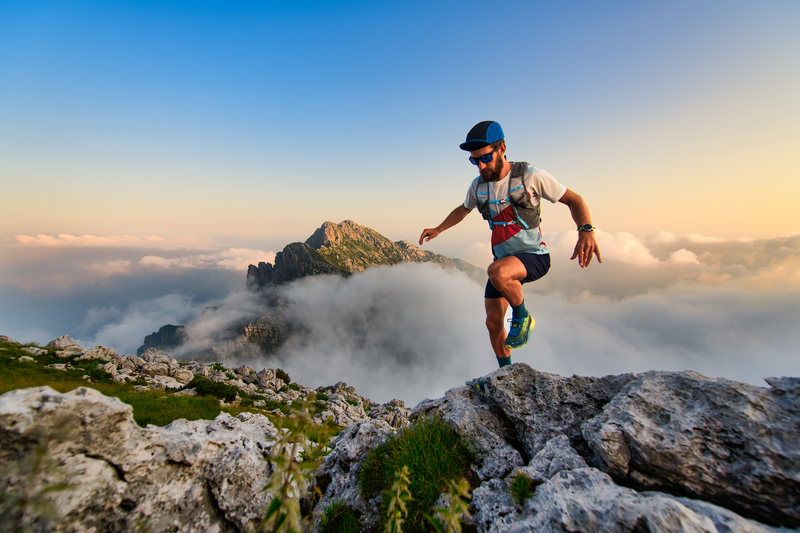
95% of researchers rate our articles as excellent or good
Learn more about the work of our research integrity team to safeguard the quality of each article we publish.
Find out more
ORIGINAL RESEARCH article
Front. Public Health , 21 August 2024
Sec. Environmental Health and Exposome
Volume 12 - 2024 | https://doi.org/10.3389/fpubh.2024.1462548
This article is part of the Research Topic Impact of Environmental Factors on the Health of Children and Older Adults View all 9 articles
Background: Previous studies indicated that exposure to ambient fine particulate matter (PM2.5) could increase the risk of metabolic syndrome (MetS). However, the specific impact of PM2.5 chemical components remains uncertain.
Methods: A national cross-sectional study of 12,846 Chinese middle-aged and older adults was conducted. Satellite-based spatiotemporal models were employed to determine the 3-year average PM2.5 components exposure, including sulfates (SO42−), nitrates (NO3−), ammonia (NH4+), black carbon (BC), and organic matter (OM). Generalized linear models were used to investigate the associations of PM2.5 components with MetS and the components of MetS, and restricted cubic splines curves were used to establish the exposure-response relationships between PM2.5 components with MetS, as well as the components of MetS.
Results: MetS risk increased by 35.1, 33.5, 33.6, 31.2, 32.4, and 31.4% for every inter-quartile range rise in PM2.5, SO42−, NO3−, NH4+, OM and BC, respectively. For MetS components, PM2.5 chemical components were associated with evaluated risks of central obesity, high blood pressure (high-BP), high fasting glucose (high-FBG), and low high-density lipoprotein cholesterol (low-HDL).
Conclusion: This study indicated that exposure to PM2.5 components is related to increased risk of MetS and its components, including central obesity, high-BP, high-FBG, and low-HDL. Moreover, we found that the adverse effect of PM2.5 chemical components on MetS was more sensitive to people who were single, divorced, or widowed than married people.
Metabolic syndrome (MetS) is characterized by a group of metabolic-related disorders, which encompass central obesity, elevated blood pressure (high-BP), increased fasting glucose levels (high-FBG), elevated triglyceride levels (high-TG), and reduced high-density lipoprotein levels (low-HDL) (1, 2). Recent studies have shown that about 20 to 30% of adults worldwide have MetS and the number of MetS patients has continued to rise (3, 4). Compared with non-MetS individuals, the prevalence of cardiovascular diseases (5, 6), respiratory diseases (7), diabetes (8, 9), and cancers are significantly elevated among MetS patients (10, 11). Genetic factors, unhealthy lifestyles, and inadequate physical activity have been reported as potential risk factors for MetS (12, 13). However, these characteristics may not fully explain the high MetS prevalence. The negative effects of a hazardous environment, especially air pollution, cannot be ignored (9).
Accumulating epidemiological studies indicated that exposure to air pollution was linked to an increased risk of MetS (4, 5, 8, 10, 12). Among these studies, the impact of fine particulate matter (PM2.5) has garnered significant attention from epidemiologists. However, consistent conclusions have not been reached (9). For instance, a cross-sectional study revealed that PM2.5 exposure was linked to an increased risk of MetS (12). However, a recent meta-analysis reported no statistically significant relationship of PM2.5 with the risk of MetS (9). In addition to inconsistent results, the existing studies focused solely on examining the relationship between PM2.5 mass concentration and MetS risk, without assessing PM2.5 chemical components, such as sulfates (SO42−), nitrates (NO3−), ammonia (NH4+), organic matter (OM) and black carbon (BC). Only one cross-sectional study has investigated the impact of PM2.5 chemical components on MetS risk (14). This study indicated that exposure to SO42− was linked to a higher prevalence of MetS, but no significant relationship was discovered between NO3−, NH4+, and OM (14). However, only a small number of participants (n = 2045) were included, and the study was conducted in the Beijing, Tianjin, and Hebei regions. Therefore, further research in larger geographical areas and with more participants to identify the key PM2.5 components that cause MetS.
In addition to the limited studies of PM2.5 components and MetS, it is important to investigate the connections between various MetS components and PM2.5 components to clarify the adverse impacts of PM2.5 on the metabolic system. To our knowledge, only 3 previous studies investigated the relationships between PM2.5 components and different components of MetS, however, a consistent conclusion has still not been obtained (5, 12, 15). For instance, a cross-sectional study involving 6,628 Chinese adults, found positive correlations between PM2.5 exposure and increased risks of high TG and high FBG. However, no significant results were observed for central obesity, low HDL, and high BP (12). A cross-sectional investigation of adolescents and children observed positive links between PM2.5 and elevated risks of central obesity. However, they found no significant results for high-FBG, high-BP, low-HDL, and high-TG (15). Based on the limited studies and inconsistent results, further studies are warranted to explore which components of MetS are linked to long-term PM2.5 components exposure.
In this nationwide study in China, our objective was to examine the relationships of exposure to PM2.5 components (SO42−, NO3−, NH4+, BC, and OM) with MetS and the components of MetS.
The participants of this study were from a national cohort study of middle-aged and older Chinese individuals called the China Health and Retirement Longitudinal Study (CHARLS) (16). In brief, approximately 21,000 adults who were at least 45 years old were chosen from 150 cities, in 28 provinces in China. Five waves of the CHARLS were completed in 2011, 2013, 2015, 2018, and 2020. Diagnostic indicators of MetS were only measured in the first wave (2011) and third wave (2015), including waist circumference (WC), BP (systolic BP, SBP; and diastolic BP, DBP), blood lipid (TG, HDL) and FBG. Similar to a previous study of CHARLS (17), we found that only one-fourth of participants could be included in a longitudinal study after matching the data of those two surveys. Therefore, we included participants from CHARLS 2015 in the study. A total of 16,406 adults had a physical examination, 3,560 adults were excluded for the reasons of missing WC, BP, TG, HDL, and FBG data. Finally, 12,846 participants were included (Supplementary Figure S1).
WC, BP, FBG, TG, and HDL of individuals were examined in physical examination. Specifically, a soft measuring tape was wrapped around each participant’s waist while they were standing to determine their WC. An electronic blood pressure monitor was worn on the left arm to measure SBP and DBP. The average of the three readings was computed. Fasting venous blood samples were obtained from every individual to determine FBG, TG, and HDL levels.
The Joint Interim Societies’ criteria were used in this study’s diagnosis of MetS (2). In brief, patients with MetS were defined as those who met two or more of the following criteria in addition to having central obesity (WC ≥ 90 cm for men and 80 cm for women): (1) high BP (SBP ≥ 130 mmHg, DBP ≥ 85 mmHg, clinically confirmed hypertension or taking anti-hypertension medicine); (2) high FBG (FBG ≥ 100 mg/dL, clinically confirmed diabetes history, taking anti-diabetes medicine or insulin injections); (3) elevated TG (>150 mg/dL); (4) low-HDL (< 40 mg/dL for men; <50 mg/dL for women).
Full-coverage near-real-time PM2.5 and its 5 major chemical components (SO42−, NO3−, NH4+, OM, and BC) were assessed at 10 km spatial resolution. Briefly, multi-source fusion PM2.5 data, ground observations, and machine learning algorithms were used to predict daily PM2.5 concentrations and components. Previous research provided a more complete description of the PM2.5 measurement methodologies and their chemical components (18–20). The three-year average concentration of PM2.5 concentrations and components for individuals was used to determine long-term exposure, which was in line with most research on the l long-term effects of air pollutants on health (5, 21, 22).
Directed acyclic graph analysis and literature review were conducted to identify the potential confounders (Supplementary Figure S2). These covariates included: (1) meteorological factors: relative humidity, and air temperature; (2) demographic characteristics: age, and sex; (3) socioeconomic characteristics: residence, marital status, and educational attainment; (4) healthy lifestyles and behaviors: smoking, drinking, physical activity, and cooking fuel use. Detailed description of covariates was clarified in our previous studies (4, 21, 23, 24).
A four-stage investigation was utilized to investigate the relationships between PM2.5 chemical components and MetS. First, generalized linear models (GLMs) were used to investigate the links between PM2.5 components and the risk of MetS. Second, we applied a restricted cubic spline to investigate the E-R relationships of PM2.5 components with MetS risk. Third, we used GLMs and RCS analysis to investigate the association of PM2.5 components with the components of MetS and establish the E-R relationships. Finally, subgroup analysis was carried out to determine whether the impact of air pollution on MetS would be modified by participant characteristics. In this stage, we categorized participants into two categories (“<65 years,” and “≥65 years”) using the cut-off of older adult adults. Physical activity was divided into two categories: “sufficient physical activity” and “insufficient physical activity,” according to the World Health Organization’s recommendations (8).
Variety sensitivity assessments were also performed. Firstly, we used two-year mean levels of PM2.5 components to re-examine the relationships of PM2.5 components with MetS and its components (5). Secondly, log-binomial Poisson regressions were conducted to assess the robustness of the positive links of PM2.5 components with MetS and its components (4, 25). Thirdly, individuals who had changed their residential address after the CHARLS 2013 and determine whether those results were altered by address change (21). Finally, taking medications for hypertension, and diabetes could potentially introduce confounding effects on the study results, we excluded those taking medication for hypertension to re-examine the association between PM2.5 components and MetS and high BP. Similarly, individuals taking antidiabetic medication were excluded to re-evaluate the association between PM2.5 components and MetS and high FBG.
Statistical analyses were carried out using R 4.3.1. The “mice” R package was used to impute the missing covariate data (4), and a two-tailed p-value of less than 0.05 was utilized to determine statistical significance.
The study included 12,846 adult participants who were selected from 125 cities located in 28 different Chinese provinces. Figure 1 shows the geographical distribution of participants in 28 provinces and Table 1 presents the basic characteristics. There were 4,357 individuals (33.9%) were diagnosed with MetS. For the indicators of MetS, the mean WC, SBP, DBP, FBG, TG, and HDL were 85.34 ± 13.11 cm, 128.31 ± 19.69 mmHg, 75.46 ± 11.20 mmHg, 103.36 ± 35.19 mg/dL, 142.59 ± 90.92 mg/dL, and 51.16 ± 11.46 mg/dL, respectively.
The descriptive characteristics of PM2.5 chemical components, temperature, and relative humidity are shown in Supplementary Table S1. The three-year mean levels of PM2.5, SO42−, NO3−, NH4+, OM and BC exposure were 52.84 ± 22.70 μg/m3, 10.01 ± 3.94 μg/m3, 11.49 ± 5.85 μg/m3, 8.01 ± 3.59 μg/m3, 12.78 ± 4.85 μg/m3, 2.55 ± 0.82 μg/m3, respectively. Pearson correlation analysis showed high collinearity of PM2.5 chemical components, with the coefficients of correlation varying from 0.901 to 0.995 (Supplementary Table S2).
In the crude and adjusted models, positive relationships between MetS risk and PM2.5, SO42−, NO3−, NH4+, OM, and BC were observed (Figure 2). In the adjusted model 3, the OR values of MetS were 1.351 (95%CI, 1.261, 1.445), 1.335 (95%CI, 1.242, 1.434), 1.336 (95%CI, 1.245, 1.434), 1.312 (95%CI,1.222, 1.409), 1.324 (95%CI,1.238, 1.415), and 1.314 (95%CI, 1.229, 1.406) for every IQR increase in PM2.5 (33.35 μg/m3), SO42− (6.30 μg/m3), NO3− (9.01 μg/m3), NH4+ (5.60 μg/m3), OM (7.21 μg/m3), and BC (1.25 μg/m3), respectively. Figure 3 presents the E-R relationships of PM2.5 components with MetS risk. We discovered that, with increases in PM2.5, SO42−, NO3−, NH4+, OM, and BC, the OR of MetS increased progressively.
Figure 2. Associations of PM2.5 and its compositions with the prevalence of metabolic syndrome (MetS). Crude model, without adjustment; Adjusted model 1, adjusted for temperate and relative humidity; Adjusted model 2, adjusted for temperate, relative humidity, age, sex, residence, marital status, education status; Adjusted Model 3, adjusted for temperate, relative humidity, age, sex, residence, marital status, education status, smoking status, drinking status, physical activity and cooking fuel use. *p-value < 0.05, **p-value < 0.01, ***p-value < 0.001.
Figure 3. Exposure-response relationship between long-term exposure to PM2.5 and its chemical components with metabolic syndrome (MetS) risk.
Figure 4 and Supplementary Table S3 present the GLM analysis of the relationships of PM2.5 components with the risks of MetS components. We discovered that exposure to the chemical components of PM2.5 was linked to a higher risk of central adiposity, high blood pressure, elevated FBG, and low HDL. As for high TG risk, NO3− and NH4+ showed negative relationships, whereas PM2.5, SO42−, OM, and BC showed no significant associations.
Figure 4. Associations between PM2.5 and its chemical components with the components of MetS. Adjusted for temperate, relative humidity, age, sex, residence, marital status, education status, smoking status, drinking status, physical activity and cooking fuel use.
The E-R relationships between PM2.5 chemical components and the components of MetS are displayed in Figure 5. Except for high TG, we found that the risks for central obesity, high BP, high FBG, and low HDL increased gradually with rising levels of PM2.5, SO42−, NO3−, NH4+, OM, and BC (p-value < 0.05).
Figure 5. Exposure-response relationship between exposure to PM2.5 chemical components with the components of metabolic syndrome (MetS).
The findings of the subgroup analysis by participant character are displayed in Table 2. We discovered that older adult adults (≥65 years), females, participants with higher education levels, non-smokers, drinkers, and solid fuel users were more susceptible to PM2.5 components, even if the P-interaction showed no statistical significance. Moreover, people who were single, divorced, or widowed were more vulnerable to PM2.5 components than married people, according to subgroup analysis by marital status (P-interaction < 0.05).
Table 2. Subgroup analysis for the associations of long-term exposure to PM2.5 chemical components with MetS.
The sensitivity analysis results are shown in Supplementary Tables S4–S8. For the relationships of PM2.5 components with MetS risk, all sensitivity analyses showed positive and significant results for PM2.5 concentration and components, which were all consistent with the main effects models. As for the components of MetS, consistent results and positive associations were also observed in all sensitivity analyses. Except for the relationships of SO42− with high TG after excluding individuals who had altered their residential address, insignificant associations were shown for PM2.5, SO42−, OM, BC, and negative associations were found for NO3−, NH4+ in all sensitivity analyses, which were consistent with the main effects models. The sensitivity analysis excluding users of anti-hypertensive or anti-diabetic medication yielded consistent and robust results.
This nationwide cross-sectional investigation in China discovered that exposure to PM2.5 chemical components (SO42−, NO3−, NH4+, OM, and BC) was significantly linked to an elevated risk of MetS and its components, except for high TG. To our knowledge, the current study may be the first nationwide study examining the long-term impact of PM2.5 components on MetS and its components. Furthermore, we discovered that single, divorced, or widowed persons were more vulnerable to the harmful effects of PM2.5 components exposure on MetS than those married adults.
Our research revealed a positive association between PM2.5 and MetS risk. Previous studies also have reported similar findings (5, 12, 14, 15, 22, 26, 27). For instance, a meta-analysis revealed that for every 5 μg/m3 increase in PM2.5, the risk of MetS increased by 14% (RR = 1.14, 95%CI: 1.03, 1.25) (10). According to the KORA F4/FF4 cohort study, there was a 14% (OR = 1.14, 95%CI: 1.02, 1.28) increase in MetS risk for every 1.4 μg/m3 rise in PM2.5 (27). The China Multi-Ethnic Cohort research showed that with every 29.55 μg/m3 increase in PM2.5, the OR value of MetS was 1.38 (95%CI, 1.23, 1.55) (22). Our research’s effect estimations were comparable to those of Feng et al.’s (22) study but lower than those of Voss et al.’ s study and Ning et al.’s study, which might be ascribed to differences in study subjects, chemical components of PM2.5, study areas, and sample size (5).
As a mixture of primary and secondary pollutants, the harmful impacts of PM2.5 components also should be noticed. Our study indicated that exposure to SO42−, NO3−, NH4+, OM, and BC were related to elevated MetS risk. Several investigations examined the relationship of PM2.5 components with MetS risk (14, 28), and most of the findings supported the findings of this investigation. A cross-sectional study involving 10,066 Chinese adolescents indicated that the OR values of MetS were1.14 (95%CI: 1.04, 1.24), 1.09 (95%CI: 1.04, 1.13), 1.07 (95%CI: 1.04, 1.11) and 1.24 (95%CI, 1.14, 1.35), for every 1 μg/m3 rise in SO42−, NO3−, OM, BC, respectively. The SCOPA-China Cohort study found that each 3.76 μg/m3 rise in SO42− was linked with a 13.3% (OR = 1.133, 95%CI: 1.053, 1.220) rise in MetS risk. However, no significant results were found for NO3−, NH4+. The in-significant results might be explained by the limited sample size (n = 2045) and region (Beijing-Tianjin-Hebei region). Compared with Yi et al.’s study, our study provided new evidence that exposure to OM and BC would increase MetS risk. In addition to the few investigations on PM2.5 components and MetS, several published research have found that exposure to PM2.5 components was related to an elevated risk of MetS-related disorders such as hypertension (29), and diabetes (30, 31), which could also support our findings. Overall, the positive relationships between MetS risk and PM2.5 chemical components were validated by this nationwide cross-sectional investigation.
This study showed positive links between the chemical components of PM2.5 and the risks of central obesity, high BP, high FBG, and low HDL. A Chinese cross-sectional study of adolescents found similar positive relationships (28). They found that exposure to SO42−, NO3−, BC, and OM were related to elevated central obesity risk, and exposure to NO3−, OM, and BC were linked with elevated high BP risk (28). Several studies focused on a specific component of MetS could also support our findings (32, 33). For example, a Chinese cross-sectional study reported the positive relationships of SO42−, NO3−, NH4+, OM, BC with FBG levels, NO3− and BC with SBP levels, and NO3−, NH4+, OM with DBP levels (32). However, no significant relationships between SO42−, OM, and BC and high TG risk were found in this investigation, which could be attributed to the various health of PM2.5 chemical components, sample size, and techniques of air pollutants measurement.
In subgroup analysis, we found that marital status could modify the impact of PM2.5 chemical components on MetS risk. When comparing the OR values of MetS in different marital status groups, we found that people who were single, divorced, or widowed had a higher risk of MetS than married individuals, with significant P-interaction values for PM2.5, SO42−, NO3−, NH4+, OM, and BC. The modification effect of marital status could be explained by lower socioeconomic status among single, divorced, or divorced adults than that of married adults (34). Firstly, individuals who were single, divorced, or divorced might have a significantly increased chance of exposure to severe PM2.5 pollution (35). Secondly, individuals who were single, divorced, or divorced may tend to have less access to social and healthcare support, resulting in poorer health outcomes and less engagement in measures to reduce exposure to air pollution (36–39).
Although the biological mechanisms of PM2.5 components on MetS were still unknown (10), several possible biological mechanisms focusing on PM2.5 mass have been proposed. Firstly, PM2.5 can get into the circulatory systems through the respiratory tract, causing oxidative stress and systematic inflammation and, leading to body weight increase (40), blood pressure rises (41), glucose metabolism disorder (42), and lipid metabolism disorders (4, 28, 43, 44). Secondly, PM2.5 could lead to autonomic nervous system dysfunction by activating pulmonary autonomic reflections (45, 46), causing elevated blood pressure (43), insulin resistance (47), and lipid metabolism disorders (48). Thirdly, epigenetic changes, such as aberrant methylation of DNA, have been recognized as critical biological mechanisms of exposure to PM2.5-induced metabolism (49). Additionally, PM2.5 may cause renin-angiotensin-aldosterone pathway dysfunction (4), leading to structural and functional kidney changes (50) and resulting in higher BP and elevated hypertension risks (51).
Some limitations need to be recognized. Firstly, due to the cross-sectional design of this study, the cause-and-effect cannot be concluded. Longitudinal studies should be conducted to strengthen our findings. Secondly, since the high co-linearity between PM2.5 chemical components was observed, a multi-pollutants model could not be performed. Thirdly, the collection of hypertension, diabetes, and most covariate data relied on self-report questionnaires, which introduces the possibility of reporting bias and recall bias. Fourthly, although missing values for covariates such as education status, cooking fuel use, and physical activity were imputed using the Monte Carlo method in this study, it should be acknowledged that there may still be some degree of error concerning the actual values. Fifthly, some participants are currently taking medications for hypertension, diabetes, and lipid-lowering, which could potentially introduce confounding effects on the study results. However, the sensitivity analysis excluding users of anti-hypertensive or anti-diabetic medication yielded consistent and robust results. The use of lipid-lowering medications was not investigated in CHARLS. Confounding factors related to the use of these medications should be considered in future studies. Finally, it should be noted that due to the lack of data on dietary factors and other lifestyle variables in the CHARLS survey, potential confounders may still exist. However, meteorological factors, demographic characteristics, socioeconomic characteristics, health lifestyles, and behaviors have been adjusted in our study, and consistent outcomes from crude and adjusted models served as evidence of the robustness of our findings. Further studies incorporating control for the confounding effects of dietary factors are necessary to validate our findings.
The present research found that long-term exposure to PM2.5 components was related to an elevated risk of MetS and its components, including central obesity, high FBG, high BP, and low HDL. The adverse effect of PM2.5 chemical components on MetS was more sensitive to people who were single, divorced, or widowed than married people. Our study provides new epidemiological insights into the potential adverse impacts of PM2.5 components on the metabolic system, and the modification effect of marital status. Further longitudinal studies should be carried out to confirm our findings.
The raw data supporting the conclusions of this article will be made available by the authors, without undue reservation.
The CHARLS study received approval from the Institutional Review Board of Peking University (Code: IRB00001052-11015). The studies were conducted in accordance with the local legislation and institutional requirements. The participants provided their written informed consent to participate in this study.
JJZ: Conceptualization, Data curation, Formal analysis, Visualization, Writing – original draft. JLZ: Conceptualization, Data curation, Formal analysis, Software, Writing – original draft. ZD: Conceptualization, Data curation, Visualization, Writing – original draft. JN: Methodology, Software, Visualization, Writing – original draft. XL: Methodology, Software, Visualization, Writing – review & editing. WY: Visualization, Writing – original draft. ZN: Conceptualization, Methodology, Software, Writing – review & editing. YY: Conceptualization, Methodology, Software, Writing – review & editing.
The author(s) declare that no financial support was received for the research, authorship, and/or publication of this article.
The authors are grateful to the survey staff and all participants of the CHARLS.
The authors declare that the research was conducted in the absence of any commercial or financial relationships that could be construed as a potential conflict of interest.
All claims expressed in this article are solely those of the authors and do not necessarily represent those of their affiliated organizations, or those of the publisher, the editors and the reviewers. Any product that may be evaluated in this article, or claim that may be made by its manufacturer, is not guaranteed or endorsed by the publisher.
The Supplementary material for this article can be found online at: https://www.frontiersin.org/articles/10.3389/fpubh.2024.1462548/full#supplementary-material
1. Eckel, RH, Grundy, SM, and Zimmet, PZ. The metabolic syndrome. Lancet. (2005) 365:1415–28. doi: 10.1016/S0140-6736(05)66378-7
2. Alberti, KG, Zimmet, P, and Shaw, J. The metabolic syndrome--a new worldwide definition. Lancet. (2005) 366:1059–62. doi: 10.1016/S0140-6736(05)67402-8
3. Pucci, G, Alcidi, R, Tap, L, Battista, F, Mattace-Raso, F, and Schillaci, G. Sex- and gender-related prevalence, cardiovascular risk and therapeutic approach in metabolic syndrome: a review of the literature. Pharmacol Res. (2017) 120:34–42. doi: 10.1016/j.phrs.2017.03.008
4. Han, S, Zhang, F, Yu, H, Wei, J, Xue, L, Duan, Z, et al. Systemic inflammation accelerates the adverse effects of air pollution on metabolic syndrome: findings from the China health and retirement longitudinal study (CHARLS). Environ Res. (2022) 215:114340. doi: 10.1016/j.envres.2022.114340
5. Liu, F, Wang, X, Pan, M, Zhang, K, Zhou, F, Tong, J, et al. Exposure to air pollution and prevalence of metabolic syndrome: a nationwide study in China from 2011 to 2015. Sci Total Environ. (2023) 855:158596. doi: 10.1016/j.scitotenv.2022.158596
6. Tang, X, Wu, M, Wu, S, and Tian, Y. Continuous metabolic syndrome severity score and the risk of CVD and all-cause mortality. Eur J Clin Investig. (2022) 52:e13817. doi: 10.1111/eci.13817
7. Baffi, CW, Wood, L, Winnica, D, Strollo, PJ, Gladwin, MT, Que, LG, et al. Metabolic syndrome and the lung. Chest. (2016) 149:1525–34. doi: 10.1016/j.chest.2015.12.034
8. Guo, Q, Zhao, Y, Zhao, J, Bian, M, Qian, L, Xue, T, et al. Physical activity attenuated the associations between ambient air pollutants and metabolic syndrome (MetS): a nationwide study across 28 provinces. Environ Pollut. (2022) 315:120348. doi: 10.1016/j.envpol.2022.120348
9. Zang, ST, Luan, J, Li, L, Wu, QJ, Chang, Q, Dai, HX, et al. Air pollution and metabolic syndrome risk: evidence from nine observational studies. Environ Res. (2021) 202:111546. doi: 10.1016/j.envres.2021.111546
10. Ning, J, Zhang, Y, Hu, H, Hu, W, Li, L, Pang, Y, et al. Association between ambient particulate matter exposure and metabolic syndrome risk: a systematic review and meta-analysis. Sci Total Environ. (2021) 782:146855. doi: 10.1016/j.scitotenv.2021.146855
11. Hernández-Pérez, JG, Torres-Sánchez, L, Hernández-Alcaráz, C, López-Carrillo, L, Rodríguez-Covarrubias, F, Vázquez-Salas, RA, et al. Metabolic syndrome and prostate Cancer risk: a population case-control study. Arch Med Res. (2022) 53:594–602. doi: 10.1016/j.arcmed.2022.07.003
12. Zheng, X-y, Tang, S-l, Liu, T, Wang, Y, Xu, X-j, Xiao, N, et al. Effects of long-term PM2.5 exposure on metabolic syndrome among adults and elderly in Guangdong, China. Environ Health. (2022) 21:84. doi: 10.1186/s12940-022-00888-2
13. Yu, Y, Paul, K, Arah, OA, Mayeda, ER, Wu, J, Lee, E, et al. Air pollution, noise exposure, and metabolic syndrome – a cohort study in elderly Mexican-Americans in Sacramento area. Environ Int. (2020) 134:105269. doi: 10.1016/j.envint.2019.105269
14. Yi, W, Zhao, F, Pan, R, Zhang, Y, Xu, Z, Song, J, et al. Associations of fine particulate matter constituents with metabolic syndrome and the mediating role of apolipoprotein B: a multicenter study in middle-aged and elderly Chinese adults. Environ Sci Technol. (2022) 56:10161–71. doi: 10.1021/acs.est.1c08448
15. Zhang, JS, Gui, ZH, Zou, ZY, Yang, BY, Ma, J, Jing, J, et al. Long-term exposure to ambient air pollution and metabolic syndrome in children and adolescents: a national cross-sectional study in China. Environ Int. (2021) 148:106383. doi: 10.1016/j.envint.2021.106383
16. Zhao, Y, Hu, Y, Smith, JP, Strauss, J, and Yang, G. Cohort profile: the China health and retirement longitudinal study (CHARLS). Int J Epidemiol. (2014) 43:61–8. doi: 10.1093/ije/dys203
17. Liu, F, Zhang, K, Chen, G, He, J, Pan, M, Zhou, F, et al. Sustained air pollution exposures, fasting plasma glucose, glycated haemoglobin, prevalence and incidence of diabetes: a nationwide study in China. Int J Epidemiol. (2022) 51:1862–73. doi: 10.1093/ije/dyac162
18. Liu, S, Geng, G, Xiao, Q, Zheng, Y, Liu, X, Cheng, J, et al. Tracking daily concentrations of PM(2.5) chemical composition in China since 2000. Environ Sci Technol. (2022) 56:16517–27. doi: 10.1021/acs.est.2c06510
19. Geng, G, Xiao, Q, Liu, S, Liu, X, Cheng, J, Zheng, Y, et al. Tracking air pollution in China: near real-time PM(2.5) retrievals from multisource data fusion. Environ Sci Technol. (2021) 55:12106–15. doi: 10.1021/acs.est.1c01863
20. Xiao, Q, Geng, G, Cheng, J, Liang, F, Li, R, Meng, X, et al. Evaluation of gap-filling approaches in satellite-based daily PM2.5 prediction models. Atmos Environ. (2021) 244:117921. doi: 10.1016/j.atmosenv.2020.117921
21. Niu, Z, Duan, Z, Wei, J, Wang, F, Han, D, Zhang, K, et al. Associations of long-term exposure to ambient ozone with hypertension, blood pressure, and the mediation effects of body mass index: a national cross-sectional study of middle-aged and older adults in China. Ecotoxicol Environ Saf. (2022) 242:113901. doi: 10.1016/j.ecoenv.2022.113901
22. Feng, S, Meng, Q, Guo, B, Guo, Y, Chen, G, Pan, Y, et al. Joint exposure to air pollution, ambient temperature and residential greenness and their association with metabolic syndrome (MetS): a large population-based study among Chinese adults. Environ Res. (2022) 214:113699. doi: 10.1016/j.envres.2022.113699
23. Zhou, Q, Li, X, Zhang, J, Duan, Z, Mao, S, Wei, J, et al. Long-term exposure to PM(1) is associated with increased prevalence of metabolic diseases: evidence from a nationwide study in 123 Chinese cities. Environ Sci Pollut Res Int. (2023) 31:549–63. doi: 10.1007/s11356-023-31098-z
24. Zhang, J, Zhang, F, Xin, C, Duan, Z, Wei, J, Zhang, X, et al. Associations of long-term exposure to air pollution, physical activity with blood pressure and prevalence of hypertension: the China health and retirement longitudinal study. Front Public Health. (2023) 11:1137118. doi: 10.3389/fpubh.2023.1137118
25. Ning, Y, Lam, A, and Reilly, M. Estimating risk ratio from any standard epidemiological design by doubling the cases. BMC Med Res Methodol. (2022) 22:157. doi: 10.1186/s12874-022-01636-3
26. Wang, Y, Liu, F, Yao, Y, Chen, M, Wu, C, Yan, Y, et al. Associations of long-term exposure to ambient air pollutants with metabolic syndrome: the Wuhan chronic disease cohort study (WCDCS). Environ Res. (2022) 206:112549. doi: 10.1016/j.envres.2021.112549
27. Voss, S, Schneider, A, Huth, C, Wolf, K, Markevych, I, Schwettmann, L, et al. ENVINT-D-20-01309: long-term exposure to air pollution, road traffic noise, residential greenness, and prevalent and incident metabolic syndrome: results from the population-based KORA F4/FF4 cohort in Augsburg. Germany Environ Int. (2021) 147:106364. doi: 10.1016/j.envint.2020.106364
28. Li, J, Song, Y, Shi, L, Jiang, J, Wan, X, Wang, Y, et al. Long-term effects of ambient PM(2.5) constituents on metabolic syndrome in Chinese children and adolescents. Environ Res. (2023) 220:115238. doi: 10.1016/j.envres.2023.115238
29. Liu, L, Zhang, Y, Yang, Z, Luo, S, and Zhang, Y. Long-term exposure to fine particulate constituents and cardiovascular diseases in Chinese adults. J Hazard Mater. (2021) 416:126051. doi: 10.1016/j.jhazmat.2021.126051
30. Zhou, P, Mo, S, Peng, M, Yang, Z, Wang, F, Hu, K, et al. Long-term exposure to PM(2.5) constituents in relation to glucose levels and diabetes in middle-aged and older Chinese. Ecotoxicol Environ Saf. (2022) 245:114096. doi: 10.1016/j.ecoenv.2022.114096
31. Kang, N, Wu, R, Liao, W, Zhang, C, Liu, X, Mao, Z, et al. Association of long-term exposure to PM(2.5) constituents with glucose metabolism in Chinese rural population. Sci Total Environ. (2023) 859:160364. doi: 10.1016/j.scitotenv.2022.160364
32. Du, X, Zhang, Y, Liu, C, Fang, J, Zhao, F, Chen, C, et al. Fine particulate matter constituents and sub-clinical outcomes of cardiovascular diseases: a multi-center study in China. Sci Total Environ. (2021) 759:143555. doi: 10.1016/j.scitotenv.2020.143555
33. He, ZZ, Guo, PY, Xu, SL, Zhou, Y, Jalaludin, B, Leskinen, A, et al. Associations of particulate matter sizes and chemical constituents with blood lipids: a panel study in Guangzhou. China Environ Sci Technol. (2021) 55:5065–75. doi: 10.1021/acs.est.0c06974
34. Tu, R, Hou, J, Liu, X, Li, R, Dong, X, Pan, M, et al. Low socioeconomic status aggravated associations of exposure to mixture of air pollutants with obesity in rural Chinese adults: a cross-sectional study. Environ Res. (2021) 194:110632. doi: 10.1016/j.envres.2020.110632
35. Stucki, L, Betnér, S, Selander, J, Lõhmus, M, Åkesson, A, and Eriksson, C. Sociodemographic inequalities in long-term exposure to air pollution, road traffic noise, and greenness: a population-based cohort study of women. Environ Epidemiol. (2023) 7:e279. doi: 10.1097/EE9.0000000000000279
36. Xiao, M, Li, A, Wang, Y, Yu, C, Pang, Y, Pei, P, et al. A wide landscape of morbidity and mortality risk associated with marital status in 0.5 million Chinese men and women: a prospective cohort study. Lancet Reg Health West Pac. (2024) 42:100948. doi: 10.1016/j.lanwpc.2023.100948
37. Leung, CY, Huang, HL, Abe, SK, Saito, E, Islam, MR, Rahman, MS, et al. Association of marital status with total and cause-specific mortality in Asia. JAMA Netw Open. (2022) 5:e2214181. doi: 10.1001/jamanetworkopen.2022.14181
38. Schultz, WM, Hayek, SS, Samman Tahhan, A, Ko, YA, Sandesara, P, Awad, M, et al. Marital status and outcomes in patients with cardiovascular disease. J Am Heart Assoc. (2017) 6:890. doi: 10.1161/jaha.117.005890
39. Kim, H, Cho, J, Isehunwa, O, Noh, J, Noh, Y, Oh, SS, et al. Marriage as a social tie in the relation of depressive symptoms attributable to air pollution exposure among the elderly. J Affect Disord. (2020) 272:125–31. doi: 10.1016/j.jad.2020.04.059
40. Liu, X, Tu, R, Qiao, D, Niu, M, Li, R, Mao, Z, et al. Association between long-term exposure to ambient air pollution and obesity in a Chinese rural population: the Henan rural cohort study. Environ Pollut. (2020) 260:114077. doi: 10.1016/j.envpol.2020.114077
41. Li, Z, Liu, Y, Lu, T, Peng, S, Liu, F, Sun, J, et al. Acute effect of fine particulate matter on blood pressure, heart rate and related inflammation biomarkers: a panel study in healthy adults. Ecotoxicol Environ Saf. (2021) 228:113024. doi: 10.1016/j.ecoenv.2021.113024
42. Liu, F, Guo, Y, Liu, Y, Chen, G, Wang, Y, Xue, X, et al. Associations of long-term exposure to PM(1), PM(2.5), NO(2) with type 2 diabetes mellitus prevalence and fasting blood glucose levels in Chinese rural populations. Environ Int. (2019) 133:105213. doi: 10.1016/j.envint.2019.105213.
43. Niu, Z, Duan, Z, Yu, H, Xue, L, Liu, F, Yu, D, et al. Association between long-term exposure to ambient particulate matter and blood pressure, hypertension: an updated systematic review and meta-analysis. Int J Environ Health Res. (2022) 33:268–83. doi: 10.1080/09603123.2021.2022106
44. Tang, H, Cheng, Z, Li, N, Mao, S, Ma, R, He, H, et al. The short- and long-term associations of particulate matter with inflammation and blood coagulation markers: a meta-analysis. Environ Pollut. (2020) 267:115630. doi: 10.1016/j.envpol.2020.115630
45. Niu, Z, Liu, F, Li, B, Li, N, Yu, H, Wang, Y, et al. Acute effect of ambient fine particulate matter on heart rate variability: an updated systematic review and meta-analysis of panel studies. Environ Health Prev Med. (2020) 25:77. doi: 10.1186/s12199-020-00912-2
46. Li, N, Chen, G, Liu, F, Mao, S, Liu, Y, Hou, Y, et al. Associations of long-term exposure to ambient PM(1) with hypertension and blood pressure in rural Chinese population: the Henan rural cohort study. Environ Int. (2019) 128:95–102. doi: 10.1016/j.envint.2019.04.037
47. Sun, J, Peng, S, Li, Z, Liu, F, Wu, C, Lu, Y, et al. Association of Short-Term Exposure to PM(2.5) with blood lipids and the modification effects of insulin resistance: a panel study in Wuhan. Toxics. (2022) 10:663. doi: 10.3390/toxics10110663
48. Mao, S, Li, S, Wang, C, Liu, Y, Li, N, Liu, F, et al. Is long-term PM(1) exposure associated with blood lipids and dyslipidemias in a Chinese rural population? Environ Int. (2020) 138:105637. doi: 10.1016/j.envint.2020.105637
49. Sun, B, Shi, Y, Yang, X, Zhao, T, Duan, J, and Sun, Z. DNA methylation: a critical epigenetic mechanism underlying the detrimental effects of airborne particulate matter. Ecotoxicol Environ Saf. (2018) 161:173–83. doi: 10.1016/j.ecoenv.2018.05.083
50. Peng, S, Lu, T, Liu, Y, Li, Z, Liu, F, Sun, J, et al. Short-term exposure to fine particulate matter and its constituents may affect renal function via oxidative stress: a longitudinal panel study. Chemosphere. (2022) 293:133570. doi: 10.1016/j.chemosphere.2022.133570
51. Aztatzi-Aguilar, OG, Uribe-Ramírez, M, Arias-Montaño, JA, Barbier, O, and De Vizcaya-Ruiz, A. Acute and subchronic exposure to air particulate matter induces expression of angiotensin and bradykinin-related genes in the lungs and heart: angiotensin-II type-I receptor as a molecular target of particulate matter exposure. Part Fibre Toxicol. (2015) 12:17. doi: 10.1186/s12989-015-0094-4
Keywords: air pollution, particulate matter, metabolic dysfunction, middle-aged and older adults, marital status
Citation: Zhang J, Zhang J, Duan Z, Nie J, Li X, Yu W, Niu Z and Yan Y (2024) Association between long-term exposure to PM2.5 chemical components and metabolic syndrome in middle-aged and older adults. Front. Public Health. 12:1462548. doi: 10.3389/fpubh.2024.1462548
Received: 10 July 2024; Accepted: 08 August 2024;
Published: 21 August 2024.
Edited by:
Yichen Wang, Xi’an Jiaotong University, ChinaCopyright © 2024 Zhang, Zhang, Duan, Nie, Li, Yu, Niu and Yan. This is an open-access article distributed under the terms of the Creative Commons Attribution License (CC BY). The use, distribution or reproduction in other forums is permitted, provided the original author(s) and the copyright owner(s) are credited and that the original publication in this journal is cited, in accordance with accepted academic practice. No use, distribution or reproduction is permitted which does not comply with these terms.
*Correspondence: Zhiping Niu, enBuaXUyM0BtLmZ1ZGFuLmVkdS5jbg==; Yangjin Yan, ZGFtYW83MThAMTI2LmNvbQ==
†These authors have contributed equally to this work
Disclaimer: All claims expressed in this article are solely those of the authors and do not necessarily represent those of their affiliated organizations, or those of the publisher, the editors and the reviewers. Any product that may be evaluated in this article or claim that may be made by its manufacturer is not guaranteed or endorsed by the publisher.
Research integrity at Frontiers
Learn more about the work of our research integrity team to safeguard the quality of each article we publish.