- 1Department of Healthcare Administration and Medical Informatics, College of Health Sciences, Kaohsiung Medical University, Kaohsiung City, Taiwan
- 2Department of Medical Research, Kaohsiung Medical University Hospital, Kaohsiung Medical University, Kaohsiung City, Taiwan
- 3Center for Big Data Research, Kaohsiung Medical University, Kaohsiung City, Taiwan
- 4Department of Public Health, College of Health Sciences, Kaohsiung Medical University, Kaohsiung City, Taiwan
- 5Division of Medical Statistics and Bioinformatics, Department of Medical Research, Kaohsiung Medical University Hospital, Kaohsiung Medical University, Kaohsiung City, Taiwan
Background: Taiwan implemented global hospital budgeting with a floating-point value, which created a prisoner's dilemma. As a result, hospitals increased service volume, which caused the floating-point value to drop to less than one New Taiwan Dollar (NTD). The recent increase in the number of hospital beds and the call to enhance the floating-point value to one NTD raise concerns about the potential for increased financial burden without adding value to patient care if hospitals expand their bed capacity for volume-based competition. The present study aimed to examine the relationship between the supply of hospital beds and hospitalizations following an emergency department (ED) visit (called ED hospitalizations) by using diabetes-related ambulatory care sensitive conditions (ACSCs) that are preventable and discretionary as an example.
Methods: The study was a pooled cross-sectional design analyzing 2011–2015 population-based claims data in Taiwan. The dependent variable was a dummy variable representing an ED hospitalization, with a treat-and-leave ED visit as the reference group. The key independent variable is the number of hospital beds per 1,000 populations. Multivariate logistic regression models with and without a clustering function were used for the analyses.
Results: Approximately 59.26% of diabetes-related ACSCs ED visits resulted in ED hospitalizations. The relationship between the supply of hospital beds and ED hospitalizations was statistically significant (OR = 1.12; 95% CI: 1.09–1.14; P < 0.001) in the model without clustering but was statistically insignificant in the model with clustering (OR = 1.03; 95% CI: 0.94–1.12; P > 0.05). Several social risk factors were positively associated with the likelihood of ED hospitalizations, such as low income and the percentage of the population without a high school diploma. In contrast, other factors, such as female patients and the Charlson comorbidity index, were negatively associated with the likelihood of ED hospitalizations.
Conclusion: Under hospital global budgeting with a floating-point value mechanism, increases in hospital beds likely motivate hospitals to admit ED patients with preventable and discretionary conditions. Our study emphasizes the urgent need to add value-based incentive mechanisms to the current global budget payment. The value-based incentive mechanisms may encourage providers to focus on quality of patient care by addressing social risk factors rather than engage in volume-based competition, which would improve population health while reducing preventable ED visits and hospitalizations.
Introduction
Hospital expenditure is the largest share of total healthcare spending in many countries (1, 2). Payers have used hospital global budgeting to contain escalating expenditures by sharing financial accountability with hospitals and encouraging hospitals to work with other providers to reduce unnecessary care or preventable hospitalizations (3–6). There are different forms of global budgeting. For example, the Maryland Total Cost of Care Model in the United States implemented a global budget without price adjustments for each service, while Taiwan and Germany implemented a global budget with price adjustments (7). The variations in global budgeting would likely affect providers' behaviors differently.
In 1995, Taiwan implemented the national health insurance (NHI) program with a single-payer system, operated by the National Health Insurance Administration (NHIA). At the beginning of NHI, providers were paid by the fee-for-service payment system. Because of the fast-growing health expenditure, the NHIA adopted the global budget payment with price adjustments through a floating-point value mechanism and gradually applied the payment to dental, Chinese medicine, and Western medicine in clinics and hospitals in 1998, 2000, 2001, and 2002, respectively. The amount of the global budget nationally depends on the total expenditure in the previous year, the change in population characteristics and providers' operating costs, the growth of the economy, and policies, such as care for high-risk populations and remote areas (8).
The features of the global budget payment with a floating-point value mechanism in Taiwan are a mixed prospective and retrospective payment. The amount of budget to pay providers is predetermined before the start of the calendar year, which is prospective. A floating-point value is equivalent to the total predetermined budget divided by the total service volume rendered by all providers in the market, which is retrospective. Using the hospital industry as an example, an individual hospital's total payment is a floating-point value multiplied by the total service volume delivered by the hospital. Given a floating-point value mechanism, hospitals do not know their total payment until the NHIA counts the service volume from all hospitals and calculates a floating-point value per service volume.
Hospital global budgeting with a floating-point value mechanism creates the phenomenon called prisoner's dilemma to hospitals (9, 10), because the total payment to an individual hospital depends on the volume of services provided by the hospital and other hospitals in the same market. Ideally, it would be good if all hospitals agreed to produce a specific volume to keep a floating-point value at their desired value. However, an individual hospital is unlikely to know the other hospitals' service volume; therefore, an individual hospital is willing to increase its service volume to secure its share from the predetermined budget. Empirical evidence from Taiwan showed that, in response to the challenges of prisoner's dilemma, hospitals engaged in volume-based competition. This included increasing services with high price/cost margins (e.g., radiology), as well as boosting the number of prescriptions, procedures, and length of stays (9–11). Due to volume-based competition, the floating-point value was, on average, less than one New Taiwan Dollar (NTD).
Hospitals may also increase their capacities, allowing them to compete with others regarding service volume. Recently, medical centers and chain hospitals in Taiwan have expanded the number of hospital beds. More than 3,000 new hospital beds are either under reconstruction or have been approved and will soon enter the market, reaching 4.5 hospital beds per 1,000 populations. This would be higher than the average of 4.3 hospital beds per 1,000 populations in the Organization for Economic Cooperation and Development (OECD) countries (12). However, evidence regarding the association between the supply of hospital beds and hospitalization rates, especially for emergency department (ED) hospitalizations under the global budget with the price adjustment payment system, is limited.
The present study aimed to examine the association between the supply of hospital beds and ED hospitalizations. We chose diabetes-related ambulatory care sensitive conditions (ACSCs) as an example because ED hospitalizations due to diabetes-related ACSCs are preventable and discretionary. Defined by the Prevention Quality Indicators (PQI) program in the Agency for Healthcare Research and Quality (AHRQ), diabetes-related ACSCs include short-term complications (e.g., ketoacidosis with/without coma), long-term complications (e.g., diabetic nephropathy or retinopathy), uncontrollable diabetes (hypo/hyper-glycemia with or without coma), and lower-extremity amputation. Hospitalizations due to those conditions are theoretically preventable if patients can receive proper care in the community. Furthermore, there is a high variation in ED physicians' decisions regarding ED admissions, which cannot be explained by patients' clinic conditions (13–15). Thus, ED hospitalizations due to diabetes-related ACSCs are considered discretionary.
Significance of the study
The concept of “supply induces demand” is commonly supported by studies based on the fee-for-service payment system, activity-based payment, or diagnosis-related group (DRG) payment (16–22). However, the results of ED hospitalizations are mixed. For example, in England, O'Cathain et al. (21) examined a broad definition of preventable hospitalizations (e.g., cellulitis and injury) at the hospital level and found that the supply of hospital beds was positively associated with the likelihood of ED hospitalizations. By using all ED conditions, the study from Sweden showed that ED patients were likely to be hospitalized when hospital beds were available (14); however, the study from the Medicare fee-for-service population in the United States showed a negative association between the supply of hospital beds and ED hospitalizations (22, 23). As many countries have moved payment systems away from the fee-for-service payment toward a global budget payment (14, 24), testing a theoretical assumption about the prisoner's dilemma by utilizing preventable and discretionary conditions is needed.
With the challenge of unpredictable revenue under the predetermined budget payment with price adjustments through a floating-point value mechanism, hospitals in Taiwan provide a good case to test the theoretical assumption (9, 10). Based on previous evidence in Taiwan (9–11, 25), we hypothesized a positive association between the supply of hospital beds and the likelihood of ED hospitalizations for preventable and discretionary conditions. Most importantly, the NHIA in Taiwan faces a significant challenge from providers that call for enhancing the budget to pay hospitals by increasing a floating-point value from less than 1 NTD to 1 NTD. If the supply of hospital beds is positively associated with discretionary and preventable hospitalizations, increasing the floating-point value would increase the financial burden on the NHIA without adding the value of care for patients. The findings in the present study could have important implications for healthcare policies.
Materials and methods
Data sources
The present study used six data sources: (1) the 2011–2015 National Health Insurance Research Database (NHIRD), (2) the Medical Care Institution file, (3) the Ministry of Interior Global Information Network (GIN), (4) the Taiwan Medical Association, and (5) DATA.GOV.TW, a platform that comprises various data at the township and district levels. The NHIRD provided enrollment and inpatient and outpatient claims data at the patient level. The enrollment file provides the insured's sociodemographic characteristics and residential locations. The inpatient and outpatient claims data that provide the International Classification of Disease 9th Revision, Clinical Modification (ICD-9-CM) for up to three diagnoses and procedures were used to identify ED hospitalizations and patients who were discharged from ED (hereafter called treat-and-leave ED visits) due to diabetes-related ACSCs, respectively. The Medical Care Institution File provides information on whether patients received care at medical centers, regional hospitals, or local hospitals. The GIN provides data concerning the number of individuals with different levels of education and the number of Aboriginginal people in each township. The Taiwan Medical Association provides the number of physicians in the workforce. Finally, DATA.GOV.TW provides the number of hospital beds at the township/district level.
Study design and study sample
The study design was a pooled cross-sectional study using data from 2011 to 2015. We selected this period because the NHIRD started providing the code of ED hospitalizations in the “Patient_source” starting in 2011, and diagnosis codes were coded by ICD-9-CM prior to 2016. The study sample was ED hospitalizations (extracted from inpatient files) and treat-and-leave ED visits (extracted from outpatient files) for individuals aged 20 and above with diabetes-related ACSCs. We applied the ICD-9-CM codes listed by the AHRQ PQI program to identify hospitalizations and ED visits due to diabetes-related ACSCs. We excluded observations with missing data in residential locations and institutions. For the ED hospitalization group, the observations without the code “Patient_source” as ED were excluded. We then merged the ED hospitalizations and treat-and-leave ED visits to form the study sample in the present study. The selection process of the study sample is shown in Figure 1.
Variable measures
Dependent variables
ED hospitalizations due to diabetes-related ACSCs
Figure 1 shows two groups of patients—ED hospitalizations and treat-and-leave ED visits—in the study sample. We created a dichotomous variable for observations with ED hospitalizations, with observations in the treat-and-leave ED visits group as the reference group.
Key independent variable
The key independent variable is the supply of hospital beds, which is measured as a continuous variable by the number of general hospital beds per 1,000 populations at the township/district level.
Control variables
Based on the ACSC-related literature, the likelihood of ED hospitalizations is associated with patients' health conditions and the context of organizations and communities (13, 14, 22, 26–28). Thus, these variables were included in the analytical models. Patient characteristics included age (50–64, 65–79, and 80+, with 20–49 as the reference group), sex (female, with male as the reference group), ethnicity, low income, education level, and the Charlson comorbidity index (29). Ethnicity was proxied by a dummy variable representing the townships/districts with the percentage of Aboriginal people at the 75 percentile and above. A dummy variable measured low-income status if individuals qualified for free premiums. Education was proxied by the percentage of the population without a high school diploma at the township/district level. Organization factors included two dummy variables: regional and local hospitals, with medical centers as the reference group. Community characteristics included the number of primary care physicians per 1,000 populations and two dummy variables representing rural and suburban areas, with urban as the reference group based on the findings from Liu et al.'s (30) study. Finally, we also added year dummies, with 2010 as the reference group to capture potential changes due to time.
Analytical approach
We conducted t-tests for continuous variables and chi-squared tests for categorical variables to compare the differences in study variables between ED hospitalizations and treat-and-leave ED visits. To observe the likelihood of ED hospitalizations associated with the supply of hospital beds, we applied multivariate logistic regression models with ED visits as the unit of analysis. We gradually added the characteristics of patients, hospitals, and communities. Theoretically, the likelihood of ED hospitalizations is affected by hospital policies, the ED's crowdedness, and the community's context (e.g., resources and support systems), which cannot be completely measured in the current study (13, 15, 23, 31). To consider these contextual factors, we conducted sensitivity analyses with clustering patients at the hospital and community levels. A P-value of < 0.05 is considered significant. Statistical analyses were performed using SAS v 9.4 (SAS Institute, Cary, NC).
Results
In the 2011–2015 outpatient files, we identified 253,134 ED visits due to diabetes-related ACSCs. After excluding patients aged under 20 years or non-citizens (N = 40,437), those with missing township/district data (N = 59), and those with missing hospital information for ED visits (N = 221), the total number of ED visits included in the analytical model was 212,417. In the 2011–2015 inpatient files, we identified 597,476 diabetes-related ACSCs admissions. We excluded patients aged under 20 years or non-citizens (N = 5,188), hospitalizations not through ED (N = 238,066), patients with missing township/district data (N = 98), and missing hospital information (N = 69). The total number of ED hospitalizations was 354,055. After merging the qualified sample from the inpatient and outpatient files, the final study sample used in the study was 566,472. Figure 1 presents the selection process of the study sample.
Table 1 presents the descriptive statistics of the study variables between ED hospitalizations and treat-and-leave ED visits. The ED hospitalization rate was ~59.26% (354,055/597,476 in Figure 1). The differences in all the study variables between treat-and-leave ED visits and ED hospitalizations were significant (P < 0.05). The number of hospital beds per 1,000 populations in the treat-and-leave ED group was 0.45, while in the ED admission group, it was 0.59. However, the differences in certain variables (e.g., low income or Aboriginal status) between the two groups were small but significant, which is likely due to the large number of observations in the present study. For example, the ED hospitalization group included 3.96% low-income patients and 19.67% Aboriginal individuals, while the treat-and-leave ED visit group had 3.47% low-income patients and 20.91 % Aboriginal individuals (P < 0.001). Surprisingly, the average Charlson comorbidity index was slightly higher in the treat-and-leave ED group (4.48) than in the ED hospitalization group (4.05). Regional hospitals had ~48% of the treat-and-leave ED visit group and 52% of the ED hospitalization group.
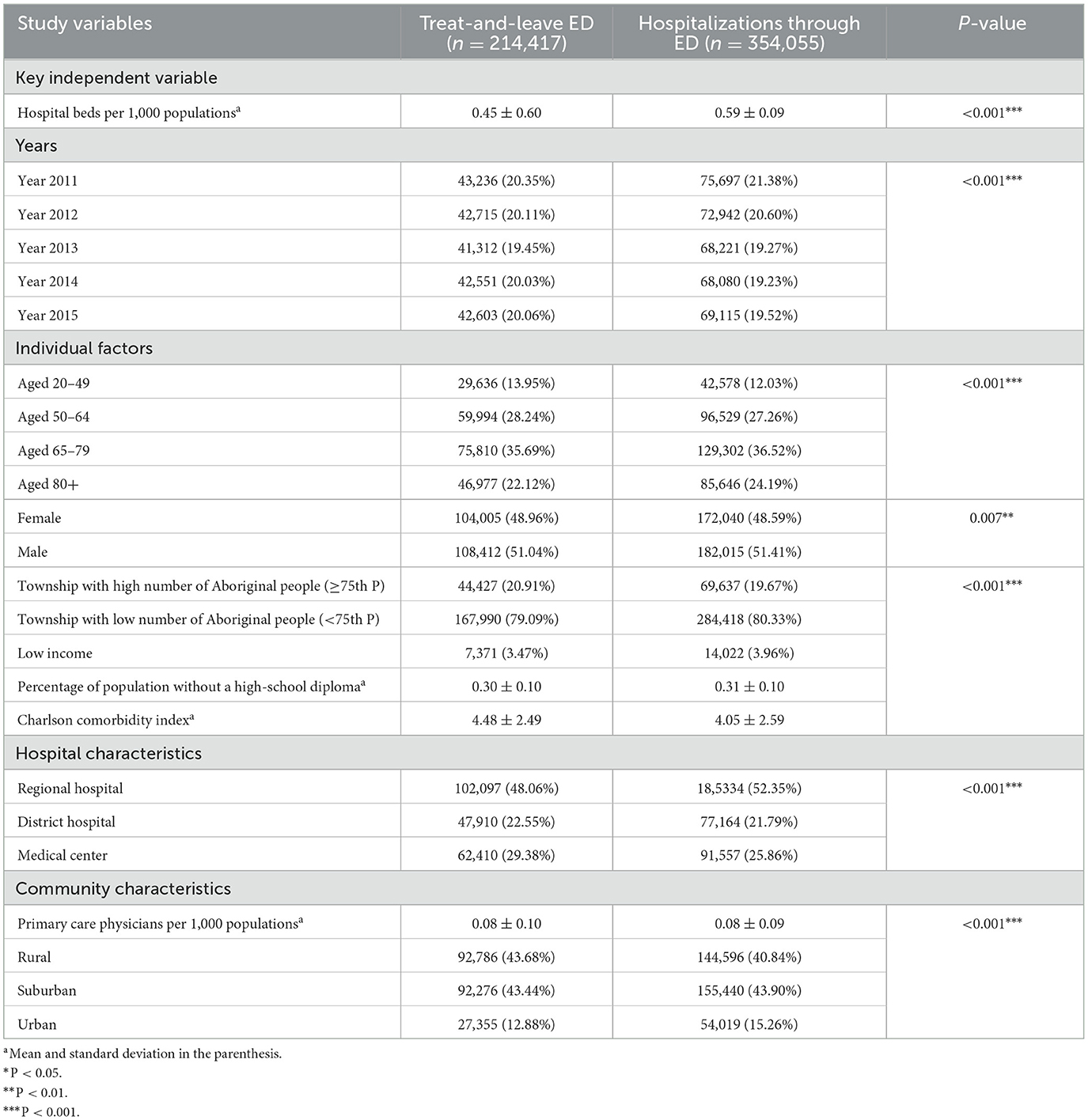
Table 1. Descriptive statistics between treat-and-leave ED visits and hospitalizations through ED due to diabetes-related ACSCs (percentage in the parenthesis).
Table 2 shows the results of four models without clustering. Model 1 includes the number of hospital beds per 1,000 populations and dummy variables for the years 2012–2015 only. Models 2 to 4 are based on Model 1 by gradually adding the characteristics of patients, hospitals, and communities. The odds ratio of the number of hospital beds per 1,000 populations in Model 1 was less than one and significant (OR = 0.94, 95% CI: 0.94–0.95; P < 0.001). However, in Models 2 and 3, the odds ratios were greater than one and insignificant (OR = 1.01 for both models, 95% CI: 0.99–1.02 in Model 2, and 95% CI: 1.00–1.02 in Model 3; P > 0.05). In Model 4 that is the full model, the odds ratio of the number of hospital beds per 1,000 populations was significant (OR = 1.12, 95% CI: 1.09–1.14; P < 0.001), indicating that after controlling for covariates, increases in one hospital bed per 1,000 populations are associated with increases in 12% likelihood of ED hospitalizations. Table 3 shows the results of four models with clustering at the hospital and community levels. The odds ratio of the number of hospital beds per 1,000 populations in Models 1–4 was similar to that in Table 2, except for the one in Model 4, which is statistically insignificant (OR = 1.03, 95% CI: 0.94–1.12; P > 0.05).
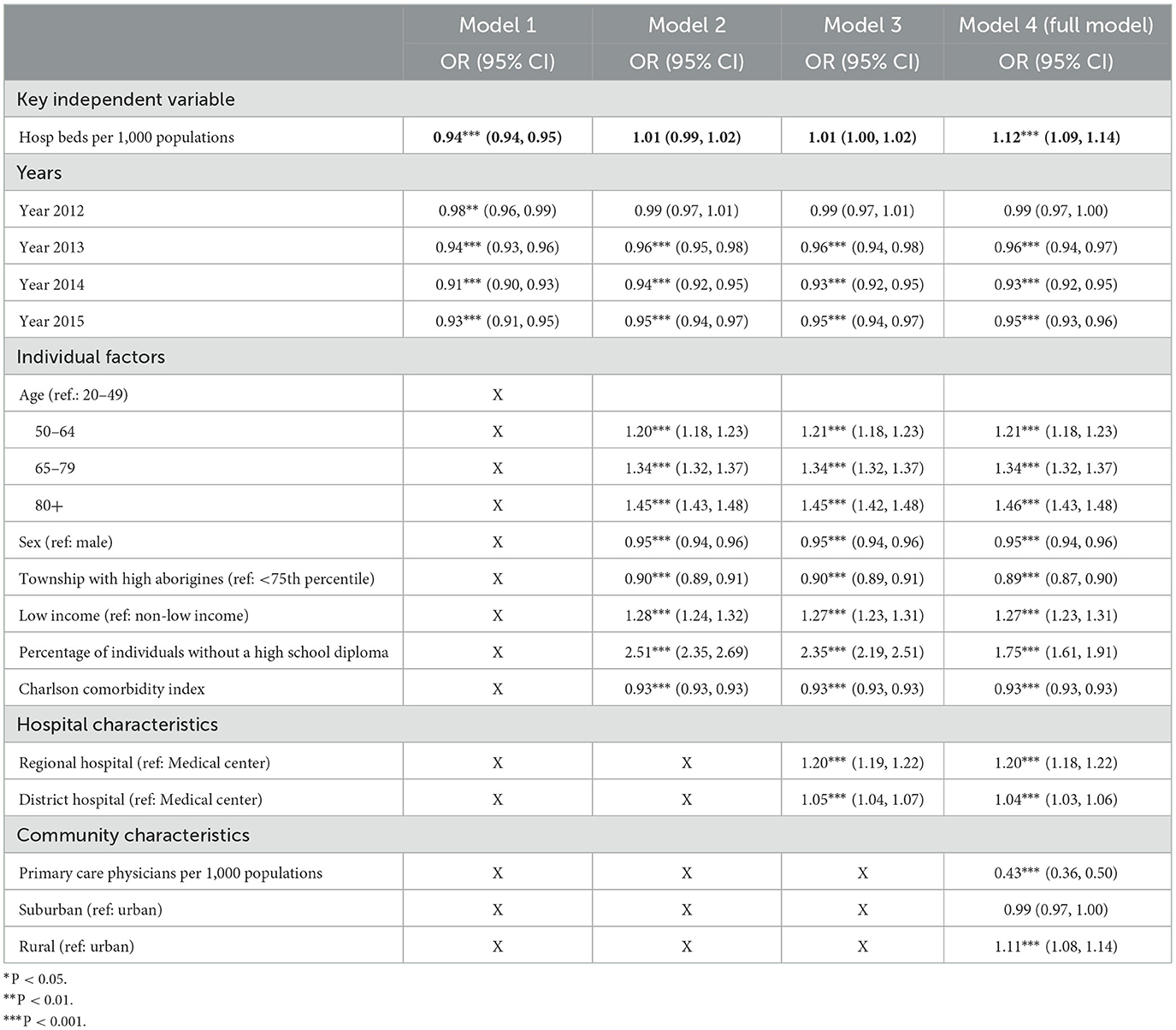
Table 2. Logistic regression for hospital beds and ED admissions due to diabetes-related ACSCs, without clustering.
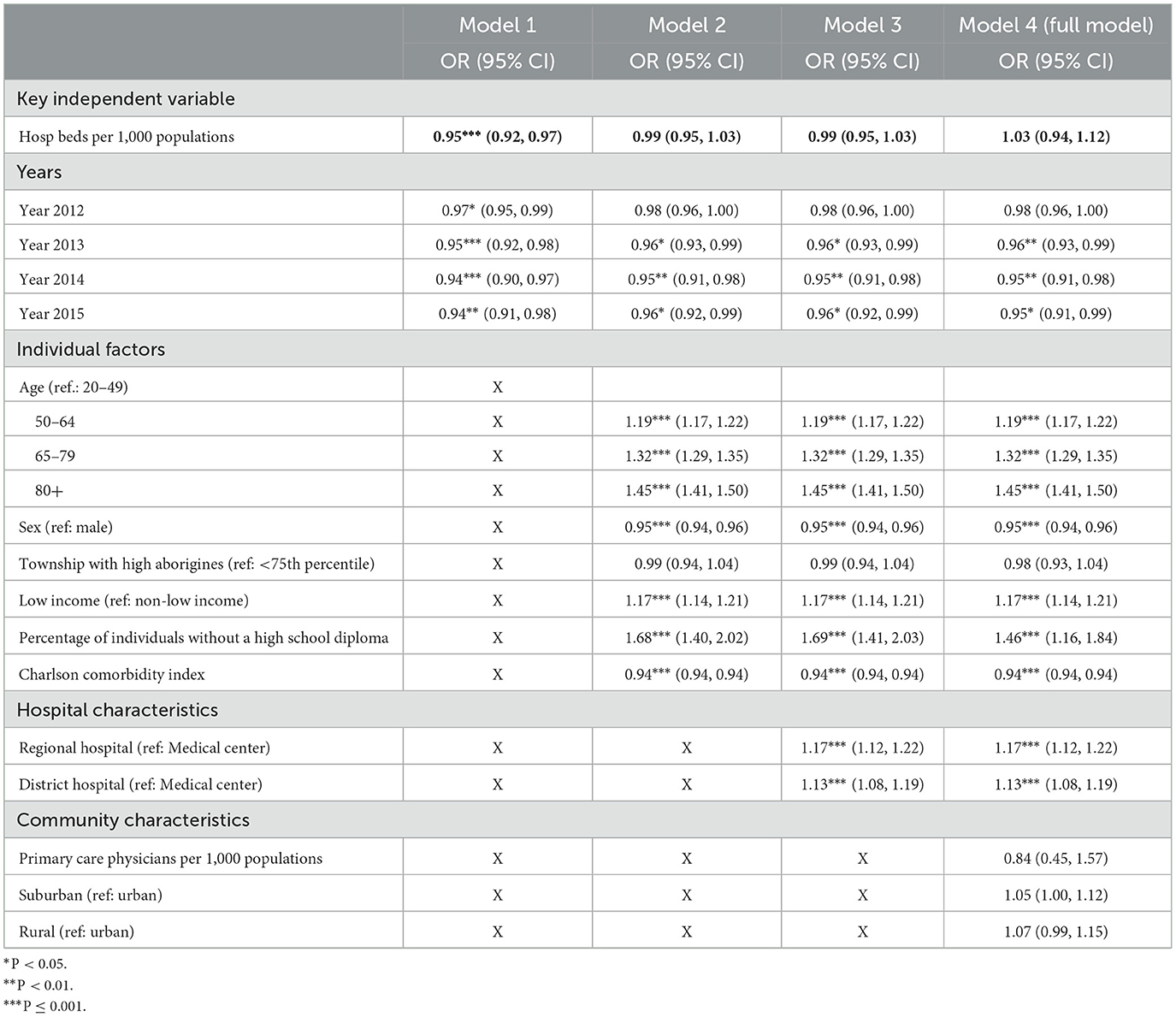
Table 3. Logistic regression for hospital beds and ED admissions due to diabetes-related ACSCs, with clustering patients at the hospital and community level.
The odds ratios among covariates in the full models with and without clustering are similar. The odds ratios for the years 2013–2015 were less than one and significant (OR: 0.93–0.96, 95% CI: 0.91–0.99; P < 0.001), indicating that the likelihood of ED hospitalizations was lower than that in the year 2011. The odds ratios of the three age dummies (50–64, 65–79, and 80+, with < 50 as the reference group) ranged from 1.21 to 1.45 (95% CI: 1.18–1.50; P < 0.001). Female patients were less likely to have ED hospitalizations than male (OR = 0.95, 95% CI: 0.94–0.96; P < 0.001). Patients with low income (OR: 1.17–1.27; 95%CI: 1.14–1.31; P < 0.001) or without a high school diploma (OR: 1.46–1.75; 95%CI: 1.16–1.91; P < 0.001) had a higher likelihood of ED hospitalizations than their counterparts. Regional and district hospitals had a higher likelihood of ED hospitalizations than medical centers (OR: 1.04–1.20, 95% CI: 1.03–1.22; P < 0.001). However, the odds ratio of Aboriginal individuals, the number of primary care physicians per 1,000 populations, and rural areas were significant in the model without clustering but became insignificant in the model with clustering. Unexpectedly, the odds ratio of the Charlson comorbidity index showed that increases in the index were negatively associated with the likelihood of ED admissions (OR = 0.93–0.94; 95%CI: 0.93–0.94; P < 0.001).
Discussion
Summary of the findings
Our study revealed a positive relationship between the supply of hospital beds and the likelihood of ED hospitalizations due to diabetes-related ACSCs; however, the association was statistically significant without a clustering function but was statistically insignificant with a clustering function after controlling for covariates. Among all covariates, the odds ratios in several variables in the models with and without clustering were consistent. Notably, variables with odds ratios higher than one and statistical significance included age groups, low income, a percentage of individuals without a high school diploma, and regional and district hospitals. Conversely, variables with odds ratios of less than one and statistical significance included dummies for study years, sex (female), and the Charlson comorbidity index.
Comparison with other studies
Although some programs in the United States and several countries gradually adopted a global budget to pay providers (32), evidence primarily focuses on the effect of a global budget on service volume or expenditure. In the United States, Massachusetts State adopted the Alternative Quality Contract (AQC), and Maryland State adopted the Maryland Total Cost of Care Model, a global budget with providers keeping the margin and incentivizing for quality of care. Both models reduced utilizations (e.g., laboratory tests and ED hospitalizations and visits) while improving quality of care (e.g., tobacco cessation and the tests of glycated hemoglobin and cholesterol for chronic disease management) (5, 33–39). The Centers for Medicare and Medicaid Services launched different types of accountable care organizations that are responsible for managing a patient population within a capped budget. Findings showed that accountable care organizations reduced inpatient use and ED visits and improved preventive care and chronic disease management (40). Outside of the United States, physicians in Germany increased their service volume after Germany applied a global budget with price adjustments on ambulatory care (4). In Denmark, hospitals funded via a global budget with value-based incentives were able to reduce the length of stay for inpatients (41).
Literature has shown that the number of hospital beds per 1,000 populations has been reduced in several OECD countries, such as Australia, Canada, Denmark, France, and Sweden, regardless of whether the countries implemented a global budget (41, 42). In the United States, the number of hospital beds per 1,000 populations in Maryland State has been reduced after implementing the Total Cost of Care Model (43). Given the recent rising number of hospital beds per 1,000 populations from 4.3 to 4.5 in Taiwan, the positive findings in our study and the increases in service volume and intensity of care found in previous studies in Taiwan (7, 10, 11, 25) indicate that the likelihood of ED hospitalizations would be increased when hospital beds are available.
In addition to the supply of hospital beds, social determinants, including income and education at the patient level and hospital characteristics at the hospital level, are positively associated with the likelihood of ED hospitalizations. On average, the ED hospitalizations rate in the current study was 59% (597,476/566,472 in Figure 1). Identifying high-risk patients and conducting interdisciplinary collaboration to meet their social and health needs would reduce ED visits and hospitalizations while keeping patients safe at home.
Limitations
The present study has limitations. First, the study used data from 2011 to 2015. The majority of the expanded hospital beds are still under reconstruction. Our findings cannot completely reflect the association when all new hospital beds are available in the market. Furthermore, the NHIA has launched several interventions, such as increasing the differences in co-payment and encouraging mutual referrals among medical centers, district hospitals, and local hospitals to reduce the burden on medical centers and strengthen local hospitals and clinics in the communities. Utilizing the most recent data to investigate the relationship between hospital beds and ED hospitalizations is encouraged. Second, the AHRQ PQI program excludes patients transferred from other institutions or receiving specific procedures. Our data did not have the codes for procedures and transfer from a hospital or other institutions; therefore, the number of diabetes-related ACSCs was likely be overestimated. However, the limitations of missing these variables in the data are unlikely to affect our findings since we applied the same rules to extract diabetes-related ACSCs from inpatient and outpatient files. Third, we assume that hospitals are engaging in the game of prisoner's dilemma. However, hospitals may adjust their ED hospitalizations policy to maximize their margin based on their historical reimbursement data, which cannot be detected by the current study, although the chance is low given the existing evidence from Taiwan (9–11, 25). Finally, the present study focused on diabetes-related ACSCs. Future studies examining other discretionary conditions (e.g., heart failure or asthma) and non-discretionary conditions (e.g., fracture or heart attack) are also recommended.
Policy implications
Despite the limitations, our findings have policy implications. First, the fast-expanding number of hospital beds in recent years may motivate hospitals to admit ED patients with preventable and discretionary conditions under a global budget with a floating-value payment system. Therefore, the call to increase the floating-point value to one NTD would eventually challenge the financial sustainability of the NHI without adding value to patient care. Given the evidence from other countries, adding value-based incentive mechanisms to the current global budget system is strongly recommended. Second, although 99% of the population in Taiwan is enrolled in the NHI, patients with low income and without high school education are at risk of ED hospitalizations due to diabetes-related ACSCs. Policies that effectively address the risks of social determinants contributing to poor health outcomes are strongly recommended, which would have the potential to significantly improve population health while reducing the financial burden on the NHIA.
Conclusion
An increase in the number of hospital beds is likely to motivate providers to admit ED patients with preventable and discretionary conditions under hospital global budgeting with a floating-point value mechanism, which would lead to high hospitalization rates without adding value to patient care. Adding value-based incentive mechanisms to the current global budget payment and addressing social risk factors contributing to poor health outcomes would improve population health and reduce preventable hospitalizations while improving the financial sustainability of the NHI.
Data availability statement
The datasets presented in this article are not readily available because the datasets used and analyzed in the present study are not publicly available but are available from the Taiwan National Health Insurance Program in Taiwan. Restrictions apply to the availability of these data, which were used under license for the present study. Requests to access the datasets should be directed at: https://nhird.nhri.org.tw with the permission of the Taiwan National Health Insurance (NHI) program.
Ethics statement
The studies involving humans were approved by the Institutional Review Board at Kaohsiung Medical University Hospital in Taiwan (KMUHIRB- SV(I)-20210063). The studies were conducted in accordance with the local legislation and institutional requirements. Written informed consent for participation was not required from the participants or the participants' legal guardians/next of kin in accordance with the national legislation and institutional requirements.
Author contributions
HFC: Conceptualization, Data curation, Formal analysis, Funding acquisition, Investigation, Methodology, Project administration, Resources, Software, Supervision, Validation, Visualization, Writing – original draft, Writing – review & editing. HMH: Conceptualization, Data curation, Formal analysis, Investigation, Methodology, Supervision, Validation, Writing – review & editing. WSC: Data curation, Formal analysis, Investigation, Software, Validation, Visualization, Writing – review & editing.
Funding
The author(s) declare financial support was received for the research, authorship, and/or publication of this article. The present study was supported by the Taiwan National Science and Technology Council (MOST110-2410-H-037-018-MY3 and NSTC 113-2410-H-037-003-MY2). None of the funding organizations had any role in study design, data collection, analysis, interpretation of data, the decision to publish, or manuscript preparation.
Acknowledgments
The authors thank the assistance from the Division of Medical Statistics, Department of Medical Research, Kaohsiung Medical University Hospital, Kaohsiung Medical University.
Conflict of interest
The authors declare that the research was conducted in the absence of any commercial or financial relationships that could be construed as a potential conflict of interest.
Publisher's note
All claims expressed in this article are solely those of the authors and do not necessarily represent those of their affiliated organizations, or those of the publisher, the editors and the reviewers. Any product that may be evaluated in this article, or claim that may be made by its manufacturer, is not guaranteed or endorsed by the publisher.
References
1. Canadian Institute for Health Information. National Health Expenditure Trends, 2022 — Snapshot. Canadian Institute for Health Information (2022). Available at: https://www.cihi.ca/en/national-health-expenditure-trends-2022-snapshot (accessed June 14, 2024).
2. Centers for Disease Control and Prevention. Health Expenditrure. National Center for Health Statistics (2023). Available at: https://www.cdc.gov/nchs/fastats/health-expenditures.htm (accessed June 14, 2024).
3. Braendle T, Colombier C. Budgetary targets as cost-containment measure in the Swiss healthcare system? Lessons from abroad. Health Policy. (2020) 124:605–14. doi: 10.1016/j.healthpol.2020.05.007
4. Benstetter F, Wambach A. The treadmill effect in a fixed budget system. J Health Econ. (2006) 25:146–69. doi: 10.1016/j.jhealeco.2005.04.004
5. Maryland Health Services Cost Review Commission. Global Budget Revenue Adjustments. Maryland State Government (2024). Available at: https://hscrc.maryland.gov/Pages/gbr-adjustments.aspx (accessed June 4, 2024).
6. Gaspar K, Portrait F, van der Hijden E, Koolman X. Global budget versus cost ceiling: a natural experiment in hospital payment reform in the Netherlands. Eur J Health Econ. (2020) 21:105–14. doi: 10.1007/s10198-019-01114-6
7. Chen B, Fan VY. Global budget payment: proposing the CAP framework. Inquiry. (2016) 53:0046958016669016. doi: 10.1177/0046958016669016
8. Ministry of Health and Welfare Taiwan. 112 Global Budget and Its Allocation (in Chinese). Ministry of Health and Welfare, Taiwan (2023). Available at: https://dep.mohw.gov.tw/NHIC/cp-1661-73150-116.html (accessed June 4, 2024).
9. Chen FJ, Laditka JN, Laditka SB, Xirasagar S. Providers' responses to global budgeting in Taiwan: what were the initial effects? Health Serv Manage Res. (2007) 20:113–20. doi: 10.1258/095148407780744624
10. Chen B, Fan VY. Strategic provider behavior under global budget payment with price adjustment in Taiwan. Health Econ. (2015) 24:1422–36. doi: 10.1002/hec.3095
11. Cheng SH, Chen CC, Chang WL. Hospital response to a global budget program under universal health insurance in Taiwan. Health Policy. (2009) 92:158–64. doi: 10.1016/j.healthpol.2009.03.008
12. O'ECDiLibrary. Health at a Glance 2023: Hospital Bed and Occupation. O'ECDiLibrary (2024). Available at: https://www.oecd-ilibrary.org/sites/bdd23022-en/index.html?itemId=/content/component/bdd23022-en (accessed June 8, 2024).
13. van den Broek S, Heiwegen N, Verhofstad M, Akkermans R, van Westerop L, Schoon Y, et al. Preventable emergency admissions of older adults: an observational mixed-method study of rates, associative factors and underlying causes in two Dutch hospitals. BMJ Open. (2020) 10:e040431. doi: 10.1136/bmjopen-2020-040431
14. Blom MC, Jonsson F, Landin-Olsson M, Ivarsson K. The probability of patients being admitted from the emergency department is negatively correlated to in-hospital bed occupancy - a registry study. Int J Emerg Med. (2014) 7:8. doi: 10.1186/1865-1380-7-8
15. Fusco M, Buja A, Piergentili P, Golfetto MT, Serafin G, Gallo S, et al. Individual and hospital-related determinants of potentially inappropriate admissions emerging from administrative records. Health Policy. (2016) 120:1304–12. doi: 10.1016/j.healthpol.2016.09.015
16. Fisher ES, Wennberg JE, Stukel TA, Sharp SM. Hospital readmission rates for cohorts of medicare beneficiaries in Boston and New Haven. N Engl J Med. (1994) 331:989–95. doi: 10.1056/NEJM199410133311506
17. Fisher ES, Wennberg JE. Health care quality, geographic variations, and the challenge of supply-sensitive care. Perspect Biol Med. (2003) 46:69–79. doi: 10.1353/pbm.2003.0004
18. Fisher ES, Wennberg DE, Stukel TA, Gottlieb DJ, Lucas FL, Pinder EL. The implications of regional variations in Medicare spending. Part 1: the content, quality, and accessibility of care. Ann Intern Med. (2003) 138:273–87. doi: 10.7326/0003-4819-138-4-200302180-00006
19. Wennberg J. Tracking Medicine: A Researcher's Quest to Understand Health Care. Oxford: Oxford University Press (2010).
20. Kim AM, Park JH, Yoon TH, Kim Y. Hospitalizations for ambulatory care sensitive conditions as an indicator of access to primary care and excess of bed supply. BMC Health Serv Res. (2019) 19:259. doi: 10.1186/s12913-019-4098-x
21. O'Cathain A, Knowles E, Maheswaran R, Turner J, Hirst E, Goodacre S, et al. Hospital characteristics affecting potentially avoidable emergency admissions: national ecological study. Health Serv Manage Res. (2013) 26:110–8. doi: 10.1177/0951484814525357
22. Falster MO, Jorm LR, Douglas KA, Blyth FM, Elliott RF, Leyland AH. Sociodemographic and health characteristics, rather than primary care supply, are major drivers of geographic variation in preventable hospitalizations in Australia. Med Care. (2015) 53:436. doi: 10.1097/MLR.0000000000000342
23. Shoff C, Caines K. Pines JM. Geographic variation in predictors of ED admission rates in US Medicare fee-for-service beneficiaries. Am J Emerg Med. (2019) 37:1078–84. doi: 10.1016/j.ajem.2018.08.060
24. Milstein R, Schreyogg J. The end of an era? Activity-based funding based on diagnosis-related groups: a review of payment reforms in the inpatient sector in 10 high-income countries. Health Policy. (2024) 141:104990. doi: 10.1016/j.healthpol.2023.104990
25. Kan K, Li SF, Tsai WD. The impact of global budgeting on treatment intensity and outcomes. Int J Health Care Finance Econ. (2014) 14:311–37. doi: 10.1007/s10754-014-9150-0
26. Satokangas M, Arffman M, Antikainen H, Leyland AH, Keskimaki I. Individual and area-level factors contributing to the geographic variation in ambulatory care sensitive conditions in Finland: a register-based study. Med Care. (2021) 59:123–30. doi: 10.1097/MLR.0000000000001454
27. Sentell TL, Seto TB, Young MM, Vawer M, Quensell ML, Braun KL, et al. Pathways to potentially preventable hospitalizations for diabetes and heart failure: a qualitative analysis of patient perspectives. BMC Health Serv Res. (2016) 16:300. doi: 10.1186/s12913-016-1511-6
28. Schumacher JR, Hall AG, Davis TC, Arnold CL, Bennett RD, Wolf MS, et al. Potentially preventable use of emergency services: the role of low health literacy. Med Care. (2013) 51:654–8. doi: 10.1097/MLR.0b013e3182992c5a
29. Quan H, Sundararajan V, Halfon P, Fong A, Burnand B, Luthi JC, et al. Coding algorithms for defining comorbidities in ICD-9-CM and ICD-10 administrative data. Med Care. (2005) 43:1130–9. doi: 10.1097/01.mlr.0000182534.19832.83
30. Liu CY, Hung Y-T, Chuang YL, Chen YJ, Weng WS, Liu JS, et al. Incorporating development stratification of Taiwan townships into sampling design of large scale health interview survey. J Health Manag. (2006) 4:1–22.
31. Pope I, Burn H, Ismail SA, Harris T, McCoy D. A qualitative study exploring the factors influencing admission to hospital from the emergency department. BMJ Open. (2017) 7:e011543. doi: 10.1136/bmjopen-2016-011543
32. The Organisation for Economic Cooperation and Development (OECD). Better ways to pay for health care. (2016). Available at: https://www.oecd-ilibrary.org/docserver/9789264258211-5-en.pdf?expires=1729944654&id=id&accname=guest&checksum=959CB891C41417CB44CF2E4F22527987 (accessed October 15, 2024).
33. Song Z, Rose S, Safran DG, Landon BE, Day MP, Chernew ME. Changes in health care spending and quality 4 years into global payment. N Engl J Med. (2014) 371:1704–14. doi: 10.1056/NEJMsa1404026
34. Song Z, Ji Y, Safran DG, Chernew ME. Health care spending, utilization, and quality 8 years into global payment. N Engl J Med. (2019) 381:252–63. doi: 10.1056/NEJMsa1813621
35. Huskamp HA, Greenfield SF, Stuart EA, Donohue JM, Duckworth K, Kouri EM, et al. Effects of global payment and accountable care on tobacco cessation service use: an observational study. J Gen Intern Med. (2016) 31:1134–40. doi: 10.1007/s11606-016-3718-y
36. Shammas RL, Coroneos CJ, Ortiz-Babilonia C, Graton M, Jain A, Offodile AC 2nd. Implementation of the Maryland Global Budget Revenue Model and variation in the expenditures and outcomes of surgical care: a systematic review and meta-analysis. Ann Surg. (2023) 277:542–8. doi: 10.1097/SLA.0000000000005744
37. Galarraga JE, DeLia D, Huang J, Woodcock C, Fairbanks RJ, Pines JM. Effects of Maryland's global budget revenue model on emergency department utilization and revisits. Acad Emerg Med. (2022) 29:83–94. doi: 10.1111/acem.14351
38. Xie L, Boudreaux M, Franzini L. Maryland's global budget revenue program: impact on neonatal intensive care unit admissions and infant mortality. Med Care. (2021) 59:663–70. doi: 10.1097/MLR.0000000000001534
39. Sharfstein JM, Stuart EA, Antos J. Global budgets in Maryland: assessing results to date. JAMA. (2018) 319:2475–6. doi: 10.1001/jama.2018.5871
40. Kaufman BG, Spivack BS, Stearns SC, Song PH, O'Brien EC. Impact of accountable care organizations on utilization, care, and outcomes: a systematic review. Med Care Res Rev. (2019) 76:255–90. doi: 10.1177/1077558717745916
41. Birk HO, Vrangbaek K, Rudkjobing A, Krasnik A, Eriksen A, Richardson E, et al. Denmark: health system review. Health Syst Transit. (2024) 26:1–186. Available at: https://iris.who.int/bitstream/handle/10665/376116/9789289059558-eng.pdf?sequence=1 (accessed November 29, 2024).
42. Janlov N, Blume S, Glenngard AH, Hanspers K, Anell A, Merkur S. Sweden: health system review. Health Syst Transit. (2023) 25:1–236. Available at: https://iris.who.int/bitstream/handle/10665/372708/9789289059473-eng.pdf?sequence=8 (Accessed November 29, 2024).
43. Kaiser Family Foundation. Total Hospital Beds. Kaiser Family Foundation (2024). Available at: https://www.kff.org/other/state-indicator/total-hospital-beds/?activeTab=graph¤tTimeframe=0&startTimeframe=7&selectedDistributions=beds-per-1000-population&selectedRows=%7B%22states%22:%7B%22arizona%22:%7B%7D,%22maryland%22:%7B%7D%7D%7D&sortModel=%7B%22colId%22:%22Location%22,%22sort%22:%22asc%22%7D (accessed June 15, 2024).
Keywords: ambulatory care sensitive conditions, treat-and-leave emergency department visits, global budget, preventable hospitalizations, diabetes-related complications, floating-point value
Citation: Chen HF, Hsieh HM and Chang WS (2025) Preventable hospitalizations through ED: does the number of hospital beds matter under the global budget in a single-payer system in Taiwan? Front. Public Health 12:1460270. doi: 10.3389/fpubh.2024.1460270
Received: 05 July 2024; Accepted: 11 November 2024;
Published: 06 January 2025.
Edited by:
Thomas T. H. Wan, University of Central Florida, United StatesReviewed by:
Sara Haddadi, University of Miami Health System, United StatesMadhu Shettian, PALS Global LLC, United States
Copyright © 2025 Chen, Hsieh and Chang. This is an open-access article distributed under the terms of the Creative Commons Attribution License (CC BY). The use, distribution or reproduction in other forums is permitted, provided the original author(s) and the copyright owner(s) are credited and that the original publication in this journal is cited, in accordance with accepted academic practice. No use, distribution or reproduction is permitted which does not comply with these terms.
*Correspondence: Hsueh-Fen Chen, Y2hlbmhmQGttdS5lZHUudHc=