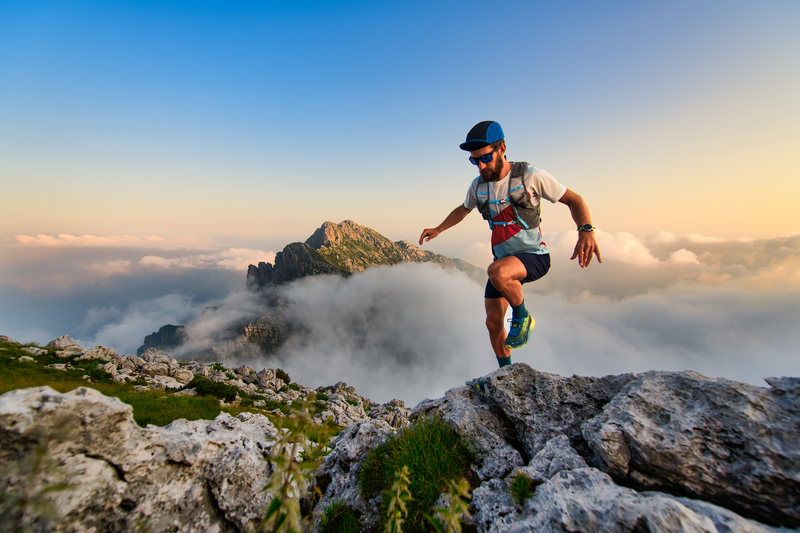
95% of researchers rate our articles as excellent or good
Learn more about the work of our research integrity team to safeguard the quality of each article we publish.
Find out more
ORIGINAL RESEARCH article
Front. Public Health , 18 December 2024
Sec. Infectious Diseases: Epidemiology and Prevention
Volume 12 - 2024 | https://doi.org/10.3389/fpubh.2024.1474855
Background: HFMD is a common infectious disease that is prevalent worldwide. In many provinces in China, there have been outbreaks and epidemics of whooping cough, posing a threat to public health.
Purpose: It is crucial to grasp the epidemiological characteristics of HFMD in Quzhou and establish a prediction model for HFMD to lay the foundation for early warning of HFMD.
Method: Descriptive epidemiological methods were used to analyze the epidemic characteristics of HFMD, the incidence map was drawn by ArcGIS software, the Seasonal Auto Regressive Integrated Moving Average (SARIMA) and Prophet model were established by R software. Then, root mean square error (RMSE) and mean absolute error (MAE) were used to evaluate the fitting and prediction performances of the model.
Results: From 2010 to 2023, Quzhou City reported a total of 66,601 cases of hand, foot, and mouth disease (HFMD), with the annual number of reported cases fluctuating between 2,265 and 7,964. The average annual incidence rate was 216.88/100,000, with the lowest in 2010 (97.08 /100,000) and the highest in 2016 (373.37/100,000) (Ptrend < 0.001). The cases exhibited a seasonal bimodal distribution, with the first peak occurring from April to July and the second peak from October to December. The incidence rate of HFMD in males (246.71/100,000) was higher than that in females (185.81 /100,000). The performance of the SARIMA (1,0,1)(2,1,0)12 model was better than that of the Prophet model in terms of prediction accuracy.
Conclusion: The incidence of hand, foot and mouth disease in Quzhou is on the rise in 2010–2016 and 2022–2023. In this study, the SARIMA prediction model was compared with the FB Prophet model. Data for more years can then be observed to better predict trends in the incidence of HFMD, providing a basis for prevention strategies and resource allocation. Further research can optimize the model to enhance predictive ability to improve the understanding and management of Chinese rival foot and mouth disease.
HFMD (hand, foot and mouth disease) is a common infectious disease in children caused by enteroviruses. HFMD is prevalent worldwide (1), Since the 1990s, HFMD has resulted in thousands of deaths in the Asia-Pacific region (2). HFMD is prevalent in China (3), with outbreaks or epidemics reported in various provinces (4, 5). In May 2008, HFMD was included in the management reporting of Category C infectious diseases in China (6). Since 2010, HFMD has consistently ranked as the most prevalent statutory infectious disease in China. From 2008 to 2017, the average annual reported incidence of HFMD nationwide was 134.59 per 100,000 (7), making China one of the countries with a high burden of HFMD. Zhejiang Province has one of the highest incidences of Hand, Foot, and Mouth Disease in China. Studies indicate that from 2017 to 2023, the cumulative reported incidence of HFMD in Zhejiang ranked among the top five in the country (8). Quzhou City, located in the western part of Zhejiang Province, is a high-incidence area for Hand, Foot, and Mouth Disease. From 2016 to 2017, the incidence rate in Quzhou exceeded the provincial average for two consecutive years (9). In recent years, many researchers have employed time series analysis to study the temporal variations of HFMD and predict future trends, utilizing models such as the SARIMA (Seasonal Autoregressive Integrated Moving Average) model (10). This model has a simple structure and requires only incidence data for predictions, making it highly feasible. It has been widely applied in the field of healthcare (11), for example, pertussis (12), tuberculosis (13) prediction. However, a smooth time series is required, and differential operations can lead to excessive data stabilization. The Prophet model is a time series forecasting tool based on an additive model that can simultaneously capture multiple seasonalities through generalized additive models. It’s fitting performance has been validated in time series predictions for infectious diseases such as Hand, Foot, and Mouth Disease (HFMD), AIDS (14, 15), syphilis (16) and flu-like case (17) prediction. The advantage is that it is suitable for time series with seasonal and trend changes. Strong robustness with missing and outliers. The disadvantage is that the processing of non-stationary data is simple and may not be sufficient to handle complex non-stationary features. Many previous studies were based on a single model or method of disease prediction analysis. Prophet models validate fitting in time series predictions for infectious diseases such as HFMD (14, 15). SARIMA (12) is also widely used in infectious disease prediction. Such as hand, foot and mouth disease (18, 19), tuberculosis (20), new coronary pneumonia (11) etc. However, at present, there are few comparisons between SARIMA and prophet models for fitting and predicting the incidence of hand, foot and mouth disease. The prediction of HFMD by the two models carried out in this study complements the existing research and reinforces the research foundation.
Despite increased efforts in recent years to control the HFMD outbreak, the disease has been transmitted strong sexuality and complex transmission pathways still contribute to family and society heavy medical burden. To better understand the epidemiological characteristics of Hand, Foot, and Mouth Disease in Quzhou City, a descriptive analysis of HFMD data from 2005 to 2023 will be conducted. Utilizing GIS technology, the spatial characteristics of HFMD in Quzhou City over the years will be analyzed. Furthermore, SARIMA and Prophet models will be employed to establish predictive models for the reported incidence of HFMD from 2005 to 2023, comparing the predictive performance of each model.
Quzhou is located in the western region of Zhejiang Province, China, situated upstream of the Qiantang River. It lies between longitudes 118°01′15″ and 119°20′20″ east and latitudes 28°15′26″ and 29°30′00″ north. The city’s topography is primarily mountainous and hilly, characterized by a subtropical monsoon climate, with an average annual temperature ranging from 16.3°C (in Kaihua) to 17.4°C (in the urban area). Quzhou experiences distinct seasons, ample sunshine, and abundant rainfall. The northern and southern mountainous areas receive more precipitation than the central plains, while the western region sees more rainfall than the eastern part. As of 2023, the city administers two districts, three counties, and one county-level city.
The HFMD case data for Quzhou City from January 2010 to December 2023 was obtained from the National Infectious Disease Reporting System (NIDRS) and the Chinese Center for Disease Control and Prevention (CDC). Cases were reported by various medical and health institutions at all levels in Quzhou and were reviewed by local disease prevention and control centers. All cases were confirmed according to the HFMD diagnostic criteria established by the Ministry of Health of the People’s Republic of China (WS588-2018).
Microsoft Excel (Microsoft Corporation, Redmond, W A, United States) was used to generate a database, organize and analyze the cases, and calculate the incidence rate. R software (version 4.2.1, R Foundation for Statistical Computing, Vienna, Austria) was used to analyze the prevalent characteristics of HFMD (time distribution, age distribution, sex distribution and occupation distribution) in Quzhou city from 2005 to 2023. Qualitative data were described as frequency and percent, and Chi-square test was used for inter-group comparison, with p < 0.05 indicating a statistically significant difference.
Get basic information on the incidence of hand, foot and mouth disease in Quzhou from NIDRS 2010–2023. After data cleansing, 66,601 case information was obtained. The incidence rate is defined as follows: Incidence = (number of new cases of a disease in a population in a given period/number of people exposed during the same period) × K, K = 100%, 1,000 ‰, 100 million or 100,000/100,000, etc. The number of onset per month according to the date of onset is included in the SARIMA and Prophet models analysis. Evaluate model fit performance with parameters such as MAE, MAPE and R2.The smaller the first two indicators the better, R2 the closer the better.
The Autoregressive Integrated Moving Average (ARIMA) model, proposed by George Box and Gwilym Jenkins, is a classic time series forecasting method that has been widely applied in the field of public health (21–23). The ARIMA (p, d, q) model is a hybrid model composed of the Autoregressive (AR) component and the Moving Average (MA) component, where p represents the order of the autoregression, d is the order of differencing, and q denotes the order of the moving average.
The Seasonal ARIMA (SARIMA) model builds upon the ARIMA framework by incorporating seasonal parameters. In the SARIMA model, P is the order of the seasonal autoregression, D is the order of seasonal differencing, Q is the order of the seasonal moving average, and S represents the length of the seasonal cycle (24). The general form of the model is (p,d,q) × (P,D,Q)s, which is applicable to both trend and seasonal variations (25). This structure allows for the modeling of time series data that exhibit long-term trends as well as seasonal patterns.
The model construction process consists of four steps: (1) stationarity testing: the application of the SARIMA model requires that the time series meets the stationarity condition. Initially, the stationarity of the data is assessed through the time series plot. An augmented Dickey-Fuller (ADF) test is then conducted. If the p-value (P) is greater than 0.05 and the series is non-stationary, it is necessary to stabilize the original series through differencing and/or seasonal differencing, as well as variable transformations. (2) Model order identification: based on the characteristics of the autocorrelation function (ACF) and the partial autocorrelation function (PACF) plots, a preliminary determination of the order is made, and multiple models are compared. The optimal model is chosen according to the Akaike Information Criterion (AIC). (3) Parameter estimation and diagnosis: parameters of the identified model are estimated, and hypothesis testing is performed. The residual series undergoes a white noise test (Ljung-Box test). If the p-value (P) is greater than 0.05, this indicates that the model is suitable for forecasting. (4) Model fitting and forecasting: the final step involves fitting the model and making predictions. The SARIMA model selects the optimal fit model according to the comparison of information criterion methods. Select SARIMA by comparing different models, selecting the AIC, AICc, BIC minimum, p < 0.05, residual test passed as the optimal model (2,1,1)(0,1,1) (12). Make predictions for the optimal model.
The Prophet model is a time series forecasting tool developed by Facebook in 2017, implemented in the C++ programming language. It is available for use in both Python and R (26). The model can flexibly handle missing data and account for the effects of holidays and special events within the time series. It fits quickly, making it suitable for forecasting time series with strong seasonal influences and multiple seasonal historical data (14). Prophet Model Step Description: (1) The environment prepares for the installation of Prophet and the R package on which it depends. (2) Data preparation, with the number of cases of HFMD in order of month. Convert the dataset to the format required by Prophet, with the ds and y columns representing the date and prediction values respectively, split the dataset into training sets and test sets, and we use the last 12 months of data as test sets. (3) Model training, training Prophet models, creating a Prophet model object, fitting models with training set data, predicting future data using models. The parameters are set as follows:growth:liner, changepoint.range:0.9, yearly.seasonality:TRUE, seasonality.mode: multiplicative, interval.wideh:0.95. (4) Evaluating optimization, prophet provides a number of methods to evaluate the fit of a model, such as visualizing prediction results, calculating prediction errors, and so on. The general form of the Prophet model is given by y(t) = g(t) + s(t) + h(t) + ε, where g(t)represents the trend function, s(t)is the seasonal function, (h(t))accounts for holiday effects, and ε denotes the error term, which employs an additive model for accumulation. The model construction process mainly consists of four steps: model building, model evaluation, problem presentation, and visual feedback (15).
This study employs the selected optimal model to fit the HFMD case data from 2005 to 2022 and then forecasts the data for 2023. The model’s fitting and predictive performance are evaluated using the Root Mean Square Error (RMSE) and the Mean Absolute Error (MAE); smaller values of these metrics indicate better model performance. The SARIMA model is constructed using the R software (version 4.2.1, R Foundation for Statistical Computing, Vienna, Austria) with the “tseries” and “forecast” packages, while the “prophet” package is used to build the Prophet model.
From 2010 to 2023, Quzhou City reported a total of 66,601 HFMD cases, with the annual number of reported cases fluctuating between 2,265 and 7,964. The average annual incidence rate was 216.88/100,000 population, with the lowest rate recorded in 2010 (97.08/100,000) and the highest in 2016 (373.37/100,000). During the study period initially declining and then rising, with a statistically significant difference observed (χ2 trend = 9234.9, p < 0.001) (Figure 1).
Among the HFMD cases, 38,650 were male (58.03%) and 27,951 were female (41.97%), resulting in a male-to-female ratio of 1.38:1. The incidence rate for males (246.71/100,000) was higher than that for females (185.81/100,000).
The age of onset ranged from 0 to 78 years, displaying a unimodal distribution, with the highest number of cases found in the 2-year age group, accounting for 32.66% (21,750 out of 66,601). The composition of cases varied across different age groups, with the highest incidence rate observed in the 1-year age group, followed by the 2-year age group, indicating a higher prevalence among younger children. The main occupations are Scattered Children 65.70% (43,760/66,601), Nursery Children 33.14% (22,069/66,601), School-age Children 1.11% (740/66,601) and Other 0.048% (32/66,601) (Table 1).
Using the HFMD incidence data from Quzhou City from 2010 to 2022, a time series was established on a monthly basis. The time series plot (Figure 2) and its decomposition (Figure 3) indicate that the HFMD incidence rate in Quzhou City is stabilizing. The decomposition of the time series shows seasonal fluctuations, necessitating seasonal differencing of the original series. The ADF test (ADF = −5.8065, p < 0.05) confirms that the series is stationary, indicating parameters (d = 1) and (D = 1). The Box-Pierce test yielded a statistic of 5.6405 with a p-value <0.05.A seasonal model with a period of 12 months was simulated: SARIMA ((p, 1, q)(P, 1, Q)12). Autocorrelation and partial autocorrelation analyses (Figure 4) determined (p = 2) and (q = 1), with (P) and (Q) generally ranging from 0 to 2. By comparing models based on the information criterion, the model with the smallest AIC was selected, resulting in the optimal model being SARIMA ((2, 1, 1)(0, 1, 1) 12). The results indicate that the model parameters are statistically significant (Table 2). Figure 5 shows that the ACF of the residuals lies within the upper and lower limits of two standard errors, and the p-values from the Ljung-Box test are significantly greater than the 0.05 significance level, suggesting that the residuals can be considered as a white noise series, indicating a good model fit. The SARIMA ((2, 1, 1)(0, 1, 1) 12) model was used to fit the HFMD incidence trend in Quzhou City from 2010 to 2022. The overall trend of the actual values aligns with the fitted values, although the peak values did not fit well. The predicted HFMD case numbers for January to December 2023 were then compared to the actual monthly case numbers (Figure 6; Table 3).
Figure 5. Residual diagnostics plots. Autocorrelation plot of the residual sequence; partial autocorrelation plot of the residual sequence; Ljung-Box test plot of the residual sequence.
Table 3. The monthly number of HFMD cases in the Quzhou City in 2023 predicted by the SARIMA, and Prophet models.
Using the Prophet package in R, a Prophet model was constructed based on the monthly HFMD incidence data from Quzhou City from 2010 to 2022. The interval width was set to 0.95, while all other parameters were kept at their default values. The model incorporates “trend” and “year” time series components (Figure 7). Figure 8 illustrates that the model effectively captures the trend in HFMD incidence and achieves good results in forecasting HFMD data. Table 3 presents the predicted values and 95% confidence intervals for both the SARIMA and Prophet models. The results indicate that the RMSE and MAE values for the Prophet model on the test set are higher than those for the SARIMA ((2,1,1)(0,1,1)12) model (Table 4). This suggests that the performance of the SARIMA ((2,1,1)(0,1,1) 12) model is superior to that of the Prophet model in terms of predictive accuracy.
The results of this study indicate that from 2010 to 2023, the incidence of HFMD in Quzhou City showed a rising and then falling on the upward trend with a significant seasonal pattern. The peak incidence periods are observed from April to July and from October to December. The disease predominantly affects males compared to females, with the age group most affected being children aged 0–5 years. The distribution of cases is primarily among children in informal settings and daycare centers. Both SARIMA and Prophet models were employed for fitting and forecasting, with the SARIMA model demonstrating superior performance.
According to the Infectious Disease Prevention and Control Law of the People’s Republic of China, Hand, Foot, and Mouth Disease (HFMD) is classified as a Category C infectious disease in the country. Over the past few decades, its incidence has consistently ranked first among legally reportable infectious diseases (7). Before 2016, Hand, Foot, and Mouth Disease (HFMD) in Quzhou City was in a naturally occurring epidemic state, with annual reported incidence rates significantly higher than the national average. The incidence exhibited a “linear” upward trend prior to 2014, followed by a fluctuating decline after 2015. The peak incidence occurred in 2014, showing a similar trend to that of Guangxi. The annual reported incidence rate in Quzhou was lower than that in Guangxi (27), higher than that in Gansu (28), and comparable to that in Fujian (29).
After 2016, the reported incidence rate of Hand, Foot, and Mouth Disease (HFMD) exhibited a downward trend; however, it remained at a relatively high level. This could be attributed to changes in the dominant strains of the HFMD virus (30), as well as the introduction and application of HFMD vaccines in Quzhou City in 2016. Additionally, factors such as the local climate, the degree of social development, and population mobility in Quzhou may also play a role in influencing the incidence rates (31, 32).During the peak season for HFMD, some regions may also experience a minor autumnal peak in cases (33, 34). The reported incidence rate of HFMD in Quzhou City exhibits a seasonal bimodal trend, with a primary peak occurring from April to June and a secondary peak from October to December, similar to the patterns observed in Shaanxi Province (32).
This indicates that spring and autumn are critical periods for the prevention and control of Hand, Foot, and Mouth Disease (HFMD) outbreaks in Quzhou City. The variations in reported incidence rates across different seasons may be related to climatic factors affecting enteroviruses. Research has shown that the EV71 virus survives longer under conditions of low temperature and high humidity (35). Quzhou, being in a subtropical monsoon climate, experiences lower temperatures and more rainfall in spring and autumn, creating favorable conditions for the survival of enteroviruses. Analysis of the reported cases of Hand, Foot, and Mouth Disease (HFMD) in Quzhou City from 2010 to 2023 reveals that the incidence rate among children aged 0–5 years is notably high, accounting for 93.99% of all reported cases. This finding is consistent with research conducted in Gansu (28) and Jinhua (36).
The highest incidence rate is observed in children aged 1 year, which may be attributed to factors such as the natural decline of maternal antibodies after the age of one and increased exposure opportunities (37). In terms of gender, the number of cases in males exceeds that in females. Possible reasons for this include: first, boys are naturally more active and engage in outdoor activities more frequently than girls, which increases their chances of virus exposure and subsequent infection. Second, children under the age of five have weaker immune systems and are more susceptible to viruses. Additionally, children who are not yet in school lack education on hygiene practices, and caregivers may also have limited knowledge about Hand, Foot, and Mouth Disease, resulting in a lack of awareness and preventive measures, thereby increasing the risk of infection (38). So we give the following clinical recommendations: Firstly, key areas and key population monitoring programmes are developed to ensure timely detection and management of outbreaks. Secondly, we need to optimize the allocation of medical resources, improve the level of medical institution construction, improve the diagnosis and treatment ability of hand, foot and mouth disease. Furthermore, the EV71 vaccine is effective in preventing hand, foot and mouth disease caused by EV71 infections. Therefore, we should further promote the vaccination of children of appropriate age EV71 and improve the immunity of the population. In addition, continuous improvement of environmental hygiene, with particular attention to hygiene management in child-intensive places such as schools and kindergartens, and regular preventive disinfection to reduce the chances of transmission of the virus. At the same time, to raise public health awareness through media publicity, health education activities and other ways to popularize the knowledge of hand, foot and mouth disease prevention and control, encourage parents to strengthen daily observation, once the discovery of suspected symptoms, immediately seek medical treatment.
This study is based on the HFMD incidence data from Quzhou City from 2010 to 2022, in which we constructed SARIMA and Prophet models to fit and predict the trends of HFMD incidence. We found that the optimal ARIMA model was more effective, with lower MAE, MAPE and higher R2 evaluation metrics compared to the Prophet model, indicating smaller prediction errors. Perhaps the model we studied was predictive of instability, and the reason for the analysis was probably the effect of: Firstly, meteorological factors. The ARIMA model only considers the effects of temporal factors on morbidity. Studies have shown that the incidence of HFMD is associated with average daily temperature, average daily humidity, and precipitation (39). Later studies may attempt to add meteorological factors to further correct the model. Secondly, The ARIMA model is a linear model in principle. But the incidence of infectious diseases is generally non-linear. This causes some limitations in model fitting. Resulting in errors, the study only divided the data into training and prediction samples. May cause overfitting or underfitting (40). Thirdly, Social factors. Infants and young children are susceptible to hand-foot-and-mouth disease. When the second-child policy is liberalized, the birth rate rises over a short period of time and decreases gradually (41). Our study also has limitations. First, the HFMD case data were collected through passive surveillance, which may introduce biases such as underreporting. Second, the model construction only considered the intrinsic characteristics of the time series and did not account for other exogenous variables, such as meteorological factors and economic variables. Thirdly, since the research data are specific to Quzhou City in China, our conclusions are only applicable to the population in that region, limiting the generalizability of the results. In the future, we need to further explore the interactions within the SARIMA model, considering various influencing factors to achieve more realistic predictions and using the ensemble technique combining multiple prediction models into a single ensemble model to improve the stability and accuracy of predictions. The reason behind the differences in model performance may be that we do not take into account the factors that affect the occurrence of HFMD, such as pathogens, hosts, natural environments, vaccines and socioeconomics. The disease prediction model of this study did not take into account the effect of the changes in the cases of new coronary infection against foot and mouth disease. It may not be possible to provide a basis for more comprehensive health policy formulation.
From 2010 to 2023, the overall incidence of Hand, Foot, and Mouth Disease (HFMD) in Quzhou City showed a declining trend; however, the epidemic remains severe in Kecheng District and Kaihua County. Infants and young children should be the primary focus of HFMD prevention and control efforts. In the future, it is important to continue enhancing the monitoring of HFMD dynamics, conduct health education initiatives before the high incidence season, and promote the vaccination of eligible children with the EV71 vaccine. The SARIMA model effectively fits and predicts the incidence trends of HFMD in Quzhou City, providing early warnings and forecasts for HFMD occurrences and offering reliable data for the prevention and control of HFMD in the region.
The overall incidence of HFMD in Quzhou City showed a trend of decline followed by an increase from 2010 to 2023, with a particularly severe situation in Kaihua County. Male children should be a key focus group for HFMD prevention and control. In the future, it is essential to continue strengthening the dynamic monitoring of HFMD outbreaks. Scientific prevention and control measures should be implemented before the peak period of HFMD, promoting vaccination and health education, especially for younger children. The SARIMA model has effectively fitted and predicted the occurrence trend of HFMD in Quzhou, providing forecasting for HFMD cases in the city.
The original contributions presented in the study are included in the article/supplementary material, further inquiries can be directed to the corresponding author.
Written informed consent was obtained from the individual(s), and minor(s)' legal guardian/next of kin, for the publication of any potentially identifiable images or data included in this article.
WX: Writing – original draft, Writing – review & editing. CZ: Formal analysis, Writing – review & editing. CF: Data curation, Writing – review & editing. XG: Data curation, Writing – review & editing. QF: Data curation, Writing – review & editing. ZY: Writing – review & editing.
The author(s) declare that no financial support was received for the research, authorship, and/or publication of this article.
The authors would like to sincerely thank Quzhou Center for Disease Control and Prevention for the support of this study, and most importantly, the participants of the study and the members of the survey team.
The authors declare that the research was conducted in the absence of any commercial or financial relationships that could be construed as a potential conflict of interest.
All claims expressed in this article are solely those of the authors and do not necessarily represent those of their affiliated organizations, or those of the publisher, the editors and the reviewers. Any product that may be evaluated in this article, or claim that may be made by its manufacturer, is not guaranteed or endorsed by the publisher.
1. Ryu, WS, Kang, B, Hong, J, Hwang, S, Kim, J, and Cheon, DS. Clinical and etiological characteristics of enterovirus 71-related diseases during a recent 2-year period in Korea. J Clin Microbiol. (2010) 48:2490–4. doi: 10.1128/JCM.02369-09
2. Solomon, T, Lewthwaite, P, Perera, D, Cardosa, MJ, McMinn, P, and Ooi, MH. Virology, epidemiology, pathogenesis, and control of enterovirus 71. Lancet Infect Dis. (2010) 10:778–90. doi: 10.1016/S1473-3099(10)70194-8
3. Wu, JT, Jit, M, Zheng, Y, Leung, K, Xing, W, Yang, J, et al. Routine pediatric enterovirus 71 vaccination in China: a cost-effectiveness analysis. PLoS Med. (2016) 13:e1001975. doi: 10.1371/journal.pmed.1001975
4. National Health Commission. Guidelines for the Diagnosis and Treatment of Hand, Foot and Mouth Disease. Chinese J Infect. Dis. (2018) 2018:347–52. doi: 10.3760cma.j.issn.1674-2397.2018.03.001
5. Qi, Z, and Junping, Z. Epidemiological status and pathogenic changes of hand, foot and mouth disease in China. J Virol. (2015) 31:554–9. doi: 10.13242/j.cnki.bingduxuebao.002776
6. Zhang, Y, Zhu, Z, Yang, W, Ren, J, Tan, X, Wang, Y, et al. An emerging recombinant human enterovirus 71 responsible for the 2008 outbreak of hand foot and mouth disease in Fuyang city of China. Virol J. (2010) 7:94. doi: 10.1186/1743-422X-7-94
7. Jing, Z. Analysis of trend and pathogen dynamics of hand, foot and mouth disease in China 2008-2017. Chinese J Epidemiol. (2019) 40:147. doi: 10.3760/cma.j.issn.0254-6450.2019.02.005
8. Raintun, Z, Yang, S, Fengfeng, L, et al. Analysis of epidemic characteristics and trend of Hfmd in China after infection with novel coronavirus type B tubes. Trop Dis Parasitol. (2023) 21:186–90.
9. Mengtao, P, Fudong, L, Junfen, L, and Xue, G. 2016-2019 epidemiological characteristics of hand, foot and mouth disease in Zhejiang Province. Prev Med. (2022) 34:307–10. doi: 10.19485/j.cnki.issn2096-5087.2022.03.019
10. Wang, T, Liu, J, Zhou, Y, Cui, F, Huang, Z, Wang, L, et al. Prevalence of hemorrhagic fever with renal syndrome in Yiyuan County, China, 2005-2014. BMC Infect Dis. (2016) 16:69. doi: 10.1186/s12879-016-1404-7
11. Ceylan, Z. Estimation of Covid-19 prevalence in Italy, Spain, and France. Sci Total Environ. (2020) 729:138817. doi: 10.1016/j.scitotenv.2020.138817
12. Yuqin, Z, Lixia, B, Min, G, Lei, Z, Hao, Z, Xiuhui, L, et al. Sarima model prediction of pertussis in Shanxi province. China Health Statis. (2024) 41:551–4. doi: 10.11783/j.issn.1002-3674.2024.04.015
13. Hao, G, in Jin, G, and Luojing, Z. Application of Sarima model and Bpnn model in prediction of tuberculosis in Jiangsu Province. Chin J Preven Med. (2024) 25:239–44. doi: 10.3784/jbjc.202201300027
14. Luo, Z, Jia, X, Bao, J, Song, Z, Zhu, H, Liu, M, et al. A combined model of Sarima and prophet models in forecasting Aids incidence in Henan Province, China. Int J Environ Res Public Health. (2022) 19:5910. doi: 10.3390/ijerph19105910
15. Xie, C, Wen, H, Yang, W, Cai, J, Zhang, P, Wu, R, et al. Trend analysis and forecast of daily reported incidence of hand, foot and mouth disease in Hubei, China by prophet model. Sci Rep. (2021) 11:1445. doi: 10.1038/s41598-021-81100-2
16. Quan, Z, Rookie, S, Wenwei, L, and Yongping, L. Analysis and trend prediction of syphilis prevalence in Aksu region 2008 - 2023. Modern Preven Med. (2024) 51:2862–8. doi: 10.20043/j.cnki.MPM.202404162
17. Yang, L, Haoyuan, Z, Jinqi, L, Yunpeng, Q, Yuanhang, W, Lei, J, et al. Prediction of influenza - like cases in Jiaxing, Zhejiang Province based on prophet model. Dis Sur. (2024) 39:629–33. doi: 10.3784/jbjc.202306300314
18. Zhicheng, D, Lin, X, Wangjian, Z, Dingmei, Z, Shicheng, Y, Yuantao, H, et al. Predicting the hand, foot, and mouth disease incidence using search engine query data and climate variables: an ecological study in Guangdong, China. BMJ Open. (2017) 7:e16263. doi: 10.1136/bmjopen-2017-016263
19. Liu, L, Luan, RS, Yin, F, Zhu, XP, and Lü, Q. Predicting the incidence of hand, foot and mouth disease in Sichuan province, China using the ARIMA model. Epidemiol Infect. (2016) 144:144–51. doi: 10.1017/S0950268815001144
20. Wang, KW, Deng, C, Li, JP, Zhang, YY, Li, XY, and Wu, MC. Hybrid methodology for tuberculosis incidence time-series forecasting based on ARIMA and a NAR neural network. Epidemiol Infect. (2017) 145:1118–29. doi: 10.1017/S0950268816003216
21. Lou, HR, Wang, X, Gao, Y, and Zeng, Q. Comparison of Arima model, Dnn model and Lstm model in predicting disease burden of occupational pneumoconiosis in Tianjin, China. BMC Public Health. (2022) 22:2167. doi: 10.1186/s12889-022-14642-3
22. Alzahrani, SI, Aljamaan, IA, and Al-Fakih, EA. Forecasting the spread of the Covid-19 pandemic in Saudi Arabia using Arima prediction model under current public health interventions. J Infect Public Health. (2020) 13:914–9. doi: 10.1016/j.jiph.2020.06.001
23. Ge, L, Zhao, Y, Zhou, K, Mu, X, Yu, H, Wang, Y, et al. Spatio-temporal pattern and influencing factors of hemorrhagic fever with renal syndrome (Hfrs) in Hubei Province (China) between 2005 and 2014. PLoS One. (2016) 11:e167836. doi: 10.1371/journal.pone.0167836
24. Hernandez-Matamoros, A, Fujita, H, Hayashi, T, and Perez-Meana, H. Forecasting of Covid19 per regions using Arima models and polynomial functions. Appl Soft Comput. (2020) 96:106610. doi: 10.1016/j.asoc.2020.106610
25. Azeez, A, Obaromi, D, Odeyemi, A, Ndege, J, and Muntabayi, R. Seasonality and trend forecasting of tuberculosis prevalence data in eastern cape, South Africa, using a hybrid model. Int J Environ Res Public Health. (2016) 13. doi: 10.3390/ijerph13080757
26. Taylor, SJ, and Letham, B. Forecasting at scale. Am Stat. (2018) 72:37–45. doi: 10.1080/00031305.2017.1380080
27. Hui, L, Lina, C, Weid, Z, et al. Trend analysis of joint point regression model of hand foot and mouth disease in Guangxi Zhuang autonomous region in 2008-2022. Dis Surveill. (2024) 39:229–34.
28. Yang, J, Yan, F, Liang, S, et al. 2010–2021 Gansu Province hand, foot and mouth disease monitoring analysis and model prediction. Dis Sur. (2024) 39:318–23.
29. Qi Xiaoyang, O, Jianming, O, Wu, C, Shaojian, C, Zhonghang, X, Shengeng, W, et al. Epidemiological characteristics and pathogenic analysis of hand, foot and mouth disease in Fujian Province 2016-2020. Chin J Preven Med. (2023) 24:960–3. doi: 10.16506/j.1009-6639.2023.09.014
30. Wanwen, M, Lei, G, Yongkang, X, Yongling, S, Yinlu, G, Sai, H, et al. Epidemiological characteristics and pathogenic changes of hand, foot and mouth disease in Anhui Province in 2015-2022. J Anhui Med Univ. (2023) 58:1763–8. doi: 10.19405/j.cnki.issn1000-1492.2023.10.026
31. Wu, H, Wang, J, Xu, X, and Hao, W. 2008 ~ 2012 epidemiological survey of hand, foot and mouth disease in Zhangjiakou area. China Med Herald. (2017) 14:59–62.
32. Yao, B, Kun, L, Xu, G, Kangjun, Z, Xiaojie, Y, and Zhongjun, S. Analysis of spatial and temporal epidemic characteristics of 2009 - 2013 hand, foot and mouth disease epidemic in Shaanxi Province. Chin J Epidemiol. (2018) 39:1152. doi: 10.3760/cma.j.issn.0254-6450.2018.09.003
33. Zhijie, An, Yan, L, Qiaohong, L, Yong, Z, Yan, L, Keli, L, et al. Technical guidelines for the use of inactivated enterovirus 71 vaccine. Vaccine Immunization China. (2016) 22:458–64. doi: 10.19914/j.cjvi.2016.04.020
34. Yuyang, X, Jing, R, and Guangcai, Z. Dynasty broad talent. Time series analysis and prediction model of monthly incidence of Hfmd in China 2008 - 2016. Chin J Dis Control. (2019) 23:1394–8. doi: 10.16462/j.cnki.zhjbkz.2019.11.019
35. Yun, C, Luofang, J, Yan, S, Yuxing, L, Qianli, W, Liwen, J, et al. Study on survival of enterovirus 71 on the surface of medium under different climatic conditions. Chin J Infect Dis. (2012) 30:398. doi: 10.3760/cma.j.issn.1000-6680.2012.07.005
36. Long, Z, Jialei, M, Wei, C, and Meili, L. Analysis of pathogenic distribution and epidemiological characteristics of hand foot and mouth disease in Jinhua City in 2019-2022. China J Health Inspec. (2023) 33:2219–21.
37. Li, L, Weijia, X, Qiaohong, L, and Hongjie, Y. Advances in serological epidemiology of enterovirus 71 and coxsackie virus a 16 infection in children (2015) 49:184. doi: 10.3760/cma.j.issn.0253-9624.2015.02.018
38. Chenchen, L, Yinyin, L, Wenna, Z, Bin, X, Tong, S, Qiuli, Y, et al. Analysis of epidemiological characteristics of Hfmd in Hebei Province in 2012 - 2019. Int J Virol. (2023) 30:46–50. doi: 10.3760/cma.j.issn.1673-4092.2023.01.010
39. Gao, Q, Liu, Z, Xiang, J, Tong, M, Zhang, Y, Wang, S, et al. Forecast and early warning of hand, foot, and mouth disease based on meteorological factors: evidence from a multicity study of 11 meteorological geographical divisions in mainland China. Environ Res. (2021) 192:110301. doi: 10.1016/j.envres.2020.110301
40. Rui, H. Establishment and evaluation of prediction model for the incidence of hand, foot and mouth disease in Liuzhou City Guangxi Medical University (2017).
Keywords: HFMD, epidemiological, SARIMA, prophet, predictive model
Citation: Xu W, Zheng C, Fu C, Gong X, Fang Q and Yin Z (2024) Epidemiological characteristics and prediction model construction of hand, foot and mouth disease in Quzhou City, China, 2005–2023. Front. Public Health. 12:1474855. doi: 10.3389/fpubh.2024.1474855
Received: 02 August 2024; Accepted: 29 November 2024;
Published: 18 December 2024.
Edited by:
Erin Kristin Zinkhan, The University of Utah, United StatesReviewed by:
Kabbilawsh P, NIT Calicut, IndiaCopyright © 2024 Xu, Zheng, Fu, Gong, Fang and Yin. This is an open-access article distributed under the terms of the Creative Commons Attribution License (CC BY). The use, distribution or reproduction in other forums is permitted, provided the original author(s) and the copyright owner(s) are credited and that the original publication in this journal is cited, in accordance with accepted academic practice. No use, distribution or reproduction is permitted which does not comply with these terms.
*Correspondence: Zhiying Yin, eXp5MTgxNUBzb2h1LmNvbQ==
†These authors have contributed equally to this work
Disclaimer: All claims expressed in this article are solely those of the authors and do not necessarily represent those of their affiliated organizations, or those of the publisher, the editors and the reviewers. Any product that may be evaluated in this article or claim that may be made by its manufacturer is not guaranteed or endorsed by the publisher.
Research integrity at Frontiers
Learn more about the work of our research integrity team to safeguard the quality of each article we publish.