception that profile text was written by AI affects trustworthiness.
17. Kim, TW, and Duhachek, A. Artificial intelligence and persuasion: a construal-level account (2020) 31:363–80. doi: 10.1177/0956797620904985
18. Duerr, S, and Gloor, PAJA. Persuasive natural language generation - a literature. Review. Artificial Intelligence-arXiv. (2021). doi: 10.48550/arXiv.2101.05786
19. Shin, D, Park, S, Kim, EH, Kim, S, Seo, J, and Hong, H. Exploring the effects of AI-assisted emotional support processes in online mental health community extended abstracts of the 2022 CHI conference on human factors in computing systems. New Orleans, LA, USA: (2022).
20. Song, M, and Zhu, T. Investigation and evaluation of influencing factors of HPV vaccination intention in young Chinese women. ChinaXiv. (2024). doi: 10.12074/202402.00261V1
21. Finale, E, Leonardi, G, Auletta, G, Amadori, R, Saglietti, C, Pagani, L, et al. MMR vaccine in the postpartum does not expose seronegative women to untoward effects. Ann Ist Super Sanita. (2017) 53:152–6. doi: 10.4415/ann_17_02_12
22. Bennett, KF, Waller, J, Ryan, M, Bailey, JV, and Marlow, LAV. Concerns about disclosing a high-risk cervical human papillomavirus (HPV) infection to a sexual partner: a systematic review and thematic synthesis. BMJ Sex Reprod Health. (2020) 47:17–26. doi: 10.1136/bmjsrh-2019-200503
23. Zhao, W, Zhao, Y, Lu, X, Wang, S, Tong, Y, and Qin, BJA. Is ChatGPT equipped with emotional dialogue capabilities? (2023) Cornell University - arXiv. doi: 10.48550/arXiv.2304.09582
24. McIntosh, TR, Liu, T, Susnjak, T, Watters, P, and Halgamuge, M. A reasoning and value alignment test to assess advanced GPT reasoning (2024) 14:1–37. doi: 10.1145/3670691,
25. Clark, E, August, T, Serrano, S, Haduong, N, Gururangan, S, and Smith, N. All Thats Human Is Not Gold: Evaluating Human Evaluation of Generated Text. Association for Computational Linguistics, (2021) 1:7282–96. doi: 10.18653/v1/2021.acl-long.565
26. Guo, B., Zhang, X., Wang, Z., Jiang, M., Nie, J., et al. (2023). How close is ChatGPT to human experts? Comparison Corpus, evaluation, and detection.
Keywords: large language models, AI-mediated communication, persuasion, public health messages, message factors
Citation: Xia D, Song M and Zhu T (2025) A comparison of the persuasiveness of human and ChatGPT generated pro-vaccine messages for HPV. Front. Public Health. 12:1515871. doi: 10.3389/fpubh.2024.1515871
Received: 23 October 2024; Accepted: 30 December 2024;
Published: 16 January 2025.
Edited by:
Shahab Saquib Sohail, VIT Bhopal University, IndiaReviewed by:
Kamuran Özdil, Nevsehir University, TürkiyeCopyright © 2025 Xia, Song and Zhu. This is an open-access article distributed under the terms of the Creative Commons Attribution License (CC BY). The use, distribution or reproduction in other forums is permitted, provided the original author(s) and the copyright owner(s) are credited and that the original publication in this journal is cited, in accordance with accepted academic practice. No use, distribution or reproduction is permitted which does not comply with these terms.
*Correspondence: Tingshao Zhu, dHN6aHVAcHN5Y2guYWMuY24=
Disclaimer: All claims expressed in this article are solely those of the authors and do not necessarily represent those of their affiliated organizations, or those of the publisher, the editors and the reviewers. Any product that may be evaluated in this article or claim that may be made by its manufacturer is not guaranteed or endorsed by the publisher.
Research integrity at Frontiers
Learn more about the work of our research integrity team to safeguard the quality of each article we publish.