- 1School of Public Health, Ningxia Medical University, Yinchuan, China
- 2Key Laboratory of Environmental Factors and Chronic Disease Control, Yinchuan, China
- 3School of Humanities and Management, Ningxia Medical University, Yinchuan, China
Objective: This study aims to explore the relationship between internet use and self-rated health among middle-aged and older adults and further investigates the mediating role of health service utilization between internet use and self-rated health, providing a reference for more effectively utilizing the internet to improve the health status of middle-aged and older adults.
Method: We analyzed data from the 2018 and 2020 waves of the China Health and Retirement Longitudinal Study (CHARLS), this includes 10,011 in 2018 and 7,080 in 2020 over the age of 45. To explore the relationship between internet use and self-rated health, we employed propensity score matching (PSM) and instrumental variable regression analysis, accounting for a wide range of potential covariates. Additionally, the Sobel test was used to examine the mediating effect of health service utilization on this relationship.
Results: According to the fully adjusted propensity score matching and instrumental variable regression model, internet use significantly enhanced self-rated health among middle-aged and older adults (β = 0.348, P < 0.01; β = 0.293, P < 0.1). However, subgroup analyses revealed that this positive effect was not significant among middle-aged and older adults who were divorced (β = 0.190, P > 0.05), lived in special zone (β = −1.379, P > 0.05), and lacked medical insurance (β = 0.314, P > 0.05). Furthermore, mediation analysis revealed that inpatient services (β = 0.0215, P < 0.01) acted as a mediator in the relationship between internet use and self-rated health.
Conclusion: Internet use positively correlates with self-rated health among middle-aged and older adults. Additionally, the utilization of health services can significantly influence this relationship. These findings underscore the importance of developing targeted government strategies to promote internet access and create a supportive online environment, thereby enhancing the health outcomes of this demographic group.
1 Introduction
Since the 1990s, the aging process in China has accelerated, characterized by its fast pace and large scale. According to the seventh national census data, the population aged 60 and above accounts for 18.7% of the total population, with those aged 65 and above accounting for 13.5% (1, 2), approaching the 14% threshold of a deeply aging society (3). The aging trend is becoming increasingly severe. Moreover, the rapid development of the internet has enabled more older adult people in China to enjoy convenient lives, with the internet penetration rate among the older adult population increasing steadily. As of December 2021, the number of older adult internet users in China aged 60 years and above reached 119 million, with an internet penetration rate of 43.2% (4). On the one hand, internet technology has facilitated people's daily lives, especially through the use of mobile internet via smartphones, which has brought great convenience (5). On the other hand, the advent of the internet has also had some negative impacts on people, particularly manifested as internet addiction (6). Health ecology theory posits that the environment's impact on individuals is multilayered and involves complex influencing factors (7). The proficiency in internet use among middle-aged and older groups is relatively low, resulting in a more challenging learning process for them. The digital anxiety they experience can lead to greater psychological resistance to using the internet, thereby causing disparities in internet usage among older adult individuals. Therefore, against the backdrop of population aging and the digital divide among older adult in China, studying the impact of internet use on the health of middle-aged and older Chinese people holds practical significance.
In recent years, scholars have explored the relationship between internet use and health. Studies have shown that internet technology overcomes the limitations of time and space, providing abundant information resources (8). By providing health knowledge, information, resources, and services to older adult people through the internet, the health status of older adult users can be effectively improved (9). The internet offers a platform for information sharing and doctor–patient interactions (10, 11). This online platform allows users to influence their health behaviors through information sharing and learning (12). Compared with non-users, older adult internet users show improvements in indicators of wellbeing, mental and physical health, and health behaviors. Additionally, internet use is significantly associated with better self-rated health (13–15), higher life satisfaction (16, 17), lower levels of depression and/or anxiety (18–20), and more favorable health behaviors (21, 22). However, other studies have reported opposite results. In the United States, Matusitz reported that internet use negatively impacts residents' health, specifically because it leads to sedentary and inactive behaviors, thereby increasing the risk of weight gain and obesity (23). Additionally, Hökby noted that both the frequency and accessibility of internet use have negative effects on health (24). Choi's survey in South Korea revealed that excessive internet use can have negative health impacts (25). Other studies have indicated that the internet compresses face-to-face communication among groups, thereby affecting the maintenance of interpersonal relationships (26), leading to self-isolation, inhibiting the expression of personal emotions (27), and increasing the tendency of internet users to suffer from depression (28).
Owing to differences in demographic characteristics and socioeconomic development levels across various countries and regions, the results of existing studies on the relationship between internet use and health vary. Overall, the current research has the following shortcomings: some studies on the relationship between internet use and health are from developed Western countries, while related research in developing countries such as China is still lacking; some studies focus on the health impact of internet use on middle-aged and older people but rarely consider the differences in self-rated health between internet users and non-users. Self-rated health (SRH) is considered a comprehensive and sensitive indicator for assessing health status (29, 30). In some cases, SRH is regarded as a more accurate predictor of mortality than many objective health indicators (31). Additionally, research on the potential mechanisms of the relationship between internet use and health is insufficient, often overlooking the important role of healthcare service utilization as a potential mediating variable and rarely considering the endogeneity issues that may lead to self-selection bias in samples.
With respect to the relationship between the internet and healthcare service utilization, research indicates that internet use provides a more convenient avenue for health management and medical services. Through the internet, people can access medical information, make doctor appointments, conduct online consultations, and purchase medications, effectively improving the efficiency and quality of medical resource utilization and services (32). Additionally, the internet can enhance self-health management, access to health information, and disease prevention (33, 34) by providing information resources and establishing interactive communication platforms (12). During the COVID-19 pandemic, the role of the internet in providing health-related information and healthcare services has increased significantly, leading some to recognize it as one of the key determinants of health (6).
In summary, the literature has not reached a consensus on the relationship between the internet and health. Previous studies have investigated the relationship between internet use and self-rated health among middle-aged and older individuals, but there is still a lack of research examining healthcare service utilization as a key mediating variable in the relationship between internet use and self-rated health among middle-aged and older adults individuals. Therefore, this study focuses on the health status disparities among older adults in China under the digital divide, explores the association between internet use and self-rated health among middle-aged and older adults self-rated health and the mediating role of healthcare service utilization. This study utilizes data from the China Health and Retirement Longitudinal Study (CHARLS) for 2018 and 2020. The sample includes a nationally representative group of individuals aged 45 and above, providing a broader and more comprehensive perspective. This study explores the relationship between internet use and self-rated health, aiming to provide new empirical evidence for a deeper understanding of technological advancements and their impact on population health.
2 Methods
2.1 Sample and data collection
The China Health and Retirement Longitudinal Study (CHARLS) is an extensive interdisciplinary survey initiative spearheaded by the National Development Institute of Peking University and executed by the China Social Science Survey Center of Peking University. This project aims to gather high-quality microdata that accurately represent the households and individuals of Chinese adults aged 45 years and above. CHARLS has conducted extensive surveys and interviews across 150 counties and 450 communities (villages) within 28 provinces (including autonomous regions and municipalities) during the years 2011, 2013, 2015, 2018, and 2020 (35). The project kicked off with the CHARLS National Baseline Survey in 2011, a comprehensive effort that spanned 2 years and involved 23,000 respondents across 12,400 households. Our current analysis draws from the data collected in the 2018 and 2020 cycles, specifically targeting adults aged 45 years and above. From these two cycles, a total of 17,091 subjects were screened, and the detailed process of participant selection is illustrated in Figure 1.
2.2 Survey instrument and variable selection
2.2.1 Explained variable (Y): self-rated health
Self-rated health (SRH) serves as a pivotal metric for evaluating aging and public health, functioning as the dependent variable in our study. Research has established a significant link between SRH and critical outcomes such as mortality, objective health indicators, and mental health (36, 37). Consequently, employing SRH as a measure to gauge the overall health status of middle-aged and older adults is both reliable and validated (38). The CHARLS questionnaire probes participants' perceptions of their current physical health, a method widely recognized for assessing SRH. This survey instrument addresses both objective and subjective health aspects, offering a comprehensive view of an individual's health. Health is classified into five levels: “very bad,” “bad,” “fair,” “good,” and “very good,” which correspond to numeric scales ranging from 1 to 5. This scale facilitates the evaluation of self-rated health in individuals aged 45 and older, providing a structured approach to health assessment.
2.2.2 Explanatory variables (X): internet use
The independent variable, internet use among middle-aged and older adults, was assessed through the CHARLS questionnaire. This questionnaire featured a specific question inquiring about participants' internet activities in the previous month, asking, “Did you go online in the past month?” The range of online activities considered included chatting, reading news, watching videos, playing games, and managing finances via mobile phone networks. The responses were categorized simply as yes (1) or no (0) to indicate whether the participants had engaged in any online activities.
2.2.3 Mediating variable (M): health service utilization
The mediating variable is healthcare service utilization among middle-aged and older individuals. According to previous research, outpatient and inpatient service utilization are often used as indicators to measure healthcare service utilization (39–41). This study uses “outpatient” and “inpatient” as two measurement indicators to assess healthcare service utilization among middle-aged and older individuals. The CHARLS questionnaire includes the following questions: “In the past month, have you visited an outpatient clinic or received home medical services?” and “Have you been hospitalized in the past year?” The answers to “outpatient” and “inpatient” are “yes” or “no” and are binary variables.
2.2.4 Control variable
Research has shown that a combination of individual characteristics, family characteristics, and social insurance policies significantly influences self-rated health (42–44). Specifically, individual characteristics encompass demographics such as sex, age, marital status, education, drinking, exercise, and sleep duration. Family characteristics consider factors such as the current address and the duration of residence of children. Additionally, social insurance coverage, comprising medical insurance and endowment insurance, (Table 1).
All the variables are defined and assigned in Supplementary Table S1. A flowchart depicting the relationship between internet use and self-rated health is shown in Figure 2.
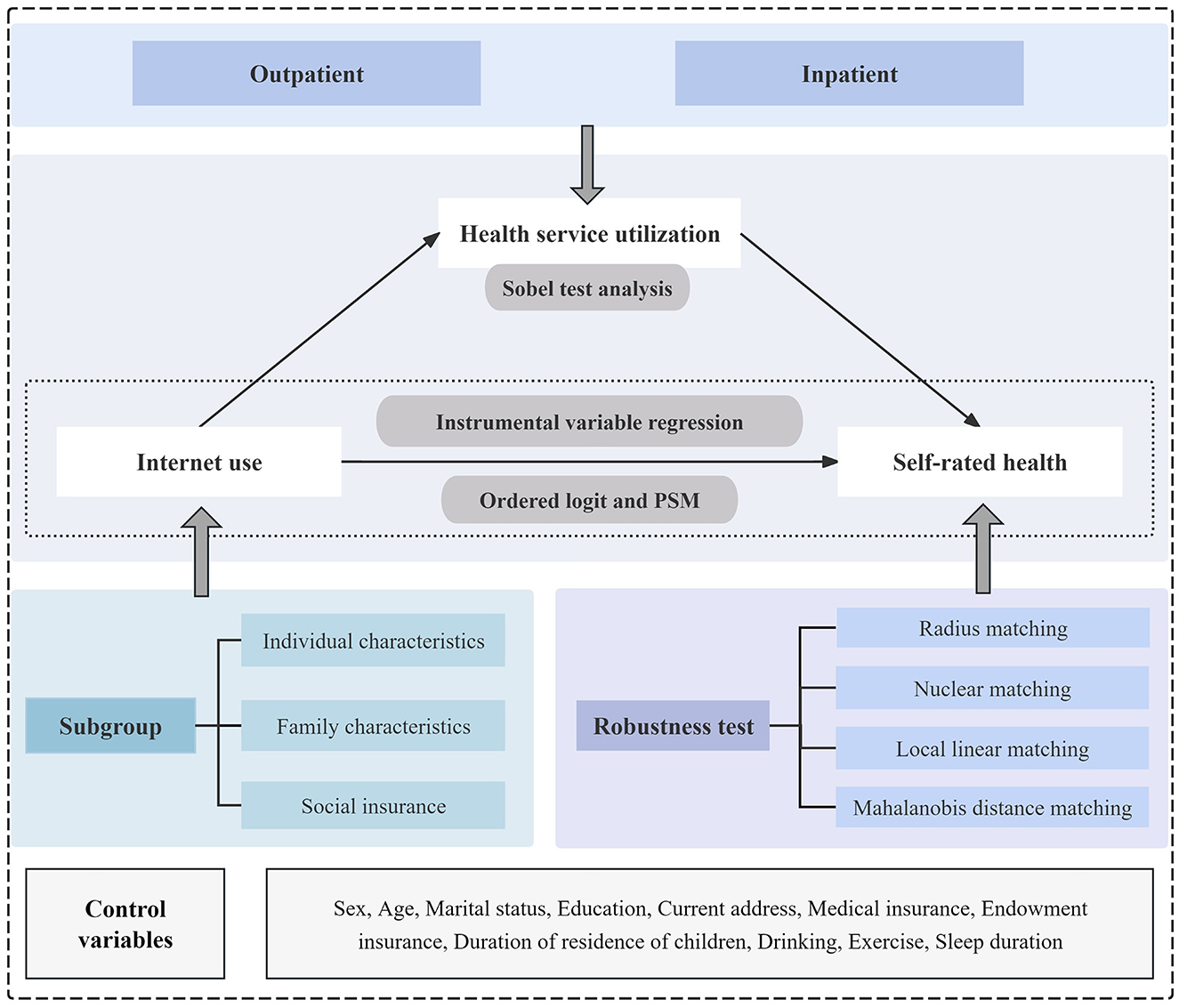
Figure 2. Diagram of the associations between internet use and self-rated health, including potential confounders.
2.3 Statistical analysis
In our study, before conducting the analysis, we rigorously examined all survey data for missing values and outliers. The statistical analysis was performed via econometric software, specifically SPSS 26.0 and STATA version 17.0. Initially, the study approached mixed factor issues via the propensity score matching (PSM) method. This involved balance testing and assessing the common support hypothesis. PSM regression and ordered logit regression analyses were subsequently performed to evaluate the association between internet use and self-rated health. To ensure robust findings, we used various propensity score matching methods and instrumental variable regression. Finally, a mediating analysis was performed to explore the pathway linking internet use and self-rated health. All the statistical tests in this study were performed at a significance level of P < 0.05 via a two-tailed approach.
3 Results
3.1 Variable definitions and descriptive statistics
This study is based on longitudinal data examining the relationship between health service utilization internet use and self-rated health, with 10,011 people included in 2018 and 7,080 people included in 2020. Table 1 shows the variable assignments and basic characteristics of the study population for both surveys in 2018 and 2020.
3.2 The propensity score matches the hypothesis of common support
For the effective application of the propensity score matching (PSM) method, it is essential to fulfill two critical conditions: the common support hypothesis and the parallel hypothesis. In our study, kernel density function plots were employed to assess the common support assumption (45–47). As illustrated in Figure 3, there was a noticeable disparity in the propensity score kernel density distributions between the experimental group (Internet users) and the control group (non-Internet users) prior to matching. However, after matching, a significant convergence in the kernel density distribution curves of both groups was observed. This convergence serves as evidence of effective matching, thereby validating the common support hypothesis.
3.3 Processing the self-selection problem
To assess the association between internet use and self-rated health among middle-aged and older adults while minimizing potential self-selection bias, we employed the propensity score matching technique. Table 2 demonstrates that the application of nearest-neighbor matching significantly reduced the standard deviations in the samples to < 10% (48, 49), indicating a more uniform variation in the observed characteristics within the sample and effectively managing confounding variables.
3.4 Comprehensive evaluation of the effect of internet use on self-rated health among middle-aged and older adults
Upon detailed analysis of Table 3, which includes columns (1) through (4), we delved into how internet use impacts the self-rated health of middle-aged and older adults. Ordered logit regression analyses were conducted for columns (1) and (2), whereas propensity score matching (PSM) methodologies were implemented in columns (3) and (4). Column (1) initially revealed a significant positive correlation between internet use and self-rated health without adjusting for control variables. The findings across columns (2) and (4) consistently demonstrated a significant positive relationship between internet use and self-rated health, even when additional variables were accounted for. Notably, in column (4), control variables such as current address, alcohol consumption, and sleep duration were found to be statistically significant at the 1% level, reinforcing the robustness of the association.
3.5 Robustness test based on the matching approach
This study adopted a variety of propensity score matching methods—including radius matching, nuclear matching, local linear matching, and Mahalanobis distance matching—to confirm the robustness of its findings (50–52). Table 4 shows that, regardless of the matching technique utilized, internet use consistently had a significant and positive influence on self-rated health (P < 0.001), underscoring the stability of our results across different methodologies.
3.6 Robustness tests: instrumental variable regression
Although this paper controls for variables such as individual and family characteristics that may affect self-rated health in the empirical model and the propensity score matching (PSM) method can alleviate biases caused by sample self-selection, there may still be endogeneity issues caused by omitted variables or reverse causality. Therefore, to avoid endogeneity issues, whether a family has broadband access is used as an instrumental variable, and a regression is conducted via an instrumental variable model to complete the endogeneity test. The instrumental variable method is an effective tool for addressing endogeneity issues, allowing for a more accurate estimation of the true effect of the explained variable by introducing an instrumental variable. The rationale for selecting this variable as an instrumental variable is 2-fold. First, the instrumental variable is closely related to the endogenous variable of internet use. Second, the instrumental variable is uncorrelated with the error term (53, 54). The estimation results of the instrumental variable are shown in Table 5. The results indicate that after controlling for other variables, the estimation results of the instrumental variable are not significant, suggesting that the instrumental variable does not affect self-rated health and has exogeneity. The first-stage estimation results of the impact of internet use on self-rated health show that family broadband coverage has a significant positive effect on internet use, confirming the validity of the instrumental variable. The second-stage estimation results reveal that internet use can significantly and positively promote self-rated health. This suggests that internet use plays a role in enhancing self-rated health, and this conclusion holds even after the endogeneity issue is addressed.
3.7 Subgroup and sensitivity analyses
Table 6 presents the subgroup analysis outcomes, exploring the relationship between internet use and self-rated health. This analysis demonstrated a stable connection across various demographic subgroups, such as sex, age, education, endowment insurance, duration of residence of children, drinking, exercise, and sleep duration. However, differences were observed based on marital status, current address and the presence of medical insurance. Notably, divorced individuals, lived in special zone and those without medical insurance exhibited no significant difference in the association between internet use and self-rated health (P > 0.05).
3.8 Analysis of the mediating effect of the action path
Our study included a mediation analysis to examine the relationships between self-rated health, internet use, health service utilization, and various covariates. The regression analysis revealed a significant positive correlation between the utilization of inpatient services and self-rated health (β = 0.0215, P < 0.01; BootSE = 0.0043), which accounted for 10.99% of the total effect observed. Conversely, the use of outpatient services did not demonstrate a significant mediating role, as indicated in Table 7 and Figure 4.The moderating effect results are shown in Supplementary Table S2 and Supplementary Figure S1.
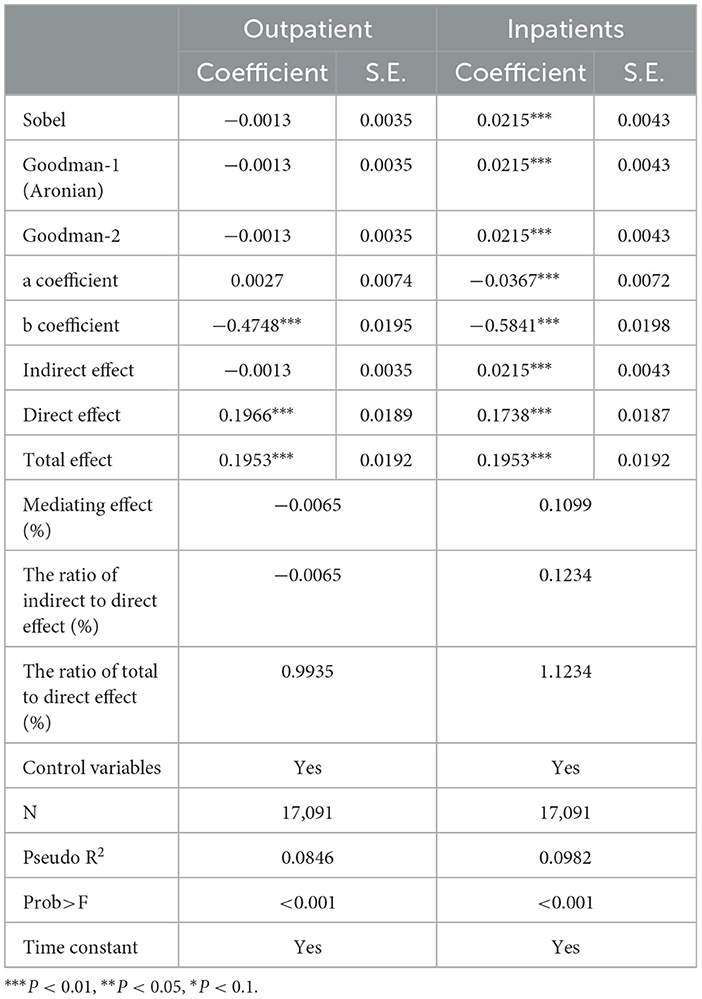
Table 7. Mediating role of health service utilization on internet use—self-rated health (N = 17,091).
4 Discussion
Investigating the association between internet use and self-rated health among middle-aged and older adults is crucial for societal and public health, particularly in the context of an aging population. This study focuses on understanding how the internet can support health promotion strategies tailored to middle-aged and older adults. We analyzed data from a nationally representative database of Chinese adults aged 45 years and older. Our findings reveal that internet use significantly enhances the health of middle-aged and older adults. This aligns with prior studies, underscoring the internet's role in improving health (55–57). The positive impact observed can be attributed to comprehensive health information (58), telemedicine services, and social support networks available online. These resources empower middle-aged and older adults in managing their health, increasing their quality of life, and fostering better health conditions.
Subgroup analyses highlighted the nuanced relationship between internet use and self-rated health in middle-aged and older adults. Notably, divorced individuals did not experience significant improvements in self-rated health associated with internet use. This lack of improvement could stem from increased feelings of loneliness and insufficient social support among this group (59). Despite having access to the internet, they may encounter obstacles in obtaining psychological support and substantial health services. In a similar vein, middle-aged and older adults lacking medical insurance, reported no significant health benefits from internet use. This absence of positive outcomes suggests that, while online health resources are accessible, uninsured individuals struggle to utilize these resources effectively and access medical services, primarily because of financial constraints (60).
This study revealed that the utilization of health services, especially inpatient services, plays a crucial role in mediating the relationship between internet use and self-rated health among middle-aged and older adults. The effect of internet use on improving self-rated health is less pronounced among individuals who regularly use outpatient services. This is likely because these services provide immediate access to professional medical advice and treatment, alleviating the uncertainties that come from solely relying on online health information. However, the impact of internet use is mediated by inpatient services, as older adults with serious health conditions benefit from the direct and comprehensive care offered by hospitals and healthcare institutions. Internet use can facilitate the booking of inpatient services and the inquiry of inpatient information, increasing personal utilization of these services. The utilization of inpatient services may, in turn, improve individual health conditions, thereby enhancing the self-assessed health levels of middle-aged and older adults.
5 Research strengths and limitations
Our study has several notable strengths. First, it utilized data from an extensive survey that covered 150 counties and districts across China, including major provinces and cities. To our knowledge, no other study has explored the association between health service utilization related to internet use and self-rated health in such a large and diverse Chinese population. Moreover, our analysis meticulously accounted for a wide range of potential confounding factors, ensuring the robustness of our findings. Additionally, the substantial sample size of our study facilitated a detailed evaluation of the heterogeneity in the relationship between internet use and self-rated health, allowing for nuanced insights into how this relationship varies across different segments of the population.
Our study has several limitations that merit consideration. Our research focuses primarily on middle-aged and older adults in China, which may limit the applicability of our findings to younger demographic data or populations from other countries. This focus inherently restricts the generalization of our results beyond the specified group. Additionally, the data for middle-aged and older adults were derived from the 2018 and 2020 CHARLS (China Health and Retirement Longitudinal Study) databases. These databases, while comprehensive, may not perfectly mirror the original populations owing to their screening processes. Moreover, our reliance on self-reported data could lead to an overestimation of the link between internet use and self-rated health.
6 Conclusion
Our study, which draws on data from the 2018 and 2020 China Health and Retirement Longitudinal Study (CHARLS), revealed a significant association between internet use and self-rated health among Chinese adults aged 45 years and older. Through subgroup analyses, we found that this association was not significant for individuals who were divorced, lived in special zone and without medical insurance. Furthermore, mediation analyses revealed that the use of inpatient services acted as a bridge connecting internet use to self-rated health. On the basis of these findings, we suggest that policymakers must devise targeted strategies that encourage internet adoption among middle-aged and older individuals. Such initiatives should aim to foster a supportive online environment, thereby improving the overall health and wellbeing of these population segments.
Data availability statement
The datasets presented in this study can be found in online repositories. The names of the repository/repositories and accession number(s) can be found below: http://charls.pku.edu.cn/index.htm.
Ethics statement
Prior to commencing the CHARLS, proficient interviewers briefed each participant on the survey's content, and each interviewee consented by signing an informed consent form. The survey content is treated with strict confidentiality, and all participant data are safeguarded by data security and privacy laws. Ethical approval for all waves of the CHARLS survey was granted by the Ethics Review Committee of Peking University (ethical approval number IRB00001052-11015). The studies were conducted in accordance with the local legislation and institutional requirements. The participants provided their written informed consent to participate in this study. Written informed consent was obtained from the individual(s) for the publication of any potentially identifiable images or data included in this article.
Author contributions
XM: Conceptualization, Data curation, Formal analysis, Writing – original draft, Writing – review & editing. QH: Conceptualization, Funding acquisition, Writing – review & editing. JH: Data curation, Writing – review & editing. WW: Data curation, Writing – review & editing. KC: Data curation, Writing – review & editing. HQ: Writing – review & editing, Funding acquisition.
Funding
This research was supported by the National Natural Science Foundation of China (grant number 72164033, 72364031, 72264032), the Natural Science Foundation of Ningxia, China (grant numbers 2023AAC03224, 2022AAC02036, and 2023AAC03241), the Open Competition Mechanism to Select the Best Candidates for Key Research Projects of Ningxia Medical University (grant number XJKF240314), the Key Research and Development Projects of Ningxia, China (grant number 2022BSB03082), and the Scientific Research Project of Higher Education Department of Ningxia, China (grant number NYG2024139).
Acknowledgments
The authors thank the CHARLS research team and all respondents for their contribution.
Conflict of interest
The authors declare that the research was conducted in the absence of any commercial or financial relationships that could be construed as a potential conflict of interest.
Publisher's note
All claims expressed in this article are solely those of the authors and do not necessarily represent those of their affiliated organizations, or those of the publisher, the editors and the reviewers. Any product that may be evaluated in this article, or claim that may be made by its manufacturer, is not guaranteed or endorsed by the publisher.
Supplementary material
The Supplementary Material for this article can be found online at: https://www.frontiersin.org/articles/10.3389/fpubh.2024.1429983/full#supplementary-material
References
1. Lu T, Kong L, Zhang H. Psychometric evaluation of the healthy aging activity engagement scale. Front Public Health. (2022) 10:986666. doi: 10.3389/fpubh.2022.986666
2. Zhang J, Xu F, Zhou Y, Wu J, Li Y, Qing W. Association between frailty and meaning in life of older adults in nursing home: the mediating effect of psychological resilience. Front Psychol. (2024) 15:1365817. doi: 10.3389/fpsyg.2024.1365817
3. Liu Y, Hou X, Tang Z, Zhang H, Liu J. The effect of different types of physical activity on cognitive reaction time in older adults in china. Front Public Health. (2022) 10:1051308. doi: 10.3389/fpubh.2022.1051308
4. Yang Y, Liu T, Jia Y. The impact of interaction with children on internet addiction in older adults: a moderated mediation model. Front Psychol. (2022) 13:989942. doi: 10.3389/fpsyg.2022.989942
5. von Rosen AJ, von Rosen FT, Tinnemann P, Muller-Riemenschneider F. Sexual health and the internet: cross-sectional study of online preferences among adolescents. J Med Internet Res. (2017) 19:e379. doi: 10.2196/jmir.7068
6. Duplaga M. The association between internet use and health-related outcomes in older adults and the elderly: a cross-sectional study. BMC Med Inform Decis Mak. (2021) 21:150. doi: 10.1186/s12911-021-01500-2
7. Rapport DJ, Howard J, Lannigan R, Mccauley W. Linking health and ecology in the medical curriculum. Environ Int. (2003) 29:353–8. doi: 10.1016/S0160-4120(02)00169-1
8. Rogers MA, Lemmen K, Kramer R, Mann J, Chopra V. Internet-delivered health interventions that work: systematic review of meta-analyses and evaluation of website availability. J Med Internet Res. (2017) 19:e90. doi: 10.2196/jmir.7111
9. Yang K. Internet use, employment performance and the health of Chinese residents. Int Health. (2022) 14:222–35. doi: 10.1093/inthealth/ihab034
10. Lee D, Helal S, Anton S, De Deugd S, Smith A. Participatory and persuasive telehealth. Gerontology. (2012) 58:269–81. doi: 10.1159/000329892
11. Meischke H, Eisenberg M, Rowe S, Cagle A. Do older adults use the internet for information on heart attacks? Results from a survey of seniors in king county, Washington. Heart Lung. (2005) 34:3–12. doi: 10.1016/j.hrtlng.2004.06.006
12. Uchino BN. Social support and health: a review of physiological processes potentially underlying links to disease outcomes. J Behav Med. (2006) 29:377–87. doi: 10.1007/s10865-006-9056-5
13. Gracia E, Herrero J. Internet use and self-rated health among older people: a national survey. J Med Internet Res. (2009) 11:e49. doi: 10.2196/jmir.1311
14. Falk EH, Ahlner F, Rydberg ST, Skoog I, Bergstrom A. Internet use and self-rated health among Swedish 70-year-olds: a cross-sectional study. BMC Geriatr. (2019) 19:365. doi: 10.1186/s12877-019-1392-8
15. Lee HY, Kim J, Sharratt M. Technology use and its association with health and depressive symptoms in older cancer survivors. Qual Life Res. (2018) 27:467–77. doi: 10.1007/s11136-017-1734-y
16. Heo J, Chun S, Lee S, Lee KH, Kim J. Internet use and well-being in older adults. Cyberpsychol Behav Soc Netw. (2015) 18:268–72. doi: 10.1089/cyber.2014.0549
17. Lam S, Jivraj S, Scholes S. Exploring the relationship between internet use and mental health among older adults in England: longitudinal observational study. J Med Internet Res. (2020) 22:e15683. doi: 10.2196/15683
18. Choi NG, Dinitto DM. Internet use among older adults: association with health needs, psychological capital, and social capital. J Med Internet Res. (2013) 15:e97. doi: 10.2196/jmir.2333
19. Wang Y, Zhang H, Feng T, Wang H. Does internet use affect levels of depression among older adults in china? A propensity score matching approach. Bmc Public Health. (2019) 19:1474. doi: 10.1186/s12889-019-7832-8
20. Liao S, Zhou Y, Liu Y, Wang R. Variety, frequency, and type of internet use and its association with risk of depression in middle- and older-aged Chinese: a cross-sectional study. J Affect Disord. (2020) 273:280–90. doi: 10.1016/j.jad.2020.04.022
21. Xavier AJ, D'Orsi E, Wardle J, Demakakos P, Smith SG, von Wagner C. Internet use and cancer-preventive behaviors in older adults: findings from a longitudinal cohort study. Cancer Epidemiol Biomarkers Prev. (2013) 22:2066–74. doi: 10.1158/1055-9965.EPI-13-0542
22. Peng YI, Chan YS. Do internet users lead a healthier lifestyle? J Appl Gerontol. (2020) 39:277–84. doi: 10.1177/0733464818785797
23. Matusitz J, Mccormick J. Sedentarism: the effects of internet use on human obesity in the united states. Soc Work Public Health. (2012) 27:250–69. doi: 10.1080/19371918.2011.542998
24. Hokby S, Hadlaczky G, Westerlund J, Wasserman D, Balazs J, Germanavicius A, et al. Are mental health effects of internet use attributable to the web-based content or perceived consequences of usage? A longitudinal study of European adolescents. JMIR Ment Health. (2016) 3:e31. doi: 10.2196/mental.5925
25. Choi M, Park S, Cha S. Relationships of mental health and internet use in Korean adolescents. Arch Psychiatr Nurs. (2017) 31:566–71. doi: 10.1016/j.apnu.2017.07.007
26. Lewandowski J, Rosenberg BD, Jordan Parks M, Siegel JT. The effect of informal social support: face-to-face versus computer-mediated communication. Comput Hum Behav. (2011) 27:1806–14. doi: 10.1016/j.chb.2011.03.008
27. Tang D, Jin Y, Zhang K, Wang D. Internet use, social networks, and loneliness among the older population in China. Front Psychol. (2022) 13:895141. doi: 10.3389/fpsyg.2022.895141
28. Xie L, Yang HL, Lin XY, Ti SM, Wu YY, Zhang S, et al. Does the internet use improve the mental health of Chinese older adults? Front Public Health. (2021) 9:673368. doi: 10.3389/fpubh.2021.673368
29. Leinonen R, Heikkinen E, Jylhä M. Changes in health, functional performance and activity predict changes in self-rated health: a 10-year follow-up study in older people. Arch Gerontol Geriatr. (2002) 35:79–92. doi: 10.1016/S0167-4943(02)00017-1
30. Huohvanainen E, Strandberg AY, Stenholm S, Pitkala KH, Tilvis RS, Strandberg TE. Association of self-rated health in midlife with mortality and old age frailty: a 26-year follow-up of initially healthy men. J Gerontol A Biol Sci Med Sci. (2016) 71:923–8. doi: 10.1093/gerona/glv311
31. Desalvo KB, Bloser N, Reynolds K, He J, Muntner P. Mortality prediction with a single general self-rated health question. A meta-analysis. J Gen Intern Med. (2006) 21:267–75. doi: 10.1111/j.1525-1497.2005.00291.x
32. Wu DC, Zhao X, Wu J. Online physician-patient interaction and patient satisfaction: empirical study of the internet hospital service. J Med Internet Res. (2023) 25:e39089. doi: 10.2196/39089
33. Alghamdi KM, Moussa NA. Internet use by the public to search for health-related information. Int J Med Inform. (2012) 81:363–73. doi: 10.1016/j.ijmedinf.2011.12.004
34. James N, Daniels H, Rahman R, Mcconkey C, Derry J, Young A, et al. A study of information seeking by cancer patients and their carers. Clin Oncol (R Coll Radiol). (2007) 19:356–62. doi: 10.1016/j.clon.2007.02.005
35. Zhao Y, Hu Y, Smith JP, Strauss J, Yang G. Cohort profile: the china health and retirement longitudinal study (charls). Int J Epidemiol. (2014) 43:61–8. doi: 10.1093/ije/dys203
36. Lyu S, Sun J. Internet use and self-rated health among Chinese older adults: the mediating role of social capital. Geriatr Gerontol Int. (2021) 21:34–8. doi: 10.1111/ggi.14090
37. Lima-Costa MF, Cesar CC, Chor D, Proietti FA. Self-rated health compared with objectively measured health status as a tool for mortality risk screening in older adults: 10-year follow-up of the Bambui cohort study of aging. Am J Epidemiol. (2012) 175:228–35. doi: 10.1093/aje/kwr290
38. Caetano SC, Silva CM, Vettore MV. Gender differences in the association of perceived social support and social network with self-rated health status among older adults: a population-based study in Brazil. BMC Geriatr. (2013) 13:122. doi: 10.1186/1471-2318-13-122
39. Huang CJ, Hsieh HM, Chiu HC, Wang PW, Lee MH, Li CY, et al. Health care utilization and expenditures of patients with diabetes comorbid with depression disorder: a national population-based cohort study. Psychiatry Investig. (2017) 14:770–8. doi: 10.4306/pi.2017.14.6.770
40. Nguyen T, Nguyen CT, Do HT, Tran HT, Vu T, Nghiem S, et al. Determinants of health-seeking behaviors among middle-aged women in Vietnam's rural-urban transition setting. Front Public Health. (2022) 10:967913. doi: 10.3389/fpubh.2022.967913
41. Hwang SE, Choi S, Kim K, Lee JK, Oh J, Park SM. Association between social trust and the risk of cardiovascular disease in older adults in Korea: a nationwide retrospective cohort study. BMC Public Health. (2020) 20:1844. doi: 10.1186/s12889-020-09964-z
42. Ming G. Associations between schemes of social insurance and self-rated health comparison: evidence from the employed migrants in urban china. Front Public Health. (2019) 7:253. doi: 10.3389/fpubh.2019.00253
43. Lieber J, Clarke L, Timus IM, Mallinson PAC, Kinra S. Changing family structures and self-rated health of India's older population (1995-96 to 2014). SSM - Population Health. (2020) 11:100572. doi: 10.1016/j.ssmph.2020.100572
44. Ningning L, Yujing H, Zhirong L. The relationship between internet use and self-rated health among older adults in china: the mediating role of social support. Int J Env Res Pub He. (2022) 19:14785. doi: 10.3390/ijerph192214785
45. Tetteh J, Adomako I, Udofia EA, Yarney E, Quansah H, Yawson AO, et al. Hygienic disposal of stools and risk of diarrheal episodes among children aged under two years: evidence from the Ghana demographic health survey, 2003-2014. PLoS One. (2022) 17:e266681. doi: 10.1371/journal.pone.0266681
46. Wei Y, Zhang Z, Zhang M. Effects of health poverty alleviation project from the perspective of vulnerability to poverty: evidence from five Chinese prefectures. Glob Health Action. (2023) 16:2260142. doi: 10.1080/16549716.2023.2260142
47. Zengxin X, Bowei L. The impact of the integration of urban and rural medical insurance on migrant workers' overwork: evidence from China. Front Public Health. (2022) 10:934524. doi: 10.3389/fpubh.2022.934524
48. Zhao J, Li W, Zhang Q. Do scholars-turned-businessmen impact green innovation? Front Psychol. (2022) 13:920782. doi: 10.3389/fpsyg.2022.920782
49. Huang W, He L, Lan H. The impact of self-employment on the health of migrant workers: evidence from China migrants dynamic survey. Int J Environ Res Public Health. (2022) 19:5868. doi: 10.3390/ijerph19105868
50. Kausik C, Peter H. The impact of COVID-19 vaccination for mental health. Eur Econ Rev. (2022) 150:104293. doi: 10.1016/j.euroecorev.2022.104293
51. Yang HL, Wu YY, Lin XY, Xie L, Zhang S, Zhang SQ, et al. Internet use, life satisfaction, and subjective well-being among the elderly: evidence from 2017 China general social survey. Front Public Health. (2021) 9:677643. doi: 10.3389/fpubh.2021.677643
52. Nan Y, Xie Y, Hu Y. Internet use and depression among Chinese older adults: the mediating effect of interpersonal relationship. Front Public Health. (2023) 11:1102773. doi: 10.3389/fpubh.2023.1102773
53. Zhou S, Wang Q. Do residential patterns affect women's labor market performance? An empirical study based on CHFS data. PLoS ONE. (2023) 18:e294558. doi: 10.1371/journal.pone.0294558
54. Ma W, Shen Z. Impact of community care services on the health of older adults: evidence from china. Front Public Health. (2023) 11:1160151. doi: 10.3389/fpubh.2023.1160151
55. Hou B, Li Y, Wang H. Internet use and health status among older adults: the mediating role of social participation. Front Public Health. (2022) 10:1072398. doi: 10.3389/fpubh.2022.1072398
56. Li L, Ding H, Li Z. Does internet use impact the health status of middle-aged and older populations? Evidence from china health and retirement longitudinal study (charls). Int J Environ Res Public Health. (2022) 19:3619. doi: 10.3390/ijerph19063619
57. Wen W, Zhang Y, Shi W, Li J. Association between internet use and physical health, mental health, and subjective health in middle-aged and older adults: nationally representative cross-sectional survey in china. J Med Internet Res. (2023) 25:e40956. doi: 10.2196/40956
58. Schueller SM, Leykin Y, Pérez-Stable EJ, Muñoz RF. Selection of intervention components in an internet stop smoking participant preference trial: beyond randomized controlled trials. Psychiat Res. (2013) 205:159–64. doi: 10.1016/j.psychres.2012.08.030
59. Mougharbel F, Sampasa-Kanyinga H, Heidinger B, Corace K, Hamilton HA, Goldfield GS. Psychological and demographic determinants of substance use and mental health during the COVID-19 pandemic. Front Public Health. (2021) 9:680028. doi: 10.3389/fpubh.2021.680028
Keywords: internet use, self-rated health, middle-aged and older adults, propensity score matching, instrumental variable, mediating effect
Citation: Ma X, Hu Q, He J, Wang W, Chen K and Qiao H (2024) Association of internet use and health service utilization with self-rated health in middle-aged and older adults: findings from a nationally representative longitudinal survey. Front. Public Health 12:1429983. doi: 10.3389/fpubh.2024.1429983
Received: 09 May 2024; Accepted: 27 August 2024;
Published: 03 October 2024.
Edited by:
Steven Hoffman, Brigham Young University, United StatesReviewed by:
Xianlong Zeng, Ohio University, United StatesJeff Bolles, Francis Marion University, United States
Li He, Zhongnan University of Economics and Law, China
Copyright © 2024 Ma, Hu, He, Wang, Chen and Qiao. This is an open-access article distributed under the terms of the Creative Commons Attribution License (CC BY). The use, distribution or reproduction in other forums is permitted, provided the original author(s) and the copyright owner(s) are credited and that the original publication in this journal is cited, in accordance with accepted academic practice. No use, distribution or reproduction is permitted which does not comply with these terms.
*Correspondence: Hui Qiao, cWlhb2h1aTcxQDE2My5jb20=
†These authors have contributed equally to this work and share first authorship