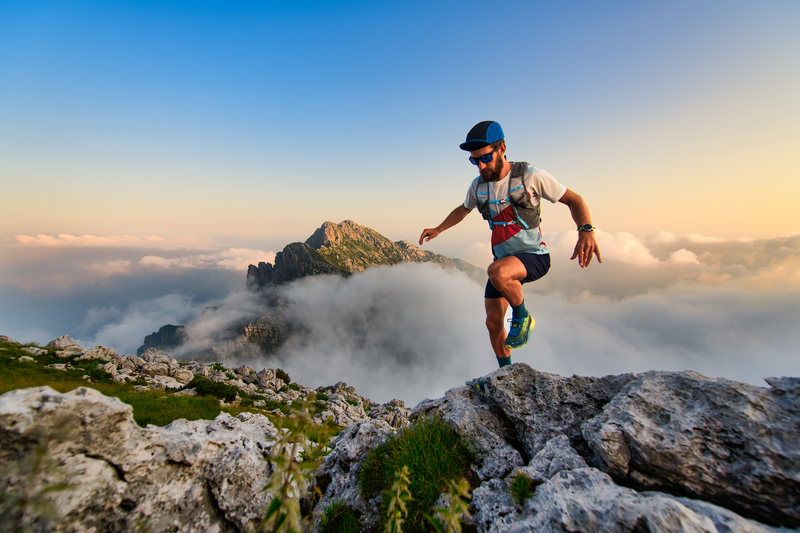
95% of researchers rate our articles as excellent or good
Learn more about the work of our research integrity team to safeguard the quality of each article we publish.
Find out more
ORIGINAL RESEARCH article
Front. Public Health , 19 November 2024
Sec. Environmental Health and Exposome
Volume 12 - 2024 | https://doi.org/10.3389/fpubh.2024.1463190
Introduction: Research increasingly links environmental exposure to toxic metals with health risks, yet the effect of combined metal exposure on Toxoplasma infection remains underexplored. This study investigates the relationship between concurrent heavy metal exposure and Toxoplasma infection in adults.
Methods: We analyzed data from 10,746 adults aged 20–80 from NHANES, with 1,869 positive for Toxoplasma gondii IgG. The study assessed associations between lead (Pb), cadmium (Cd), and mercury (Hg) with Toxoplasma infection risk using single-metal logistic regression, RCS analysis, WQS regression, and qgcomp models.
Results: Each metal showed an independent association with Toxoplasma infection risk. Pb had a non-linear association, while Hg had a linear one. Analysis of multiple metals indicated a positive correlation between heavy metal exposure and infection risk, particularly in younger and middle-aged adults, with Pb showing the strongest link.
Discussion: Our findings reveal a significant association between heavy metal exposure and Toxoplasma infection risk, especially in younger demographics, with lead being a key factor. This highlights the importance of understanding environmental metal exposure’s impact on public health and informs the development of prevention strategies.
Toxoplasma gondii, a single-celled eukaryotic protozoan parasite, is primarily transmitted through oral, bloodborne, and congenital routes of infection. It is estimated that approximately one-third of the global population may be infected with Toxoplasma gondii (1, 2). Although Toxoplasma gondii infection rates continue to decline in the United States, overall infection rates remain at approximately 7% (3). Individuals experiencing an acute Toxoplasma infection may exhibit symptoms akin to the flu. Fortunately, those with robust immune systems can typically eliminate the majority of the parasites during the acute phase. The parasites that survive tend to linger within the body, forming slow-growing cysts composed of bradyzoites, particularly in tissues that are less accessible to immune surveillance, such as the brain, eyes, heart, and skeletal muscles (4). For the majority of adults, the infection does not lead to serious illness. However, congenital infections in children can result in developmental issues such as mental retardation and blindness. Additionally, children with compromised immunity may experience more serious disorders (5).
With the development of industrialization, heavy metals are persistently threatening human health in a series of ways, including industrial products, food, soil, air, and drinking water (6). Environmental pollutants have many negative effects on human health through their diversification, accumulation, and ecological destruction (7). Heavy metals are regarded as a constant threat to humans since they cannot be eradicated (8). Studies have shown that combined exposure to multiple heavy metals can damage innate and adaptive immune responses, leading to enhanced susceptibility to infection and the occurrence of inflammatory and autoimmune disorders (9, 10). Prenatal exposure to heavy metals affects infectious disease-related genes within the glucocorticoid receptor signaling pathways (11). While the immunotoxic effects of heavy metals have been explored through animal studies, in vitro experiments, clinical assessments, and occupational exposure studies, there is a scarcity of large-scale human epidemiological research examining the detrimental immunological impacts of exposure to these metals. Furthermore, heavy metals are often found together in the environment, potentially leading to a range of interactions such as additive, antagonistic, synergistic, or other complex effects (12, 13). Hence, there is a need to explore the combined effect of heavy metals on common chronic infections.
Thus far, the relationship between heavy metal exposure and Toxoplasma infection has not been well-established. Previous studies on the origin of blood Toxoplasma and its interaction with heavy metals were not adequately reviewed. In this research, we leveraged data from the National Health and Nutrition Examination Survey (NHANES), which pertains to the general U.S. population, to conduct a comprehensive assessment of the association between three prevalent blood heavy metals and their combined effects on Toxoplasma infection. We used multivariable logistic regression, restricted cubic spline (RCS) analysis, logistic and weighted quantile sum (WQS) regression, and quantile-based g computation (qgcomp) models to elucidate these relationships. The findings of this study are expected to yield novel perspectives for the prevention and management of Toxoplasma infections.
The NHANES is a continual, national, multi-stage, and cross-sectional program conducted by the National Cancer Research Center for Health Statistics (NCHS) that represents the health and nutrition status of the non-institutional U.S. civilian population (14, 15). In the present study, data were collected from three NHANES cycles (2009–2010, 2011–2012, and 2013–2014). A total of 30,468 participants were involved in these study periods, with 19,344 completing the Toxoplasma gondii IgG analyses. We excluded individuals whose data on three blood heavy metals were missing (n = 2,901). Furthermore, we excluded individuals with missing information on covariates (n = 5,697). Finally, 10,746 participants were recruited, with 1,869 testing positive for Toxoplasma gondii infection. The specific inclusion and exclusion processes are shown in Figure 1.
The Toxoplasma gondii. IgG levels were measured using enzyme immunoassay. The experimental protocol has previously been described in detail (16). The IgG levels were measured in IU/ml and ranged from 0 to 308 IU/mL. The Toxoplasma gondii. IgG levels were divided into negative (−) /positive (+) variables (<33 IU/mL, ≥33 IU/mL) (NHANES Analysis Guidelines) (17). Since the skewed distribution of IgG levels cannot be normalized using exponents, logarithms, or contravariant transformations, we did not use IgG concentrations as a continuous variable (3).
From NHANES 2009–2014, the data of three common heavy metals [lead (Pb), cadmium (Cd), and mercury (Hg)] in blood were available. Inductively coupled plasma mass spectrometry (ICP-MS) (PerkinElmer SIMAA 6000) was applied. Detailed experimental protocols and approaches can be found on the NHANES Laboratory Protocol official website.1 According to the NHANES criterion, metal concentrations below the limit of detection (LOD) were replaced with the value of LOD divided by the square root of 2 (14).
The covariates corrected in the statistical model included the following variables: age (old people: ≥60 years old, young and middle-aged people: 20–59 years old), sex (male, female), ethnicity (Mexican American, non-Hispanic white, non-Hispanic black, other Hispanic, or other/multiracial), levels of education (<high school, ≥high school), marital status (married or living with partner, widowed or divorced or separated, and never married), body mass index (BMI) (<25, 25–29.90, ≥30 kg/m2), family poverty–income ratio (PIR) (≤1.30, 1.31–3.50, >3.50), serum cotinine concentration (>0.011, ≤0.011 ng/mL), and NHANES cycles (18). Serum cotinine concentration has been recognized as a reliable biomarker for objective assessment of smoking and secondhand smoke exposure and is a better measure than self-reported actual smoking dose (19).
Descriptive statistics of the participants’ characteristics and blood metal concentrations were given as mean ± standard deviation (SD), median and interquartile range (IQR), or frequencies (%). Baseline characteristics and blood metal concentrations between Toxoplasma gondii. IgG (+) and Toxoplasma gondii. IgG (−) participants were compared using a t-test, Mann–Whitney U-test, or χ2 test. The concentrations of blood metal were Ln-transformed to approximate normality and further divided into four quartiles (Q1, Q2, Q3, and Q4). We calculated Pearson’s correlation coefficients to pinpoint the correlations between the Ln-transformed blood metal concentrations. All data analyses and processing were carried out by R software (version 4.2.2), and the statistical significance was set as a double-tailed p-value of <0.05. Stratified analyses were further conducted based on age (20 ≤ age ≤ 59, age ≥ 60).
First, the weighted multivariate logistic regression was carried out to estimate the association between each heavy metal in blood and Toxoplasma infection. The first quartile (Q1) served as the reference, and the results were expressed as odds ratios (ORs) and corresponding 95% confidence intervals (CIs). Age, sex, ethnicity, PIR, marital status, levels of education, BMI, serum cotinine, and NHANES cycles were adjusted in the models to avoid underlying confounders. The dose–reaction relationship between exposure to blood heavy metals and Toxoplasma infection was identified using RCS regression performed by the R-packet “rms.” It can not only reflect the linear relationship between heavy metals and Toxoplasma infection risk but also uncover the non-linear relationship between the two.
Next, we used two novel and complementary approaches, WQS regression and qgcomp models, to comprehensively investigate the overall effects of three heavy metals in blood on the risk of Toxoplasma infection. The WQS model has been described in detail in the past (20). Data were separated into two datasets at random (40% for the training subset and 60% for the validation subset). After bootstrapping 500 times, the R package (“gWQS”) calculated a weighted linear index (ranging from 0 to 1) that represented the total human load of all three metals. The corresponding weight of each metal was equal to its contribution to the WQS index. Considering the uncertainty of the direction of each component in the mixture, we further applied the qgcomp method (21). The model assigned positive and negative weights to each component in the mixture, and the sum of weights in different directions was 1.0.
A total of 10,746 participants aged 20–80 were incorporated in the analyses, of which 1,869 were Toxoplasma gondii IgG (+). The demographic features of all participants are shown in Table 1. It can be found that there were statistical significances in age, sex, race, levels of education, marital status, BMI, and family PIR between Toxoplasma gondii IgG (−) and Toxoplasma gondii IgG (+) participants (all p-values <0.05). People with Toxoplasma gondii IgG (+) were more likely to be younger, male, non-Hispanic black, and overweight, with lower education levels and family PIR. Moreover, there was no significant difference between Toxoplasma gondii IgG (−) and Toxoplasma gondii IgG (+) participants regarding serum cotinine level.
Supplementary Table S1 presents the distributions and the detection rates of three blood heavy metals. The detection rates of three heavy metals in blood were all more than 85%. The median and IQR of blood Pb, Cd, and Hg were 1.16 (1.08), 0.33 (0.39), and 0.85 (1.30), respectively. Subgroup analyses based on age showed that blood Pb and Hg were significantly higher in older adults than in young and middle-aged populations (all p-values <0.001). Figure 2 shows Pearson’s correlation coefficients between these metals after being Ln-transformed. There were relatively weak correlations between blood Pb and Cd (r = 0.15), Pb and Hg (r = 0.05), and Cd and Hg (r = 0.00).
To evaluate the effect of single blood heavy metals on the risk of Toxoplasma gondii infection, multivariate logistic regression adjusted for several covariates was conducted, and the results can be found in Table 2. The highest quantile (Q4) of Pb (OR: 2.06, 95%CI: (1.74, 2.45), p < 0.001) and Hg (OR: 1.37, 95%CI: (1.18, 1.60), p < 0.001) increased the risk of Toxoplasma gondii infection compared to the first quantile (Q1). Such significant associations were kept when setting Ln-transformed blood metal concentration as an independent variable [Pb: OR: 1.50, 95%CI: (1.37, 1.63), p < 0.001; Hg: OR: 1.13, 95%CI: (1.07, 1.19), p < 0.001]. Moreover, the Ln-transformed blood Cd level was also positively correlated with the risk of Toxoplasma gondii infection (Cd: OR: 1.08, 95%CI: (1.00, 1.16), p = 0.036). Furthermore, subgroup analyses were performed according to age. The positive correlation between blood Pb concentration and Toxoplasma gondii infection was statistically significant in both the young and middle-aged group and the older adult group, but more significant in the young and middle-aged group. Moreover, the positive association between blood Hg concentration and Toxoplasma gondii infection was significant in the young and middle-aged group but not in the older adult group.
Table 2. Associations of single blood metals with Toxoplasma infection risk in the NHANES 2009–2014 cycles.
The dose–reaction relationship between three blood heavy metals and the risk of Toxoplasma gondii infection estimated using RCS regression is shown in Figure 3. In general, the non-linear and positive association between blood Pb level and Toxoplasma gondii infection was observed (Pnonlinear < 0.001, Poverall < 0.001). The risk of Toxoplasma gondii infection increased with elevated blood Pb level. The subgroup analyses also indicated a positive association between blood Pb level and Toxoplasma gondii infection risk in both the young and middle-aged group and the older adult group, but it was non-linear in the young and middle-aged group (Pnonlinear = 0.003, Poverall < 0.001) and linear in the older adult group (Pnonlinear = 0.223, Poverall = 0.018). The non-linear association between blood Cd level and Toxoplasma gondii infection risk in the older adult group (Pnonlinear = 0.013, Poverall = 0.045), but not in the total group and the young and middle-aged group. Moreover, there was a linear and positive association between blood Hg level and Toxoplasma gondii infection (Pnonlinear = 0.085, Poverall = 0.001). A similar trend was also identified in the young and middle-aged group (Pnonlinear = 0.095, Poverall < 0.001), but not in the older adult group.
Figure 3. Dose-response relationship between urinary blood metals and Toxoplasma gondii infection were estimated by RCS models in total population and subgroups.
Next, we further explored the effect of combined exposure to multiple blood heavy metals on the risk of toxoplasma infection. The WQS regression correcting for all covariates was conducted. It can be found that the combined exposure to blood heavy metals was significantly associated with an increased risk of Toxoplasma gondii infection (OR: 1.36, 95%CI: (1.25, 1.47), p < 0.001) (Figure 4). Among the mixed blood heavy metals, Pb accounted for the highest weight with a value of 0.830, followed by Hg (0.143) and Cd (0.027) (Supplementary Figure S1). Furthermore, subgroup analyses were carried out by age. The results showed that the significant association between co-exposure to blood heavy metals and Toxoplasma gondii infection remained in the young and middle-aged group (OR: 1.55, 95%CI: (1.39, 1.73), p < 0.001), but not in the older adult group. In the young and middle-aged group, Pb accounted for the highest weight with a value of 0.744, followed by Hg (0.251) and Cd (0.005).
Figure 4. Odds ratios (95% CI) of Toxoplasma gondii infection associated with co-exposure to blood metal mixtures by WQS (A) and qgcomp (B) analyses.
In the qgcomp regression model, after correcting for all confounding factors, we also observed the significant and positive association between co-exposure to blood heavy metals and the risk of Toxoplasma gondii infection (OR: 1.04, 95%CI: (1.03, 1.05), p < 0.001). The estimated weights of the positive and negative factors are shown in Supplementary Figure S2, where Pb (weighted 0.756) was still the strongest positive driver, followed by Hg (weighted 0.244). The results of subgroup analyses revealed that the significant association between co-exposure to blood heavy metals and Toxoplasma gondii infection remained in both the young and middle-aged group (OR: 1.04, 95%CI: (1.03, 1.05), p < 0.001) and the older adult group (OR: 1.03, 95%CI: (1.01, 1.05), p = 0.012). In the young and middle-aged group, Pb accounted for the highest weight with a value of 0.788, followed by Hg (0.212). In the older adult group, Pb accounted for the highest weight with a value of 0.577, followed by Hg (0.305) and Cd (0.118).
In our cross-sectional analysis of U.S. adults, we applied four diverse statistical techniques, accounting for multiple confounding variables, to thoroughly evaluate the distinct and cumulative influences of three prevalent heavy metals—lead (Pb), cadmium (Cd), and mercury (Hg)—on the risk of Toxoplasma gondii infection. The single-metal logistic regression models demonstrated independent correlations between each metal and the risk of infection. Furthermore, restricted cubic spline (RCS) analysis exposed non-linear associations for blood Pb and linear associations for blood Hg with Toxoplasma gondii infection. When examining multiple-metal models, both the weighted quantile sum (WQS) and quantile-based g computation (qgcomp) models consistently revealed a positive link between co-exposure to these heavy metals and the risk of Toxoplasma gondii infection. Subgroup analyses indicated that this link was particularly pronounced among younger and middle-aged individuals. Notably, the most robust association was observed between lead (Pb) and Toxoplasma gondii infection across all statistical methodologies.
Pb is one of the most toxic metals with no biological function (22). Pb is ubiquitous in the environment and may account for 10% of total heavy metal pollution (23). Activities that constitute major sources of lead exposure include industrial activities, mining, lead-glazed ceramic manufacturing, battery recycling, and improper waste disposal (24, 25). There is growing evidence that Pb has multiple adverse health effects, such as cardiovascular disorders, kidney disease, and neurological disorders (26–29). Pb can also enhance the rate of chronic infection by affecting the immune responses. Park et al. found that the blood Pb level was positively correlated with Helicobacter pylori infection (30). In a study on the neuroethology of children, Martinez et al. revealed the underlying interaction between Toxoplasmosis prevalence and Pb exposure, which supported the findings of our study (31). Moreover, the non-linear association of blood Pb with Toxoplasma gondii infection was observed, which may be related to the relatively long half-life of lead (32). In addition to Pb, the independent association between Cd and Hg with Toxoplasma gondii infection was also identified in our study. Cd is a toxic heavy metal that poses a serious threat to human health (33). The rapid development of industry has caused serious Cd pollution (34). Cd can enter the body via a variety of ways, such as the atmosphere, food, water, and soil, and has a half-life of 10–30 years (35). A cross-sectional research using the NHANES data reported that the immune effect of Cd toxicity may be correlated with increased susceptibility to chronic infections (36). Hg is a toxic heavy metal that occurs naturally in the environment in various forms (37). Human activities can release Hg into the air, water, and soil (38). Hg is also released into the environment after it is converted to methylmercury by bacteria. Hg has long been considered a neurotoxicant; nevertheless, recent studies in animals have shown that Hg is also an immunotoxic agent (39). Toxoplasmosis can cause a lifelong infection that can reactivate and arouse severe illness or even death in immunocompromised individuals (40). In short, the influence of these blood heavy metals on toxoplasma infection may be related to their immunotoxicity.
Though the link between exposure to specific metals and Toxoplasma gondii infection is established, data on the synergistic effects of metal mixtures remain scarce. In this study, we used weighted quantile sum (WQS) and quantile-based g computation (qgcomp) regression models to assess the collective impact of three prevalent blood heavy metals on the risk of Toxoplasma gondii infection. Our findings consistently indicated a positive correlation between exposure to these metal mixtures and the risk of infection. Notably, lead (Pb) was identified as the metal with the highest weighted compound risk by both models. Subsequently, we conducted stratified analyses based on age groups to further explore this association. It can be found that the positive association between co-exposure to blood heavy metal mixtures and Toxoplasma gondii infection was stronger in the young and middle-aged group, which may be due to more occupational exposure opportunities to heavy metals in the young and middle-aged population (18).
While the current study utilized a suite of complementary statistical models to explore both the individual and combined influences of blood heavy metals on Toxoplasma gondii infection, yielding relatively robust findings, certain inherent limitations should be acknowledged. First, the cross-sectional nature of the survey design precludes any definitive conclusions about causality between heavy metal exposure and Toxoplasma infection. Second, the varying half-lives of different metals within the human body complicate the precise measurement of their concentrations. Finally, despite accounting for a range of covariates, not all potential confounding factors could be fully addressed due to data limitations. In conclusion, the relationship between metal mixtures and the risk of Toxoplasma gondii infection merits further investigation and validation through prospective, large-scale cohort studies.
The original contributions presented in the study are included in the article/Supplementary material, further inquiries can be directed to the corresponding author.
JZ: Writing – original draft. CX: Investigation, Writing – original draft. YC: Formal analysis, Writing – original draft. JS: Writing – review & editing.
The author(s) declare that no financial support was received for the research, authorship, and/or publication of this article.
Thanks to all authors for their joint efforts.
The authors declare that the research was conducted in the absence of any commercial or financial relationships that could be construed as a potential conflict of interest.
All claims expressed in this article are solely those of the authors and do not necessarily represent those of their affiliated organizations, or those of the publisher, the editors and the reviewers. Any product that may be evaluated in this article, or claim that may be made by its manufacturer, is not guaranteed or endorsed by the publisher.
The Supplementary material for this article can be found online at: https://www.frontiersin.org/articles/10.3389/fpubh.2024.1463190/full#supplementary-material
1. Tenter, AM, Heckeroth, AR, and Weiss, LM. Toxoplasma gondii: from animals to humans. Int J Parasitol. (2000) 30:1217–58. doi: 10.1016/S0020-7519(00)00124-7
2. Dubey, JP, and Jones, JL. Toxoplasma gondii infection in humans and animals in the United States. Int J Parasitol. (2008) 38:1257–78. doi: 10.1016/j.ijpara.2008.03.007
3. Krueger, WS, Hilborn, ED, Converse, RR, and Wade, TJ. Drinking water source and human toxoplasma gondii infection in the United States: a cross-sectional analysis of NHANES data. BMC Public Health. (2014) 14:711. doi: 10.1186/1471-2458-14-711
4. Zhao, XY, and Ewald, SE. The molecular biology and immune control of chronic toxoplasma gondii infection. J Clin Invest. (2020) 130:3370–80. doi: 10.1172/JCI136226
5. Zhou, P, Chen, Z, Li, HL, Zheng, H, He, S, Lin, RQ, et al. Toxoplasma gondii infection in humans in China. Parasit Vectors. (2011) 4:165. doi: 10.1186/1756-3305-4-165
6. Renu, K, Chakraborty, R, Myakala, H, Koti, R, Famurewa, AC, Madhyastha, H, et al. Molecular mechanism of heavy metals (lead, chromium, arsenic, mercury, nickel and cadmium) - induced hepatotoxicity - a review. Chemosphere. (2021) 271:129735. doi: 10.1016/j.chemosphere.2021.129735
7. Chormare, R, and Kumar, MA. Environmental health and risk assessment metrics with special mention to biotransfer, bioaccumulation and biomagnification of environmental pollutants. Chemosphere. (2022) 302:134836. doi: 10.1016/j.chemosphere.2022.134836
8. Patwa, J, and Flora, SJS. Heavy metal-induced cerebral small vessel disease: insights into molecular mechanisms and possible reversal strategies. Int J Mol Sci. (2020) 21:3862. doi: 10.3390/ijms21113862
9. Popov Aleksandrov, A, Mirkov, I, Tucovic, D, Kulas, J, Zeljkovic, M, Popovic, D, et al. Immunomodulation by heavy metals as a contributing factor to inflammatory diseases and autoimmune reactions: cadmium as an example. Immunol Lett. (2021) 240:106–22. doi: 10.1016/j.imlet.2021.10.003
10. Pollard, KM, Cauvi, DM, Toomey, CB, Hultman, P, and Kono, DH. Mercury-induced inflammation and autoimmunity. Biochim Biophys Acta Gen Subj. (2019) 1863:129299. doi: 10.1016/j.bbagen.2019.02.001
11. Rager, JE, Yosim, A, and Fry, RC. Prenatal exposure to arsenic and cadmium impacts infectious disease-related genes within the glucocorticoid receptor signal transduction pathway. Int J Mol Sci. (2014) 15:22374–91. doi: 10.3390/ijms151222374
12. Chen, L, Sun, Q, Peng, S, Tan, T, Mei, G, Chen, H, et al. Associations of blood and urinary heavy metals with rheumatoid arthritis risk among adults in NHANES, 1999-2018. Chemosphere. (2022) 289:133147. doi: 10.1016/j.chemosphere.2021.133147
13. Li, W, Li, X, Su, J, Chen, H, Zhao, P, Qian, H, et al. Associations of blood metals with liver function: analysis of NHANES from 2011 to 2018. Chemosphere. (2023) 317:137854. doi: 10.1016/j.chemosphere.2023.137854
14. Chen, Y, Shen, J, Wu, Y, Fang, L, Xu, S, Peng, Y, et al. Associations between urinary phthalate metabolite concentrations and rheumatoid arthritis in the U.S. adult population. Chemosphere. (2023) 337:139382. doi: 10.1016/j.chemosphere.2023.139382
15. R Cardoso, B, Machado, P, and Steele, EM. Association between ultra-processed food consumption and cognitive performance in US older adults: a cross-sectional analysis of the NHANES 2011-2014. Eur J Nutr. (2022) 61:3975–85. doi: 10.1007/s00394-022-02911-1
16. Babekir, A, Mostafa, S, and Obeng-Gyasi, E. The Association of Toxoplasma gondii IgG antibody and chronic kidney disease biomarkers. Microorganisms. (2022) 10:115. doi: 10.3390/microorganisms10010115
17. Wiener, RC, Waters, C, and Bhandari, R. The association of toxoplasma gondii IgG and cognitive function scores: NHANES 2013-2014. Parasitol Int. (2020) 78:102123. doi: 10.1016/j.parint.2020.102123
18. Chen, Y, Pan, Z, Shen, J, Wu, Y, Fang, L, Xu, S, et al. Associations of exposure to blood and urinary heavy metal mixtures with psoriasis risk among U.S. adults: a cross-sectional study. Sci Total Environ. (2023) 887:164133. doi: 10.1016/j.scitotenv.2023.164133
19. Xu, C, Liang, J, Xu, S, Liu, Q, Xu, J, and Gu, A. Increased serum levels of aldehydes are associated with cardiovascular disease and cardiovascular risk factors in adults. J Hazard Mater. (2020) 400:123134. doi: 10.1016/j.jhazmat.2020.123134
20. Yu, L, Liu, W, Wang, X, Ye, Z, Tan, Q, Qiu, W, et al. A review of practical statistical methods used in epidemiological studies to estimate the health effects of multi-pollutant mixture. Environ Pollut. (2022) 306:119356. doi: 10.1016/j.envpol.2022.119356
21. Keil, AP, Buckley, JP, O'Brien, KM, Ferguson, KK, Zhao, S, and White, AJ. A quantile-based g-computation approach to addressing the effects of exposure mixtures. Environ Health Perspect. (2020) 128:47004. doi: 10.1289/EHP5838
22. Zeng, X, Huo, X, Xu, X, Liu, D, and Wu, W. E-waste lead exposure and children's health in China. Sci Total Environ. (2020) 734:139286. doi: 10.1016/j.scitotenv.2020.139286
23. Levin, R, Zilli Vieira, CL, Rosenbaum, MH, Bischoff, K, Mordarski, DC, and Brown, MJ. The urban lead (Pb) burden in humans, animals and the natural environment. Environ Res. (2021) 193:110377. doi: 10.1016/j.envres.2020.110377
24. Hernández-Pellón, A, Nischkauer, W, Limbeck, A, and Fernández-Olmo, I. Metal(loid) bioaccessibility and inhalation risk assessment: a comparison between an urban and an industrial area. Environ Res. (2018) 165:140–9. doi: 10.1016/j.envres.2018.04.014
25. Baloch, S, Kazi, TG, Baig, JA, Afridi, HI, and Arain, MB. Occupational exposure of lead and cadmium on adolescent and adult workers of battery recycling and welding workshops: adverse impact on health. Sci Total Environ. (2020) 720:137549. doi: 10.1016/j.scitotenv.2020.137549
26. Brown, L, Lynch, M, Belova, A, Klein, R, and Chiger, A. Developing a health impact model for adult lead exposure and cardiovascular disease mortality. Environ Health Perspect. (2020) 128:97005. doi: 10.1289/EHP6552
27. Harari, F, Sallsten, G, Christensson, A, Petkovic, M, Hedblad, B, Forsgard, N, et al. Blood lead levels and decreased kidney function in a population-based cohort. Am J Kidney Dis. (2018) 72:381–9. doi: 10.1053/j.ajkd.2018.02.358
28. Gui, C, Shan, X, Liu, C, He, L, Zhao, H, and Luo, B. Disease burden of chronic kidney disease attributable to lead exposure: a global analysis of 30 years since 1990. Chemosphere. (2023) 341:140029. doi: 10.1016/j.chemosphere.2023.140029
29. Bihaqi, SW. Early life exposure to lead (Pb) and changes in DNA methylation: relevance to Alzheimer’s disease. Rev Environ Health. (2019) 34:187–95. doi: 10.1515/reveh-2018-0076
30. Park, WJ, Kim, SH, Kang, W, Ahn, JS, Cho, S, Lim, DY, et al. Blood lead level and Helicobacter pylori infection in a healthy population: a cross-sectional study. Arch Environ Occup Health. (2020) 75:333–8. doi: 10.1080/19338244.2019.1654969
31. Martinez, VO, Lima, FWM, Rocha, RBA, Bah, HAF, Carvalho, CF, and Menezes-Filho, JA. Interaction of toxoplasma gondii infection and elevated blood lead levels on children’s neurobehavior. Neurotoxicology. (2020) 78:177–85. doi: 10.1016/j.neuro.2020.03.010
32. Aliomrani, M, Sahraian, MA, Shirkhanloo, H, Sharifzadeh, M, Khoshayand, MR, and Ghahremani, MH. Blood concentrations of cadmium and Lead in multiple sclerosis patients from Iran. Iran J Pharm Res. (2016) 15:825–33.
33. Satarug, S, Garrett, SH, Sens, MA, and Sens, DA. Cadmium, environmental exposure, and health outcomes. Environ Health Perspect. (2010) 118:182–90. doi: 10.1289/ehp.0901234
34. Franzaring, J, Fangmeier, A, Schlosser, S, and Hahn, V. Cadmium concentrations in German soybeans are elevated in conurbations and in regions dominated by mining and the metal industry. J Sci Food Agric. (2019) 99:3711–5. doi: 10.1002/jsfa.9548
35. Wang, M, Chen, Z, Song, W, Hong, D, Huang, L, and Li, Y. A review on cadmium exposure in the population and intervention strategies against cadmium toxicity. Bull Environ Contam Toxicol. (2021) 106:65–74. doi: 10.1007/s00128-020-03088-1
36. Krueger, WS, and Wade, TJ. Elevated blood lead and cadmium levels associated with chronic infections among non-smokers in a cross-sectional analysis of NHANES data. Environ Health. (2016) 15:16. doi: 10.1186/s12940-016-0113-4
37. Yang, L, Zhang, Y, Wang, F, Luo, Z, Guo, S, and Strähle, U. Toxicity of mercury: molecular evidence. Chemosphere. (2020) 245:125586. doi: 10.1016/j.chemosphere.2019.125586
38. Bhan, A, and Sarkar, NN. Mercury in the environment: effect on health and reproduction. Rev Environ Health. (2005) 20:39–56. doi: 10.1515/REVEH.2005.20.1.39
39. Gardner, RM, Nyland, JF, and Silbergeld, EK. Differential immunotoxic effects of inorganic and organic mercury species in vitro. Toxicol Lett. (2010) 198:182–90. doi: 10.1016/j.toxlet.2010.06.015
Keywords: Toxoplasma infection, heavy metal, mixtures, NHANES, independent and comprehensive associations
Citation: Zhou J, Xing C, Chen Y and Shen J (2024) Associations of exposure to blood heavy metal mixtures with Toxoplasma infection among U.S. adults: a cross-sectional study. Front. Public Health. 12:1463190. doi: 10.3389/fpubh.2024.1463190
Received: 11 July 2024; Accepted: 04 November 2024;
Published: 19 November 2024.
Edited by:
Mohiuddin Md. Taimur Khan, Washington State University Tri-Cities, United StatesReviewed by:
Dieyi Chen, The Pennsylvania State University, United StatesCopyright © 2024 Zhou, Xing, Chen and Shen. This is an open-access article distributed under the terms of the Creative Commons Attribution License (CC BY). The use, distribution or reproduction in other forums is permitted, provided the original author(s) and the copyright owner(s) are credited and that the original publication in this journal is cited, in accordance with accepted academic practice. No use, distribution or reproduction is permitted which does not comply with these terms.
*Correspondence: Jilu Shen, c2hlbmppbHVAYWhtdS5lZHUuY24=
Disclaimer: All claims expressed in this article are solely those of the authors and do not necessarily represent those of their affiliated organizations, or those of the publisher, the editors and the reviewers. Any product that may be evaluated in this article or claim that may be made by its manufacturer is not guaranteed or endorsed by the publisher.
Research integrity at Frontiers
Learn more about the work of our research integrity team to safeguard the quality of each article we publish.