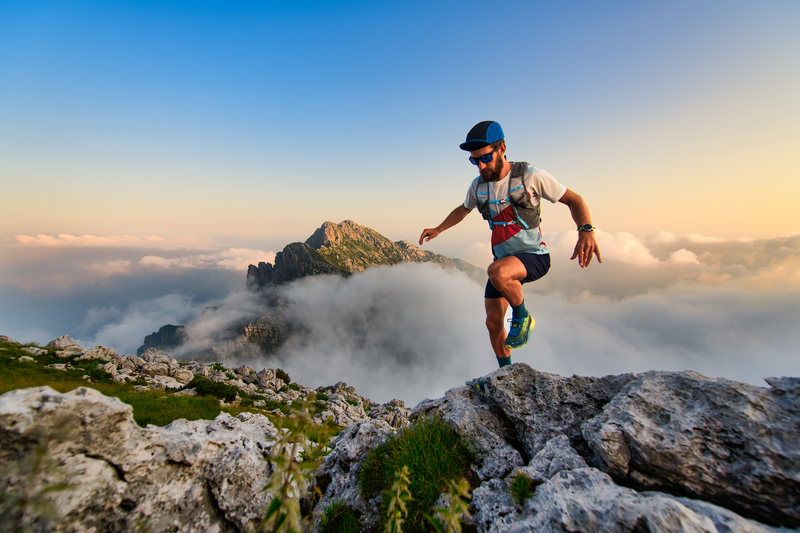
94% of researchers rate our articles as excellent or good
Learn more about the work of our research integrity team to safeguard the quality of each article we publish.
Find out more
ORIGINAL RESEARCH article
Front. Public Health , 04 December 2024
Sec. Health Economics
Volume 12 - 2024 | https://doi.org/10.3389/fpubh.2024.1448343
Background: Multimorbidity, the coexistence of two or more chronic conditions in an individual, has emerged as a significant public health challenge with profound economic implications, exerting substantial strain on healthcare systems and economies worldwide. This study aimed to estimate the prevalence of non-communicable diseases (NCD) related multimorbidity, catastrophic health expenditure (CHE), and associated factors among adults aged ≥40 years in Ernakulam district.
Methods: A community-based cross-sectional study was conducted among 420 individuals aged ≥40 years using population probability sampling. The tools used were the Multimorbidity Assessment Questionnaire for Primary Care (MAQ-PC), the WHO STEPS Questionnaire, and the Patient Health Questionnaire-9 (PHQ-9), which assessed non-communicable diseases, diet, physical activity, smoking, alcohol consumption, and depression. A pretested semi-structured questionnaire collected data on health and household expenditures. Multimorbidity was defined as having two or more self-reported NCDs, and CHE was identified when health costs exceeded 10% of a household’s expenditure in the past month. Multivariable logistic regression was performed to find independent predictors of multimorbidity and CHE.
Results: The mean age of participants was 60.02 ± 10 years. The prevalence of NCD multimorbidity was 42.6% (95% CI: 37.9–47.3%). The most common dyad was diabetes and hypertension (24.5, 95% CI: 20.4–28.6%). Higher odds of multimorbidity were observed in those aged ≥60 years (aOR = 3.03, 95% CI: 1.95–4.73), unmarried/widowed/divorced (aOR = 2.15, 95% CI: 1.28–3.63), unemployed (aOR = 1.81, 95% CI: 1.14–2.87), and tobacco users (aOR = 3.72, 95% CI: 1.85–7.48). Approximately 32.4% (95% CI: 25.5–39.3%) of households incurred catastrophic health expenditure (CHE) by treating adults with multimorbidity. Age ≥ 60 (aOR = 2.39, 95% CI: 1.99–5.77) and use of outpatient services (aOR = 4.09, 95% CI: 2.01–8.32) were independently associated with higher odds of CHE. IP services and each additional morbidity add ₹22,082.37 (β = 0.557, p < 0.001, 95% CI: ₹17,139.88– ₹27,024.86) and ₹1,278.75 (β = 0.128, p = 0.044, 95%CI: ₹35.58–₹2,521.92) to healthcare costs, respectively.
Conclusion: The high prevalence of multimorbidity and associated CHE among individuals over 60 years highlights the urgent need for the National Programme for the Prevention and Control of Non-Communicable Diseases to prioritise multimorbidity and its management, especially above 60 years within this age group.
Multimorbidity presents a significant challenge to health services, both currently and in the foreseeable future (1). It refers to the simultaneous presence of two or more chronic conditions within the same individual. Although they are frequently associated with non-communicable diseases (NCDs), these problems are not just related to them (2). The rising older population and the escalating incidence of chronic illnesses underscore multimorbidity as a critical issue in global public health. Up to 90% of people worldwide, including various age groups, suffer from multimorbidity (3). The prevalence of multimorbidity in South Asia varies widely, ranging from 4.5 to 83% (4). In India, a 2017 study reported an overall prevalence of 23.3%, with Kerala showing the highest prevalence at 42% (5). Another community-based study from the South Indian state of Andhra Pradesh estimated multimorbidity at 58.5% (6), while research across seven Indian states found a prevalence of 30.7% (7). A community-based study in Kerala reported a prevalence of 45.4% (8), whereas two hospital-based studies showed varying prevalence rates, from 16.2 to 39.8% (9, 10).
Understanding the factors contributing to multimorbidity is essential for devising successful strategies aimed at the early identification of at-risk individuals and preventing future health complications (11). These factors encompass a wide array of influences, including individual characteristics as well as broader societal and environmental factors. Given India’s present demographics, it is more important to identify multimorbidity in all adult groups, not only the older population (12). Even though multimorbidity is known to be significantly correlated with age, additional research into potential risk factors is required.
Kerala is in an advanced stage of epidemiological transition characterised by the rising burden of diabetes and hypertension, with multimorbidity emerging as a significant public health concern impacting approximately half of the adult population within the productive age group (8). While several studies have attempted to estimate the prevalence of multimorbidity, the majority have focussed on primary healthcare settings and the older population.
Multimorbidity poses significant health challenges, leading to adverse outcomes such as disability, increased mortality rates, poor quality of life, frequent hospitalisations, heightened utilisation of medical resources, and escalated healthcare expenditure (13). Many health systems, geographic locations, illness combinations, and individual characteristics such as age and social disadvantage will likely have very diverse effects on the costs and resources of healthcare due to multimorbidity. In some instances, the treatment of individuals with multimorbidity can impose a significant financial burden on families, leading to Catastrophic Health Expenditure (CHE). The incidence of CHE is on the rise, often driven by high out-of-pocket healthcare spending (14). Additional factors contributing to CHE include lack of health insurance, poverty, the type of medical conditions, and the absence of a robust government-funded healthcare system.
The objective of universal health coverage, as outlined in Target 3.8 of the United Nations’ Sustainable Development Goals for 2030, is to establish a healthcare system wherein individuals can access services without facing financial hardships (15). On the contrary, according to an analysis of Social Consumption Health data from the National Sample Survey Organisation’s 75th round which was carried out in India between 2017 and 2018, 46.7% of households incurred catastrophic healthcare costs (using a 10% threshold) as a result of any non-communicable disease (NCD). This percentage increased to approximately 63.3% in the presence of multimorbidity, compared to approximately 39.4% for any communicable disease (16).
Therefore, a comprehensive understanding of the epidemiology of multimorbidity, particularly related to non-communicable diseases (NCDs), is essential for restructuring healthcare services to offer integrated care for individuals with multiple chronic conditions. There is a notable knowledge gap that exists on the community-level prevalence of multimorbidity among the adult population, its factors, and the diverse patterns of multimorbidity. The catastrophic health expenditure encountered by households in the treatment of these conditions has also not been studied much.
The current study aimed at determining the prevalence of NCD-related multimorbidity at the community level, along with its associated factors among adults aged 40 years and above in Ernakulam district, Kerala. We also attempted to estimate the proportion of households that encountered catastrophic health expenditure by treating such adults.
A community-based cross-sectional study was conducted in rural and urban areas of Ernakulam district, situated in the central part of Kerala in Southern India, which has a population of 3.2 million. A cluster sampling with probability proportionate to size sampling (PPS) was carried out, and 20 clusters from the district were chosen for the study. A sampling frame with all the Community Development (CD) Blocks/towns of Ernakulam district was initially prepared, and the population of all the areas was listed. The total population was divided by the number of clusters to determine the sampling interval, and a random number was generated with the help of an online random number generator. A panchayat/block was then selected as the first cluster containing the cumulative population less than or equal to the random number. The sampling interval was then added 19 times to obtain the 20 clusters. From each cluster, a ward was identified randomly using computer-generated random numbers.
Adults aged 40 years and above who had been residing in Ernakulam district were included in the study. The exclusion criteria were those who had been staying in the district for less than 6 months. The sample size was determined based on a study by Rohini et al. (8), which reported a prevalence of 45.4% for non-communicable disease multimorbidity. With an absolute precision of 7 and applying a design effect of 2, the sample size was calculated to be 388, and we have included 420 participants in our study.
The outcome variables in the study were NCD multimorbidity and households that incurred catastrophic health expenditure (CHE). ‘Multimorbidity’ is defined as the coexistence of two or more chronic conditions in the same individual (17) and a household is said to incur ‘Catastrophic Health Expenditure’, when the total expenditure on health exceeds 10% of total household expenditure or income in the preceding month (SDG 3.8.2) (18). Data on NCD multimorbidity were collected by identifying the coexistence of two or more chronic conditions over the past 12 months, as self-reported by the study participants and verified against their corresponding medical records. To calculate the health expenditure, details regarding outpatient services, inpatient services, home care services, and other monthly healthcare charges in the previous month were gathered. Outpatient services’ expenses consisted of consultation fees, cost of medicines, investigations, and transportation costs. Inpatient services in the previous month details were gathered regarding the amount of total hospital bill, cost of medicines and investigations (not included in the main hospital bill), cost of food and transport, the duration of hospital stay, and the caregiver’s or the accompanying person’s income loss. Inquiries were made regarding the fees associated with home care services, including charges for a home nurse, consultation at home, and the expenses related to medications or investigations after a visit by a healthcare provider. The independent variables collected included sociodemographic details, information regarding diet, physical activity, smoking and alcohol consumption, anthropometric measurements, health insurance patterns, and monthly household expenditure. To calculate monthly household expenditure, we included house rent, loan repayment, travel, food, media, educational expenditure, electricity and water charges, and domestic help.
Sociodemographic details were collected by using a structured questionnaire. Data on NCD multimorbidity were collected using the Multimorbidity Assessment Questionnaire for Primary Care (MAQ-PC) developed and validated in India (19) and translated into the local language (Malayalam). The Patient Health Questionnaire-9 (PHQ-9) (20) was used to screen and assess the severity of depression in individuals. Each question was scored on a scale from 0 to 3, representing “Not at all,” “Several days,” “More than half the days,” and “Nearly every day,” and PHQ-9 scores of 5, 10, 15, and 20 represented mild, moderate, moderately severe, and severe depression, respectively. Information on diet, physical activity, smoking, and alcohol consumption was collected using the WHO Stepwise Approach to Surveillance (STEPS) Questionnaire (21). A pretested semi-structured questionnaire was used to gather data relating to health expenditure and household spending incurred by individuals in the preceding month.
The data collected using Epicollect 5 software (22) were exported to Microsoft Excel, and the analysis was conducted using SPSS version 21. The quantitative variables were expressed as mean and SD, and qualitative variables were mentioned as proportions. The chi-square test was used to assess the factors associated with multimorbidity and catastrophic health expenditure. All determinants with a p-value in chi-square test <0.2 were further used in the logistic regression modelling, using a backward conditional model. A p-value of less than 0.05 was considered to be statistically significant. The multivariable logistic regression helped to determine the independent predictors of multimorbidity and catastrophic health expenditure and was expressed as an odds ratio with a 95% confidence interval. Multiple linear regression analysis was conducted to determine the independent predictors influencing OOPE. Latent class analysis (LCA) was performed to identify subgroups (latent classes) based on patterns of multimorbidity. The optimal number of latent classes was determined using model fit indices such as the Akaike Information Criterion (AIC), Bayesian Information Criterion (BIC), deviance statistic (G2), and chi-square goodness of fit (χ2) (23, 24). Class population proportions and probabilities of non-communicable diseases (NCDs) within each class were then reported. Analysis for this study was conducted using the ‘poLCA’ package in RStudio V.1.1.463 (R Studio).
Prior to the commencement of the study, an ethical clearance certificate (ECASM-AIMS-2023-006) was obtained from the Amrita School of Medicine Ethical Committee. Informed consent was taken from all the study participants.
The mean age of the study participants was 60.02 ± 10 years, and more than a third 151 (36%) of the participants were 60–69 years old. The majority (278, 66.2%) of the participants were females. The majority (296, 70.5%) had an education above high school, and only 18 (4.3%) had no formal schooling. Only 47 participants (11.2%) reported being smokers, and only 45 (10.7%) reported alcohol consumption. Approximately half of the participants 196 (46.8%) were in the obese category (Table 1).
The overall prevalence of NCD multimorbidity was found to be 42.6% (95% CI: 37.9–47.3) and less than a third (31.7%) of the study participants reported only one condition. The prevalence of multimorbidity begins to noticeably increase from the age of 45 (8.9, 95% CI: 6.2–11.6) and continues to rise steadily until the age of 75, reaching 83.3% (95% CI: 81.5–85.1; Figure 1). Approximately a quarter of the study population 21.9% (95% CI: 17.9–25.0) reported having two chronic conditions (dyad). Additionally, 13.3% (95% CI: 10.0–16.6), 3.3% (95% CI: 1.6–5.0), and 4% (95% CI: 2.1–5.9) reported having three (triad), four (quad), and more than five (penta and above) chronic conditions, respectively.
The study identified diabetes and hypertension as the most common dyad (pairs of conditions) observed in multimorbidity 24.5% (95% CI: 20.4–28.6; Figure 2). The second most common pair was hypertension–depression (11.4, 95% CI: 8.4–14.4) followed by diabetes and depression (10.7, 95% CI: 7.7–13.6).
Based on the bivariate analysis to determine the association of multimorbidity and its determinants, factors such as age, marital status, education, occupation, type of family, family size, and tobacco use were significant with a p-value less than 0.05 (Table 2).
The multivariable logistic regression model showed that factors such as age, marital status, occupation, and tobacco use were the independent predictors associated with multimorbidity. Individuals aged 60 years and above had 3.03 (95% CI: 1.95–4.73) times higher odds of multimorbidity. Those who were unmarried/widowed/divorced had 2.15 (95% CI: 1.28–3.63) times more risk of multimorbidity compared to married individuals. Regarding employment status, those who were unemployed had 1.81 times higher risk of multimorbidity (95% CI: 1.14–2.87). In terms of tobacco use, individuals who had ever used tobacco had 3.72 times higher odds of multimorbidity compared to those who had never used tobacco (95% CI: 1.85–7.48; Table 3).
Table 3. Results of multivariable logistic regression analysis for independent predictors of multimorbidity.
Table 4 illustrates the results of the LCA model fit. The AIC and BIC values decreased from the one-class to the three-class models, and the G2 and χ2 values also showed a similar decline, reflecting an improvement in the model fit. However, starting with the four-class model, the AIC and BIC values began to rise, which means that adding more latent classes does not significantly improve the model. Based on these metrics—AIC, BIC, G2, and χ2—the 3-latent class model provided the best balance between goodness-of-fit and model simplicity.
The results from class proportion and item-response probabilities (ρ) are presented in Table 5. The item responses with high probabilities were used to assign labels to the identified three-class model. Class 1 comprised the majority (73.42%) of the population and generally had low probabilities of having the majority of morbidities except for diabetes (0.40) and hypertension (0.23), making this a relatively healthy class. Class 2 represented 21.17% of the population and was labelled as cardiometabolic/mental and reported high probabilities of hypertension (1.00), diabetes (0.73), depression (0.40), and ischaemic heart disease (0.32), indicating a moderate risk class. Class 3 represented a small proportion of 5.41% of the study population was labelled as cardiorespiratory/metabolic/mental and reported high probabilities for a wide range of morbidities, including COPD/Asthma (1.00), hypertension (0.54), depression (0.53), ischaemic heart disease (0.50), and diabetes (0.48), making it the high-risk group. In all three latent classes, diabetes and hypertension emerged as common morbidities, indicating their widespread prevalence across different risk groups within the population.
Table 5. Class proportions and item-response probabilities from the three-latent class model of morbidities.
Approximately a third of the households 32.4% (95% CI: 25.5–39.3) encountered catastrophic health expenditure. In this context, only a quarter of the individuals 24.6% (95% CI: 20.5–28.7) experiencing multimorbidity had some form of health insurance. The majority of this insurance 61.4% (95% CI: 56.7–66.0) was from the government.
Factors such as the age of the adult with multimorbidity (p < 0.05), socioeconomic status (p < 0.05), requirement of outpatient and inpatient services in the past month (p < 0.001), and mode of meeting expenses during the healthcare visit in the previous month (p < 0.001) had a significant association with catastrophic health expenditure on the bivariate analysis (Table 6). Households with individuals aged 60 years and above who have multimorbidity were 2.39 (95% CI: 1.99–5.77) times and utilisation of outpatient services in the past month were found to have 4.09 (95% CI: 2.01–8.32%) times more risk of incurring catastrophic health expenditure compared to their counterparts (Table 7).
Table 7. Multivariable logistic regression analysis for identifying the independent predictors of catastrophic health expenditure among households.
The distribution of Out-of-Pocket Expenditure (OOPE) among the different multimorbidity groups is shown in Table 8. The median OOPE was found to be higher among the penta and above multimorbidity group than the other groups (dyad, triad, and quad). Multiple linear regression analysis to explore potential factors influencing OOPE observed that inpatient (IP) services and the total number of morbidities were significant predictors of healthcare costs. IP services emerged as the stronger predictor (β = 0.557, p < 0.001), with patients who utilised IP services incurring an average additional cost of ₹22,082.37 (95% CI: ₹17,139.88 to ₹27,024.86) compared to those who did not. Additionally, each extra morbidity (from dyad to penta or more) was associated with an increase in healthcare costs by ₹1,278.75 (β = 0.128, p = 0.044, 95% CI: ₹35.58 to ₹2,521.92; Table 9).
Table 9. Multiple linear regression analysis for identifying the independent predictors of out-of-pocket health expenditure.
In the present study, non-communicable disease multimorbidity was prevalent in 42.6% of the individuals aged 40 years and above in Ernakulam district. Older adults, as well as those who were unmarried/widowed/divorced, unemployed, and individuals with a history of tobacco use, were more likely to have multimorbidity. Our study provides initial estimates of the costs related to multimorbidity. Approximately a third of the households 32.4% encountered catastrophic health expenditure. Households with individuals aged 60 years and above having multimorbidity, the utilisation of outpatient services in the past month had a double and quadruple times higher risk of incurring catastrophic health expenditure than their counterparts.
The higher prevalence of multimorbidity in Kerala may be due to the advanced stage of epidemiological and demographic transition. The state’s rapid urbanisation, changing lifestyles, and ageing population have contributed to a rising burden of chronic conditions. Kerala’s high literacy rates and healthcare awareness create both opportunities and challenges in dealing with multimorbidity (25).
Studies from various parts of Kerala, hospital-based and community-based studies have also reported a similar prevalence ranging from 39.8% (10) to 45.4% (8), respectively. However, another study in six states of India (26) among younger individuals aged 18 years and above, reported a lower prevalence of 8.9%. Secondary data analysis from a national survey revealed the multimorbidity prevalence to be 30.7% in the older adults aged 60 years and above (7). In a systematic review and meta-analysis conducted across 54 countries, multimorbidity was 37.2% (27).
An increase in age above 60 years has been found to elevate the risk of multimorbidity, a finding corroborated by both Indian and global studies (3, 4, 28). The ageing population is more prone to multimorbidity due to several factors related to ageing, such as prolonged exposure to risk factors, natural physiological decline, cascading health issues where one condition triggers others, increased use of medications, and the associated side effects. A recent systematic analysis (27) has also found the same. In Kerala, where life expectancy is higher than the national average, the extended lifespan provides more time for multiple chronic conditions to develop and coexist.
In the present study, individuals who were unmarried, widowed, or divorced also had a high risk of multimorbidity compared to those who were married. A longitudinal study in multiple countries showed that those who were widowed, divorced, or separated had higher odds of multimorbidity (29). Unmarried/widowed/divorced individuals might face barriers in accessing healthcare services and seeking healthy behaviours due to financial constraints, and lack of social and family support, leading to less healthy lifestyle choices and an increased risk of developing multiple chronic conditions (30).
In our study, it was observed that unemployed individuals had an increased risk of multimorbidity. The finding was consistent with a previous study in Kerala, where unemployed individuals had higher odds of multimorbidity (8). The higher prevalence of multimorbidity in unemployed individuals could potentially be due to inadequate physical activity and unhealthy dietary habits. Our study also found a higher odds of multimorbidity among those who ever used tobacco. Increased risk of multimorbidity among smokers was also confirmed by an English longitudinal study of ageing (31).
In this study, dyads (comprising two chronic conditions) were the most prevalent pattern of multimorbidity, affecting 21.9% of participants, followed by triads at 13.3%. This pattern appears to be consistent globally, with similar findings reported in countries such as India, China (32, 33), and various developed nations. This was further underscored by the latent class analysis where diabetes and hypertension (metabolic cluster) were found to have high probabilities across all three latent classes. As highly prevalent conditions in all multimorbid classes, diabetes and hypertension may serve as gateway diseases, facilitating the development of other non-communicable diseases.
Our study found that approximately a third 32.4% households of adults living with multimorbidity incurred CHE and this was more among households with older adult members. Outpatient care in the preceding month also led the patient’s family to incur catastrophic health expenditures. The National Sample Survey Office (NSSO) 75th round (2017–2018) reported that approximately 63.3% of households incurred catastrophic spending (using a 10% threshold) under an NCD multimorbidity scenario. They also noted that outpatient care for conditions such as cancer and cardiovascular diseases is underfinanced by 45–50% in the presence of multimorbidity in comparison to standalone diseases (16). CHE prevalence was, however, lower than 45.6% in a longitudinal study conducted in households of older adults (≥60 years) living with multimorbidity in China (34). Older adults with chronic diseases or multimorbidity often require long-term medication and regular medical check-ups, leading to increased demand for medical services and imposing economic burdens on their families. Despite the importance of health insurance in preventing catastrophic health expenditure, our study did not find any significant differences in its protective role compared to households without insurance.
With Kerala bearing a significant burden of NCDs, this study, with a representative sample of 420 participants from both rural and urban areas, offers valuable insights into the current prevalence and risk factors of multimorbidity. To our knowledge, this is the first-ever study conducted in Kerala to estimate the catastrophic health expenditure encountered by households while treating adults with multimorbidity. The findings of this study could pave the way for further research initiatives within the state and can also guide the policymakers to formulate strategies ensuring financial risk protection among households in the future.
There is a possibility of recall bias since multimorbidity was determined through self-reporting, which may lead to an underestimation of its true prevalence. However, the high literacy rate, strong health awareness, and proactive healthcare-seeking behaviour of the population reduce the likelihood of underestimating or overestimating the issue. In this study, a 1-month recall was used to estimate the health expenditure among the households, which might influence the accuracy of the results. CHE was estimated based on the previous month. This may not be representative of the year.
The research underscores the increasing burden of multimorbidity among the older adults, unmarried individuals, unemployed, and tobacco users, emphasising the urgent need for the National Programme for the Prevention and Control of Non-Communicable Diseases to formulate comprehensive protocols that address multimorbidity among these groups. These protocols should be facilitated through comprehensive health assessments and integrated management strategies for patients with multiple co-existing conditions. To protect households from significant catastrophic health expenditures, the research highlights the necessity for drafting better policies that provide financial protection to households in the future.
The raw data supporting the conclusions of this article will be made available by the authors, without undue reservation.
The studies involving humans were approved by Institutional Ethics Committee, Amrita Institute of Medical Sciences, Kochi. (ECASM-AIMS-2023-142 006). The studies were conducted in accordance with the local legislation and institutional requirements. The participants provided their written informed consent to participate in this study.
SJ: Conceptualization, Data curation, Formal analysis, Methodology, Project administration, Resources, Software, Writing – original draft. AS: Conceptualization, Methodology, Resources, Supervision, Validation, Visualization, Writing – review & editing. RB: Data curation, Formal analysis, Writing – review & editing.
The author(s) declare that no financial support was received for the research, authorship, and/or publication of this article.
We would like to acknowledge all the eminent faculties and staff of the Department of Community Medicine, Amrita Institute of Medical Sciences, Kochi. The authors also thank all the study participants for their sincere support throughout the research.
The authors declare that the research was conducted in the absence of any commercial or financial relationships that could be construed as a potential conflict of interest.
All claims expressed in this article are solely those of the authors and do not necessarily represent those of their affiliated organizations, or those of the publisher, the editors and the reviewers. Any product that may be evaluated in this article, or claim that may be made by its manufacturer, is not guaranteed or endorsed by the publisher.
1. Pearson-Stuttard, J, Ezzati, M, and Gregg, EW. Multimorbidity-a defining challenge for health systems. Lancet Public Health. (2019) 4:e599–600. doi: 10.1016/S2468-2667(19)30222-1
2. Technical Series on Safer Primary Care. Multimorbidity. (2024). Available from: https://www.who.int/publications-detail-redirect/9789241511650 (Accessed on April 12, 2024)
3. Garin, N, Koyanagi, A, Chatterji, S, Tyrovolas, S, Olaya, B, Leonardi, M, et al. Global multimorbidity patterns: a cross-sectional, population-based, multi-country study. J Gerontol A Biol Sci Med Sci. (2016) 71:205–14. doi: 10.1093/gerona/glv128
4. Pati, S, Swain, S, Hussain, MA, van den Akker, M, Metsemakers, J, Knottnerus, JA, et al. Prevalence and outcomes of multimorbidity in South Asia: a systematic review. BMJ Open. (2015) 5:e007235. doi: 10.1136/bmjopen-2014-007235
5. Himanshu, H, and Talukdar, B. Prevalence of multimorbidity (chronic NCDS) and associated determinants among elderly in India. Demography India. (2017) 69–76.
6. Gummidi, B, Gautam, V, John, O, Ghosh, A, and Jha, V. Patterns of multimorbidity among a community-based cohort in rural India. J Multimorb Comorb. (2023) 13:26335565221149623. doi: 10.1177/26335565221149623
7. Mini, GK, and Thankappan, KR. Pattern, correlates and implications of non-communicable disease multimorbidity among older adults in selected Indian states: a cross-sectional study. BMJ Open. (2017) 7:e013529. doi: 10.1136/bmjopen-2016-013529
8. Jeemon, P. Prevalence and patterns of multi-morbidity in the productive age group of 30-69 years: a cross-sectional study in Pathanamthitta District, Kerala. Wellcome Open Res. (2020) 5:233. doi: 10.12688/wellcomeopenres.16326.1
9. Vargese, SS, Mathew, E, Johny, V, and Kurian, N. Prevalence and pattern of multimorbidity among adults in a primary care rural setting. Clinical epidemiology and global. Health. (2020) 8:482–5. doi: 10.1016/j.cegh.2019.10.014
10. Ismail, S, Stanley, A, and Jeemon, P. Prevalence of multimorbidity and associated treatment burden in primary care settings in Kerala: a cross-sectional study in Malappuram District, Kerala. India Wellcome Open Res. (2022) 7:67. doi: 10.12688/wellcomeopenres.17674.2
11. Harkness, E, Macdonald, W, Valderas, J, Coventry, P, Gask, L, and Bower, P. Identifying psychosocial interventions that improve both physical and mental health in patients with diabetes: a systematic review and meta-analysis. Diabetes Care. (2010) 33:926–30. doi: 10.2337/dc09-1519
12. Reddy, MM, Zaman, K, Yadav, R, Yadav, P, Kumar, K, and Kant, R. Prevalence, associated factors, and health expenditures of noncommunicable disease multimorbidity-findings from Gorakhpur health and demographic surveillance system. Front Public Health. (2022) 10:842561. doi: 10.3389/fpubh.2022.842561
13. Garin, N, Olaya, B, Moneta, MV, Miret, M, Lobo, A, Ayuso-Mateos, JL, et al. Impact of multimorbidity on disability and quality of life in the Spanish older population. PLoS One. (2014) 9:e111498. doi: 10.1371/journal.pone.0111498
14. Wagstaff, A, Flores, G, Hsu, J, Smitz, MF, Chepynoga, K, Buisman, LR, et al. Progress on catastrophic health spending in 133 countries: a retrospective observational study. Lancet Glob Health. (2018) 6:e169–79. doi: 10.1016/S2214-109X(17)30429-1
15. Bernardes, GM, Saulo, H, Fernandez, RN, and Lima-Costa, MF. Catastrophic health expenditure and multimorbidity among older adults in Brazil. Rev Saude Publica. (2020) 54:125. doi: 10.11606/s1518-8787.2020054002285
16. Karan, A, Farooqui, HH, Hussain, S, Hussain, MA, Selvaraj, S, and Mathur, MR. Multimorbidity, healthcare use and catastrophic health expenditure by households in India: a cross-section analysis of self-reported morbidity from national sample survey data 2017-18. BMC Health Serv Res. (2022) 22:1151. doi: 10.1186/s12913-022-08509-x
17. Academy of Medical Sciences, UK. (2018) 82222577.pdf [online]. Available at: https://acmedsci.ac.uk/file-download/82222577 (Accessed April 23, 2024)
18. SDG 3.8.2. Catastrophic health spending (and related indicators). (2024). Available from: https://www.who.int/data/gho/data/themes/topics/financial-protection/GHO/financial-protection (Accessed April, 23 2024).
19. Pati, S, Hussain, MA, Swain, S, Salisbury, C, Metsemakers, JFM, and Knottnerus, JA. Development and validation of a questionnaire to assess multimorbidity in primary care: an Indian experience. Biomed Res Int. (2016) 2016:1–9. doi: 10.1155/2016/6582487
20. Kroenke, K, Spitzer, RL, and Williams, JB. The PHQ-9: validity of a brief depression severity measure. J Gen Intern Med. (2001) 16:606–13. doi: 10.1046/j.1525-1497.2001.016009606.x
21. World Health Organisation. Standard STEPS instrument. (2024). Available from: https://www.who.int/publications/m/item/standard-steps-instrument (Accessed May, 9 2024).
22. CGPS Team of Oxford BDI. Free and easy-to-use mobile data-gathering platform. (2024). Available from: https://five.epicollect.net/ (Accessed June, 12 2024).
23. Larsen, FB, Pedersen, MH, Friis, K, Glümer, C, and Lasgaard, M. A latent class analysis of multimorbidity and the relationship to socio-demographic factors and health-related quality of life. A National Population-Based Study of 162,283 Danish adults. PLoS One. (2017) 12:e0169426. doi: 10.1371/journal.pone.0169426
24. Weller, BE, Bowen, NK, and Faubert, SJ. Latent class analysis: a guide to best practice. J Black Psychol. (2020) 46:287–311. doi: 10.1177/0095798420930932
25. Muraleedharan, M, and Chandak, AO. Emerging challenges in the health systems of Kerala, India: qualitative analysis of literature reviews. J Health Res. (2021) 36:242–54. doi: 10.1108/JHR-04-2020-0091
26. Pati, S, Agrawal, S, Swain, S, Lee, JT, Vellakkal, S, Hussain, MA, et al. Non communicable disease multimorbidity and associated health care utilization and expenditures in India: cross-sectional study. BMC Health Serv Res. (2014) 14:451. doi: 10.1186/1472-6963-14-451
27. Chowdhury, SR, Chandra Das, D, Sunna, TC, Beyene, J, and Hossain, A. Global and regional prevalence of multimorbidity in the adult population in community settings: a systematic review and meta-analysis. EClinicalMedicine. (2023) 57:101860. doi: 10.1016/j.eclinm.2023.101860
28. Violan, C, Foguet-Boreu, Q, Flores-Mateo, G, Salisbury, C, Blom, J, Freitag, M, et al. Prevalence, determinants and patterns of multimorbidity in primary care: a systematic review of observational studies. PLoS One. (2014) 9:e102149. doi: 10.1371/journal.pone.0102149
29. Wang, D, Dai, X, Mishra, SR, Lim, CCW, Carrillo-Larco, RM, Gakidou, E, et al. Association between socioeconomic status and health behaviour change before and after non-communicable disease diagnoses: a multicohort study. Lancet Public Health. (2022) 7:e670–82. doi: 10.1016/S2468-2667(22)00157-8
30. Pandey, KR, Yang, F, Cagney, KA, Smieliauskas, F, Meltzer, DO, and Ruhnke, GW. The impact of marital status on health care utilization among Medicare beneficiaries. Medicine (Baltimore). (2019) 98:e14871. doi: 10.1097/MD.0000000000014871
31. Mounce, LTA, Campbell, JL, Henley, WE, Tejerina Arreal, MC, Porter, I, and Valderas, JM. Predicting incident multimorbidity. Ann Fam Med. (2018) 16:322–9. doi: 10.1370/afm.2271
32. Kshatri, JS, Palo, SK, Bhoi, T, Barik, SR, and Pati, S. Prevalence and patterns of multimorbidity among rural elderly: findings of the AHSETS study. Front Public Health. (2020) 8:582663. doi: 10.3389/fpubh.2020.582663
33. Zhang, R, Lu, Y, Shi, L, Zhang, S, and Chang, F. Prevalence and patterns of multimorbidity among the elderly in China: a cross-sectional study using national survey data. BMJ Open. (2019) 9:e024268. doi: 10.1136/bmjopen-2018-024268
Keywords: non-communicable diseases, multimorbidity, health expenditure, catastrophic, Kerala
Citation: Joy S, Sreedevi A and Bhaskaran R (2024) Non-communicable diseases related multimorbidity, catastrophic health expenditure, and associated factors in Ernakulam district. Front. Public Health. 12:1448343. doi: 10.3389/fpubh.2024.1448343
Received: 28 June 2024; Accepted: 18 November 2024;
Published: 04 December 2024.
Edited by:
Amrit Banstola, Brunel University London, United KingdomReviewed by:
Sayem Ahmed, University of Glasgow, United KingdomCopyright © 2024 Joy, Sreedevi and Bhaskaran. This is an open-access article distributed under the terms of the Creative Commons Attribution License (CC BY). The use, distribution or reproduction in other forums is permitted, provided the original author(s) and the copyright owner(s) are credited and that the original publication in this journal is cited, in accordance with accepted academic practice. No use, distribution or reproduction is permitted which does not comply with these terms.
*Correspondence: Aswathy Sreedevi, ZHJhc3dhdGh5Z29wYW5AZ21haWwuY29t
Disclaimer: All claims expressed in this article are solely those of the authors and do not necessarily represent those of their affiliated organizations, or those of the publisher, the editors and the reviewers. Any product that may be evaluated in this article or claim that may be made by its manufacturer is not guaranteed or endorsed by the publisher.
Research integrity at Frontiers
Learn more about the work of our research integrity team to safeguard the quality of each article we publish.