- 1China Basketball College, Beijing Sport University, Beijing, China
- 2School of Strength and Conditioning, Beijing Sport University, Beijing, China
- 3Sport Science School, Beijing Sport University, Beijing, China
- 4China Institute of Sports and Health Science, Beijing Sport University, Beijing, China
- 5Department of Physical Education, Jiangsu Normal University, Xuzhou, China
Background: It has been shown that diabetes is associated with insufficient physical activity among middle-aged and older adults, but the association between different physical activity levels (PAL) and diabetes incidence needs to be further explored.
Objective: This study aims to explore the correlation and dose–response relationship between different PAL and the diabetes incidence in middle-aged and older adults.
Methods: Utilizing data from the 2018 China Health and Retirement Longitudinal Study (CHARLS), this cross-sectional analysis included 17,226 middle-aged and older adults aged 45 and above. Binary logistic regression models and restricted cubic spline (RCS) were used to explore the correlation and dose–response relationship between different PAL and the incidence of diabetes in the total middle-aged and older adults population as well as in subgroups. Sensitivity analyses were also performed to verify the robustness of the findings.
Results: In the entire study population, compared with the lowest PAL, participants in the third and fourth quartiles PAL saw diabetes incidence significantly reduced by 16% (p = 0.005) and 33% (p < 0.001), respectively (pfor trend < 0.001). In subgroup analyses, the fourth quartile PAL significantly reduced the diabetes incidence among females, individuals aged 60–69, and rural residents by 25% (p = 0.011), 38% (p < 0.001) and 28% (p < 0.001), respectively. For males, middle-aged (45–59 years), and urban residents, the third quartile PAL reduced diabetes incidence by 22% (p = 0.004), 24% (p = 0.012), 21% (p = 0.013), respectively. When the fourth quartile PAL was reached, the diabetes incidence was significantly reduced in these populations by 41% (p < 0.001), 39% (p < 0.001), and 41% (p < 0.001), respectively. There was a negative dose–response relationship between physical activity and diabetes incidence in specific Chinese middle-aged and older adults population. In addition, sensitivity analyses indicated the robustness of the findings.
Conclusion: Higher PAL was associated with lower diabetes incidence in specific Chinese middle-aged and older adults population. It is feasible to use physical activity to predict diabetes incidence in this demographic, and high PAL may be an effective means of preventing and controlling diabetes.
1 Introduction
Diabetes is one of the leading causes of death worldwide, with an estimated 4.2 million deaths in 2019 (1). By 2030, global diabetes diagnoses are projected to reach 578 million, surging to an anticipated 700 million by 2045 (2). Diabetes has a high prevalence in the middle-aged and older adults population (3, 4) and exert a huge impact on the health of them; it is a contributing factor to a number of diseases such as myocardial infarction, dementia, and depression (5–7), and can lead to a variety of complications such as fractures, falls, and pain (8–11), which not only leads to their moodiness and irritability, and can even lead to frailty, disability and suicide. Additionally, population aging is a problem faced by the whole world, especially China. Currently, population aging in China is intensifying, and it is expected that by 2050, China’s older adults population over 65 years old will reach 400 million, of which 150 million will be over 80 years old (12, 13). As one of the fastest aging countries in the world, the number of older adults people with diabetes will continue to grow in China. This will not only seriously affect people’s quality of life, but also bring a heavy economic burden to the affected families and society (14). Consequently, how to prevent and control diabetes in middle-aged and older adults people in a healthy and effective way has become a hot topic in the field of public health.
Research indicates that insufficient physical activity contributes to diabetes and its complications among middle-aged and older adults individuals, while regular physical activity can prevent diabetes in this demographic (15–17). Physical activity, defined as any movement that expends energy through musculoskeletal contraction and relaxation, encompasses all daily physical movements, and it is a broad concept that includes more specific parameters such as type, intensity, duration and frequency (18). While some research has identified a negative association between physical activity and diabetes incidence (19), other studies suggest that moderate physical activity level (PAL) reduces diabetes incidence more effectively than other levels (20). Currently, the association between the protective effect of physical activity on the incidence of diabetes in middle-aged and older adults people has been demonstrated. However, the association between different PAL and diabetes incidence remains unclear, especially given the limited studies within this demographic in China. Moreover, it has been shown that the prevalence of diabetes is largely influenced by age, gender, and residency (21), but it is also unclear whether there are age, gender, and residency differences in the association between physical activity and diabetes incidence.
Further research is needed to investigate the association between varying PAL and diabetes incidence in Chinese middle-aged and older adults populations. This study aimed to explore the correlation and dose–response relationship between various PAL and diabetes incidence, assessing differences by age, gender, and residency. Exploring this relationship may facilitate early prediction of diabetes and inform effective interventions. Therefore, this study investigated the association between different PAL and the diabetes incidence in the middle-aged and older adults population and the differences in gender, age, and residency, thus providing a scientific basis for the prediction and treatment of diabetes in this groups.
2 Methods
2.1 Data sources and participants
This is a cross-sectional study based on data from the 2018 China Health and Retirement Longitudinal Study (CHARLS). The survey is a nationally representative community-based demographic survey of middle-aged and older adults people in China, conducted by the China National Development Research Institute (CNDI) in collaboration with the China Social Science Research Centre (CSRC) of Peking University (22). Employing a stratified, multi-stage,probability sampling method proportional to population size, the survey sampled individuals aged 45 and above from 150 counties and 450 communities (villages) across 28 Chinese provinces (autonomous regions and municipalities). It collected demographic, economic, pension, and health data The CHARLS database has good reliability and validity, accurately representing China’s middle-aged and older adults populations, and it has been used by many scholars to conduct health-related research in recent years (23). Additionally, Ethical approval for CHARLS was granted by Peking University’s Biomedical Ethics Review Committee (Approval No.: IRB00001052-11015) (22). Since the data is publicly available, no further ethical approval was necessary for this study.
According to the definition of middle-aged and older adults in China, respondents aged 45 years and above were included as the research subjects. Eligible participants must have complete data on physical activity, diabetes status, and relevant covariates. Therefore, respondents under 45 or with missing data in these areas were excluded. Initially, 19,750 adults aged 45 and older were selected. After excluding 2,524 for age non-compliance or missing relevant data, 17,226 participants remained who met the inclusion criteria.
2.2 Physical activity assessment
The CHARLS database collected the number of days the respondents were physically active for at least 10 min per day in the past week and the duration of daily activity, and physical activity was assessed using the International Physical Activity Questionnaire-Short Form (IPAQ-SF) (18). Metabolic equivalents (METs) for each physical activity were assigned using the IPAQ, a widely used tool for measuring PAL in adults with demonstrated reliability and validity (18). This method estimated the total weekly energy expenditure of the respondents’ physical activities. MET values of 3.3, 4.0, and 8.0 were, respectively, assigned for low, moderate, and high intensity activities. Total weekly energy expenditure was calculated using the formula: MET × activity time per day (min) × active days per week(d) (24). Physical activity levels were categorized into quartiles for analysis as follows: Q1 ≤ 1732.5 MET/week, 1732.5 < Q2 ≤ 4612.5 MET/week, 4612.5 < Q3 ≤ 10,458 MET/week, Q4 > 10,458 MET/week.
2.3 Diabetes assessment
Diabetes assessment was based on self-reported physician diagnosis, high fasting blood glucose levels (fasting blood glucose ≥126 mg/dL or glycated hemoglobin (HbA1c) ≥6.5%) (25), or ongoing antidiabetic treatment. Participants were classified as diabetic based on a positive response to the diabetes diagnosis question.
2.4 Covariates
This study’s covariates, selected for their potential impact on diabetes incidence, included age, gender, residency (urban or rural), education level (illiteracy, primary, secondary, and high school and above), health behaviors (alcohol and smoking status), daily living ability, and comorbidities such as hypertension, heart disease, and depression. Depression was assessed using the Center for Epidemiologic Studies Depression Scale (CESD), which consists of 10 entries with 4 answers to each question. The respondent scores each symptom according to the frequency of occurrence of each symptom in the past week, with total scores ranging from 0 to 30, with scores <10 defined as no depression and score ≥ 10 defined as depression (26). The Chinese version of the CESD has been used in the Chinese older adults population, with good reliability and validity, and can effectively measure depression level of middle-aged and older adults people (27).
2.5 Data analysis
In this study, data were collated and analyzed using R 4.0.3 software. Measurement data were expressed as mean ± standard deviation (mean ± SD), count data as percentages, categorical variables as frequencies and percentages. Group comparisons were made using the χ2 test or Fisher’s exact test. Binary logistic regression was employed to analyze the relationship between different PAL and diabetes incidence in the middle-aged and older adults population. Model 1 was not adjusted for any variables, model 2 was adjusted for demographic characteristics (age, gender, residency, education level) and health behaviors (smoking status and alcohol status), and model 3 was adjusted for demographic characteristics, health behaviors, daily living ability, and comorbidities (hypertension, heart disease, depression). Subgroup analyses of sex, age and residency were also conducted to explore differences across subgroups. Restricted cubic spline (RCS) was used to assess the dose–response relationship between physical activity and diabetes incidence in the total middle-aged and older adults population and within each subgroup. In addition, sensitivity analyses were used to validate the results for robustness. Results were expressed as odds ratios (ORs) and 95% confidence intervals (CIs), and differences were considered statistically significant when the p value was less than 0.05.
3 Results
3.1 Basic information of the participants
Table 1 shows baseline characteristics for 17,226 middle-aged and older adults participants, with an average age of 61.28 ± 9.71 years. Of these, 8,916 (51.76%) were female and 8,310 (48.24%) were male. There were 7,885 (45.77%) aged 45–59 years, 5,896 (34.23%) aged 60–69 years and 3,445 (20%) aged 70 years and above. Among participants, 2,223 (12.90%) were diagnosed with diabetes, accounting for 12.90% of the total number of people, and the diabetes prevalence is increasing with age. The correlation between diabetes incidence and age, sex, residency, education level, smoking status, drinking status, ability of daily living, hypertension, heart disease, depression, and PAL among the middle-aged and older adults were statistically significant (p < 0.05).
3.2 Binary logistic regression analysis of physical activity and diabetes
This study used diabetes status as the dependent variable and physical activity as the independent variable, incorporating additional covariates in the regression model to assess their relationship. Results are presented in Table 2. The results showed that compared to those in the lowest quartile PAL, participants in the second, third, and fourth quartiles had diabetes prevalence reduced by 16% [OR = 0.84, 95% CI (0.74, 0.94), p = 0.003], 31% [OR = 0.69, 95% CI (0.61, 0.78), p < 0.001], and 53% [OR = 0.47, 95% CI (0.41, 0.53), p < 0.001], respectively. After adjusting for sex, age, residency, education level, smoking status, drinking status, daily living ability, hypertension, heart disease, and depression, participants in the third and fourth quartiles PAL saw diabetes incidence reduced by 16% [OR = 0.84, 95% CI (0.74, 0.95), p = 0.005] and 33% [OR = 0.67, 95% CI (0.58, 0.77), p < 0.001], respectively, compared to the first quartile. No significant incidence reduction was observed in the second quartile.
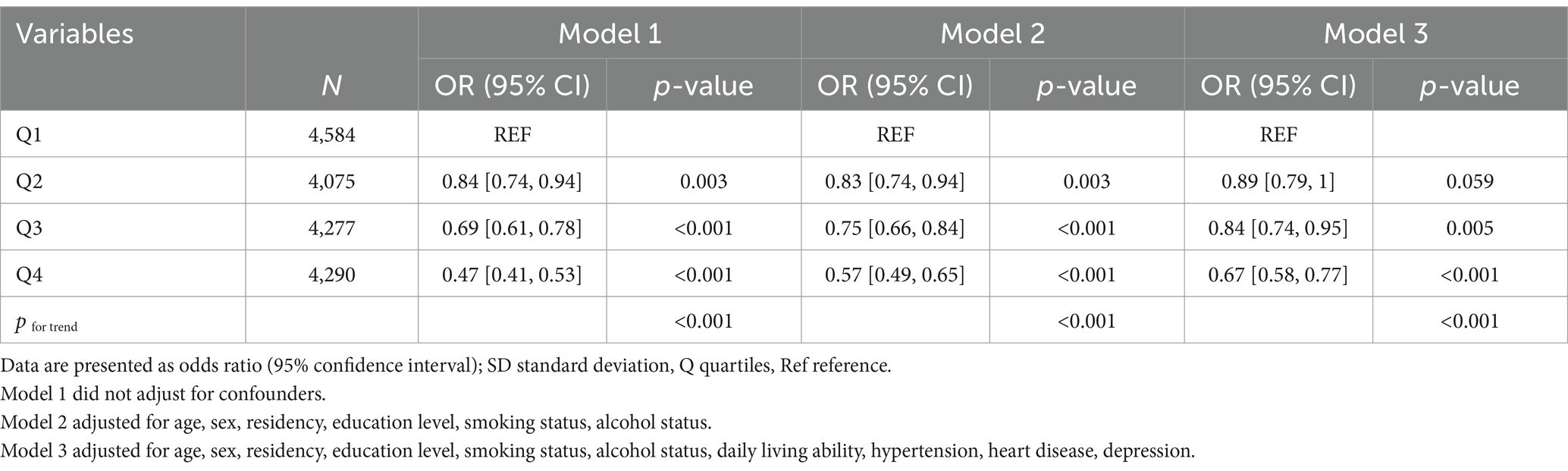
Table 2. Binary logistic regression analysis for the associations between physical activity and diabetes.
In this study, physical activity was introduced as a continuous variable in restricted cubic spline (RCS) curve fitting, and the RCS curve showed a negatively correlated dose–response relationship between physical activity and the diabetes incidence in the middle-aged and older adults population (see Figure 1).
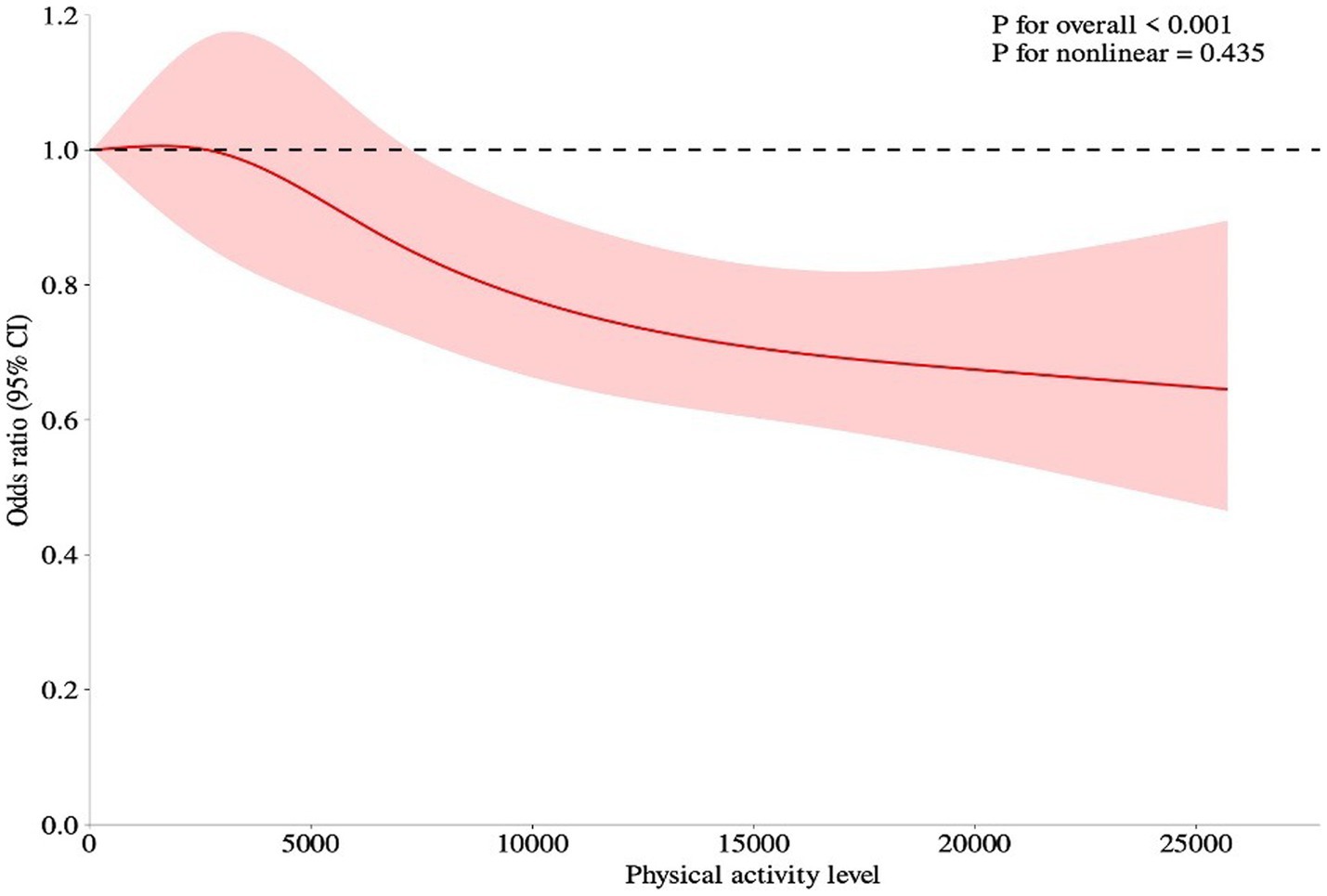
Figure 1. Dose–response relationship between physical activity and the incidence of diabetes in middle-aged and older adults people. The RCS curves were adjusted for age, sex, residency, education level, smoking status, drinking status, daily living ability, hypertension, heart disease, and depression. The solid red lines represented the ORs of diabetes, the red region indicated corresponding 95% CIs. The short dashed black lines indicated the reference value.
3.3 Subgroup analyses
This study conducted subgroup analyses to examine the impact of sex, age, and residency on diabetes incidence among middle-aged and older adults individuals. Age was also categorized into three groups: middle-aged (45–59), early older adults (60–69), and late older adults (70+) to investigate the relationship between physical activity and diabetes incidence in different age intervals. Results are presented in Table 3. Additionally, the RCS analysis was applied to explore the dose–response relationship between physical activity and diabetes incidence within various subgroups. The results are detailed in Supplementary Figures S1–S7.
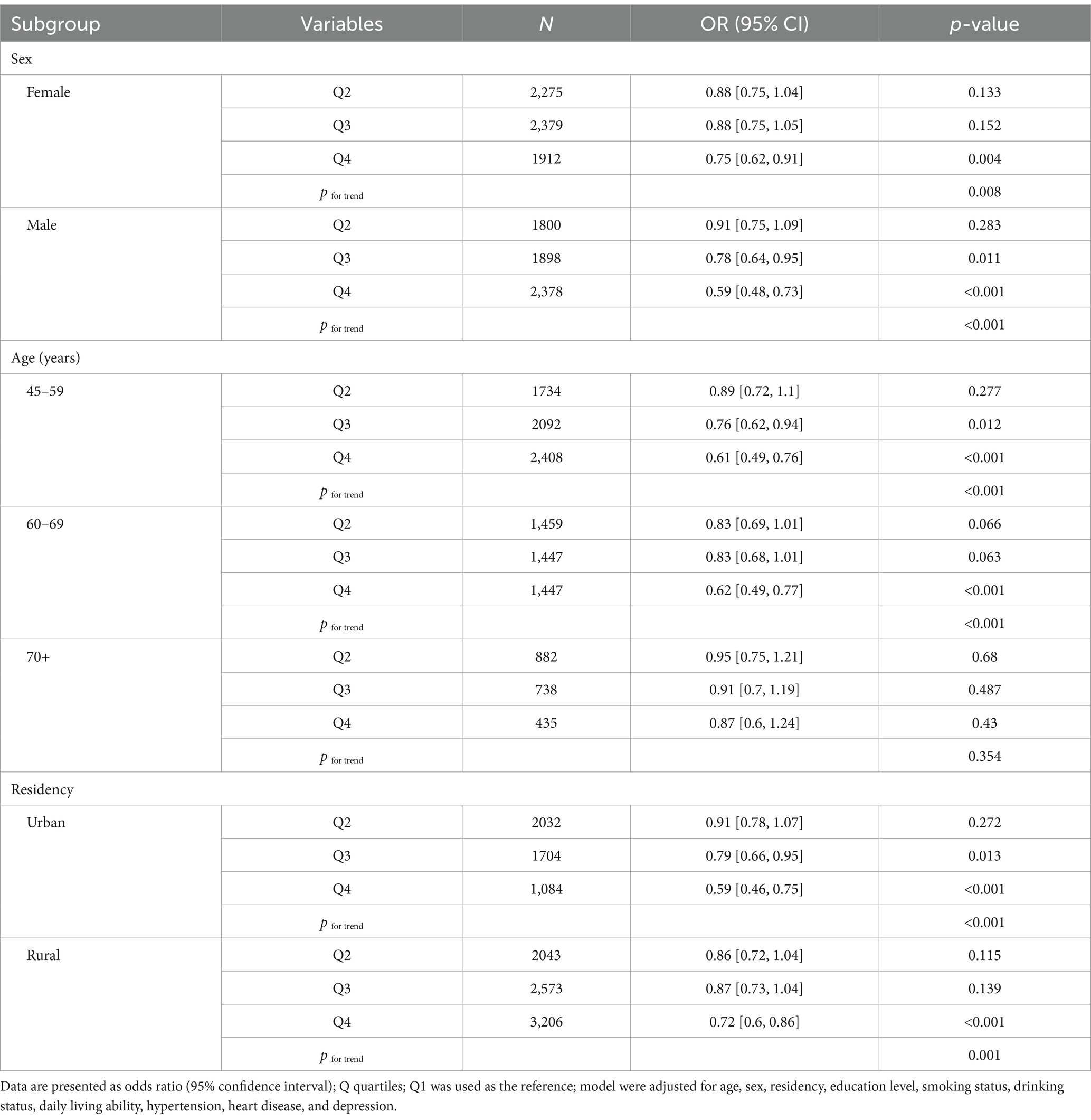
Table 3. Binary logistic analysis for the association between physical activity and diabetes prevalence in various subgroups.
After adjusting for confounders, compared with the first quartile PAL, the fourth quartile PAL significantly reduced the diabetes incidence among females, individuals aged 60–69 and rural residents by 25% [OR = 0.75, 95% CI (0.62, 0.91), p = 0.011], 38% [OR = 0.62, 95% CI (0.49, 0.77), p < 0.001] and 28% [OR = 0.72, 95% CI (0.6, 0.86), p < 0.001], respectively. Whereas, for males, middle-aged (45–59 years), and urban residents, the third quartile PAL reduced diabetes incidence by 22% [OR = 0.78, 95% CI (0.64, 0.95), p = 0.004], 24% [OR = 0.76, 95% CI (0.62, 0.94), p = 0.012], 21% [OR = 0.79, 95% CI (0.66, 0.95), p = 0.013], respectively. When the fourth quartile PAL was reached, the diabetes incidence was significantly reduced in these populations by 41% [OR = 0.59, 95% CI (0.48, 0.73), p < 0.001], 39% [OR = 0.61, 95% CI (0.49, 0.76), p < 0.001], and 41% [OR = 0.59, 95% CI (0.46, 0.75), p < 0.001], respectively. And there was no statistically significant association between other PAL and diabetes incidence.
3.4 Sensitivity analysis
In binary logistic regression analyses, confounding variables may influence the association between physical activity and diabetes incidence, potentially biasing the results. Consequently, this study will assess result robustness by sequentially excluding these confounders. Sensitivity analyses confirmed that the relationship between physical activity and diabetes incidence remained robust (refer to Supplementary Table S1).
4 Discussion
In this study, we used a nationally representative cross-sectional study to assess the correlation and dose–response relationship between different PAL and the diabetes incidence in the middle-aged and older adults population in China, and we found that there was a negative dose relationship between physical activity and diabetes incidence, with differences in gender, age, and residency.
The finding that engaging in physical activity reduces the diabetes incidence is consistent with previous research findings (28). Current diabetes prevention guidelines state that individuals can reduce their diabetes incidence through engaging in various physical activities (29). Most previous research has focused on the effects of leisure physical activity on diabetes incidence, with fewer studies on non-leisure physical activity (30). However, in the Chinese middle-aged and older adults population, the proportion of daily participation in leisure physical activity is low (31). Non-leisure physical activities, such as household, commuting, and occupational physical activities, are the main sources of daily energy expenditure. Additionally, it has been suggested that the protective effect of physical activity on diabetes incidence may be related to total energy expenditure (32), not just leisure physical activity. Therefore, this study only examined the relationship between different levels of physical activity and diabetes incidence in middle-aged and older adults without categorizing the types of physical activity.
Several biological mechanisms may explain the beneficial effects of physical activity on diabetes. Initially, physical activity improves energy balance and mitigates obesity (33), which is a major risk factor for diabetes (34, 35). Secondly, muscle contraction improves glucose homeostasis by increasing skeletal muscle glucose uptake by translocating the GLUT4 glucose transporter to skeletal muscle cell membranes and increasing glycogen synthase activity (36, 37). Nextly, physical activity normalizes insulin resistance in liver and skeletal muscles (38). Finally, research has shown that inflammation contributes to the onset of diabetes (39), and physical activity raises interleukin-6(IL-6) levels, which combat inflammation, thus indirectly regulating glycemic irregularities (40, 41). Additionally, Cardiovascular disease is recognized as a risk factor for diabetes (42). Physical activity can increase cardiac efficiency and improve vascular health, thereby reducing the incidence of cardiovascular disease in middle-aged and older adults individuals (43), which is also one of the mechanisms. High PAL have been found to modulate diabetes incidence better than low levels (44–48), aligning with the negative correlation noted in our study between PAL and diabetes incidence. Sustained high PAL can induce adaptations in middle-aged and older adults, such as weight loss, increased GLUT4 and interleukin-6 expression, and reduction in cardiovascular diseases, all contributing to reduced diabetes incidence. However, some studies contrast with our findings, with finding indicating that moderate PAL activity may reduce diabetes incidence more effectively than other levels (20, 49). The reason for this difference may be related to ethnicity, and study found that genetic polymorphisms may be one of the reasons why physical activity has different effects on diabetes incidence in different populations (50). A multiracial cohort study validated this conjecture that differences in gene may have an impact on the relationship between physical activity and diabetes (46). Furthermore, a study provides a possible explanation for this differentiation (51). The interaction between genes and the environment may cause varying rates of fat oxidation during exercise among different ethnic groups, which in turn leads to the need for different PAL in different ethnic groups to modulate diabetes incidence.
Further subgroup analyses revealed gender, age, and residency differences in the effect of physical activity on diabetes among Chinese middle-aged and older adults. Female middle-aged and older adults may require higher PAL than their male counterparts to significantly reduce diabetes incidence, potentially due to differences in gender-induced biological and psychosocial factors (52). At the age level, we found that the beneficial impact of physical activity on diabetes incidence decreases with age, aligning with previous research findings (53). The reason for this phenomenon may be that the increased incidence of diabetes due to aging diminishes the protective effect of physical activity on diabetes (54, 55). At the level of residency, compared to the first quartile PAL, urban middle-aged and older adults experienced significant diabetes incidence reduction at the third and fourth quartile of physical activity, while rural counterparts saw comparable benefits only upon reaching the fourth quartile. Moreover, the same PAL is more effective in reducing diabetes incidence among urban middle-aged and older adults compared to their rural counterparts, a disparity attributed to various factors. A study in India found that (56) urban areas possess superior healthcare systems compared to rural areas, allowing urban older adults to better manage diabetes under the same circumstances. Given that both China and India are developing countries, the disparities between urban and rural areas in China may mirror those in India. It has also been found that differences in self-health management awareness among residents may contribute to the urban–rural disparities (57). Urban middle-aged and older adults individuals may focus on diabetes prevention and treatment earlier than those in rural areas and may take other protective measures to reduce the diabetes incidence when performing the same physical activities. Moreover, there will still be numerous factors contributing to the urban–rural differences, and more further studies are needed to explore the mechanisms in the future.
Several notable strengths exist in this study. Firstly, the data were derived from a representative sample of middle-aged and older adults individuals across China, with a large sample size and a wide range of data collection, encompassing 150 counties and 450 communities in 28 provinces. This broad data collection allows for a comprehensive analysis of the relationship between physical activity and diabetes incidence among this demographic. Secondly, the use of a standardized international questionnaire, combined with a large data sample and sensitivity analyses, enhances the study’s reliability. Thirdly, the study investigates the relationship between various PAL and diabetes incidence, while also analyzing differences across gender, age, and residency. This enriches the research field and provides a valuable reference for future studies.
This study also has several limitations. Firstly, the data were sourced from 17,226 respondents in 2018 without longitudinal data over many years, thus the cross-sectional design could not establish causality between physical activity and diabetes incidence. Secondly, this study focused on a Chinese middle-aged and older adults population, thus its findings are specific to China and may not be generalizable to other countries. Thirdly, this study used self-report questionnaires, and the reporting of physical activity scores and other covariates relied on self-reporting provided by subjects or their informants and is therefore subject to some bias. Fourthly, despite adjustments for several important influencing factors, confounding factors not accounted for may have impacted the study’s results.
5 Conclusion
Higher PAL was associated with lower diabetes incidence in specific Chinese middle-aged and older adults population. It is feasible to use physical activity to predict diabetes incidence in this demographic, and high PAL may be an effective means of preventing and controlling diabetes. This study provides the basis and clues for early clinical prediction and reduction of diabetes incidence in middle-aged and older adults people. Future research should intensify efforts to investigate the causal links between physical activity and diabetes incidence, thereby providing a theoretical foundation for diabetes prevention and treatment in the group.
Data availability statement
The datasets presented in this study can be found in online repositories. The names of the repository/repositories and accession number(s) can be found in the article/Supplementary material.
Ethics statement
The studies involving humans were approved by Peking University’s Biomedical Ethics Review Committee. The studies were conducted in accordance with the local legislation and institutional requirements. Written informed consent for participation in this study was provided by the participants’ legal guardians/next of kin. Written informed consent was obtained from the individual(s) for the publication of any potentially identifiable images or data included in this article.
Author contributions
YZ: Conceptualization, Data curation, Investigation, Methodology, Software, Supervision, Writing – original draft, Writing – review & editing. FM: Data curation, Formal analysis, Methodology, Supervision, Writing – original draft, Writing – review & editing. XF: Conceptualization, Data curation, Writing – review & editing. KW: Data curation, Supervision, Writing – review & editing. YW: Conceptualization, Investigation, Writing – review & editing. XW: Conceptualization, Investigation, Software, Writing – original draft, Writing – review & editing.
Funding
The author(s) declare that no financial support was received for the research, authorship, and/or publication of this article.
Acknowledgments
We would like to acknowledge the China Health and Retirement Longitudinal Study (CHARLS) team for providing high-quality, nationally representative data.
Conflict of interest
The authors declare that the research was conducted in the absence of any commercial or financial relationships that could be construed as a potential conflict of interest.
Publisher’s note
All claims expressed in this article are solely those of the authors and do not necessarily represent those of their affiliated organizations, or those of the publisher, the editors and the reviewers. Any product that may be evaluated in this article, or claim that may be made by its manufacturer, is not guaranteed or endorsed by the publisher.
Supplementary material
The Supplementary material for this article can be found online at: https://www.frontiersin.org/articles/10.3389/fpubh.2024.1430229/full#supplementary-material
References
1. Saeedi, P, Salpea, P, Karuranga, S, Petersohn, I, Malanda, B, Gregg, EW, et al. Mortality attributable to diabetes in 20-79 years old adults, 2019 estimates: results from the international diabetes federation diabetes atlas. Diabetes Res Clin Pract. (2020) 162:108086. doi: 10.1016/j.diabres.2020.108086
2. Saeedi, P, Petersohn, I, Salpea, P, Malanda, B, Karuranga, S, Unwin, N, et al. Global and regional diabetes prevalence estimates for 2019 and projections for 2030 and 2045: results from the international diabetes federation diabetes atlas. Diabetes Res Clin Pract. (2019) 157:107843. doi: 10.1016/j.diabres.2019.107843
3. Kuan, V, Denaxas, S, Patalay, P, Nitsch, D, Mathur, R, Gonzalez-Izquierdo, A, et al. Identifying and visualising multimorbidity and comorbidity patterns in patients in the English National Health Service: a population-based study. Lancet Digit Health. (2023) 5:e16–27. doi: 10.1016/S2589-7500(22)00187-X
4. Hauguel-Moreau, M, Hergault, H, Cazabat, L, Pépin, M, Beauchet, A, Aïdan, V, et al. Prevalence of prediabetes and undiagnosed diabetes in a large urban middle-aged population: the CARVAR 92 cohort. Cardiovasc Diabetol. (2023) 22:31. doi: 10.1186/s12933-023-01761-3
5. Biessels, GJ, and Despa, F. Cognitive decline and dementia in diabetes mellitus: mechanisms and clinical implications. Nat Rev Endocrinol. (2018) 14:591–604. doi: 10.1038/s41574-018-0048-7
6. Moulton, CD, Pickup, JC, and Ismail, K. The link between depression and diabetes: the search for shared mechanisms. Nat Rev Endocrinol. (2015) 3:461–71. doi: 10.1016/S2213-8587(15)00134-5
7. Laugsand, LE, Janszky, I, Vatten, LJ, Dalen, H, Midthjell, K, Grill, V, et al. Autoimmune diabetes in adults and risk of myocardial infarction: the HUNT study in Norway. J Intern Med. (2016) 280:518–31. doi: 10.1111/joim.12530
8. Rasmussen, NH, and Dal, J. Falls and fractures in diabetes-more than bone fragility. Curr Osteoporos Rep. (2019) 17:147–56. doi: 10.1007/s11914-019-00513-1
9. Feldman, EL, Nave, KA, Jensen, TS, and Bennett, DLH. New horizons in diabetic neuropathy: mechanisms, bioenergetics, and pain. Neuron. (2017) 93:1296–313. doi: 10.1016/j.neuron.2017.02.005
10. Calcutt, NA . Diabetic neuropathy and neuropathic pain: a (con)fusion of pathogenic mechanisms? Pain. (2020) 161:S65–86. doi: 10.1097/j.pain.0000000000001922
11. Napoli, N, Chandran, M, Pierroz, DD, Abrahamsen, B, Schwartz, AV, Ferrari, SL, et al. Mechanisms of diabetes mellitus-induced bone fragility. Nat Rev Endocrinol. (2017) 13:208–19. doi: 10.1038/nrendo.2016.153
12. Chen, R, Xu, P, Li, F, and Song, P. Internal migration and regional differences of population aging: an empirical study of 287 cities in China. Biosci Trends. (2018) 12:132–41. doi: 10.5582/bst.2017.01246
13. Fang, EF, Scheibye-Knudsen, M, Jahn, HJ, Li, J, Ling, L, Guo, H, et al. A research agenda for aging in China in the 21st century. Ageing Res Rev. (2015) 24:197–205. doi: 10.1016/j.arr.2015.08.003
14. Bommer, C, Sagalova, V, Heesemann, E, Manne-Goehler, J, Atun, R, Bärnighausen, T, et al. Global economic burden of diabetes in adults: projections from 2015 to 2030. Diabetes Care. (2018) 41:963–70. doi: 10.2337/dc17-1962
15. Shakoor, H, Platat, C, Ali, HI, Ismail, LC, Al Dhaheri, AS, Bosevski, M, et al. The benefits of physical activity in middle-aged individuals for cardiovascular disease outcomes. Maturitas. (2023) 168:49–52. doi: 10.1016/j.maturitas.2022.11.002
16. Lucertini, F, Ferri Marini, C, Sisti, D, Stocchi, V, Federici, A, Gregorio, F, et al. Discontinuously supervised aerobic training vs. physical activity promotion in the self-management of type 2 diabetes in older Italian patients: design and methods of the “TRIPL-A” randomized controlled trial. BMC Geriatr. (2019) 19:11. doi: 10.1186/s12877-018-1022-x
17. Zheng, Y, Ley, SH, and Hu, FB. Global aetiology and epidemiology of type 2 diabetes mellitus and its complications. Nat Rev Endocrinol. (2018) 14:88–98. doi: 10.1038/nrendo.2017.151
18. Craig, CL, Marshall, AL, Sjöström, M, Bauman, AE, Booth, ML, Ainsworth, BE, et al. International physical activity questionnaire: 12-country reliability and validity. Med Sci Sports Exerc. (2003) 35:1381–95. doi: 10.1249/01.MSS.0000078924.61453.FB
19. Lao, XQ, Deng, HB, Liu, X, Chan, TC, Zhang, Z, Chang, LY, et al. Increased leisure-time physical activity associated with lower onset of diabetes in 44 828 adults with impaired fasting glucose: a population-based prospective cohort study. Br J Sports Med. (2019) 53:895–900. doi: 10.1136/bjsports-2017-098199
20. Koloverou, E, Tambalis, K, Panagiotakos, DB, Georgousopoulou, E, Chrysohoou, C, Skoumas, I, et al. Moderate physical activity reduces 10-year diabetes incidence: the mediating role of oxidative stress biomarkers. Int J Public Health. (2018) 63:297–305. doi: 10.1007/s00038-017-1052-8
21. Kaleta, M, Leutner, M, Thurner, S, Endel, G, Kiss, N, Robausch, M, et al. Trends in diabetes incidence in Austria 2013-2017. Sci Rep. (2023) 13:8715. doi: 10.1038/s41598-023-35806-0
22. Zhao, Y, Hu, Y, Smith, JP, Strauss, J, and Yang, G. Cohort profile: the China health and retirement longitudinal study (CHARLS). Int J Epidemiol. (2014) 43:61–8. doi: 10.1093/ije/dys203
23. Tang, S, Yang, T, Ye, C, Liu, M, Gong, Y, Yao, L, et al. Research on grandchild care and depression of chinese older adults based on CHARLS 2018: the mediating role of intergenerational support from children. BMC Public Health. (2022) 22:137. doi: 10.1186/s12889-022-12553-x
24. Lee, PH, Macfarlane, DJ, Lam, TH, and Stewart, SM. Validity of the international physical activity questionnaire short form (IPAQ-SF): a systematic review. Int J Behav Nutr Phys Act. (2011) 8:115. doi: 10.1186/1479-5868-8-115
25. Basevi, V, Di Mario, S, Morciano, C, Nonino, F, and Magrini, NAmerican Diabetes Association. Standards of medical care in diabetes--2011. Diabetes Care. (2011) 34:S11–61. doi: 10.2337/dc11-S011
26. Zhou, Z, Yu, Y, Zhou, R, Luan, R, and Li, K. Associations between sleep duration, midday napping, depression, and falls among postmenopausal women in China: a population-based nationwide study. Menopause. (2021) 28:554–63. doi: 10.1097/GME.0000000000001732
27. Chen, H, and Mui, AC. Factorial validity of the Center for Epidemiologic Studies Depression Scale short form in older population in China. Int Psychogeriatr. (2014) 26:49–57. doi: 10.1017/S1041610213001701
28. Rietz, M, Lehr, A, Mino, E, Lang, A, Szczerba, E, Schiemann, T, et al. Physical activity and risk of major diabetes-related complications in individuals with diabetes: a systematic review and Meta-analysis of observational studies. Diabetes Care. (2022) 45:3101–11. doi: 10.2337/dc22-0886
29. Colberg, SR, Sigal, RJ, Yardley, JE, Riddell, MC, Dunstan, DW, Dempsey, PC, et al. Physical activity/exercise and diabetes: a position statement of the American Diabetes Association. Diabetes Care. (2016) 39:2065–79. doi: 10.2337/dc16-1728
30. Aune, D, Norat, T, Leitzmann, M, Tonstad, S, and Vatten, LJ. Physical activity and the risk of type 2 diabetes: a systematic review and dose-response meta-analysis. Eur J Epidemiol. (2015) 30:529–42. doi: 10.1007/s10654-015-0056-z
31. Zhang, M, Chen, X, Wang, Z, Wang, L, and Jiang, Y. Leisure-time physical exercise and sedentary behavior among Chinese elderly, in 2010. Zhonghua Liu Xing Bing Xue Za Zhi. (2014) 35:242–5. doi: 10.3760/cma.j.issn.0254-6450.2014.03.005
32. Wareham, NJ . Epidemiological studies of physical activity and diabetes risk, and implications for diabetes prevention. Appl Physiol Nutr Metab. (2007) 32:778–82. doi: 10.1139/H07-032
33. Mozaffarian, D, Hao, T, Rimm, EB, Willett, WC, and Hu, FB. Changes in diet and lifestyle and long-term weight gain in women and men. N Engl J Med. (2011) 364:2392–404. doi: 10.1056/NEJMoa1014296
34. Wei, Y, Wang, J, Han, X, Yu, C, Wang, F, Yuan, J, et al. Metabolically healthy obesity increased diabetes incidence in a middle-aged and elderly Chinese population. Diabetes Metab Res Rev. (2020) 36:e3202. doi: 10.1002/dmrr.3202
35. Yan, Y, Wu, T, Zhang, M, Li, C, Liu, Q, and Li, F. Prevalence, awareness and control of type 2 diabetes mellitus and risk factors in Chinese elderly population. BMC Public Health. (2022) 22:1382. doi: 10.1186/s12889-022-13759-9
36. Röckl, KSC, Witczak, CA, and Goodyear, LJ. Signaling mechanisms in skeletal muscle: acute responses and chronic adaptations to exercise. IUBMB Life. (2008) 60:145–53. doi: 10.1002/iub.21
37. Perseghin, G, Price, TB, Petersen, KF, Roden, M, Cline, GW, Gerow, K, et al. Increased glucose transport-phosphorylation and muscle glycogen synthesis after exercise training in insulin-resistant subjects. N Engl J Med. (1996) 335:1357–62. doi: 10.1056/NEJM199610313351804
38. Yeo, SE, and Coker, RH. Aerobic exercise training versus the aetiology of insulin resistance. Eur J Sport Sci. (2008) 8:3–14. doi: 10.1080/17461390701832645
39. Donath, MY, and Shoelson, SE. Type 2 diabetes as an inflammatory disease. Nat Rev Immunol. (2011) 11:98–107. doi: 10.1038/nri2925
40. Timper, K, Dalmas, E, Dror, E, Rütti, S, Thienel, C, Sauter, NS, et al. Glucose-dependent Insulinotropic peptide stimulates glucagon-like peptide 1 production by pancreatic islets via interleukin 6, produced by α cells. Gastroenterology. (2016) 151:165–79. doi: 10.1053/j.gastro.2016.03.003
41. Braune, J, Weyer, U, Hobusch, C, Mauer, J, Brüning, JC, Bechmann, I, et al. IL-6 regulates M2 polarization and local proliferation of adipose tissue macrophages in obesity. J Immunol (Baltimore, Md: 1950). (2017) 198:2927–34. doi: 10.4049/jimmunol.1600476
42. Lee, SI, Patel, M, Jones, CM, and Narendran, P. Cardiovascular disease and type 1 diabetes: prevalence, prediction and management in an ageing population. Ther Adv Chronic Dis. (2015) 6:347–74. doi: 10.1177/2040622315598502
43. Feng, H, Yang, L, Liang, YY, Ai, S, Liu, Y, Liu, Y, et al. Associations of timing of physical activity with all-cause and cause-specific mortality in a prospective cohort study. Nat Commun. (2023) 14:930. doi: 10.1038/s41467-023-36546-5
44. Yang, J, Zhang, YL, Ye, J, Guo, Q, Sun, YN, and Zeng, Q. Association between household physical activity level and type 2 diabetes among urban males in northern China. Public Health. (2018) 163:113–20. doi: 10.1016/j.puhe.2018.07.010
45. InterAct ConsortiumEkelund, U, Palla, L, Brage, S, Franks, PW, Peters, T, et al. Physical activity reduces the risk of incident type 2 diabetes in general and in abdominally lean and obese men and women: the EPIC-InterAct study. Diabetologia. (2012) 55:1944–52. doi: 10.1007/s00125-012-2532-2
46. Steinbrecher, A, Erber, E, Grandinetti, A, Nigg, C, Kolonel, LN, and Maskarinec, G. Physical activity and risk of type 2 diabetes among native Hawaiians, Japanese Americans, and Caucasians: the multiethnic cohort. J Phys Act Health. (2012) 9:634–41. doi: 10.1123/jpah.9.5.634
47. Tian, C, Bürki, C, Westerman, KE, and Patel, CJ. Association between timing and consistency of physical activity and type 2 diabetes: a cohort study on participants of the UK biobank. Diabetologia. (2023) 66:2275–82. doi: 10.1007/s00125-023-06001-7
48. Demakakos, P, Hamer, M, Stamatakis, E, and Steptoe, A. Low-intensity physical activity is associated with reduced risk of incident type 2 diabetes in older adults: evidence from the English longitudinal study of ageing. Diabetologia. (2010) 53:1877–85. doi: 10.1007/s00125-010-1785-x
49. Fretts, AM, Howard, BV, McKnight, B, Duncan, GE, Beresford, SAA, Calhoun, D, et al. Modest levels of physical activity are associated with a lower incidence of diabetes in a population with a high rate of obesity: the strong heart family study. Diabetes Care. (2012) 35:1743–5. doi: 10.2337/dc11-2321
50. Bray, MS, Hagberg, JM, Pérusse, L, Rankinen, T, Roth, SM, Wolfarth, B, et al. The human gene map for performance and health-related fitness phenotypes: the 2006-2007 update. Med Sci Sports Exerc. (2009) 41:35–73. doi: 10.1249/MSS.0b013e3181844179
51. Gill, JMR, Celis-Morales, CA, and Ghouri, N. Physical activity, ethnicity and cardio-metabolic health: does one size fit all? Atherosclerosis. (2014) 232:319–33. doi: 10.1016/j.atherosclerosis.2013.11.039
52. Kautzky-Willer, A, Harreiter, J, and Pacini, G. Sex and gender differences in risk, pathophysiology and complications of type 2 diabetes mellitus. Endocr Rev. (2016) 37:278–316. doi: 10.1210/er.2015-1137
53. Fan, S, Chen, J, Huang, J, Li, Y, Zhao, L, Liu, X, et al. Physical activity level and incident type 2 diabetes among Chinese adults. Med Sci Sports Exerc. (2015) 47:751–6. doi: 10.1249/MSS.0000000000000471
54. Kirkman, MS, Briscoe, VJ, Clark, N, Florez, H, Haas, LB, Halter, JB, et al. Diabetes in older adults: a consensus report. J Am Geriatr Soc. (2012) 60:2342–56. doi: 10.1111/jgs.12035
55. Koo, BK, and Moon, MK. Are we in the same risk of diabetes mellitus? Gender- and age-specific epidemiology of diabetes in 2001 to 2014 in the Korean population. Diabetes Metab J. (2016) 40:175–81. doi: 10.4093/dmj.2016.40.3.175
56. Rai, P, Sahadevan, P, Mensegere, AL, Issac, TG, Muniz-Terrera, G, and Sundarakumar, JS. Rural-urban disparities in the diagnosis and treatment of hypertension and diabetes among aging Indians. Alzheimers Dement. (2024) 20:2943–51. doi: 10.1002/alz.13771
Keywords: physical activity, diabetes, middle-aged and older adults, China, CHARLS
Citation: Zhang Y, Meng F, Fei X, Wang K, Wu Y and Wang X (2024) Association between physical activity level and diabetes incidence among Chinese middle-aged and older adults: a cross-sectional study from the China health and retirement longitudinal study. Front. Public Health. 12:1430229. doi: 10.3389/fpubh.2024.1430229
Edited by:
Feng Jiang, Shanghai Jiao Tong University, ChinaReviewed by:
Adela Badau, Transilvania University of Brașov, RomaniaFátima Méndez-López, University of Zaragoza, Spain
Copyright © 2024 Zhang, Meng, Fei, Wang, Wu and Wang. This is an open-access article distributed under the terms of the Creative Commons Attribution License (CC BY). The use, distribution or reproduction in other forums is permitted, provided the original author(s) and the copyright owner(s) are credited and that the original publication in this journal is cited, in accordance with accepted academic practice. No use, distribution or reproduction is permitted which does not comply with these terms.
*Correspondence: Xueting Wang, 6020200042@jsnu.edu.cn
†These authors have contributed equally to this work and share first authorship