- 1Department of Psychiatry, The Third People's Hospital of Huzhou Municipal, The Affiliated Hospital of Huzhou University, Huzhou, Zhejiang, China
- 2Lishui Second Hospital, Wenzhou Medical University, Lishui, Zhejiang, China
- 3School of Mental Health, Wenzhou Medical University, Wenzhou, Zhejiang, China
- 4Cixi Biomedical Research Institute, Wenzhou Medical University, Ningbo, China
- 5The Affiliated Wenzhou Kangning Hospital, Wenzhou Medical University, Wenzhou, Zhejiang, China
Background: The rapid growth of internet use among older adults in rural China offers a unique opportunity to examine its potential impact on their health. This study seeks to explore the relationship between internet use and the emotional, physical, and cognitive health of empty-nest older adults in rural China, while also considering the mediating role of social participation in this relationship.
Methods: A total of 3,478 empty-nest older adults were selected from the China Health and Retirement Longitudinal Survey (CHARLS) in 2020. Among them, 820 individuals were included in the study using a propensity score matching (PSM) method to reduce the impact of selection bias. The participants' depression, physical health, and cognitive health were measured using the Center for Epidemiological Studies Depression Scale (CES-D), the Activities of Daily Living (ADL) scale, and a composite cognition score, respectively. Social participation was assessed based on engagement in various activities over the past month.
Results: After controlling for confounding factors through PSM, it was found that older adults who used the internet exhibited significantly lower level of depression and higher physical health compared to older adults who did not use the internet. However, there was no significant difference in cognitive health between the two groups. The mediating effect analysis revealed that social participation partially mediated the relationship between internet use and physical health, but not emotional health.
Conclusion: The study suggests that internet use can improve emotional and physical health of older adults living in rural China without children at home, with social participation being a mediator in the relationship between internet use and physical health. Future research should explore the underlying mechanisms and develop targeted interventions to promote internet use and social engagement in this population.
1 Introduction
The global aging issue is receiving increasing attention, with China seeing the highest growth rate of aging population globally (1). According to the National Bureau of Statistics of China, the number of individuals aged 60 and above in China had reached 280 million by the end of 2022, accounting for 19.8% of the total population. A large portion of this demographic consists of empty-nest older adults residing in rural areas, totaling ~67 million individuals, or one-fourth of the older population (2).
In China's older population, empty-nest rural older adults represent a group that warrants particular attention. Defined as individuals aged 60 and above living in rural areas without children or family members (3), these older adults differ significantly from their urban counterparts. They encounter unique challenges, including limited access to healthcare services, social support, and technological infrastructure. encounter unique challenges such as social isolation, loneliness, and limited healthcare access (4–7). These disparities can profoundly impact their emotional, physical, and cognitive health (8–10). Furthermore, China is undergoing rapid aging and urbanization, resulting in a substantial migration of younger generations from rural to urban areas. This migration leaves many older adults in rural regions without the support of their children, thereby exacerbating the challenges they face (11).
The absence of close relatives may result in feelings of loneliness, isolation, and despair, heightening the risk of depression (12, 13). For example, a study conducted in rural China found that the prevalence of depressive symptoms among empty-nest older adults was 44.2%, which was significantly higher than that of non-empty-nest older adults (26.3%) (14, 15). Additionally, the lack of mental stimulation and social interaction can lead to cognitive decline, affecting memory, attention, and executive function, potentially increasing the risk of dementia (16, 17). A meta-analysis of studies conducted in China found that empty-nest older adults (22.9%, 95% CI: 18.9–27.3%) had a higher risk of cognitive impairment compared to non-empty-nest older adults (19.3%, 95% CI: 15.9–23.0%) (18). Moreover, the absence of assistance in daily activities may contribute to a decline in physical function, impacting mobility, strength, and balance, and raising the risk of physical inactivity and chronic diseases such as cardiovascular disease, diabetes, and cancer (19–21). Therefore, it is crucial to investigate the factors contributing to these health challenges and develop effective interventions to improve the emotional, cognitive, and physical health of empty-nest older adults in rural China.
The rapid development of the internet has been recognized as a potential strategy to address challenges and promote the health of empty-nest rural older adults (22). In China, digital technology, notably smartphones, has seen increased adoption among older age groups (23). As of 2021, more than half of older adults aged 65–69 use smartphones, with 31.2% of those aged 70–79 and 1.3% of those aged 100 and above also using smartphones (24). Additionally, according to recent statistics, the overall internet penetration rate among older adults in China has been steadily increasing. For instance, the China Internet Network Information Center (CNNIC) reported that as of 2021, the number of older adult Internet users aged 60 years or older in China reached 119 million, and the Internet penetration rate reached 43.2%, indicating a significant rise in internet use among the older adult population (25). However, the digital divide remains more pronounced in rural areas (26). Exploring how internet use can benefit rural empty-nest older adults is essential for bridging this divide and promoting equitable access to technology. China's rural revitalization strategy aims to narrow the urban-rural gap, and understanding the impact of internet use on the health of rural empty-nest older adults can inform policy decisions and interventions. The Social Support Theory posits that social relationships and support can buffer against stress, promote health and wellbeing, and encourage healthier behaviors (27). In the context of internet use, online communication and engagement can expand social networks and provide additional sources of social support, which can be particularly beneficial for empty-nest older adults in rural areas who may otherwise feel socially isolated. Previous studies have indicated that internet use can have a positive impact on the health of older adults (23, 28, 29). For instance, a study in America revealed that internet use was linked to improved self-rated health and reduced depressive symptoms in older adults (30). Similarly, a study in China demonstrated that internet use was associated with enhanced cognitive function and decreased feelings of loneliness among older adults (31). Nonetheless, there have been conflicting findings in the literature. Some studies have found no significant relationship between internet use and health outcomes, while others have even reported negative associations (32, 33). For instance, a study in the United States showed that internet use did not correlate with improved physical or mental health, and excessive internet use was linked to adverse health consequences like depression and sleep issues (34).
Inconsistent findings on the relationship between internet use and health in older adults may be attributed to the lack of control for confounding variables. These extraneous factors can influence both internet use and health outcomes, leading to a potentially misleading association. Variables such as age, gender, education level, marital status, number of children, chronic disease, smoking, alcohol consumption, physical activity, and children's support can impact both internet use and health outcomes in older adults (32, 35–37). For instance, younger older adults are more inclined to use the internet compared to their older counterparts, and health issues related to age can affect internet use (38). Higher levels of education are associated with increased internet use among older adults (38). Moreover, older men often use the internet for information and communication purposes, while older women utilize it for social support (35). The number of children may also be a factor, with individuals having more children potentially having greater social support and relying less on online communication (35). Therefore, it is imperative to consider these variables in order to establish a more precise relationship between internet use and health.
In an effort to address potential biases from confounding variables, this research employs propensity score matching (PSM) to balance the characteristics of individuals who use the internet and those who do not. PSM is a statistical technique that matches individuals based on their probability of receiving a specific treatment or intervention, while accounting for the factors that affect this probability. By utilizing PSM, this study aims to reduce sample selection bias and improve the precision of determining the causal link between internet use and health results.
Furthermore, the impact of internet use on the health of empty-nest older adults in rural China and the underlying mechanisms are not yet fully understood. Social participation is widely acknowledged as a crucial factor that can significantly influence the health of older adults (39). Social participation involves engaging in activities that facilitate interaction with others, such as volunteering, participating in community events, joining social clubs, and attending religious services (40). These activities not only provide opportunities for social interaction and integration for older adults but also foster a sense of purpose and belonging, which can lead to improved health outcomes (41, 42).
The Social Capital Theory provides a theoretical framework for understanding how social participation can mediate the relationship between internet use and health outcomes. According to this theory, social capital refers to the resources embedded in social networks and relationships, which can be accessed and utilized to achieve certain goals (43). Internet use facilitates the accumulation and mobilization of social capital by expanding social networks, fostering trust, and promoting reciprocity. This enhanced social capital can then translate into improved health outcomes through various mechanisms (44). Studies have shown that social participation in older adults is associated with positive health benefits, including a reduced risk of depression (45, 46), enhanced cognitive function (47, 48), better physical health (49, 50), and increased longevity (51, 52). For example, Glass et al. (53) found that participating in social activities lowered the risk of dementia development in older adults. Similarly, Li et al. noted a connection between social participation and enhanced physical functioning as well as a decrease in chronic health conditions among the older adults (54).
The potential of internet use to promote social participation among older adults has been recognized (55). The internet serves as a platform for older individuals to connect with others, access information, and engage in social activities, irrespective of their physical location or mobility. A growing body of research suggests that internet use can not only enhance social participation but also improve health outcomes in older adults (56–58). For example, Cotten et al. (59) found that internet use was linked to increased social participation and decreased loneliness in older adults. Similarly, Chopik (90) discovered a positive association between internet use and social support and wellbeing in older adults (59). Furthermore, Shapira et al. found that internet use was correlated with improved cognitive function in older adults, potentially due to increased social participation and engagement (60). Therefore, it is hypothesized that internet use may promote health by enhancing social participation among empty-nest older adults in rural China, as facilitated by the accumulation and mobilization of social capital.
To address these challenges, this study aims to investigate the relationship between internet use and health outcomes in empty-nest older adults in rural China while controlling for confounding variables such as age, gender, education level, marital status, number of children, chronic diseases, smoking, drinking alcohol, physical activity, and children's support (35). Additionally, the study aims to explore the mediating role of social participation in this relationship. Using data from the China Health and Retirement Longitudinal Survey (CHARLS) 2020 and employing propensity score matching (PSM) to reduce sample selection bias, the research seeks to provide valuable insights into the potential health benefits of internet use for this population.
2 Method
2.1 Participants
The data for this study were collected from the China Health and Retirement Longitudinal Survey (CHARLS) conducted by the National Development Institute of Peking University (http://charls.ccer.edu.cn/charls/). CHARLS is a nationally representative longitudinal survey of individuals in China aged 45 and above, covering various aspects such as demographic background, family, health status, healthcare, work, retirement, income, expenditure, assets, and interviewer observations. The baseline national wave of CHARLS took place in 2011 and continued every 2 years. Data will be made public 1 year after collection (61). The study protocol was approved by the Ethical Review Committee of Peking University (IRB00001052-11015). Respondents were given a statement explaining the purpose of the study, and all study participants signed a written informed consent prior to being investigated. All methods will be carried out in accordance with relevant guidelines and regulations of the Declaration of Helsinki.
For our analysis, participants were selected from the latest 2020 data of CHARLS. In this study, empty-nest rural older adults were defined as individuals aged 60 and above who live in rural areas and do not have children or other family members living with them. Initially, 4,951 participants without rural household registration and 6,166 adults under 60 were excluded, leaving 8,250 rural participants aged 60 and above. Subsequently, 4,772 non-empty nest participants and 916 invalid cases (e.g., missing values on internet use) were removed, resulting in 2,562 subjects. Using PSM, 454 older adults in the internet use group were matched with 2020 older adults in the non-internet use group based on twelve covariates, resulting in 410 participants in each group (refer to Figure 1 for samples election).
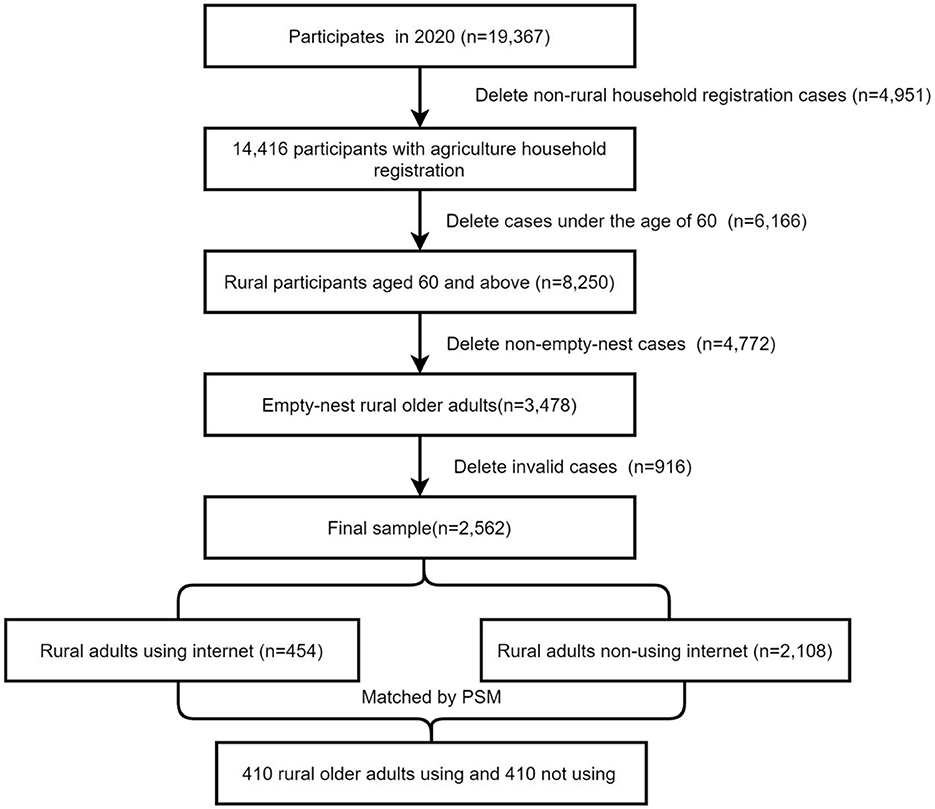
Figure 1. The construction of data. Source: Zhao et al. (91).
2.2 Measures
2.2.1 Independent variable
In this paper, Internet use is selected as the independent variable. According to the previous study, internet use was determined based on a question from the CHARLS 2020 Questionnaire, which asked respondents whether they had used the internet in the preceding month (61). Activities considered as being online included chatting on mobile networks, reading news, watching videos, playing games, and others. Respondents who answered “yes” were categorized as internet users, while those who answered “no” were categorized as non-internet users. This categorization was used to differentiate between older adults in rural areas who use the internet and those who do not.
2.2.2 Outcome variable
2.2.2.1 Emotional health
Depression, a prevalent emotional health concern among older adults, was evaluated in this research using a simplified version of the Center for Epidemiologic Studies Depression Scale (CES-D). The CES-D, a commonly used depression assessment tool in household surveys (62), has been validated for use in the Chinese population (63). In the CHARLS study, depression levels were measured through 10 items, each representing a different scenario related to depressive symptoms. Participants were asked to report how often they experienced these scenarios in the past week, with response options ranging from “almost none” (less than 1 day) to “most of the time” (5–7 days). Responses were scored from 0 to 3, with higher scores indicating higher levels of depression. The CES-D has good psychometric properties, with high internal consistency (Cronbach's alpha coefficients typically ranging from 0.85 to 0.90), good test-retest reliability (correlations typically ranging from 0.50 to 0.70), and strong construct validity (63–65). In our study, the Cronbach's alpha value for the CES-D 10 was 0.85, indicating good internal consistency and reliability of the measurement.
2.2.2.2 Physical health
The physical health of participants in CHARLS was evaluated using the Activities of Daily Living (ADL) scale, a reliable and valid tool widely utilized in both China and internationally (66). This scale comprises 12 items that assess an individual's capability to carry out fundamental self-care activities such as dressing, bathing, eating, and managing money, among others. Participants rated their difficulty level for each item on a 4-point scale, ranging from “No difficulty” to “Cannot do it”, with higher scores indicating increased task difficulty. A cumulative score was calculated by summing the scores for all items, with a higher total score reflecting greater independence in ADL. Individuals reporting difficulty in any of the 12 items were categorized as having an ADL disability. Previous research has demonstrated that the Chinese version of the ADLscale exhibits strong internal consistency (Coefficient Alpha = 0.86) and good test-retest reliability (r = 0.90) (67). The ADLs scale in CHARLS has proven to be a valid measure of older adults' daily performance (68). Measurement of ADL functions is essential as it serves as an early sign of functional decline in old age (69). These measurements are also predictors of the need for alternative living arrangements, the utilization of paid home care, and admission to nursing homes (69). In the current sample, the ADLscale show good internal consistency (Coefficient Alpha = 0.83).
2.2.2.3 Cognitive health
The evaluation of cognitive health in the CHARLS questionnaire uses the episodic memory and mental intactness sections from the Mini-Mental State Examination (MMSE) designed and developed by Folstein et al. (70). Episodic memory was assessed through immediate and delayed word recall tests, while mental intactness was measured using tasks such as numerical ability (serial subtraction of 7 from 100, five times), time orientation (including today's date, day of the week, month, year), and picture drawing (intersecting pentagon copying test). The mental intactness tasks were graded on a scale of 0 to 11, with higher scores indicating better cognitive function (71). Episodic memory performance was determined by participants' ability to recall a list of 10 words immediately and after a delay, with scores ranging from 0 to 10 for both instances (72). A composite cognition score was computed by adding the scores from the episodic memory and mental intactness tasks, resulting in a total score ranging from 0 to 31. Previous research has shown that the MMSE have strong internal consistency (Coefficient Alpha = 0.85–0.96) and good test-retest reliability (r = 0.80-0.85) (70, 73). These tests have been widely used and validated for measuring cognitive ability utilized in CHARLS (74–76).
2.2.2.4 Social participation
Based on the previous studies, the social participation of respondents was assessed using the CHARLS questionnaire, which inquired about engagement in various activities over the past month. These activities included interacting with friends or neighbors, playing games like mahjong or cards, caring for sick or disabled adults, participating in physical activities like dancing or qigong, involvement in community organizations, volunteering, attending educational courses, and engaging in other social activities. A score of 0 was assigned to respondents who did not partake in any of these activities, while those who participated received a score corresponding to the number of activities they engaged in.
2.2.3 Covariates
In this study, based on data from the 2020 CHARLS survey, eleven potential confounding covariates were identified: age, gender, education status, marital status, number of children, chronic disease, smoking, drinking alcohol, physical activity, children's emotional support, and children's financial support. Education level categories ranged from 1 (illiteracy) to 11 (doctoral degree/Ph.D.), while marital status was categorized as 0 (single) or 1 (partnered/married). Physical activity was assessed by the frequency of engaging in physical activity for at least 10 min per week. Children's emotional support was evaluated by the frequency of communication through various means when not residing with the participant. Children's financial support was measured by the amount of financial assistance received from children in the previous year.
2.3 Statistical analysis
In this study, PSM is a crucial statistical method used to mitigate sample-selection bias and mixed bias by pairing individuals in the treatment group with similar counterparts in the control group (77, 78). PSM analysis typically involves two main steps (77). The initial step entails computing the propensity score and then matching subjects based on this score. The propensity score, derived from a logit regression model, is utilized to match subjects from the Internet use group and non-usage group under comparable circumstances. It is estimated as follows:
In the above formula, P represents the probability of subjects using internet, D = 1 represents subjects in the using internet group, and X refers to covariates.
The secondly analysis is to estimate the Average Treatment effect on the Treated (ATT) by the following model, which reflects the effect of using internet on the emotional, physical and cognitive health of older adults. The significance of ATT is tested by a paired t-test (79).
In the above formula, Y1 and Y0 are the dependent variables of the matched samples in the using internet and non-using internet groups respectively. P is the propensity value, D=0 indicates the subjects non-using internet group, and D=1 indicates subjects with using internet. As Y0|D = 1 cannot be directly observed, the establishment of the above model must satisfy the “unconfoundedness assumption”, which requires to control factors associating with older adults' using internet group. In order to test the robustness of PSM, various matching methods are generally used. In our study, we used four matching methods: nearest neighbor matching, kernel matching, optimal matching and radius matching in all.
Finally, to examine the mediating role of social participation in the relationship between Internet use and health outcomes, a mediation analysis might have been conducted. This analysis would involve assessing the direct and indirect effects of Internet use on emotional and physical health, with social participation as the mediator.
To compare differences between the using internet group and the non-using internet group, the independent sample t-test and Chi-square test were employed. The significance level for all tests was set at 0.05. PSMATCH2 and NNMATCH in Stata 14.0 were performed to conduct the statistical analysis.
3 Results
3.1 Descriptive statistics of each variable
The study includes a total of 16 variables, comprising three outcome variables, one independent variable, and 12 covariates. Table 1 presents the item coding, measurement units, and descriptive statistics for each variable.
3.2 Covariates' balancing test before matching
Covariate balancing tests were conducted between groups of older adults based on internet use. Table 2 displays significant differences in 11 covariates, with the exception of chronic disease, between the two groups. The internet user group exhibited higher levels of education, social participation, physical activity, and other factors compared to the non-internet user group. These notable variations should be taken into account when assessing the relationships between internet use and the emotional, physical, and cognitive health of older adults.
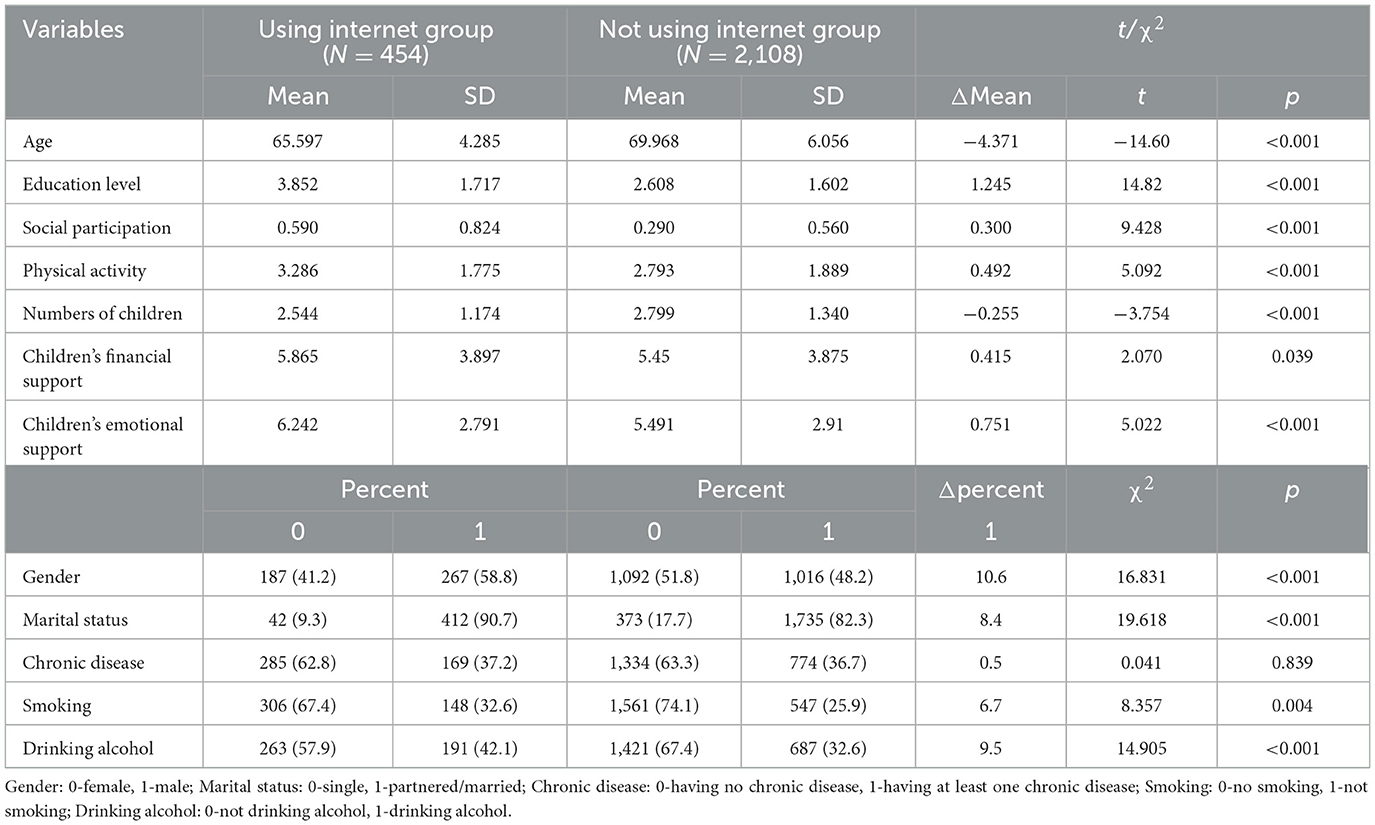
Table 2. Covariates' differences test between using internet group and not using internet group before matching.
3.3 Propensity score matching analysis
A logistic regression model was conducted with internet use as the output variable and twelve covariates as predictor variables. Specifically, age (p < 0.01), education level (p < 0.01), physical activity (p = 0.017), children's emotional support (p = 0.02), children's financial support (p = 0.010), and social participation (p < 0.01) were found to be significant predictors of older adults' internet use (as shown in Table 3). Subsequently, these regression results were utilized to develop a prediction model that calculates the likelihood of an older adult using the internet. A higher propensity score indicates a greater likelihood of internet use among older adults.
3.4 Matching and balancing test
The propensity score was calculated to match 454 older adults who use the internet with 2,018 older adults who do not use the internet. The nearest-neighbor matching method (1:1) was employed, resulting in a total of 410 pairs of successfully matched samples. Following matching, the independent sample t-test for each covariate between the internet-using and non-using groups no longer showed significant differences (p > 0.05) (as shown in Table 4). Figure 2 displays the absolute values of standardized mean differences before matching (circles) and after matching (triangles). With the exception of the chronic disease covariate, the standard deviations of all covariates substantially improved after matching, with absolute values below 0.1, indicating that the assumption of covariate balance was met.
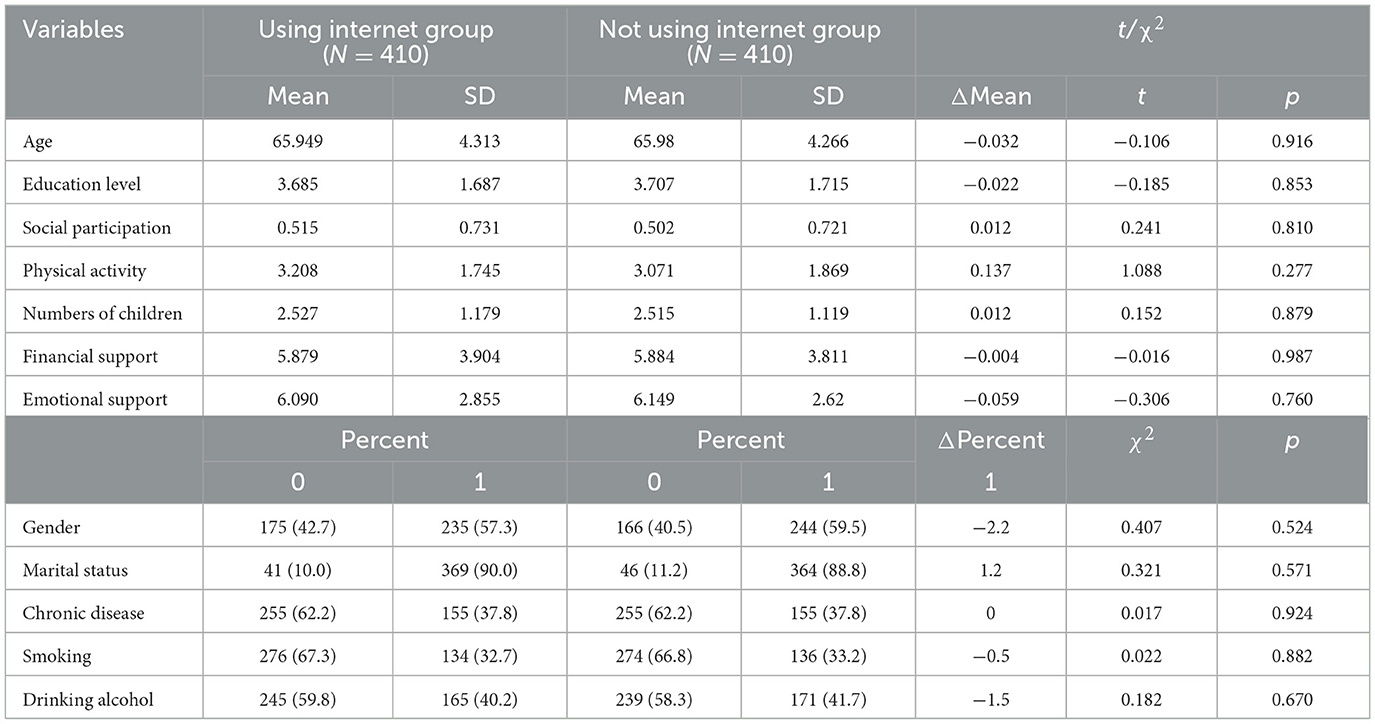
Table 4. Covariates' balancing test between using internet group and not using internet group after matching.
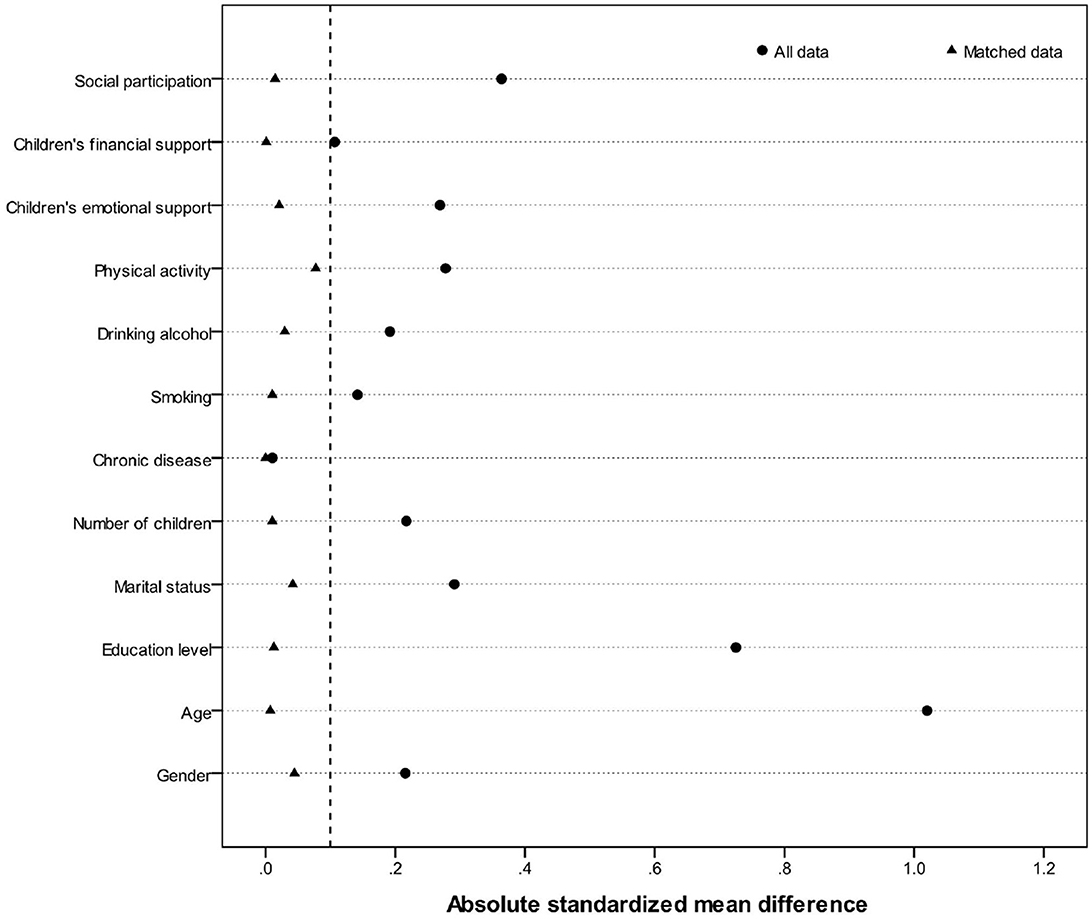
Figure 2. The change in the absolute value of the standard deviation of the covariates before and after the matching of 12 covariates.
3.5 Common support assumption test
The common support hypothesis, as proposed by Rosenbaum and Rubin, posits that the propensity score ranges of groups using the internet and not using the internet should be similar (78). In Figure 3, the distribution of propensity scores for four groups is illustrated. Following the matching process, 44 older adults using the internet and 1,698 older adults not using the internet were excluded, as depicted in the first and fourth columns of Figure 3, respectively. The scatterplots of propensity scores for matched older adults using and not using the internet were nearly identical, as shown in the second column compared to the third column in Figure 3, indicating that the common support assumption was met.
3.6 Average treatment effect on the treated
The bootstrap method was utilized in this study to estimate the average treatment effect on the treated (ATT) and empirical standard error post propensity score matching. The findings indicate that older adults who use the internet exhibited significantly lower levels of depression (p < 0.01) and higher physical health (p < 0.05) compared to older adults who do not use the internet, after controlling for confounding factors through PSM. However, there was no significant difference in cognitive health between the two groups. Specifically, depression levels decreased significantly by 0.795 and physical health improved significantly by 0.433 (refer to Table 5). Overall, internet use was associated with a 0.928% improvement in physical health and an 8.659% reduction in depression levels compared to non-users.
3.7 Robustness test
To verify the robustness of the nearest neighbor matching method (1:1), we used optimal matching, kernel matching using normal density, and radius (caliper = 0.05) to conduct the PSM. The results of three matching methods were consistent (see Table 6), indicating that the estimation results of 1:1 non repetitive nearest neighbor matching were robust.
3.8 Social participation's mediating effect analysis
The results of propensity score matching analysis revealed a positive impact of internet use on both physical and emotional health. To investigate the potential mediating role of social participation in these relationships, separate regression analyses were conducted for physical health (model 1), emotional health (model 2), and social participation (model 3), with other variables serving as covariates. The findings showed that all three regression models were statistically significant. internet use was found to significantly predict emotional health and social participation, but not physical health. On the other hand, social participation was a significant predictor of physical health but not emotional health. Detailed results can be found in Table 7.
To compare the direct and indirect effects of internet use on physical and emotional health, we conducted an analysis using 5,000 bootstrap samples. The indirect effect of internet use on physical health through social participation was found to be significant at a 95% confidence interval (Indirect effect = 0.064, L.L. = 0.024, U.L. = 0.111), while the direct effect of internet use was deemed insignificant (Indirect effect = 0.396, L.L. = −0.010, U.L. = 0.802). Similarly, the indirect effect of internet use on emotional health through social participation was found to be insignificant at a 95% confidence interval (Indirect effect = −0.067, L.L. = −0.168, U.L. = 0.138), whereas the direct effect of internetuse was significant (indirect effect = −0.910, L.L. = −1.623, U.L. = −0.197). Detailed results can be found in Table 8.
4 Discussion
The study delved into the impact of internet use on the emotional, physical, and cognitive health of empty-nest older adults in rural China, with a focus on the mediating role of social participation. Findings indicated that internet use was linked to enhanced emotional and physical health, though not cognitive health. Moreover, social participation was found to partially mediate the relationship between internet use and physical health, but not emotional health.
Several specific findings emerged. Firstly, the findings of this study suggest that empty-nest older adultsusing the internet showed lower levels of depression compared to those not using the internet, after adjusting for confounding factors. This result is consistent with the theory of social interaction, which posits that engaging in social interactions can strengthen relationships and ultimately help reduce depressive symptoms in older adults (80). It is also in line with previous research that has found a positive association between internet use and emotional wellbeing in older adults (29, 81). For example, a study conducted in America found that internet use was associated with a decrease in depressive symptoms among older adults (30).
Secondly, consistent with prior research, this study also highlights the positive impact of Internet use on the physical health of empty-nest older adults in rural China (82). By providing access to health-related information and resources, the internet enables these individuals to enhance their knowledge on disease prevention, nutrition, and exercise, ultimately leading to improvements in their physical wellbeing (83, 84). Additionally, the internet fosters social connections, mitigating feelings of loneliness and isolation commonly experienced by this demographic, thereby benefitting both their mental and physical health (9, 12). It is worth noting that the influence of internet use on older adults' health outcomes may vary based on factors such as digital literacy, the quality of online health information accessed, and socioeconomic status. Hence, while the study corroborates existing literature, it is essential to take these factors into account when interpreting the results.
Thirdly, the study results indicated no significant correlation between internet use and cognitive health in rural Chinese older adults who are empty-nesters. This finding contradicts previous research suggesting a positive link between internet use and cognitive health in older adults (85). For instance, a study in China showed that internet use was linked to enhanced cognitive function in the older adults (86). The discrepancies in these findings may stem from insufficient control over confounding variables in earlier studies. In this study, propensity score matching was used to address sample selection bias and control for confounding factors, possibly explaining the lack of significant results. This underscores the importance of rigorous methodological approaches when examining the relationship between internet use and cognitive health in older adults.
Fourthly, the findings of this study indicate that social participation partially mediates the relationship between internet use and physical health, but not emotional health, among empty-nest older adults in rural China. This aligns with previous research showing a positive link between internet use and social engagement in older populations (56). For instance, a study conducted in the United States demonstrated that internet use was connected to increased social participation among older adults (87). Moreover, past studies have highlighted the positive correlation between social participation and physical health in older individuals (39). For example, a study in China revealed that social engagement was linked to better self-rated health in older adults (88). However, the benefits of internet use on emotional wellbeing can be direct and independent of social participation (89). This suggests that the internet can positively impact emotional health through avenues such as access to information, entertainment, online support groups, and educational resources. These benefits can foster a sense of purpose, self-efficacy, and positive mood, all of which contribute to emotional wellbeing. While social participation remains crucial for emotional health, the positive effects of internet use on emotional wellbeing are not solely dependent on social interactions, indicating the presence of other beneficial mechanisms. Therefore, promoting internet use could be a valuable strategy for enhancing emotional wellbeing, even if social participation is not the primary focus.
Finally, this study has several limitations that should be acknowledged. Firstly, although our study found that internet use can improve the emotional and physical health of rural empty-nest older adults, with social participation acting as a mediator in the relationship between internet use and physical health, the causal validity of this relationship remains to be verified. Our study is cross-sectional in nature, which limits our ability to establish causal relationships between internet use, social participation, and health outcomes. Longitudinal studies are needed to track changes in internet use, social participation, and health over time and to better understand the directionality of these relationships. Secondly, the measures of emotional, physical, and cognitive health used in this study are self-reported, which may be subject to bias. Thirdly, the digital divide remains a significant challenge, particularly in rural areas, and may limit the potential benefits of internet use. While we employed PSM to balance the characteristics of internet users and non-users, the digital divide may still influence the generalizability of our findings. Future research should explore ways to bridge this divide and ensure that the benefits of internet use are accessible to all older adults, regardless of their socio-economic status or geographical location. Furthermore, while we considered a range of variables as covariates, several important factors, such as socio-economic status (SES), were not included in our analysis. Therefore, future research should explicitly incorporate SES and other relevant variables as key covariates to enhance our understanding of their role in the relationship between internet use, social participation, and health outcomes among rural empty-nest older adults. Lastly, our study did not differentiate between types of internet use or consider the frequency and duration of usage, both of which may impact health outcomes differently. Future research should examine these factors more closely to understand how specific online behaviors affect health.
5 Implications
The study's findings carry significant implications for enhancing the wellbeing of empty-nest older adults in rural China. The results indicate that utilizing the internet could positively impact the emotional and physical health of this demographic, with social engagement potentially acting as a mediator in this association. As such, interventions targeting increased internet use and social participation among empty-nest older adults in rural China could prove beneficial for their overall health. It is worth noting, however, that the study's cross-sectional design precludes definitive causal conclusions. Future research employing longitudinal approaches is necessary to establish the causal link between internet use, social participation, and health outcomes in this population.
6 Conclusion
In conclusion, this study suggests that internet use can contribute to improved emotional and physical health among empty-nest older adults in rural China, with social participation playing a mediating role in the relationship between internet use and physical health. Further research is needed to explore the potential mechanisms underlying these associations and to develop targeted interventions aimed at promoting internet use and social participation among this population.
Data availability statement
The original contributions presented in the study are included in the article/supplementary material, further inquiries can be directed to the corresponding authors.
Ethics statement
The data for this study came from the China Health and Retirement Longitudinal Study (CHARLS) was reviewed and approved by the Institute of Social Science Survey (ISSS) of Peking University (approval number: IRB00001052-14010). Respondents are given a statement explaining the purpose of the study and all study participants had signed written informed consent prior to being investigated.
Author contributions
LS: Writing – original draft. YZ: Writing – original draft. MW: Writing – original draft. HP: Writing – original draft. WJ: Writing – original draft. XY: Writing – original draft. WW: Writing – review & editing. LC: Writing – review & editing.
Funding
The author(s) declare financial support was received for the research, authorship, and/or publication of this article. This work was supported by the [National Social Science Foundation of China] under Grant [No.21BSH010]. The funders had no role in study design, data collection and analysis, decision to publish, or preparation of the manuscript.
Acknowledgments
We thank the Institute of Social Science Survey at Peking University for allowing access to the “China Health and Retirement Longitudinal Survey” data.
Conflict of interest
The authors declare that the research was conducted in the absence of any commercial or financial relationships that could be construed as a potential conflict of interest.
Publisher's note
All claims expressed in this article are solely those of the authors and do not necessarily represent those of their affiliated organizations, or those of the publisher, the editors and the reviewers. Any product that may be evaluated in this article, or claim that may be made by its manufacturer, is not guaranteed or endorsed by the publisher.
References
1. Feng Z, Wu B. Embracing challenges for population aging in china: building scientific evidence to inform long-term care policymaking and practice. J Aging Soc Policy. (2023) 35:543–53. doi: 10.1080/08959420.2023.2217979
2. National Bureau of Statistics Main Information of the Seventh National Census Data. (2022). Available at: http://www.stats.gov.cn/tjsj/zxfb/202105/t20210510_1817176.html [Ref list] (accessed May 28, 2022).
3. Chang H, Zhou J, Wang Z. Multidimensional factors affecting successful aging among empty-nesters in china based on social-ecological system theory. Int J Environ Res Public Health. (2022) 19:11885. doi: 10.3390/ijerph191911885
4. Wang G, Hu M, Xiao SY, Zhou L. Loneliness and depression among rural empty-nest elderly adults in Liuyang, China: a cross-sectional study. BMJ Open. (2017) 7:e016091. doi: 10.1136/bmjopen-2017-016091
5. Pickering J, Wister AV, O'Dea E, Chaudhury H. Social isolation and loneliness among older adults living in rural areas during the COVID-19 pandemic: a scoping review. BMC Geriatr. (2023) 23:511. doi: 10.1186/s12877-023-04196-3
6. Hussain B, Mirza M, Baines R, Burns L, Stevens S, Asthana S, et al. Loneliness and social networks of older adults in rural communities: a narrative synthesis systematic review. Front Public Health. (2023) 11:1113864. doi: 10.3389/fpubh.2023.1113864
7. Wu Y, Zhang Q, Huang Y, Qiu S. Seeking medical services among rural empty-nest elderly in China: a qualitative study. BMC Geriatr. (2022) 22:202. doi: 10.1186/s12877-022-02911-0
8. Brandt L, Liu S, Heim C, Heinz A. The effects of social isolation stress and discrimination on mental health. Transl Psychiatry. (2022) 12:398. doi: 10.1038/s41398-022-02178-4
9. Joyce J, Ryan J, Owen A, Hu J, McHugh Power J, Shah R, et al. Social isolation, social support, and loneliness and their relationship with cognitive health and dementia. Int J Geriatr Psychiatry. (2021) 37:5644. doi: 10.1002/gps.5644
10. Gao M, Li Y, Zhang S, Gu L, Zhang J, Li Z, et al. Does an empty nest affect elders' health? Empirical evidence from China. Int J Environ Res Public Health. (2017) 14:463. doi: 10.3390/ijerph14050463
11. Hao X, Li M, Li J, Lv M, Qin Y, Li K. Sleep quality in relation to social support and resilience among rural empty-nest older adults in China. Sleep Med. (2021) 82:193-199. doi: 10.1016/j.sleep.2021.03.026
12. Holt-Lunstad J. Loneliness and social isolation as risk factors: the power of social connection in prevention. Am J Lifestyle Med. (2021) 15:567–73. doi: 10.1177/15598276211009454
13. Wolters NE, Mobach L, Wuthrich VM, Vonk P, Van der Heijde CM, Wiers RW, et al. Emotional and social loneliness and their unique links with social isolation, depression and anxiety. J Affect Disord. (2023) 329:207–17. doi: 10.1016/j.jad.2023.02.096
14. Zhang HH, Jiang YY, Rao WW, Zhang QE, Qin MZ, Ng CH, et al. Prevalence of depression among empty-nest elderly in china: a meta-analysis of observational studies. Front Psychiatry. (2020) 11:608. doi: 10.3389/fpsyt.2020.00608
15. Song C, Yao L, Chen H, Song Y, Liu L. Prevalence and factors influencing depression among empty nesters in China: a meta-analysis. BMC Geriatr. (2023) 23:333. doi: 10.1186/s12877-023-04064-0
16. Guarnera J, Yuen E, Macpherson H. The impact of loneliness and social isolation on cognitive aging: a narrative review. J Alzheimers Dis Rep. (2023) 7:699–714. doi: 10.3233/adr-230011
17. Cardona M, Andrés P. Are social isolation and loneliness associated with cognitive decline in ageing? Front Aging Neurosci. (2023) 15:1075563. doi: 10.3389/fnagi.2023.1075563
18. Deng Y, Zhao S, Cheng G, Yang J, Li B, Xu K, et al. The prevalence of mild cognitive impairment among chinese people: a meta-analysis. Neuroepidemiology. (2021) 55:79–91. doi: 10.1159/000512597
19. Zhang M, Ma Y, Xie X, Sun M, Huang Z, Zhao Z, et al. Trends in insufficient physical activity among adults in China 2010-18: a population-based study. Int J Behav Nutr Phys Act. (2023) 20:87. doi: 10.1186/s12966-023-01470-w
20. Bai X, Soh KG, Omar Dev RD, Talib O, Xiao W, Soh KL, et al. Aerobic exercise combination intervention to improve physical performance among the elderly: a systematic review. Front Physiol. (2021) 12:798068. doi: 10.3389/fphys.2021.798068
21. Adams M, Gordt-Oesterwind K, Bongartz M, Zimmermann S, Seide S, Braun V, et al. Effects of physical activity interventions on strength, balance and falls in middle-aged adults: a systematic review and meta-analysis. Sports Med Open. (2023) 9:61. doi: 10.1186/s40798-023-00606-3
22. Wu H, Ba N, Ren S, Xu L, Chai J, Irfan M, et al. The impact of internet development on the health of Chinese residents: transmission mechanisms and empirical tests. Socioecon Plann Sci. (2022) 81:101178. doi: 10.1016/j.seps.2021.101178
23. Zhang C. Smartphones and telemedicine for older people in China: Opportunities and challenges. Digit Health. (2022) 8:20552076221133695. doi: 10.1177/20552076221133695
24. China Daily. (2023). Available at: https://www.chinadaily.com.cn/a/202302/14/WS63eaef79a31057c47ebaea90.html (accessed February 02, 2023).
25. Center CINI. The 49th Statistical Report on China's Internet Development. Beijing: China Internet Network Information Center. (2022).
26. Peng Z, Dan T. Digital dividend or digital divide? Digital economy and urban-rural income inequality in China. Telecommun Policy. (2023) 47:102616. doi: 10.1016/j.telpol.2023.102616
27. Hupcey JE. Clarifying the social support theory-research linkage. J Adv Nurs. (1998) 27:1231–41. doi: 10.1046/j.1365-2648.1998.01231.x
28. Khalaila R, Vitman-Schorr A. Internet use, social networks, loneliness, and quality of life among adults aged 50 and older: mediating and moderating effects. Qual Life Res. (2018) 27:479–89. doi: 10.1007/s11136-017-1749-4
29. Zhang H, Wang H, Yan H, Wang X. Impact of internet use on mental health among elderly individuals: a difference-in-differences study based on 2016-2018 CFPS data. Int J Environ Res Public Health. (2021) 19:101. doi: 10.3390/ijerph19010101
30. Cotten SR, Ford G, Ford S, Hale TM. Internet use and depression among older adults. Comput Human Behav. (2012) 28:496−9. doi: 10.1016/j.chb.2011.10.021
31. Li Y, Bai X, Chen H. Social isolation, cognitive function, and depression among chinese older adults: examining internet use as a predictor and a moderator. Front Public Health. (2022) 10:809713. doi: 10.3389/fpubh.2022.809713
32. Duplaga M. The association between Internet use and health-related outcomes in older adults and the elderly: a cross-sectional study. BMC Med Inform Decis Mak. (2021) 21:150. doi: 10.1186/s12911-021-01500-2
33. Forsman AK, Nordmyr J. Psychosocial links between internet use and mental health in later life: a systematic review of quantitative and qualitative evidence. J Appl Gerontol. (2017) 36:1471–518. doi: 10.1177/0733464815595509
34. Bessière K, Pressman S, Kiesler S, Kraut R. Effects of internet use on health and depression: a longitudinal study. J Med Internet Res. (2010) 12:e6. doi: 10.2196/jmir.1149
35. Wen W, Zhang Y, Shi W, Li J. Association between internet use and physical health, mental health, and subjective health in middle-aged and older adults: nationally representative cross-sectional survey in China. J Med Internet Res. (2023) 25:e40956. doi: 10.2196/40956
36. Huang R, Gong R, Deng Q, Hu Y. The effect of intergenerational support from children on loneliness among older adults-the moderating effect of internet usage and intergenerational distance. Front Public Health. (2024) 12:1330617. doi: 10.3389/fpubh.2024.1330617
37. Kwon DM, Santiago-Torres M, Mull KE, Sullivan BM, Bricker JB. Older adults who smoke: Do they engage with and benefit from web-based smoking cessation interventions? Preventive Medicine. (2022) 161:107118. doi: 10.1016/j.ypmed.2022.107118
38. Yang HL, Zhang S, Zhang SQ, Xie L, Wu YY, Yao YD, et al. Internet use and depressive symptoms among older adults in China. Front Psychiatry. (2021) 12:739085. doi: 10.3389/fpsyt.2021.739085
39. Dawson-Townsend K. Social participation patterns and their associations with health and well-being for older adults. SSM Popul Health. (2019) 8:100424. doi: 10.1016/j.ssmph.2019.100424
40. Levasseur M, Richard L, Gauvin L, Raymond E. Inventory and analysis of definitions of social participation found in the aging literature: proposed taxonomy of social activities. Soc Sci Med. (2010) 71:2141–9. doi: 10.1016/j.socscimed.2010.09.041
41. Michalski CA, Diemert LM, Helliwell JF, Goel V, Rosella LC. Relationship between sense of community belonging and self-rated health across life stages. SSM Popul Health. (2020) 12:100676. doi: 10.1016/j.ssmph.2020.100676
42. Löfgren M, Larsson E, Isaksson G, Nyman A. Older adults' experiences of maintaining social participation: creating opportunities and striving to adapt to changing situations. Scand J Occup Ther. (2022) 29:587–97. doi: 10.1080/11038128.2021.1974550
43. DiClemente RJ, Crosby RA, Kegler MC. Emerging Theories in Health Promotion Practice and Research: Strategies for Improving Public Health, Vol. 1. San Francisco, CA: John Wiley & Sons (2002).
44. Pénard T, Poussing N. Internet use and social capital: the strength of virtual ties. J Econ Issues. (2010) 44:569–95. doi: 10.2753/JEI0021-3624440301
45. Wang X, Guo J, Liu H, Zhao T, Li H, Wang T. Impact of social participation types on depression in the elderly in china: an analysis based on counterfactual causal inference. Front Public Health. (2022) 10:792765. doi: 10.3389/fpubh.2022.792765
46. Choi E, Han KM, Chang J, Lee YJ, Choi KW, Han C, et al. Social participation and depressive symptoms in community-dwelling older adults: emotional social support as a mediator. J Psychiatr Res. (2021) 137:589–96. doi: 10.1016/j.jpsychires.2020.10.043
47. Lv R, Yang L, Li J, Wei X, Ren Y, Wang W, et al. Relationship between social participation and life satisfaction in community-dwelling older adults: multiple mediating roles of depression and cognitive function. Arch Gerontol Geriatr. (2024) 117:105233. doi: 10.1016/j.archger.2023.105233
48. Zhai Y, Zhang T, Li Q, Lin J, Wang X, Li F, et al. Association of empty nest and group activity with cognitive impairment in Chinese older adults: a cross-sectional study. Arch Gerontol Geriatr. (2020) 90:104120. doi: 10.1016/j.archger.2020.104120
49. Gao Y, Chen L, Jia Z, Zhao L, Yang Y, Liu C. Social participation and health in middle-aged and older empty nesters: a study on gender differences. SSM Popul Health. (2024) 25:101641. doi: 10.1016/j.ssmph.2024.101641
50. Chen J, Zeng Y, Fang Y. Effects of social participation patterns and living arrangement on mental health of Chinese older adults: a latent class analysis. Front Public Health. (2022) 10:915541. doi: 10.3389/fpubh.2022.915541
51. Larsson E, Padyab M, Larsson-Lund M, Nilsson I. Effects of a social internet-based intervention programme for older adults: an explorative randomised crossover study. Br J Occup Therapy. (2016) 79:629–36. doi: 10.1177/0308022616641
52. Suragarn U, Hain D, Pfaff G. Approaches to enhance social connection in older adults: an integrative review of literature. Aging Health Res. (2021) 1:100029. doi: 10.1016/J.AHR.2021.100029
53. Glass TA, de Leon CM, Marottoli RA, Berkman LF. Population based study of social and productive activities as predictors of survival among elderly. Americans BMJ. (1999) 319:478–83. doi: 10.1136/bmj.319.7208.478
54. Li P, Zhang C, Gao S, Zhang Y, Liang X, Wang C, et al. Association between daily internet use and incidence of chronic diseases among older adults: prospective cohort study. J Med Internet Res. (2023) 25:e46298. doi: 10.2196/46298
55. Wang J, Liang C, Li K. Impact of internet use on elderly health: empirical study based on Chinese General Social Survey (CGSS) data. Healthcare (Basel). (2020) 8:482. doi: 10.3390/healthcare8040482
56. Hou B, Li Y, Wang H. Internet use and health status among older adults: the mediating role of social participation. Front Public Health. (2022) 10:1072398. doi: 10.3389/fpubh.2022.1072398
57. Nakagomi A, Shiba K, Kawachi I, Ide K, Nagamine Y, Kondo N, et al. Internet use and subsequent health and well-being in older adults: an outcome-wide analysis. Comput Human Behav. (2022) 130:107156. doi: 10.1016/j.chb.2021.107156
58. Shi J-g, Liu M, Fu G, Dai X. Internet use among older adults: determinants of usage and impacts on individuals' well-being. Comp Human Behav. (2023) 139:107538. doi: 10.1016/j.chb.2022.107538
59. Cotten SR, Anderson WA, McCullough BM. Impact of internet use on loneliness and contact with others among older adults: cross-sectional analysis. J Med Internet Res. (2013) 15:e39. doi: 10.2196/jmir.2306
60. Shapira N, Barak A, Gal I, Gal I. Promoting older adults' well-being through Internet training and use. Aging Ment Health. (2007) 11:477–84. doi: 10.1080/13607860601086546
61. Zhao Y, Hu Y, Smith JP, Strauss J, Yang G. Cohort profile: the China Health and Retirement Longitudinal Study (CHARLS). Int J Epidemiol. (2014) 43:61–8. doi: 10.1093/ije/dys203
62. Andresen EM, Malmgren JA, Carter WB, Patrick DL. Screening for depression in well older adults: evaluation of a short form of the CES-D (Center for Epidemiologic Studies Depression Scale). Am J Prev Med. (1994) 10:77–84.
63. Zhang J, Sun W, Kong Y, Wang C. Reliability and validity of the Center for Epidemiological Studies Depression Scale in 2 special adult samples from rural China. Compr Psychiatry. (2012) 53:1243–51. doi: 10.1016/j.comppsych.2012.03.015
64. Huang Q, Wang X, Chen G. Reliability and validity of 10-item CES-D among middle aged and older adults in China. Chin J Health Psychol. (2015) 7:1036–41. doi: 10.1016/j.comppsych.2012.03.015
65. Boey KW. Cross-validation of a short form of the CES-D in Chinese elderly. Int J Geriatr Psychiatry. (1999) 14:608–17. doi: 10.1002/(sici)1099-1166(199908)14:8<608::aid-gps991>3.0.co;2-z
66. Chan S, Jia S, Chiu H, Chien WT., D RT, Hu Y, et al. Subjective health-related quality of life of Chinese older persons with depression in Shanghai and Hong Kong: relationship to clinical factors, level of functioning and social support. Int J Geriatr Psychiatry. (2009) 24:355–62. doi: 10.1002/gps.2129
67. Tong AY, Man DW. The validation of the Hong Kong Chinese version of the Lawton Instrumental Activities of Daily Living Scale for institutionalized elderly persons. OTJR: Occup Part Health. (2002) 22:132–42. doi: 10.1177/153944920202200402
68. Liu H, Ma Y, Lin L, Sun Z, Li Z, Jiang X. Association between activities of daily living and depressive symptoms among older adults in China: evidence from the CHARLS. Front Public Health. (2023) 11:1249208. doi: 10.3389/fpubh.2023.1249208
69. Katz S, Ford AB, Moskowitz RW, Jackson BA, Jaffe MW. Studies of illness in the aged: the index of ADL: a standardized measure of biological and psychosocial function. JAMA. (1963) 185:914–9. doi: 10.1001/jama.1963.03060120024016
70. Folstein MF, Folstein SE, McHugh PR. “Mini-mental state”. A practical method for grading the cognitive state of patients for the clinician. J Psychiatr Res. (1975) 12:189–98. doi: 10.1016/0022-3956(75)90026-6
71. Pan X, Luo Y, Roberts AR. Secondhand smoke and women's cognitive function in China. Am J Epidemiol. (2018) 187:911–8. doi: 10.1093/aje/kwx377
72. Wang T, Wu Y, Sun Y, Zhai L, Zhang D. A prospective study on the association between uric acid and cognitive function among middle-aged and older Chinese. J Alzheimers Dis. (2017) 58:79–86. doi: 10.3233/jad-161243
73. O'Connor DW, Pollitt PA, Hyde JB, Fellows JL, Miller ND, Brook CPB, et al. The reliability and validity of the mini-mental state in a British community survey. J Psychiatr Res. (1989) 23:87–96. doi: 10.1016/0022-3956(89)90021-6
74. Li H, Li C, Wang A, Qi Y, Feng W, Hou C, et al. Associations between social and intellectual activities with cognitive trajectories in Chinese middle-aged and older adults: a nationally representative cohort study. Alzheimers Res Ther. (2020) 12:115. doi: 10.1186/s13195-020-00691-6
75. Yang X, Pan A, Gong J, Wen Y, Ye Y, Wu JH, et al. Prospective associations between depressive symptoms and cognitive functions in middle-aged and elderly Chinese adults. J Affect Disord. (2020) 263:692–7. doi: 10.1016/j.jad.2019.11.048
76. Cohn-Schwartz E. Pathways from social activities to cognitive functioning: the role of physical activity and mental health. Innov Aging. (2020) 4:igaa015. doi: 10.1093/geroni/igaa015
77. Silver IA, Wooldredge J, Sullivan CJ, Nedelec JL. Longitudinal propensity score matching: A demonstration of counterfactual conditions adjusted for longitudinal clustering. J Quant Criminol. (2021) 37:267–301. doi: 10.1007/s10940-020-09455-9
78. Rosenbaum PR, Rubin DB. The central role of the propensity score in observational studies for causal effects. Biometrika. (1983) 70:41–55. doi: 10.1093/BIOMET/70.1.41
79. Austin PC. An introduction to propensity score methods for reducing the effects of confounding in observational studies. Multivariate Behav Res. (2011) 46:399–424. doi: 10.1080/00273171.2011.568786
80. Mackenzie CS, Abdulrazaq S. Social engagement mediates the relationship between participation in social activities and psychological distress among older adults. Aging Ment Health. (2021) 25:299–305. doi: 10.1080/13607863.2019.1697200
81. Nan Y, Xie Y, Hu Y. Internet use and depression among Chinese older adults: The mediating effect of interpersonal relationship. Front Public Health. (2023) 11:1102773. doi: 10.3389/fpubh.2023.1102773
82. Guo E, Li J, Luo L, Gao Y, Wang Z. The effect and mechanism of Internet use on the physical health of the older people-Empirical analysis based on CFPS. Front Public Health. (2022) 10:952858. doi: 10.3389/fpubh.2022.952858
83. Luo X, Pu H, Wang S, Zhong D, Liu F, Li Z. Influence of Internet use on Chinese residents' health: the mediating role of health knowledge. Technol Soc. (2024) 76:102413. doi: 10.1016/j.techsoc.2023.102413
84. Fitzpatrick PJ. Improving health literacy using the power of digital communications to achieve better health outcomes for patients and practitioners. Front Digit Health. (2023) 5:1264780. doi: 10.3389/fdgth.2023.1264780
85. Yu X, Mu A, Wu X, Zhou L. Impact of internet use on cognitive decline in middle-aged and older adults in China: longitudinal observational study. J Med Internet Res. (2022) 24:e25760. doi: 10.2196/25760
86. Yu D, Fiebig DG. Internet use and cognition among middle-aged and older adults in China: a cross-lagged panel analysis. J Econ Ageing. (2020) 17:100262. doi: 10.1016/j.jeoa.2020.100262
87. Ang S. Internet use and cohort change in social connectedness among older adults. Adv Life Course Res. (2022) 54:100514. doi: 10.1016/j.alcr.2022.100514
88. Yuan Y, Peng C, Lin Y, Qu S. Older adults' social participation and social relationship and association with partner's cognition: a latent profile analysis. China Popul Dev Stud. (2023) 7:418–37. doi: 10.1007/s42379-023-00148-x
89. Duplaga M, Szulc K. The association of internet use with wellbeing, mental health and health behaviours of persons with disabilities. Int J Environ Res Public Health. (2019) 16:3252. doi: 10.3390/ijerph16183252
90. Chopik WJ. The benefits of social technology use among older adults are mediated by reduced loneliness. Cyberpsychol Behav Soc Netw. (2016) 19:551–6. doi: 10.1089/cyber.2016.0151
Keywords: older adults, internet use, physical health, cognitive health, depression, social participation, propensity score matching
Citation: Shen L, Zheng Y, Wang M, Pan H, Jian W, Yang X, Wang W and Chen L (2024) Does internet use promote the health of empty-nest older adults in rural China? The mediating role of social participation using a propensity score matching approach. Front. Public Health 12:1436525. doi: 10.3389/fpubh.2024.1436525
Received: 22 May 2024; Accepted: 05 November 2024;
Published: 22 November 2024.
Edited by:
Ted K. S. Ng, Rush University Medical Center, United StatesReviewed by:
Yaolin Pei, New York University, United StatesChong Zhang, University of Electronic Science and Technology of China, China
Copyright © 2024 Shen, Zheng, Wang, Pan, Jian, Yang, Wang and Chen. This is an open-access article distributed under the terms of the Creative Commons Attribution License (CC BY). The use, distribution or reproduction in other forums is permitted, provided the original author(s) and the copyright owner(s) are credited and that the original publication in this journal is cited, in accordance with accepted academic practice. No use, distribution or reproduction is permitted which does not comply with these terms.
*Correspondence: Wei Wang, d2FuZ3dlaUB3bXUuZWR1LmNu; Li Chen, cHN5Y2hvbG9neWNoZW5saUAxNjMuY29t
†These authors have contributed equally to this work