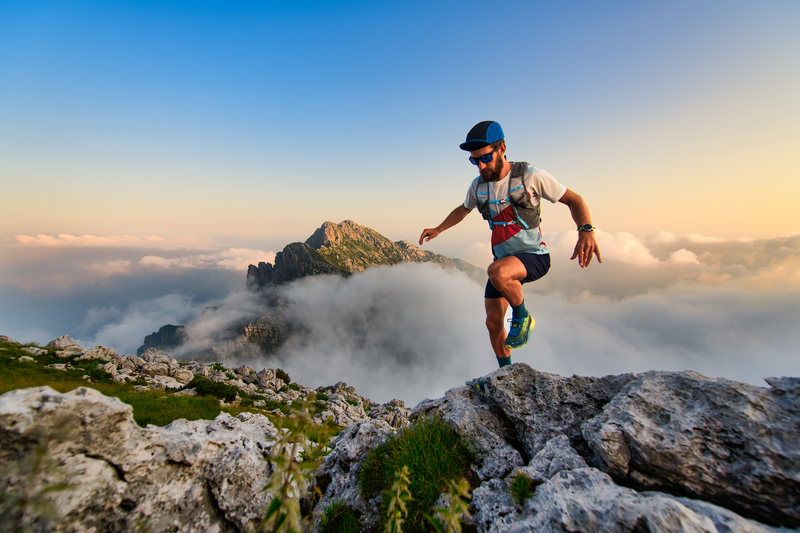
95% of researchers rate our articles as excellent or good
Learn more about the work of our research integrity team to safeguard the quality of each article we publish.
Find out more
ORIGINAL RESEARCH article
Front. Public Health , 17 October 2024
Sec. Occupational Health and Safety
Volume 12 - 2024 | https://doi.org/10.3389/fpubh.2024.1459809
This article is part of the Research Topic Physical Activity Behavior, Obesity, and Stress as Crucial Sources of Health Issues in Stressful Occupations, Volume II View all 3 articles
This study sought to analyze the influence of occupational stress on the body composition of hospital workers after one year of follow-up. This prospective cohort study included 218 workers from one of the leading private hospitals in the municipality of Santo Antônio de Jesus, Recôncavo da Bahia region, Northeast Brazil. Body composition was analyzed by proxy (Body Mass Index and Waist Circumference) and Bioelectrical Impedance Analysis. The primary exposure adopted in the present study was the perception of occupational stress, assessed with the adapted and reduced version of the Job Content Questionnaire evaluating demand and control dimensions. The covariates were work characteristics; biological characteristics; sociodemographic characteristics and lifestyle. Statistical analyses were performed using descriptive, bivariate and multivariate analysis. At the first stage of the study, we identified that 55.96% (n = 122) of workers had high work demand and 25.22% (n = 55) had low control. Among those who had high demand and low control at the beginning of the study, the majority were women, non-white, with low educational and income levels, sleeping less than 7 h/day. After 12 months of follow-up, the median value for demand continued as 13 (IQR: 5–25) and for control, it increased to 16 (IQR: 9–23). In this second moment of the study, 62.38% (n = 136) of workers showed high demand and 45.87% (n = 100) low control. The characteristics of workers with high demand and low control were similar to those of the first moment. The results indicate that high demand and low control at work are risk factors for changes in body mass index, fat mass and fat-free mass in hospital workers. This study shows the importance and need for clinical and epidemiological assessments regarding the body composition of professionals working in hospitals, since high rates of overweight and obesity are triggers of chronic health problems such as dyslipidemia, diabetes mellitus and cardiovascular diseases, among others. Therefore, managers must promote adequate working conditions and understand the need for periodic body composition assessments.
Occupational stress is present when stressful factors are related to the work environment and workers do not have the capacity or resources to adapt to such factors (1). Stress is considered a public health problem affecting different individuals and is present in several organizations, including hospitals (1–3). Evidence point to a high prevalence of occupational stress among hospital workers, ranging from 23.8 to 87% (4–7), reinforcing the need for studies and interventions in this work environment.
Not neglecting occupational stress and talking about it is essential and urgent, especially given the precariousness of work environments (8, 9). Understanding that adequate working conditions constitute a human right and, given millions of people worldwide suffering from the consequences of precarious work, an attentive and dedicated look from managers and an approach that reduces occupational stress are priorities, as they contribute to physical and mental health.
Several theoretical models regarding occupational stress are observed in the literature, with the Demand-Control Model being a reference model and one of the most used in the world. The work psychosocial dimension in this model is Job Demand, which refers to the psychological stressors involved in carrying out work, such as unexpected tasks, the volume of work, time pressures, level of attention and concentration required, etc. (10–12). The Control dimension concerns to the control that the worker has when carrying out his tasks and the conduct adopted during the working day, such as autonomy, use and development of skills in carrying out tasks, initiative, and influence on organizational policy, among others (10–12). In this sense, it is the capacity to face work demands, acting as a protective factor against stress (13). Thus, in the presence of high psychological demand with low control over work, a work situation can harm the individual’s health (10, 14).
Occupational stress affects workers’ health differently, given the diversity of work environments (1, 5). In this scenario, hospital workers have experiences that impact their health in different ways, including changes in their body composition (15, 16). Body composition encompasses the assessment of body parameters divided into fat-free mass, adipose tissue, body cell mass, total body water and extracellular water (17–19).
Adequate body composition is essential for an individual’s health because excess adipose tissue favors metabolic disorders and, consequently, contributes to the emergence and/or worsening of chronic diseases—cardiovascular diseases, diabetes mellitus, high blood pressure, etc. Furthermore, assessing body composition is important to obtain information about the nutritional status of individuals, and the functional capacity of the human body, in addition to offering information necessary for intervention measures, among others (19–21).
Occupational stress impacts lifestyle levels, represented by low frequency or absence of physical activity, alcohol and tobacco consumption, failure to prepare one’s own meals (22, 23), in addition to exposure to high food consumption, whether in volume and/or caloric density (24, 25). Thus, workers immersed in a stressful work environment are at risk of developing overweight and obesity. Therefore, body composition is a significant aspect of the study of stress because overweight workers have a higher risk of occupational injuries (26), reduced work capacity concerning the physical demands of the job (27, 28), decreased productivity or increased absence due to illness (16, 29). Still, it is especially necessary to evaluate development among hospital workers, as they are immersed in environments with precarious working conditions such as long working hours, many hours standing, shift work, among others.
There is a gap in the scientific literature that concerns the health of hospital workers when addressing the body composition of this group, as they basically use indicators such as the Body Mass Index (BMI), Waist Circumference (WC) and, to a lesser extent, the percentage of body fat, assessed through the sum of skinfolds. However, the use of anthropometry for such an assessment is limiting because it is a method in which accuracy and precision depend on experienced and trained evaluators, the use of appropriate and properly calibrated equipment, in addition to the regression models used in the formulas specific to each population, which makes it necessary to adopt specific and more complex parameters, among other factors (19, 21, 30). On the other hand, the gold standard methods for assessing body composition are magnetic resonance imaging and computed tomography, which are expensive and unavailable in most occupational medicine services, especially in continental and developing countries, such as Brazil. A good alternative is the use of Bioelectrical Impedance Analysis (BIA), which has a high correlation with anthropometric methods and techniques considered the gold standard, in addition to having a relatively low cost (19, 31). Given this context, this study sought to analyze the influence of occupational stress on hospital workers’ body composition (using BIA, BMI and WC) after one year of follow-up.
The Ethics and Research Committee of the School of Nutrition at the Federal University of Bahia approved this study (CAAE approval number 4,316,252). The procedures followed the guidelines established by the Declaration of Helsinki and all workers who agreed to participate in the study signed an informed consent form.
This prospective cohort study included 218 workers from one of the leading private hospitals in the municipality of Santo Antônio de Jesus, Recôncavo da Bahia region, Northeast Brazil, with secondary and tertiary levels of care (32, 33). This municipality has an estimated population of 103.055 thousand inhabitants (34).
Sampling was non-probability and was carried out for convenience by inviting all the hospital workers. Of the 302 workers who began the study, 218 completed both interviews, totaling a definitive loss of 27.81% (n = 84). During follow-up, the loss occurred due to dismissal (n = 66), sick leave (n = 8), pregnancy (n = 4), vacation (n = 4) and withdrawal from participating in the study (n = 2). Data from the sample was used to calculate the sampling power, considering that specific information about the variability of exposure and outcome in a similar population was unknown and/or was not identified. Using sample data, we sought to estimate parameters necessary for calculating power, allowing a more adaptive and realistic approach given the initial uncertainty (35). Thus, this sample size (218 individuals) has power ranging from 91.9 to 99.9% to detect an unbiased association between occupational stress (in its different domains) and body composition (BMI, WC, FFM and CG) after a year of follow-up in the study population. The sample power calculations (1-∂) were based on the 5% significance level and two-tailed tests, indicating that this sample size is sufficient to make unbiased estimates of the parameters of the population under study (36).
The inclusion criteria adopted were being of legal age (≥ 18 years in Brazil), carrying out work activities (regardless of the job position), the possibility of carrying out anthropometric measurements and being able to carry out bioelectrical impedance analysis, in addition to women who are not pregnant or in the postpartum period. In turn, the exclusion criteria were no longer being a worker at the hospital under study, becoming pregnant, expressing the desire to leave the research, and being away from the work environment for reasons such as vacation or sick leave (37).
All measurements were collected at two follow-up moments: between May and October 2019, to form the study baseline; and between October and December 2020, for follow-up with the same workers, instruments, techniques and interviewers.
Body composition was analyzed in two ways: the use of proxy and Bioelectrical Impedance Analysis (BIA). The proxies used were Body Mass Index (BMI) and Waist Circumference (WC). Anthropometric weight and height measurements were taken to calculate BMI later. A portable digital scale with bioimpedance on a platform was used to measure weight, where the workers were wearing light clothing, were barefoot, and were without adornments or any object that could interfere with the measurement. Height was measured with the worker in an upright posture, feet together and heels against the wall, using a portable stadiometer. The BMI was calculated using measurements of weight and height (weight–kg/height m2) (38). For inclusion in the model, the variable was used in a continuous and time-varying manner.
The WC was measured with a flexible and inelastic measuring tape. The men were asked to remove their blouses and the women to lift them at the waist. The workers stood upright, with their abdomen relaxed, arms extended and weight equally distributed between their legs, with their feet close and parallel. The measurement was taken at the end of expiration, at the midpoint marked between the last rib and the iliac crest, which was located and marked previously (39). For inclusion in the model, the variable was used in a continuous and time-varying manner.
Body composition was assessed using a tetrapolar bioelectrical impedance device (Biodynamics®), according to the protocol described by Kyle et al. (40): fasting for 4 h and no alcohol consumption in the 48 h before the exam; empty bladder before measurement; no physical exercise in the last 48 h; skin at room temperature and without visible lesions; the same side of the body was measured and with a minimum distance of 5 cm between the electrodes; the electrodes were cleaned with alcohol; arms separated from the trunk by around 30° and legs separated by around 45°; supine position for 5 to 10 min. There was no contact with the metal structure of the stretcher and in a neutral environment (without strong electric or magnetic fields). From the information provided by the BIA, we used Fat Free Mass (FFM) and Body Fat (BF) data. For inclusion in the model, both variables were used in a continuous and time-varying manner.
The primary exposure adopted in the present study was the perception of occupational stress, assessed with the adapted and reduced version of the Job Content Questionnaire (JCQ), validated for the Brazilian population by Alves et al. (10) (Supplementary Table S1).
The JCQ was an instrument created by Robert Karasek to assess social relationships in the workplace that generated stress and impacted health outcomes. Originally, the instrument contained 49 questions distributed between the “Demand” and “Control” dimensions (10). Years later, in Sweden, Töres Theorell adapted the original instrument by reducing it to 11 questions and calling it the Job Stress Scale (JSS) distributed as follows: five questions to assess Demand and six questions to assess Control (10).
At the National School of Public Health in Rio de Janeiro—Brazil, Alves et al. (10), after obtaining the rights, adapted the scale once reduced by Töres Theorell in Sweden into Brazilian Portuguese. The adaptation has response options presented on a Likert scale (1–4 items), varying between “often” and “never/almost never” for the Demand and Control dimensions. With the adaptation process, Brazilian researchers obtained two reliability components of the scale, which were the intraclass correlation coefficients for the Demand (0.88) and Control (0.87) dimensions and the Cronbach’s alpha of 0.79 (Demand) and 0.67 (Control) also for both dimensions (10).
Workers were asked about both dimensions—Demand and Control—at both data collection times for this research. Given that the concepts of these dimensions are theoretically distinct (41, 42) and to achieve the objective of this study, we used information from the two dimensions separately (10, 43).
For its categorization, the first step was to construct the “Demand” and “Control” dimensions, which was done by adding up the questions present in the JSS. Then, to classify the worker as high demand (risk category) and low demand (reference category), and high control (reference category) and low control (risk category), we sum the scores above or below the value from the median (44).
The research form was prepared for the present study and completed by previously trained interviewers. Thus, the variables were grouped into: (a) work characteristics (job position: administrative and health professionals; working time in the position in full years: <1 year to 3 years, 4 to 5 years and ≥ 6 years; working hours weekly working hours: ≤44 h and ≥ 45 h (45); other employment: yes and no; work shift: shift, day and night; type of contract: permanent/contracted and others); (b) biological characteristics (biological sex: man and woman; reported race/color: white and non-white; age in years: 18 to 30 years, 31 to 43 years and 44 to 61 years); (c) sociodemographic characteristics (education: elementary/high school and higher education/specialization; family income in minimum wages: 1 to <3 and ≥ 3; number of dependents: 1 to 4 and ≥ 5); (d) lifestyle (smoking: yes and no; alcohol consumption: yes and no; hours of sleep: <7 h and ≥ 7 h; number of meals per day: 1 to 5 and ≥ 6; physical activity: low, moderate and high).
The physical activity variable was assessed with the reduced version of the International Physical Activity Questionnaire, validated for the Brazilian population (46). The workers were asked about the levels of activities (physical exercise, domestic, leisure and commuting activities) carried out seven days before data collection in the two study periods and considering the frequency and duration criteria. Finally, for this study, workers were classified as having low, moderate and high levels of physical activity, if they had Metabolic Equivalents (MET) <600/min/week, 600 to 3,000 MET/min/week and ≥ 3,000 MET/week minutes/week, respectively (47).
Statistical analyses were carried out in three main steps: (i) Descriptive analysis of sample characteristics: categorical variables were summarized by absolute (n) and relative (%) frequencies. Prevalences were estimated by demand and control categories (high or low) in each period. Quantitative variables were summarized using mean and standard deviation (SD), after applying the Shapiro–Wilk test to assess normality. The mean values of weight, BMI, WC, FFM and BF according to high or low demand and control in each period were compared using the Student’s t-test for equal or unequal variances. In the analyses, a significance of 5% was adopted (48).
(ii) Bivariate analysis (assessment of the association of outcomes with the main exposure and covariates): comparing outcome variables and covariates of interest according to the exposure variable was performed to select candidate variables for the multivariate model. Those associated with exposure and outcome, expressed by a change <20% in the p-value compared to that measured in the raw model, were considered potential confounders and included in the multivariate models using the Linear Mixed Effects Model analysis (48, 49). In addition to statistical criteria, the theoretical framework was considered when choosing the final model.
(iii) Multivariate analysis: to evaluate the influence of occupational stress (variant over time and used in a categorized way: demand and control) on the variation in BMI, WC, FFM and BF over time, we constructed Linear Mixed Effects Models (LMEM). Such analysis is pertinent when measurements repeated over time are obtained from the same individual, as it considers the correlation between measurements at each moment in time, as well as the variability between and within individuals over time, considering the temporal variable in the relationship between exposure and outcome (50, 51). To evaluate the desired relationship, a model was built for each outcome (anthropometric data included in its continuous and longitudinal form) as a function of the primary exposure variable (demand and control, included simultaneously in the models). The variables selected in step ii were included in the model as adjustment variables. For the present study, the correlation matrix chosen was auto-regressive, given that time had an equal space to obtain the measurements and that the data were not unbalanced (52).
The model’s adjusted data were evaluated based on the Akaike Information Criterion (AIC), where the lower the value, the better the model fit (53) and also by the likelihood ratio test. All analyses were performed using STATA® for MAC Version 17.0 statistical software (StataCorp, College Station).
At baseline, the median value for demand was 13 (IQR: 5–20) and for control, 15 (IQR: 8–24). At the first stage of the study, we identified that 55.96% (n = 122) of workers had high work demand and 25.22% (n = 55) had low control. Among those who had high demand and low control at the beginning of the study, the majority were women (71.31% vs. 70.91%), of race/skin color referred to as non-white (86.06% vs. 87. 27%), with primary/high school education (56.55% vs. 60%), income from 1 to <3 minimum wages (74.59% vs. 70.91%), with 1 to 4 dependents in the family (90 0.98% vs. 89.09%), with less than 7 h of sleep/day (59.83% vs. 61.82%), had 1 to 5 meals/day (88.52% vs. 83. 64%), without another job (81.14% vs. 76.36%), worked during the day shift (64.75% vs. 70.91%) and with other types of employment relationships other than those governed by labor legislation of the country (96.73% vs. 90.91%) (Table 1).
Table 1. Sociodemographic characteristics of workers at baseline, according to high or low presence of demand and control.
After 12 months of follow-up, the median value for demand continued as 13 (IQR: 5–25) and for control, it increased to 16 (IQR: 9–23). In this second moment of the study, 62.38% (n = 136) of workers showed high demand and 45.87% (n = 100) low control. Among workers with high demand and low control, the majority remained women (71.32% vs. 73%), of race/skin color referred to as non-white (86.03% vs. 90%), with an income of 1 to <3 minimum wages (64.71% vs. 65%), with 1 to 4 dependents in the family (91.91% vs. 89%), had 1 to 5 meals/day (88.24% vs. 90%), had no other employment relationship (80.15% vs. 76%) and had other forms of employment other than the consolidation of Brazilian labor laws (96.32% vs. 91%) (Table 2).
Table 2. Sociodemographic characteristics of workers after 12 months of follow-up, according to high or low presence of demand and control.
Evaluating the average of the outcomes, workers in high demand had the highest indicators in both periods. At baseline, only BMI showed a statistically significant difference between workers with high and low demand (p = 0.024), whereas, after follow-up, only WC (p = 0.035) and FFM (p = 0.047) showed a difference statistically significant between workers with high and low demand (Table 3).
Table 3. Average values of body composition indicators according to demand and control at baseline and after follow-up, in hospital workers in Santo Antônio de Jesus, Bahia, Brazil, 2019–2020.
Regarding workers with high and low control at baseline, higher mean values for weight, BMI, WC, FFM and BF were identified in workers with low control. However, no statistically significant difference was observed for the outcomes evaluated. After follow-up, all mean values of the outcome variables were higher for workers with low control, with the difference being statistically significant for weight (p = 0.010), BMI (p = 0.013) and FFM (p = 0.012) (Table 3).
Table 4 presents the raw and adjusted LMEM results for the relationship between stress (assessed through the dimensions of demand and control) and adiposity indicators. After adjusting for age, weekly working hours, alcohol consumption, physical activity, having another job and education, we observed that individuals classified as high demand had an increase of 0.979 kg/m2 (p = 0.023) in mean BMI after the follow-up period, when compared to those classified as low demand; on the other hand, individuals classified in the low control category had a decrease of −1,180 kg/m2 (p = 0.008) in the mean of this indicator after follow-up, when compared to those classified as high control (Table 4).
Table 4. Linear mixed effects model for the relationship between occupational stress and body composition at 12 months of follow-up.
For WC, only high demand (estimate: 2.636; p = 0.018) presented a statistically significant result in the crude model. However, when adjusting for age, weekly working hours, number of meals/day, having another job, contract work and work shift, this significance did not remain (p = 0.066).
For FFM, in the crude model, high demand (estimate: 2.423; p = 0.008) was significant, but the significance was not maintained when adjusting for covariates. As for control, we observed that workers classified as low control had a reduction of −1,396 kg (p = 0.054) in the mean FFM after follow-up, compared to those classified as high control (Table 4).
When evaluating the BF outcome, we observed that, in the adjusted model, individuals classified as high demand showed an increase of 1,448 kg (p = 0.044) in mean BF after the follow-up period, compared to those with low demand. For control, workers classified as low control showed a decrease of −1,782 kg (p = 0.015) in mean BF after follow-up, compared to workers with high control (Table 4).
The constructed models were well adjusted to the data, according to the AIC and Log Restricted-Likelihood criteria identified, considering that there was a reduction in these indicators in the final models when compared to the raw models (Table 4).
This investigation suggests that high demand at work contributed to the increase in average BMI and body fat values, while low control at work reduced average BMI and BF values after one year of follow-up. Furthermore, low control at work influenced the average reduction in BMI, BF and FFM.
Assessing and monitoring workers’ body composition is essential to identify possible nutritional changes, contributing to improving health and performance of work activities (8, 54, 55). Despite the importance of the topic, we observe a rare scenario of studies in the literature that investigate longitudinal information, incorporating temporal variations between occupational stress and body composition in hospital workers.
Investigating the consequences of exposure to occupational stress, most studies adopt cross-sectional designs, which are unable to identify changes that occur in the work environment and that may impact health, as well as not being able to detect the influence of working conditions work on the health status of workers (56). Furthermore, they limit themselves to using variables such as weight, BMI and WC and do not use data from tests such as bioimpedance (23). Longitudinal studies are relevant because they allow the identification of variations of the same variable over time and consider the observations of a subject with himself and him with another subject over time. As a result, estimates become more precise, and the test is more sensitive to capturing significant differences (51, 57).
This investigation provides longitudinal information that suggests that high demand and low control influence BMI, therefore, BMI may be a consequence of working conditions. In this sense, this result corroborates previous findings where Bean et al. (42) found in a cohort in South Australia with 450 employees, that an increase of 1 unit in discretionary skill was associated with a decrease of 0.168 kg/m2 in the crude analysis of BMI (p = 0.005). Fujishiro et al. (58), in a cohort of nurses from the Nurses’ Health Study II, when associating changes in work tension and changes in BMI, identified that those who experienced a decrease (b = 0.063; p = 0.03) or increase (b = 0.077; p = 0.01) of work stress had greater BMI gain than those without exposure to work stress at any time.
At this juncture, working conditions can trigger stress and thus contribute to harmful outcomes for workers’ health, such as changes in the sleep–wake cycle, overweight and obesity (23, 25, 59, 60). Occupational stress is a worldwide public health problem that can influence body weight in several ways, such as, for example, favoring increased consumption of foods, that are primarily hyper-palatable and ultra-processed, and immersion in work activities. In everyday life, the individual does not notice the change in consumption patterns and the unhealthy increase in weight (24, 61, 62).
Eating habits can be altered by stressful situations (63), especially ego-threatening stressors (e.g., situations where there is “fear of failure”) and interpersonal stressors (e.g., ostracism, arguments) (64–66). Thus, when a worker is exposed to stress, his or her hypothalamus stimulates the pituitary gland to release corticotropin-releasing factor, leading to the secretion of adrenocorticotropic hormone in the anterior pituitary gland. This hormone then stimulates the adrenal glands to increase the rate of cortisol synthesis and release (67). High levels of cortisol, which also increase the sensitivity of the brain’s reward system, can lead to greater intake of hyperpalatable foods (24, 61, 62), increased consumption of saturated fat and sugar (68, 69), among others.
It is known that stress can be experienced from different dimensions of the environment due to physiological, psychological and social stressors (70). Given the complex social structure and the fact that control over the stressor does not always depend on it, the effects on appetite and weight may appear differently. Thus, recognizing the causes of stress is paramount in trying to reverse the problems (61). It is also essential to understand that positive energy balance, that is, the amount of calories ingested is greater than those expended, is not solely and exclusively in the physiological scope, but also in the environmental, social, cultural and genetic sphere (71).
The dynamics of the work carried out in hospitals—due to the uninterrupted operation, a large number of professions, the work shared between different occupations, the differences inherent to each work activity, the hierarchical rigidity and the undersizing of human resources—is favorable for trigger occupational stress (72–74). This population has stressful work dynamics with risk factors for increased weight and body fat, until overweight and obesity sets in Kołcz et al. (23). Among health-care professionals, for example, long working hours and shift work are observed (75–78), as well as limitations in the practice of physical activity (79, 80), reduction in the quality of food intake (23, 68, 79, 80), and undesirable impacts on sleep (81, 82).
Therefore, the literature is consistent in identifying that overweight and obesity are highly prevalent among hospital workers, especially health-care professionals (83–86). Furthermore, it is known that, despite being distinct, nutritional status and occupational stress are related (87, 88). Thus, in the presence of stress, nutritional status can be affected and, in this study, the dimensions of occupational stress assessed with the JSS demonstrated an influence on the increase in BMI. This finding was similar to those of Fujishiro et al. (58) in a longitudinal study with nurses, in which they identified that those with tension at work at both times increased their BMI compared to nurses who did not experience tension at work. A Swiss study of manual and administrative workers also identified that control and social stressors were predictors of BMI during study follow-up (89). Kivimäki et al. (90), in a cohort of workers from 20 Public Service departments in the Whitehall II study in London, identified that among men, high tension and low control at work in the highest BMI quintile (>27 kg/m2), were associated with later weight gain, but no corresponding interaction was observed among women.
In epidemiological studies evaluating the anthropometric status of individuals, only the BMI indicator is used, which, despite having the advantages of low cost, easy performance and serving as an indicator for more sensitive assessments, only considers weight regarding the individual’s height. In an individualized context, this is not the best option, as it does not distinguish body composition and distribution nor does it consider other aspects of obesity, such as genetic, metabolic, physiological or psychological factors. In other words, it does not diagnose, it only serves as an indicator for a more sensitive assessment and, in population assessments, it is the best tool available to date (21, 91). Thus, among the most accurate and reliable alternatives, the use of electrical bioimpedance is a good choice, as it is low cost, non-invasive, has good reproducibility, and there is the possibility of working with portable and easy-to-use equipment (20, 31, 37). However, despite these advantages, its use is still limited in the occupational medicine services of organizations.
This study identified a significant association between high demand and FFM in the raw model, high demand and BF in the adjusted model (p = 0.044) and low control with FFM and BF. The “Demand” dimension present in the instrument refers to how much the individual works, that is, it observes issues related to the development of their work activities, such as volume, pace, workload, etc. (43, 44, 60), while the “Control” dimension involves making decisions about one’s work and how much they develop creativity, learning from new things at work, carrying out multiple tasks, among others (43, 44, 60).
Thus, the findings can be explained by the characteristics that such dimensions represent in hospital work, where it is observed that workers have, for example, a large volume of work, long working hours, undersized staff, decision-making needs to take place in conjunction with other professionals and multiple tasks are performed, issues that contribute to work overload, impact on lifestyle, favoring the accumulation of adipose tissue and reduction in lean mass.
Furthermore, the mechanisms underlying the reduction in mean BMI and BF values associated with low control at work after one year of follow-up may have been because, for high control, workers need, among other things, to be able to make decisions about their own work and be able to develop their creativity. During the pandemic, such autonomy was not possible, and there was a need to perform multiple tasks. Another contributing factor was that most of our sample were women who historically experienced double work shifts. That is, in societies such as Brazil, even after working in the hospital, they are largely responsible for domestic work (92). This intensified during the pandemic, especially for those who were health professionals, due to the assistance and support they needed to offer to their families. Therefore, this scenario impacts diet, physical activity practices, and, consequently, body composition.
As far as we could ascertain, this study is a pioneer study in such an assessment with hospital workers and with this methodological approach. We did not identify studies that tested associations between occupational stress and FFM or BF in hospital workers, but we identified in a cross-sectional analysis and using BIA in Piauí—Brazil, with university hospital workers that 49.1% of the 53 health professionals studied had altered BF% and 15.1 had altered skeletal muscle mass index (82). In India, a study with 1,150 individuals, including students and health professionals, observed, using BIA, higher average values of %BF regardless of age group, sex and life cycles (adults vs. older adult) (93).
Given the above, we reaffirm that checking the body composition of individuals is important to identify possible nutritional disorders, in addition to guiding nutritional behaviors (31). In the field of workers’ health, this assessment remains necessary, mainly due to the labor dynamics within organizations and the growing and permanent precariousness of imposed working conditions. Although overweight and obesity are not occupational diseases, the high levels observed in institutions cannot be ignored. These high prevalences present challenges not only at the individual level, but also for the institution (42), resulting in more accidents at work and sick days (8), impacting productivity and incurring more expensive health plans for employers (94, 95), increased rates of absenteeism and presenteeism (16, 95), among other problems.
At the same time, it is worth highlighting the importance of evaluating stressful factors according to the different groups of professionals allocated to hospitals, especially among health workers, as depending on the role performed, work dynamics change and so do the risks of unwanted changes in body composition (55, 83, 84, 86). Despite this importance, it was not possible to perform such stratification in our study due to the sample size, which becomes a challenge to be achieved in future investigations.
Longitudinal investigations commonly have limitations. Firstly, in this study that data collection, at the time of follow-up, took place in the context of a pandemic, something rare to happen and, even historically, this workspace is recognized as favorable to occupational stress, a pandemic state certainly reinforces the high levels of stress experienced by these workers. However, we reinforce that the data collection and conduct of the study took place in the same way as in the baseline, that is, without a state of public calamity.
A second limitation is possibly the study’s follow-up period (12 months), which may not have been sufficient to observe some changes in body composition, especially variations in adiposity. However, the data obtained is reliable, as it comes from research with a robust methodology, where monitoring and conduction were well defined. Even though it presents such a limitation, we reinforce that the evidence listed here is based on a cohort study design with careful logistics and adoption of statistical treatment that is more appropriate to the data structure and an epidemiological approach. Furthermore, although the findings corroborate many studies with a cross-sectional design, this confirms the importance and need to study the influence of occupational stress on changes in body composition in hospital workers over time. A third limitation is that in this study, information on the use of medications (e.g., systemic corticosteroids, antidepressants, etc.) was not collected as well as the lack of detailed information on how moch and what the participant consume in each meal.
The study results indicate that high demand and low control at work are risk factors for changes in body mass index, fat mass and fat-free mass in hospital workers. This study shows the importance and need for clinical and epidemiological assessments regarding the body composition of professionals working in hospitals, since high rates of overweight and obesity are triggers of chronic health problems such as dyslipidemia, diabetes mellitus and cardiovascular diseases, among others. Therefore, managers need, initially, to promote adequate working conditions and understand the need for periodic body composition assessments.
Furthermore, our findings encourage future research on body composition associated with the dimensions of occupational stress independently in this population, instead of using present or absent stress globally, especially from a longitudinal perspective. Finally, even though our findings are not capable of bringing recommendations for changes in policies, it is worth highlighting that even though occupational stress is seen as prevalent in hospital work, it is urgent to realize that the work environment is potentially modifiable at the individual, collective and/or organizational.
The original contributions presented in the study are included in the article/Supplementary material, further inquiries can be directed to the corresponding author.
The studies involving humans were approved by Ethics and Research Committee of the School of Nutrition of the Federal University of Bahia, with the necessary updates (CAAE approval number 4.316.252) for studies involving humans. The studies were conducted in accordance with the local legislation and institutional requirements. The participants provided their written informed consent to participate in this study.
CL: Conceptualization, Formal analysis, Validation, Writing – original draft, Writing – review & editing. RA: Conceptualization, Methodology, Validation, Writing – review & editing. LC: Conceptualization, Methodology, Validation, Writing – review & editing. RZ: Validation, Writing – review & editing. PC: Conceptualization, Formal analysis, Methodology, Validation, Writing – review & editing.
The author(s) declare financial support was received for the research, authorship, and/or publication of this article. To the Fundação de Amparo a Pesquisa do Estado da Bahia (FAPESB) for the scholarship granted to CL (BOL0789/2020); and to the Coordenação de Aperfeiçoamento de Pessoal de Superior (CAPES).
The authors declare that the research was conducted in the absence of any commercial or financial relationships that could be construed as a potential conflict of interest.
The author(s) declared that they were an editorial board member of Frontiers, at the time of submission. This had no impact on the peer review process and the final decision.
All claims expressed in this article are solely those of the authors and do not necessarily represent those of their affiliated organizations, or those of the publisher, the editors and the reviewers. Any product that may be evaluated in this article, or claim that may be made by its manufacturer, is not guaranteed or endorsed by the publisher.
The Supplementary material for this article can be found online at: https://www.frontiersin.org/articles/10.3389/fpubh.2024.1459809/full#supplementary-material
SUPPLEMENTARY TABLE S1 | Job content questionnaire.
2. Khaghanizadeh, M, Ebadi, A, Sirati Nir, M, and Rahmani, M. The study of relationship between job stress and quality of work life of nurses in military hospitals. J. Mil. Med. (2008) 10:175–84. Available at: https://www.sid.ir/en/Journal/ViewPaper.aspx?ID=140964
3. Li, J, Riedel, N, Barrech, A, Herr, RM, Aust, B, Mörtl, K, et al. Long-term effectiveness of a stress management intervention at work: A 9-year follow-up study based on a randomized wait-list controlled trial in male managers. Biomed Res Int. (2017) 2017:1–11. doi: 10.1155/2017/2853813
4. Santana, LC, Ferreira, LA, and Santana, LPM. Occupational stress in nursing professionals of a university hospital. Rev Bras Enferm. (2020) 73:1–7. doi: 10.1590/0034-7167-2018-0997
5. Zare, S, Mohammadi dameneh, M, Esmaeili, R, Kazemi, R, Naseri, S, and Panahi, D. Occupational stress assessment of health care workers (HCWs) facing COVID-19 patients in Kerman province hospitals in Iran. Heliyon. (2021) 7:e07035. doi: 10.1016/j.heliyon.2021.e07035
6. Canazaro, BC, de Aguiar, OB, Moreno, AB, Alves, MGM, and Fonseca, MJM. Association between job stress and quality of life in nutritionists working in public hospitals in Rio de Janeiro, Brazil. Ciênc Saúde Colet. (2022) 27:1951–63. doi: 10.1590/1413-81232022275.11642021
7. Zhu, D, Wang, J, Zhao, Y, Yang, L, Gao, J, Chang, X, et al. The status of occupational stress and its influence on the health of medical staff in Lanzhou, China. Int J Environ Res Public Health. (2022) 19:1–13. doi: 10.3390/ijerph191710808
8. K, S, A, S, A, T, and G, K. The relationship of occupational stress, physical fitness levels, body fat percentage and absenteeism”. J Adv Sport Phys Educ. (2020) 3:23–8. doi: 10.36348/jaspe.2020.v03i02.001
9. Stuber, F, Seifried-Dübon, T, Tsarouha, E, Rahmani Azad, Z, Erschens, R, Armbruster, I, et al. Feasibility, psychological outcomes and practical use of a stress-preventive leadership intervention in the workplace hospital: the results of a mixed-method phase-II study. BMJ Open. (2022) 12:e049951. doi: 10.1136/bmjopen-2021-049951
10. Alves, M., Chor, D., Faerstein, E., Lopes, C., and Werneck, G., “Short version of the ‘job stress scale’: a Portuguese-language adaptation,” (2004). Available at: www.fsp.usp.br/rsp
11. dos Reis, ALPP, Fernandes, SRP, and Gomes, AF. Estresse e fatores psicossociais. Psicol Ciência e Profissão. (2010) 30:712–25. doi: 10.1590/s1414-98932010000400004
12. Dutheil, F, Pereira, B, Bouillon-Minois, JB, Clinchamps, M, Brousses, G, Dewavrin, S, et al. Validation of visual analogue scales of job demand and job control at the workplace: A cross-sectional study. BMJ Open. (2022) 12:e046403. doi: 10.1136/bmjopen-2020-046403
13. De Almeida, H, Brito-Costa, S, Alberty, A, Gomes, A, Lima, P, and Vicente Castro, F. Modelos De Stress Ocupacional: Sistematização, Análise E Descrição. Int J Dev Educ Psychol Rev INFAD Psicol. (2016) 2:434. doi: 10.17060/ijodaep.2016.n1.v2.309
14. Araújo, T, Graça, C, and Araújo, E. Estresse ocupacional e saúde: contribuições do Modelo Demanda-Controle. Ciênc Saúde Colet. (2003) 8:991–1003. doi: 10.1590/S1413-81232003000400021
15. Montzel, DRB, Costa, BVL, and Silva, FM. Ganho de peso por década entre trabalhadores de um hospital público: estudo de coorte histórica. Ciênc Saúde Colet. (2019) 24:2453–60. doi: 10.1590/1413-81232018247.17272017
16. de Wet, T, Kruger, WH, and Joubert, G. Obesity and sickness absenteeism among health workers in a private hospital in South Africa. South African Fam Pract. (2022) 64:e1–8. doi: 10.4102/safp.v64i1.5418
17. Heymsfield, SB, Wang, ZM, Baumgartner, RN, and Ross, R. Human body composition: advances in models and methods. Annu Rev Nutr. (1997) 17:527–58. doi: 10.1146/annurev.nutr.17.1.527
19. Freitas, J. I. F., Padronização de medidas antropométricas e avaliação da composição corporal. Conselho Regional de Educação Física da 4a Região– CREF4/SP (2018).
20. Eickemberg, M, De Oliveira, CC, Roriz, AKC, and Sampaio, LR. Bioelectric impedance analysis and its use for nutritional assessments. Rev Nutr. (2011) 24:883–93. doi: 10.1590/S1415-52732011000600009
21. Holmes, CJ, and Racette, SB. The utility of body composition assessment in nutrition and clinical practice: an overview of current methodology. Nutrients. (2021) 13:1–16. doi: 10.3390/nu13082493
22. Gram Quist, H, Christensen, U, Christensen, KB, Aust, B, Borg, V, and Bjorner, JB. Psychosocial work environment factors and weight change: A prospective study among Danish health care workers. BMC Public Health. (2013) 13:21–3. doi: 10.1186/1471-2458-13-43
23. Kołcz, A, Baran, M, Walewicz, K, Paprocka-Borowicz, M, and Rosińczuk, J. Analysis of selected body composition parameters and ergonomic safety among professionally active nurses in Poland: A preliminary prospective monocentric and observational study. Biomed Res Int. (2020) 2020:1–9. doi: 10.1155/2020/9212587
24. Groesz, LM, McCoy, S, Carl, J, Saslow, L, Stewart, J, Adler, N, et al. What is eating you? Stress and the drive to eat q. Appetite. (2012) 58:717–21. doi: 10.1016/j.appet.2011.11.028
25. Solovieva, S, Lallukka, T, Virtanen, M, and Viikari-Juntura, E. Psychosocial factors at work, long work hours, and obesity: A systematic review. Scand J Work Environ Health. (2013) 39:241–58. doi: 10.5271/sjweh.3364
26. Fitzgerald, S, Kirby, A, Murphy, A, and Geaney, F. Obesity, diet quality and absenteeism in a working population. Public Health Nutr. (2016) 19:3287–95. doi: 10.1017/S1368980016001269
27. Tonnon, SC, Robroek, SRJ, van der Beek, AJ, Burdorf, A, van der Ploeg, HP, Caspers, M, et al. Physical workload and obesity have a synergistic effect on work ability among construction workers. Int Arch Occup Environ Health. (2019) 92:855–64. doi: 10.1007/s00420-019-01422-7
28. Andersen, LL, Izquierdo, M, and Sundstrup, E. Overweight and obesity are progressively associated with lower work ability in the general working population: cross-sectional study among 10,000 adults. Int Arch Occup Environ Health. (2017) 90:779–87. doi: 10.1007/s00420-017-1240-0
29. van den Berg, S, Burdorf, A, and Robroek, SJW. Associations between common diseases and work ability and sick leave among health care workers. Int Arch Occup Environ Health. (2017) 90:685–93. doi: 10.1007/s00420-017-1231-1
30. Ramos, LB. Avaliação nutricional: escolares, adultos, idosos e hospitalizados. Salvador-BA: EDUFBA (2021).
31. Sampaio, LR, Eickemberg, M, Moreira, PA, and Oliveira, CC, Bioimpedância elétrica. Sampaios LR, org. Avaliação nutricional [online]. Salvador: EDUFBA. (2012) 113–132. Sala de aula collection.
32. Coelho, LG, Costa, PRF, Kinra, S, Mallinson, PAC, and Akutsu, RCCA. Association between occupational stress, work shift and health outcomes in Hospital Workers of the Recôncavo of Bahia, Brazil: the impact of Covid-19 pandemic. Br J Nutr. (2022) 129:147–56. doi: 10.1017/S0007114522000873
33. de Lira, CRN, Akutsu, RCCA, Coelho, LG, Zandonadi, RP, and Costa, PRF. Dietary patterns, occupational stressors and body composition of hospital workers: A longitudinal study comparing before and during the COVID-19 pandemic. Int J Environ Res Public Health. (2023) 20:1–16. doi: 10.3390/ijerph20032166
34. Instituto Brasileiro de Geografia e Estatística, “Densidade demográfica: Censo 2022: População e Domicílios.” Disponible: https://www.ibge.gov.br/estatisticas/sociais/trabalho/22827-censo-demografico-2022.html (2023).
35. Thomas, L. Retrospective power analysis. Conserv Biol. (1997) 11:276–80. doi: 10.1046/j.1523-1739.1997.96102.x
37. Eickemberg, M, Oliveira, CC, Roriz, AKC, Fontes, GAV, Mello, AL, and Sampaio, LR. Bioimpedância elétrica e gordura visceral: uma comparação com a tomografia computadorizada em adultos e idosos Bioelectrical impedance and visceral fat: a comparison with computed tomography in adults and elderly. Arquivos Brasileiros de Endocrinologia Metabologia. (2013) 57:27–32. doi: 10.1590/S0004-27302013000100004
38. World Health Organization. Obesity: Preventing and managing the global epidemic. 894th ed. Geneva, Switzerland: World Health Organization (2000).
39. Organización Mundial de la Salud, “WHO expert committee on physical status: The use and interpretation of anthropometry (1993: Geneva, Switzerland) & World Health Organization. El estado físico: uso e interpretación de la antropometría: informe de un comité de expertos de la OMS. Organización Mundial de la Salud. 1995,” Available at: https://iris.who.int/handle/10665/42132 (1995).
40. Kyle, UG, Bosaeus, I, de Lorenzo, AD, Deurenberg, P, Elia, M, Manuel Gómez, J, et al. Bioelectrical impedance analysis—part II: utilization in clinical practice. Clin Nutr. (2004) 23:1430–53. doi: 10.1016/j.clnu.2004.09.012
41. de Jonge, J, Reuvers, MM, Houtman, IL, Bongers, PM, and Kompier, MA. Linear and nonlinear relations between psychosocial job characteristics, subjective outcomes, and sickness absence: baseline results from SMASH. J Occup Health Psychol. (2000) 5:256–68. doi: 10.1037/1076-8998.5.2.256
42. Bean, CG, Winefield, HR, Sargent, C, and Hutchinson, AD. Differential associations of job control components with both waist circumference and body mass index. Soc Sci Med. (2015) 143:1–8. doi: 10.1016/j.socscimed.2015.08.034
43. Karasek, RA. Job demands, job decision latitude, and mental strain: implications for job redesign. Administrative Science Quarterly, vol. 24 (1979). 285–308.
44. Jacinto, A, and Tolfo, SR. Psychosocial risk factors at work and common mental disorder: a systematic review of studies using the JCQ instruments, JSS and SRQ-20. Rev Psicol Da Imed. (2018) 9:107–24. doi: 10.18256/2175-5027.2017.v9i2.1432
45. Senado Federal. Consolidação das Leis do Trabalho-CLT e normas correlatas. Brasília: Senado Federal, Coordenação de Edições Técnicas, 189. (2017).
46. Matsudo, S, Araujo, T, Matsudo, V, Andrade, D, Andrade, E, Oliveira, LC, et al. Questionario internacional de ativi dade fisica (i paq): estudo de vall dade ereprodutibilidade no brasil. Atividade Física e Saúde. (2001) 6:1–18. doi: 10.12820/rbafs.v.6n2p5-18
47. Craig, CL, Marshall, AL, Sjöström, M, Bauman, AE, Booth, ML, Ainsworth, BE, et al. International physical activity questionnaire: 12-country reliability and validity. Med Sci Sports. (2003) 35:1381–95. doi: 10.1249/01.MSS.0000078924.61453.FB
48. Rothman, K, Greenland, S, and Lash, T. Modern Epidemiology. 3rd ed. Philadelphia: Lippincott Williams & Wilkins (2008).
49. Hosmer, DW, and Lemeshow, S. Applied Logistic Regression Wiley-Interscience Publication 2nd edn. (2000).
50. Fausto, MA, Carneiro, M, Antunes, CM, Pinto, JA, and Colosimo, EA. Mixed linear regression model for longitudinal data: application to an unbalanced anthropometric data set. Cad Saude Publica. (2008) 24:513–24. doi: 10.1590/S0102-311X2008000300005
51. Twisk, JWR. Applied longitudinal data analysis for epidemiology: a practical guide, Department of Epidemiology and Biostatistics, Medical Centre and the Department of Health Sciences of the Vrije Universteit, Amsterdam. 2nd edition. vol. 38 (2013).
52. Belloco, R. Analysis of longitudinal data in Stata, SPlus and SAS. Stockholm: Department of Medical Epidemiology, Karolinska Institutet (2001).
53. Akaike, H. Information theory and an extension of the maximum likelihood principle In: GKE Parzen and K Tanabe, editors. Selected papers of Hirotugu Akaike. New York: New York: Springer-Verlag Inc (1998)
54. Schulte, PA, Wagner, GR, Ostry, A, Blanciforti, LA, Cutlip, RG, Krajnak, KM, et al. Work, obesity, and occupational safety and health. Am J Public Health. (2007) 97:428–36. doi: 10.2105/AJPH.2006.086900
55. Banwat, ME, Haruna, SA, Vongdip, NG, Duru, AK, and Afolaranmi, TO. Assessment of the nutritional knowledge, eating habits and nutritional statuses of healthcare workers in Jos, north-Central Nigeria. Res J Food Sci Nutr. (2018) 3:15–22. doi: 10.31248/RJFSN2018.037
56. Dobson, KG, Gilbert-Ouimet, M, Mustard, C, and Smith, PM. Body mass index trajectories among the Canadian workforce and their association with work environment trajectories over 17 years. Occup Environ Med. (2020) 77:374–80. doi: 10.1136/oemed-2019-106023
57. Baxter-Jones, ADG, Cardy, AH, Helms, PJ, Phillips, DO, and Smith, WCS. Influence of socioeconomic conditions on growth in infancy: the 1921 Aberdeen birth cohort. Arch Dis Child. (1999) 81:5–9. doi: 10.1136/adc.81.1.5
58. Fujishiro, K, Lawson, CC, Hibert, EL, Chavarro, JE, and Rich-Edwards, JW. Job strain and changes in the body mass index among working women: A prospective study. Physiol Behav. (2016) 176:100–6. doi: 10.1038/ijo.2015.91.Job
59. Theorell, T. The demand-control-support model for studying health in relation to the work environment: an interactive model In: K Orth-Gómer and N Schneiderman, editors. Behavioral medicine approaches to cardiovascular disease. Mahwah, NJ: Lawrence Erlbaum Associates (1996). 69–85.
60. Karasek, RA. “El modelo de Demandas-Control: enfoque social, emocional y fisiologico del risco de estres y desarrollo de comportamientos activos. In Organización Internacional del Trabajo (OIT). Enciclopedia de Salud y Seguridad en el Trabajo. Genebra: OIT. (1998) 34.6–34.16.
61. Razzoli, M, and Bartolomucci, A. The dichotomous effect of chronic stress on obesity. Trends Endocrinol Metab. (2017) 27:504–15. doi: 10.1016/j.tem.2016.04.007.The
62. Geiker, NRW, Astrup, A, Hjorth, MF, Sjödin, A, Pijls, L, and Markus, CR, “Does stress influence sleep patterns, food intake, weight gain, abdominal obesity and weight loss interventions and vice versa?,” Obesity Reviews. (2018). doi: 10.1111/obr.1260372
63. Scott, C, and Johnstone, AM. Stress and eating behaviour: implications for obesity. Obes Facts. (2012) 5:277–87. doi: 10.1159/000338340
64. Oliver, KG, Huon, GF, Zadro, L, and Williams, KD. The role of interpersonal stress in overeating among high and low disinhibitors. Eat Behav. (2001) 2:19–26. doi: 10.1016/S1471-0153(00)00020-9
65. O’Connor, DB, Jones, F, Conner, MT, and McMillan, B. Effects of daily hassles and eating style on eating behavior. Health Psychol. (2008) 27:S20–31. doi: 10.1037/0278-6133.27.1.S20
66. Wallis, DJ, and Hetherington, MM. Emotions and eating. Self-reported and experimentally induced changes in food intake under stress. Appetite. (2009) 52:355–62. doi: 10.1016/j.appet.2008.11.007
67. Foster, JA, Rinaman, L, and Cryan, JF. Stress & the gut-brain axis: regulation by the microbiome. Neurobiol Stress. (2017) 7:124–36. doi: 10.1016/j.ynstr.2017.03.001
68. Souza, RV, Sarmento, RA, de Almeida, JC, and Canuto, R. The effect of shift work on eating habits: a systematic review. Scand J Work Env. Heal. (2019) 45:7–21. doi: 10.5271/sjweh.3759
69. Hemiö, K, Lindström, J, Peltonen, M, Härmä, M, Viitasalo, K, and Puttonen, S. The association of work stress and night work with nutrient intake – a prospective cohort study. Scand J Work Environ Health. (2020) 46:533–41. doi: 10.5271/sjweh.3899
70. Stephens, MA, Mahon, PB, McCaul, ME, and Wand, GS. Hypothalamic-pituitary-adrenal axis response to acute psychosocial stress: effects of biological sex and circulating sex hormones. Psychoneuroendocrinology. (2016) 66:47–55. doi: 10.1016/j.psyneuen.2015.12.021
71. Faith, MS, and Kral, TV. Social environmental and genetic influences on obesity and obesity-promoting behaviors: fostering research integration In: L Hernandez and D Blazer, editors. Genes, behavior, and the social environment: Moving beyond the nature/nurture debate. Washington: Institute of Medicine, National Academies Press (2006)
72. Monteiro, J. K., and Carlotto, M. S., “Preditores da Síndrome de Burnout em Trabalhadores da Saúde no Contexto Hospitalar,” pp. 287–295, (2014).
73. Rama-maceiras, P, Jokinen, J, and Kranke, P, Stress and burnout in anaesthesia: a real world problem?, Curr Opin Anaesthesiol. (2015) 28:151–158. doi: 10.1097/ACO.0000000000000169
74. Caicedo, KPE, Urréa, HER, Reinoso, GNS, and Sanchez, EMM, “Estilos de Vida del Profesional Enfermero y su relación en la calidad de atención,” Revista Salud y Bienestar Colectivo. 4, pp. 44–584, (2020).
75. Aslam, M, Siddiqui, AA, Sandeep, G, and Madhu, SV. High prevalence of obesity among nursing personnel working in tertiary care hospital,” diabetes Metab. Syndr Clin Res Rev. (2017) 12:12–5. doi: 10.1016/j.dsx.2017.12.014
76. Liu, Q, Shi, J, Duan, P, Liu, B, Li, T, Wang, C, et al. Is shift work associated with a higher risk of overweight or obesity? A systematic review of observational studies with meta-analysis. Int J Epidemiol. (2018) 6:1–16. doi: 10.1093/ije/dyy079
77. Sánchez-Jiménez, B, Sámano, R, Chinchilla-ochoa, D, Morales-Hernández, R, and Rodríguez-Ventura, A, Demographics and psychological factors associated with adiposity in nurses. Int. J. Environ. Res. Public Health. (2018) 15:1–7. doi: 10.3390/ijerph150406344–10
78. Bernstrøm, VH, and Houkes, I, Shift work and sickness absence at a Norwegian hospital: a longitudinal multilevel study. Occup Environ Med. (2020) 1–9. doi: 10.1136/oemed-2019-106240
79. Fernandes, C, and Portela, LF. Jornada de trabalho e comportamentos de saúde entre enfermeiros de hospitais públicos, Rev. Latino-Am. Enfermagem, vol. 21 (2013) 1–8.
80. Diaz-carrion, EG, Failoc-rojas, VE, Pedro, N, Gallo, R, and De Loyola, SI, “Estilos de vida en profesionales de salud de un hospital en Chiclayo, Perú 2017,” Revista Habanera de Ciencias Médicas, 19, pp. 1–10, (2020).
81. Watanabe, K, Sugimura, N, Shishido, I, Konya, I, and Yamaguchi, S. Effects of 90 min napping on fatigue and associated environmental factors among nurses working long night shifts: a longitudinal observational study. Int J Environ Res Public Health. (2022) 19:9429. doi: 10.3390/ijerph19159429
82. Sousa, MAS, Barros, GM, Souza, FL, Vilar, TM, Dias, RSC, Batista, MMSL, et al., “Associação entre qualidade do sono e composição corporal de profissionais da saúde de um hospital universitário,” Research, Society and Development. (2023), pp. 1–12. doi: 10.33448/rsd-v12i4.40821
83. Kyle, RG, Neall, RA, and Atherton, IM. Prevalence of overweight and obesity among nurses in Scotland: A cross-sectional study using the Scottish health survey. Int J Nurs Stud. (2016) 53:126–33. doi: 10.1016/j.ijnurstu.2015.10.015
84. Kyle, RG, Wills, J, Mahoney, C, Hoyle, L, Kelly, M, and Atherton, IM. Obesity prevalence among healthcare professionals in England: a cross- sectional study using the health survey for England. BMJ Open. (2017) 7:1–8. doi: 10.1136/bmjopen-2017-018498
85. Adaja, TM, and Idemudia, OJ, “Prevalence of overweight and obesity among health—care Workers in University of Benin teaching hospital, Benin City, Nigeria,” Annals of Tropical Pathology. 9:150–154, (2018). doi: 10.4103/atp.atp_30_18
86. Kunyahamu, MS, Daud, A, and Jusoh, N. Obesity among health-care workers: which occupations are at higher risk of being obese. Int J Environ Res Public Health. (2021) 18:4381. doi: 10.3390/ijerph18084381
87. Singh, M. B., Fotedar, R., and Lakshminarayana, L., “Occupational morbidities and their association with nutrition and environmental factors among textile Workers of Desert Areas of Rajasthan, India,” Journal of Occupational Health. (2005) 47:371–377. doi: 10.1539/joh.47.371
88. Medhi, GK, Hazarika, NC, Shah, B, and Mahanta, J. Health problems and nutritional status of tea garden population of Assam. J Med Sci. (2006) 60:496–505. doi: 10.4103/0019-5359.28979
89. Berset, M, Semmer, NK, Elfering, A, Jacobshagen, N, and Meier, LL. Does stress at work make you gain weight? A two-year longitudinal study. Scand J Work Env Heal. (2011) 37:45–53. doi: 10.5271/sjweh.3089
90. Kivimäki, M, Head, J, Ferrie, JE, Shipley, MJ, Brunner, E, Vahtera, J, et al. Work stress, weight gain and weight loss: evidence for bidirectional effects of job strain on body mass index in the Whitehall II study (2006) 30:982–7. doi: 10.1038/sj.ijo.0803229
92. Harryson, L, Strandh, M, and Hammarström, A. Domestic work and psychological distress-what is the importance of relative socioeconomic position and gender inequality in the couple relationship? PLoS One. (2012) 7:1–7. doi: 10.1371/journal.pone.0038484
93. Rai, R, Ghosh, T, Jangra, S, Sharma, S, Panda, S, and Kochhar, KP. Relationship between body mass index and body fat percentage in a Group of Indian Participants: A cross-sectional study from a tertiary care hospital. Cureus. (2023) 15:e47817. doi: 10.7759/cureus.47817
94. Heinen, L, and Darling, H. Addressing obesity in the workplace: the role of employers. The Milbank Quarterly. (2009) 87:101–22. doi: 10.1111/j.1468-0009.2009.00549.x
Keywords: linear models, worker’s health, likelihood functions, anthropometry, mixed effects models, cohort study
Citation: de Lira CRN, Akutsu RdCCdA, Coelho LG, Zandonadi RP and Costa PRdF (2024) Occupational stress and body composition of hospital workers: a follow-up study. Front. Public Health. 12:1459809. doi: 10.3389/fpubh.2024.1459809
Received: 04 July 2024; Accepted: 30 September 2024;
Published: 17 October 2024.
Edited by:
Filip Kukic, University of Banja Luka, Bosnia and HerzegovinaReviewed by:
Tanja Sobot, University of Banja Luka, Bosnia and HerzegovinaCopyright © 2024 de Lira, Akutsu, Coelho, Zandonadi and Costa. This is an open-access article distributed under the terms of the Creative Commons Attribution License (CC BY). The use, distribution or reproduction in other forums is permitted, provided the original author(s) and the copyright owner(s) are credited and that the original publication in this journal is cited, in accordance with accepted academic practice. No use, distribution or reproduction is permitted which does not comply with these terms.
*Correspondence: Carlos Rodrigo Nascimento de Lira, Y2FybG9zLnJvZHJpZ28ubkBob3RtYWlsLmNvbQ==
Disclaimer: All claims expressed in this article are solely those of the authors and do not necessarily represent those of their affiliated organizations, or those of the publisher, the editors and the reviewers. Any product that may be evaluated in this article or claim that may be made by its manufacturer is not guaranteed or endorsed by the publisher.
Research integrity at Frontiers
Learn more about the work of our research integrity team to safeguard the quality of each article we publish.