- 1Department of Neurology, Chengdu Seventh People’s Hospital, Chengdu, China
- 2General Practice Ward/International Medical Center Ward, General Practice Medical Center, West China Hospital, Sichuan University, Chengdu, China
- 3State Key Laboratory of Oral Diseases, National Clinical Research Center for Oral Diseases, National Center of Stomatology, West China School of Stomatology, Sichuan University, Chengdu, China
- 4Department of Emergency Medicine, West China Hospital, Sichuan University, Chengdu, Sichuan, China
Background: Cardiometabolic multimorbidity (CMM) and aging are increasing public health concerns. This prospective study used UK Biobank cohort to investigate the relationship between biological aging and the trajectory of CMM to dementia and mortality.
Methods: CMM is the coexistence of at least two cardiometabolic diseases (CMD), including stroke, ischemic heart disease, and diabetes. Biological age was calculated using the KDM-BA and PhenoAge algorithms. Accelerated aging indicated biological age advances more rapidly than chronological age.
Results: The study included 415,147 individuals with an average age of 56.5 years. During the average 11-year follow-up period, CMD-free individuals with accelerated aging had a significantly greater risk of CMD (KDM-BA, HR 1.456; PhenoAge, HR 1.404), CMM (KDM-BA, HR 1.952; PhenoAge, HR 1.738), dementia (KDM-BA, HR 1.243; PhenoAge, HR 1.212), and mortality (KDM-BA, HR 1.821; PhenoAge, HR 2.047) in fully-adjusted Cox regression models (p < 0.05 for all). Accelerated aging had adjusted HRs of 1.489 (KDM-BA) and 1.488 (PhenoAge) for CMM, 1.434 (KDM-BA) and 1.514 (PhenoAge) for dementia, and 1.943 (KDM-BA) and 2.239 (PhenoAge) for mortality in participants with CMD at baseline (p < 0.05 for all). CMM significantly mediated accelerated aging’s indirect effects on dementia by 13.7% (KDM-BA, HR) and 21.6% (PhenoAge); those on mortality were 4.7% (KDM-BA) and 5.2% (PhenoAge). The population attributable-risk of Life’s Essential 8 score (≥80 vs. <80) were 0.79 and 0.43 for KDM-BA and PhenoAge accelerated aging, respectively.
Conclusion: Biological aging involves the entire trajectory of CMM from a CMD-free state to CMD, to CMM, and ultimately to dementia and death. Life’s Essential 8 may be a potential target to counter age acceleration.
Introduction
Amidst the intensification of societal aging, cardiometabolic diseases (CMDs) have emerged as the most significant health challenges, with related deaths increasing by 56% from 1990 to 2019, reaching 15.7 million (1, 2). Cardiometabolic multimorbidity (CMM) refers to the coexistence of two or more CMDs, including conditions such as stroke, ischemic heart disease (IHD), and type 2 diabetes (T2D) (3). Populations with CMM have a two-fold increased mortality risk and a 12–15 year reduced life expectancy than those with single CMDs (1). The co-occurrence of multiple chronic CMDs has become increasingly common as the population ages (4, 5). Compared with people aged ≥40 years, the prevalence of CMM was 2.5-fold greater in people aged ≥60 years (6). Additionally, approximately one-third of older adults suffer from at least two co-morbid CMDs (7). Essentially, biological aging, which is associated with decreased metabolic rates, vascular stiffening, chronic inflammation, and oxidative stress, as well as the interplay of comorbidities, may underlie the progression of CMDs to CMM (8, 9). However, few studies have demonstrated the critical role of biological aging in this transition, and there is a notable lack of focus on biological aging as a mediator of health outcomes.
Aging is a complicated, gradual impairment of reserve function, recovery ability, and integrity of organs, tissues, and cells (10). The ideal measurement strategy for biological aging should include as many system indicators as possible (11). For predicting disease, the PhenoAge and Klemera–Doubal method Biological Age (KDM-BA) are the best-validated algorithms according to blood-chemistry–derived measures in multi-ethnic cohorts of older adults (12–15). Accelerated aging refers to the phenomenon where an individual’s biological age advances more rapidly than their chronological age. This concept goes beyond the mere passage of time, as it emphasizes the underlying biological processes and pathological changes. Thus, this study used both algorithms to test the association between biological aging and CMM.
Aging is the most influential factor in cognitive decline, regardless of dementia (16, 17). The 2020 Lancet Commission highlighted the need for a combination of cardiovascular and metabolic risk factors to minimize dementia and cognitive decline (18). CMM accelerates cognitive decline and increases the risk of cognitive impairment and dementia (19, 20). Thus, we hypothesize that accelerated biological aging is a pivotal factor in the transition from a CMD-free state to CMD, CMM, and ultimately to dementia and mortality.
Prolonging healthy aging improves the quality of life and reduces healthcare costs (10, 21, 22). High blood sodium levels, weight fluctuations, and a highly inflammatory diet are associated with the risk of accelerated aging (10, 23, 24). However, the contribution of cardiovascular health primordial prevention factors to biological aging is unclear (25). The American Heart Association’s Life’s Essential 8 (LE8) is a comprehensive metric that encapsulates eight key factors contributing to cardiovascular health, this framework include blood pressure, cholesterol, blood glucose, healthy diet, physical activity, weight, tobacco, and alcohol consumption (26). It serves as a critical tool for identifying risk factors and guiding interventions that can prevent or delay the onset of CMDs and mortality incident. Thus, we hypothesize that LE8 can serve as a preventive target to slow this aging process and mitigate adverse health outcomes.
Therefore, this study used data from the UK Biobank cohort to (1) investigate the relationship between accelerated aging and the risk of CMM, dementia, and mortality in healthy individuals; (2) analyze the relationship of accelerated aging with the risk of dementia and mortality in individuals with a single CMD or CMM; (3) clarify the mediating effect of CMM on accelerated aging leading to dementia or death; and (4) determine the relationship between the LE8 score and its components with accelerated aging.
Methods
Study design and population
The UK Biobank is an international health research cohort that included 502,655 UK adults (37–73 years old) between 2006 and 2010 (27). At baseline, the participants provided information on their lifestyle and health and their biological samples. For details of data collection.1 Data and materials can be obtained.2 All participants provided written informed consent before data collection. Ethics approval of UK Biobank study was approved by NHS National Research Ethics Service (16/NW/0274). The experimental protocols were established according to the ethical guidelines of the Helsinki Declaration. Written informed consent was obtained from individual or guardian participants. All methods were carried out in accordance with guidelines and regulations developed by UK Biobank. Data usage was approved by the Human Ethical Committee of the West China Hospital of Sichuan University (2023–1829).
This study included 415,147 participants after excluding those who withdrew from the UK Biobank study (n = 242), participants with dementia (n = 177) at baseline, participants without available data on biological age (n = 82,089), non-Caucasians (n = 3486), and individuals whose missing covariates were greater than 20% (n = 1756). The participants were divided into three groups according to their baseline status (Supplementary eFigure 1), as follows: Group 1: Participants without CMD (n = 373,400); Group 2: participants with at least one CMD (n = 41,747); and Group 3: all participants (n = 415,147).
Assessment of CMDs, dementia, and all-cause mortality
Outcomes were ascertained using hospital records containing data on admissions and diagnoses obtained from the Hospital Episode Statistics for England, the Scottish Morbidity Record data for Scotland, and the Patient Episode Database for Wales. Diagnoses were recorded using the International Classification of Diseases–10th revision (ICD-10) coding system. The primary outcomes of this study were CMDs (stroke, IHD, or T2D), all-cause dementia (Alzheimer’s disease, vascular dementia, and other causes), and all-cause mortality. According to the ICD-10, we identified the outcomes: T2D (E11), IHD (I20–I25), stroke (I60–I69), and dementia (F00, F01, F02, F03, F05.1, G30, G31.1, and G31.8). Cases of all-cause death were identified by linking them to the national death registries. Follow-up time was calculated from the enrollment date to the earliest date of the first diagnosis of events (stroke, IHD, T2D, or dementia), death, or end of the current follow-up.
Assessment of biological ages and age accelerations
Biological age was measured using the best-validated algorithms, KDM-BA and PhenoAge, which can be implemented with 12 blood chemistry traits, systolic blood pressure, and lung function data in the UK Biobank (12–14). Briefly, KDM-BA was computed from systolic blood pressure, forced expiratory volume in 1 s (FEV1), and seven other blood chemistry traits (albumin, alkaline phosphatase, creatinine, C-reactive protein, blood urea nitrogen, glycated hemoglobin, and total cholesterol). PhenoAge was computed from nine blood chemistries; four overlapped with KDM-BA (albumin, alkaline phosphatase, creatinine, C-reactive protein, glucose, mean cell volume, red cell distribution width, white blood cell count, and lymphocyte proportion). Two biological ages were constructed for different purposes. KDM-BA was computed from an algorithm derived from a series of regressions of nine individual biomarkers on chronological age in the reference population to quantify the decline in system integrity (28); PhenoAge was computed from an algorithm derived from multivariate analysis of mortality hazards to estimate the risk of death (29). Details of the selected traits and algorithms are provided in the Supplementary materials.
Assessment of life’s essential 8 score
The LE8 score was calculated to assess cardiovascular health, including four biological (blood lipids, blood glucose, blood pressure, and body mass index) and four behavioral (smoking status, physical activity, diet, and sleep) targets (26). Detailed algorithms for calculating LE8 scores have been published and are provided in the Supplementary materials.
Measurements of covariates
Age, sex, educational status, income, sleep duration, diet, drinking status, smoking status, physical activity, body mass index, systolic pressure, diastolic pressure, total cholesterol, high-density lipoprotein cholesterol, low-density lipoprotein cholesterol, triglycerides, and blood glucose were included as covariates to identify potential confounding factors.
Statistical analysis
Parametric and non-parametric continuous variables are reported as medians (25th, 75th) or means ± standard deviations and were compared using analysis of variance or Mann–Whitney U test, respectively. Categorical variables are reported as n (%) and were compared using the chi-squared test. A total of 1.3% of covariates were missing, with income being the most significantly missing at 4.3%. Missing covariables were imputed with multivariate imputation using a chained equation (30). After 10 iterations, we generated 5 imputed datasets. Utilizing the ‘quickpred’ function from the ‘mice’ package in R, we identified predictors for each variable. We adapted the original function to include a maximum of 11 predictors, with the stipulation that sex must be included.
For Analysis 1, to investigate the impact of biological aging on the risk of outcome events over time, we used the Cox proportional hazards model to examine the prospective associations between baseline accelerated aging (PhenoAge and KDM-BA) and the incidence of CMDs, dementia, and all-cause mortality among participants without any CMDs at baseline. This model is designed to analyze time-to-event data, which is the time until an event of interest occurs, and is associated with a set of covariates. It is particularly useful for handling multiple covariates and can accommodate right-censored data, where the event of interest has not occurred by the end of the study period. In this study, the groups without accelerated aging were set as the reference group to calculate the hazard ratio (HR) and 95% confidence interval (CI) for the group with accelerated aging. Additionally, we calculated the increased relative risk associated with each additional year of aging acceleration. In these models, we adjusted for covariates, including age, sex, education status, income, sleep duration, diet, drinking status, smoking status, physical activity, body mass index, systolic pressure, diastolic pressure, total cholesterol, high-density lipoprotein cholesterol, low-density lipoprotein cholesterol, triglycerides, and blood glucose. Sensitivity analyses were conducted among individuals with >2 years of follow-up to avoid reverse causation and those without cardiovascular disease at baseline.
For Analysis 2, among participants with at least one CMD at baseline, Cox proportional hazard models were used to analyze the prospective associations between baseline biological aging and the incidence of dementia and all-cause mortality. Furthermore, the association between baseline biological aging and CMM incidence was examined in patients with a first CMD (T2D, IHD, or stroke). Similar subgroup and sensitivity analyses were performed. All Models were adjusted for these covariates.
For Analysis 3, based on the assumption that CMD or CMM is a crucial mediator between accelerated aging and dementia or death, we analyzed the degree to which CMD or CMM mediated this association using mediation analysis with structural equation modeling (31). Mediation Analysis is used to investigate the indirect effect of an independent variable (X) on a dependent variable (Y) through one or more mediator variables (M). It begins by analyzing the impact of X on M, then the impact of M on Y, and finally the total effect of X on Y. The mediating effect was calculated as the estimated indirect effect size divided by the estimated total effect size. The significance of the indirect effect is determined by constructing 95%CI or conducting hypothesis tests, and the results are shown as adjusted HRs and mediated proportions. For bootstrapping, 1000 iterations were performed to estimate the 95% bias-corrected confidence interval. The mediation percentage was calculated as log (estimated indirect effect)/log (estimated total effect).
For Analysis 4, to explore the potential factors that affect biological aging, we tested the cross-sectional associations of the LE8 score and each factor with the odds ratio (OR) of KDM-BA and PhenoAge biological aging at baseline using multivariate logistic regression and linear regression models. The relationships between the LE8 score and the KDM-BA and PhenoAge accelerations were analyzed in different age groups and sexes. All models were adjusted for sex, age, income, education, and eight LE8 factors. To visualize the changing trends in biological aging, chronological age, and LE8 score, 3D mesh plots were drawn using SigmaPlot 12.5 software with LOESS smoothing. Population attributable risk was calculated for LE8 and each individual component. All metrics were set as binary variables and simultaneously included in the model. When LE8 scores ≥80 or each component scores 100, these metrics were defined as the low-risk category.
All statistical analyses were performed using the R software 4.1.0 (Vienna, Austria). Statistical significance was set at p < 0.05 for all tests.
Results
Baseline characteristics and biological ages
The baseline characteristics of the groups are presented in Table 1. Among the 415,147 individuals (Group 3), the average age was 56.5 years (46.3% male). Group 1 included 373,400 individuals without baseline CMD; Group 2 comprised 41,747 individuals with ≥CMD. Group 2 had a greater value or likelihood of cardiometabolic risk factors, including low physical activity, unhealthy sleep, smoking, high blood pressure, high lipids, and high glucose levels than Group 1.
At baseline, Group 1 had a lower biological age (48.9–50.6 vs. 54.9–58.6 years) and ratio of accelerated aging (11.4–11.8% vs. 20.7–31.2%) than Group 2, regardless of the KDM-BA or PhenoAge algorithms (Table 1). Chronological age correlated highly with biological age (Pearson coefficient: 0.75 for KDM-BA and 0.85 for PhenoAge) in all participants. Biological ages and age acceleration calculated by KDM-BA and PhenoAge had significant correlations (Pearson coefficient: 0.77 and 0.38, respectively).
Analysis 1: Association of biological aging with CMD, CMM, dementia, and mortality in CMD-free individuals
During an average of 11.7 years of follow-up, 35046 (9.4%), 3914 (1.0%), and 3722 (1.0%) CMD-free individuals developed CMD, CMM, and dementia, respectively, while 20778 (5.6%) died. In the fully adjusted Cox proportional hazards model (Table 2; Figure 1), CMD-free individuals with accelerated aging had greater risk of any CMD (KDM-BA, HR 1.456, 95%CI 1.414–1.500; PhenoAge, HR 1.404, 95%CI 1.366–1.444), CMM (KDM-BA, HR 1.952, 95%CI 1.802–2.114; PhenoAge, HR 1.738, 95%CI 1.612–1.874), all-cause dementia (KDM-BA, HR 1.243, 95%CI 1.121–1.378; PhenoAge, HR 1.212, 95%CI 1.106–1.329), and all-cause mortality (KDM-BA, HR 1.821, 95%CI 1.753–1.892; PhenoAge, HR 2.047, 95%CI 1.980–2.116). This study suggests that, in an ideal scenario, reversing the aging process could potentially prevent 31% of CMD, 49% of CMM, 19% of dementia, and 51% of mortality events. Moreover, accelerated aging was associated significantly with diabetes, ischemic heart disease, and stroke (p < 0.001). These associations were weaker in the older adults and males (P for interaction <0.05; Supplementary eTables 4–7). In the sensitivity analyses, participants with <2 years of follow-up or any cardiovascular disease were excluded, and the association between biological aging and these adverse events was consistent (p < 0.001; Supplementary eTables 8–9). Similar analyses and results for age acceleration were presented.
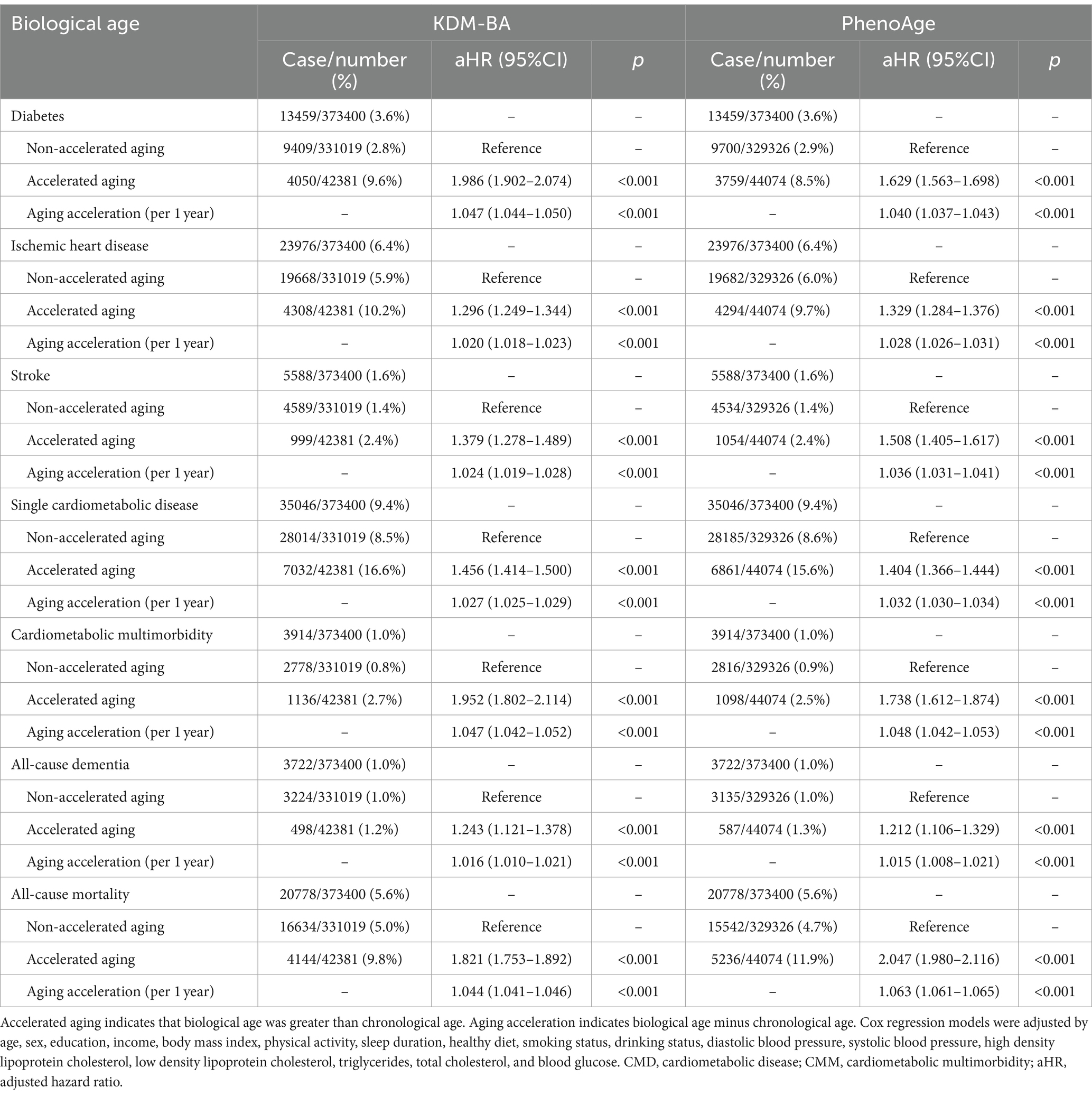
Table 2. Accelerated aging was significantly associated with CMD, CMM, dementia, and mortality among individuals who were free of CMD at baseline.

Figure 1. The panoramic trajectory of accelerated aging: from CMD to CMM, eventually developing into dementia and death. (A,B) Summary and comparison of the risks of dementia and death for individuals without CMD (Analysis 1), with CMD (Analysis 2), and with CMM (Analysis 3) are presented, respectively. Cox proportional hazards models were conducted to examine the prospective associations. (C,D) Mediation effect of CMM on relationship of accelerated aging with dementia and mortality. Indirect effect indicates the mediating effect of CMM on association between accelerated aging, dementia and mortality. Direct effect indicates total effect subtracted indirect effect. Model adjustment is the same as Table 2. The percentage was calculated by log (estimated indirect effect)/log (estimated total effect). CI, confidence interval; CMD, cardiometabolic disease; CMM, cardiometabolic multimorbidity; aHR, adjusted hazards ratio.
Analysis 2: Association of biological aging with CMM, dementia, and mortality in CMD individuals
During the average of 11.2 years follow-up, 5686 (15.5%) and 1130 (3.1%) cases of single baseline CMD developed CMM and dementia, respectively, while 5386 (14.7%) died; in those individuals, accelerated aging had adjusted HR of 1.489 (KDM-BA, 95%CI 1.338–1.656) and 1.488 (PhenoAge, 95%CI 1.348–1.643) for CMM, adjusted HRs of 1.493 (KDM-BA, 95%CI 1.146–1.943) and 1.195 (PhenoAge, 95%CI 0.940–1.519) for all-cause dementia, and adjusted HRs of 1.951 (KDM-BA, 95%CI 1.736–2.193) and 1.985 (PhenoAge, 95%CI 1.774–2.223) for all-cause mortality, respectively (Figures 1, 2). In individuals with baseline CMM, accelerated aging was independently associated with dementia (HR: 1.493 and 1.595, respectively; p < 0.001) and mortality (HR: 1.951 and 1.985, respectively; p < 0.001). These results underscore that in individuals with CMD, the detrimental effects of accelerated aging may be magnified, significantly increasing their risk of dementia by approximately half and doubling their risk of mortality. These associations were weaker in the older adults or males (p < 0.001; Supplementary eTables 11–13) and participants with follow-up of >2 years (Supplementary eTable 14). Accelerated aging was associated significantly with CMM, all-cause dementia, and all-cause mortality in individuals with DM, IHD, or stroke (Supplementary eTable 15).
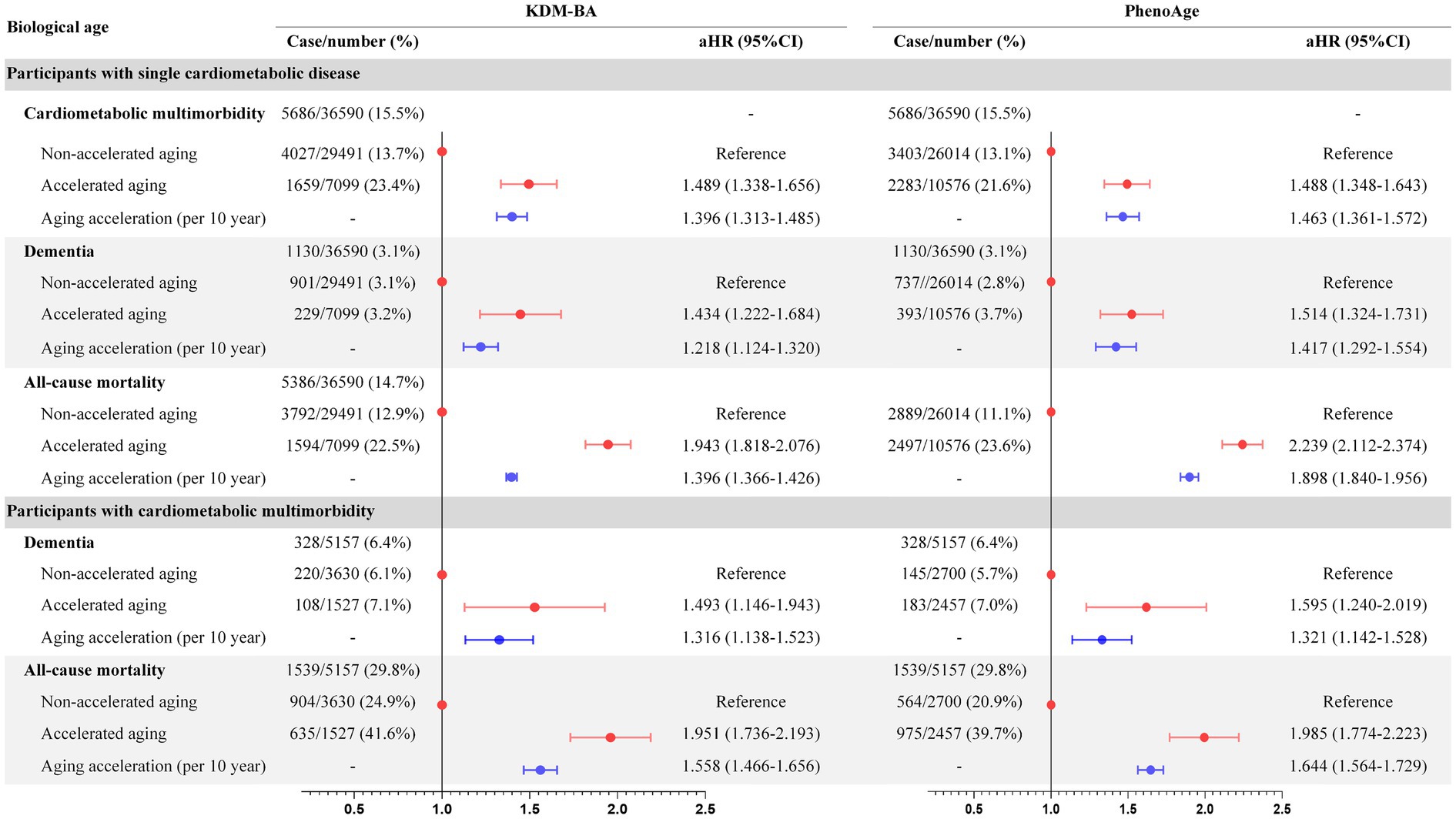
Figure 2. Association of biological aging with CMM, dementia, and mortality in CMD individuals. CMD, cardiometabolic disease; CMM, cardiometabolic multimorbidity.
Analysis 3: Effect of CMM on the relationship of biological aging with dementia and mortality
In structural equation modeling (Supplementary eTables 16–20), the indirect effects of accelerated aging on all-cause dementia mediated by CMM were 13.7% (KDM-BA, aHR 1.044) and 21.6% (PhenoAge, aHR 1.078); the indirect effects on all-cause mortality were 4.7% (KDM-BA, aHR 1.037) and 5.2% (PhenoAge, aHR 1.054). Significant mediating effects of CMD on biological aging were observed for dementia (intermediate value 22.9–37.9%, p < 0.001) and mortality (intermediate value 7.8–9.4%, p < 0.001; Figure 2). This statistical value suggests that one-third of the dementia caused by accelerated aging can be explained by CMDs. However, while CMDs are also a significant mediator in the relationship between accelerated aging and mortality, the mediation effect size is notably lower, approximately 5%, compared to dementia. Diabetes mellitus, IHD, and stroke also significantly mediated dementia and mortality. To emphasize the risks of dementia and death for individuals without CMD, with CMD, and with CMM, respectively, a comprehensive presentation and comparison were shown in Figure 1. According to the results, the HRs for dementia and death were higher in individuals with CMD or CMM compared to those without CMD. This indicated that accelerated aging had a stronger association with health outcomes in the context of CMD.
Analysis 4: Relationship between the life essential 8 score and biological aging
Individuals with low LE8 score (0–49) had adjusted OR of 16.615 (95%CI: 15.589–17.709) and 4.190 (95%CI: 4.021–4.365) for accelerated aging as calculated by KDM-BA and PhenoAge (Supplementary eTable 21), respectively, compared to those with a high score (80–100). These associations were weaker in the older adults or males (p < 0.001; Supplementary eTable 22). Moreover, 3D mesh graph visualization showed that participants with lower LE8 scores or chronological ages had greater aging acceleration and a greater likelihood of accelerated aging (Figure 3; Supplementary eFigure 3). Each LE8 component was an independent factor of biological aging (Supplementary eTable 23). The LE8 population attributable risk score was 0.79 (95%CI: 0.78–0.80) and 0.43 (95%CI: 0.41–0.45) for KDM-BA and PhenoAge accelerated aging, respectively; among the LE8 components, blood pressure and blood lipids were the main contribution factors to accelerated aging algorithm (Figure 3). This implies that among numerous aging risk factors, LE8 carries a very high weight. The data can still suggest that if the cardiovascular health indicated by LE8 is protected, it may be possible to prevent aging in 80% of the population at risk.
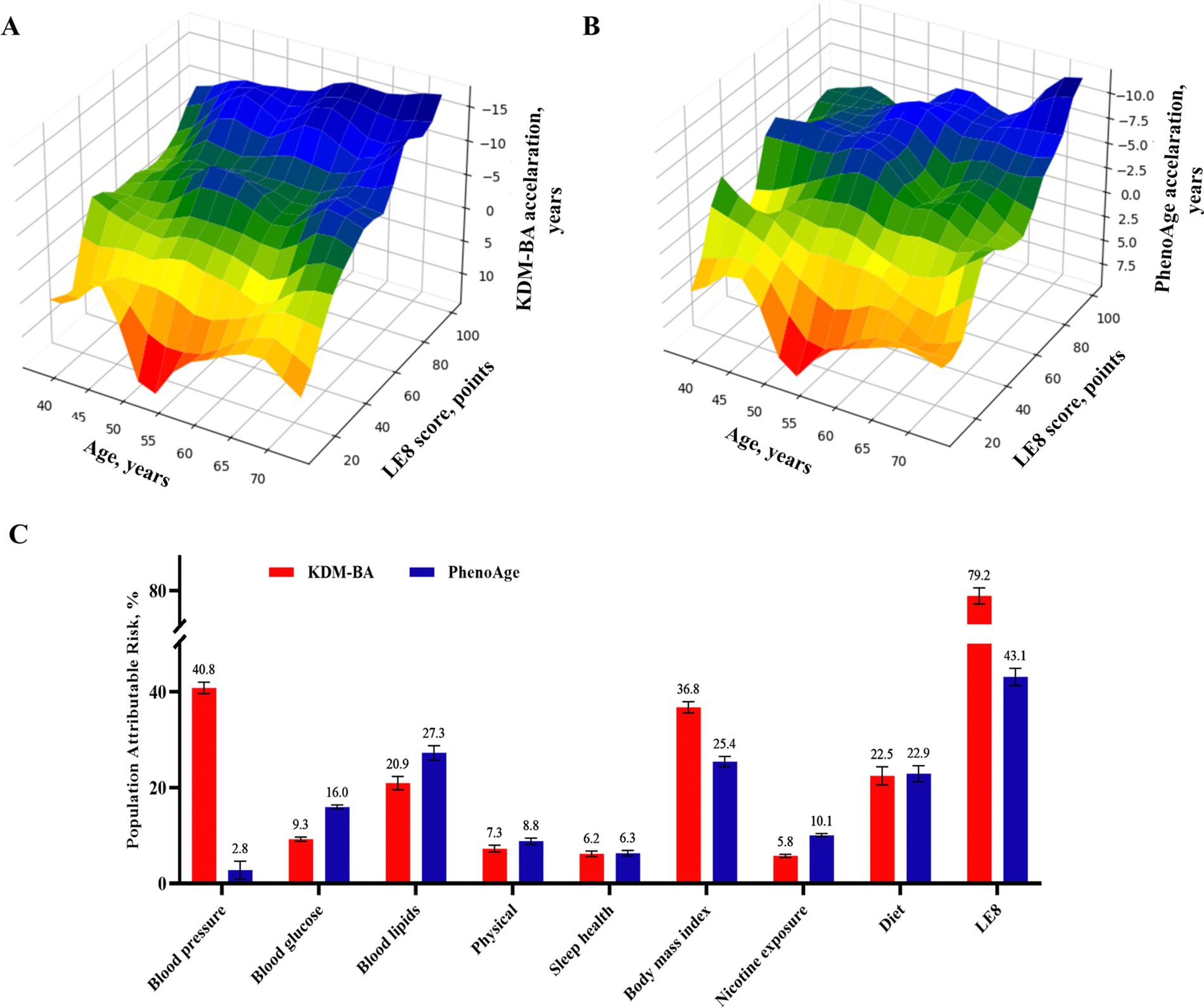
Figure 3. Dose–response relationship and crucial contribution of LE8 to biological aging. (A,B) 3D Mesh Plots visualizing differences between accelerated age, chronological age, and LE8 score. (C) Populations Attributable risk of LE8 score (≥80 vs. <80) and each risk factor for accelerated aging. LE8, life’s essential 8.
Discussion
Using data from a community-based large-sample cohort from the UK Biobank, we found accelerated aging elevated risk of CMD by 40–45%, risk of CMM by 74–95%, risk of dementia by 21–24%, and risk of mortality by 82–105% in individuals without any CMD. While, for individuals with CMD, accelerated aging had about 50 and 100% higher risk of dementia and mortality, respectively. Regardless of baseline CMD status, individuals with biological aging had a significantly increased risk of all-cause dementia and mortality, partly (5–38%) mediated by CMD or CMM. These results suggest that aging plays a substantial role in the occurrence, progression, and prognosis of CMM, and that inhibiting the acceleration of aging may improve the adverse outcomes of dementia and death in one-third and one-half of the population, respectively. Considering biological aging is a more valuable intervention target than treating any single disease, we found the cardiovascular health assessment tool LE8 recommended by the AHA has a 41–80% population-attributed risk for biological aging, indicating cardiovascular health primordial prevention is an important strategy for suppressing biological aging.
Age acceleration, the epigenetic clock measured using whole blood DNA methylation, and shortened leukocyte telomere length are CMD risk factors and related positively to increased CVD (32, 33). Epigenetic age acceleration is a robust marker of myocardial infarction and cardiovascular disease mortality risk (34). Leukocyte telomere length negatively correlates with an increased risk of myocardial infarction and cardiovascular death (35). This study expands on the discovery that biological age acceleration measured using blood biochemical parameters is associated with CMD (including diabetes, stroke, and IHD) and CCM.
Diabetes mellitus was associated with a 37% increase in the leukocyte telomere-length attrition risk (35). Disrupted regulation of sirtuins and the mammalian target of rapamycin, which is related to longevity, induces abnormal glucose metabolism (36, 37). Therefore, a bidirectional relationship may exist between diabetes and biological aging. We found aging promotes CMD development toward CCM, especially stroke, and the transition to comorbidities.
Aging decreases life expectancy; biological age is a stronger predictor of age-related adverse events than chronological age, making it a valuable tool in precision medicine (29). Consistent with previous results, the acceleration of phenotypic age was associated with all-cause mortality in patients with diabetes. We also found individuals with other CMDs show a significant association between biological aging and mortality. Age is an important factor for cognitive decline; brain aging is an important initiating factor for dementia (38–40). However, no strong evidence exists regarding accelerated epigenetic aging associated with dementia or mild cognitive impairment (41). Herein, phenotypic age acceleration was a robust predictive factor for dementia independent of chronological age and traditional risk factors in participants with different CMD statuses.
The effects of CMD and CMM on dementia and mortality have been validated, but studies fail to assess the effect of biological aging on different CMM transition stages, including the transformation from CMD-free to CMD, then CMM, dementia, and death. We applied a marginal structural approach to test the indirect effects of accelerated aging on all-cause dementia and CMM-mediated mortality. We suggest that CMM is a critical link in age-related adverse events.
Several factors explain the relationship between biological aging and CMM. Cardiometabolic disorders, including arterial hypertension, dyslipidemia, insulin resistance, and hyperglycemia, share common pathophysiological mechanisms with aging (4, 42). For example, insulin resistance is mechanically induced by inflammation, oxidative stress, immune disorders, which are also complex biological processes involved in aging (43–45). Additionally, the blood vessels themselves undergo age-related changes, such as stiffening of the arteries, which can increase the risk of hypertension and cardiovascular diseases (46). Certain genetic predispositions and epigenetic modifications that accumulate over time can influence the rate of biological aging and the susceptibility to CMM (47). Moreover, Aging manifests as an impairment of the reserve and recovery ability of organs, tissues, and cells; thus, aging individuals are more susceptible to adverse cardiometabolic substrates, leading to progression from sustained chronic injury to CMD and comorbidities (42, 48). Demonstrating these potential mechanisms may promote the clinical application of biological aging concepts and contribute to precise individual health assessments, identifying high-risk populations before disease onset and implementing early intervention strategies.
Studies have stressed the protective effects of behavioral and environmental factors on vascular aging, including PM2.5, caloric restriction and fasting, physical exercise, smoking cessation, healthy diet, and low sodium intake (49–52). The American Heart Association included eight modifiable risk factors to optimize cardiovascular health when updating the LE8 (26). However, the significance of LE8 adherence for preventing biological aging remains unclear. Our results showed that 8 factors were independently associated with accelerated aging. Blood pressure, blood lipid levels, BMI, and diet were the main accelerated aging factors; LE8 adherence may counter aging. These results emphasize the importance of new perspectives for cardiovascular health prevention. Given this, future studies should design randomized controlled trials to test comprehensive intervention measures targeting the LE8 score. And during the follow-up process, continuous measurement of phenotypic age, telomere length, CMD events and death should be employed to verify the effectiveness and effect size of cardiovascular health in inhibiting the aging process. Additionally, preclinical experiments are equally important and may provide novel insights into how to inhibit the increase of phenotypic age at the molecular and cellular levels, as well as counteract the onset and progression of CMD caused by aging.
The study limitations include, first, the lack of repeated measurements of biological age, possibly explaining the significance of biological aging in the CMM disease trajectory. This defect is also limited to cross-sectional rather than prospective analysis of biological age’s influencing factors. Second, although the UK Biobank cohort enrolled the largest possible population, the response rate was only 5%; most were Caucasians, and the health status of these individuals was better than that of the general population (53). The CMM incidence rate was significantly lower than that in the Chinese cohort (0.9% vs. 6.3%) (5). Therefore, the universality of the results must be verified. Third, although potential confounding factors were corrected as much as possible, residual confounding factors may have affected the results. For example, the presence of other chronic diseases may affect the speed of aging and the occurrence of age-related diseases. The interaction between genes and environmental factors may affect aging, but this interaction is often complex and difficult to measure (47). Fourth, our models were adjusted for numerous cardiometabolic risk factors; biological age may have been affected by subclinical CVD at baseline; therefore, we cannot exclude the possibility of reverse causation. Fifth, although adjustments were made for many cardiometabolic risk factors, baseline biological age may be affected by subclinical CVD. In addition, diabetes promotes aging, meaning reverse causality is possible. Sixth, this study did not use epigenetic DNA methylation or telomere length to assess biological aging. Finally, considering that we conducted multiple tests in the main analysis (two measurements of biological age and multiple outcomes), our results should be interpreted cautiously and replicated in other study populations.
Conclusion
Biological aging measured by the blood biochemical index was a strong risk marker for CMD, CMM, dementia, and death, independent of chronological age and other traditional cardiovascular risk factors. Notably, biological aging involved whole trajectory of CMM from a disease-free state to single CMD, and subsequently to CMM, and ultimately to dementia and death. Given the robust correlation and significant impact of LE8 on biological aging, this study offers avenues for future research, including the design of comprehensive interventions targeting LE8 in clinical trials to verify the effects of cardiovascular health interventions on inhibiting aging, CMD, and related adverse events. This also encompasses preclinical experiments aimed at developing new anti-aging targeted drugs from the perspective of cardiovascular health, to suppress the critical trajectory of biological aging that leads to CMM, dementia, and mortality.
Data availability statement
The original contributions presented in the study are included in the article/Supplementary material, further inquiries can be directed to the corresponding authors.
Ethics statement
The studies involving humans were approved by Ethics approval of UK Biobank study was approved by NHS National Research Ethics Service (16/NW/0274). Data usage was approved by the Human Ethical Committee of the West China Hospital of Sichuan University (2023–1829). The studies were conducted in accordance with the local legislation and institutional requirements. The participants provided their written informed consent to participate in this study.
Author contributions
YH: Conceptualization, Data curation, Methodology, Writing – original draft, Writing – review & editing. YJ: Methodology, Writing – original draft, Data curation, Investigation. YzL: Data curation, Investigation, Methodology, Writing – review & editing. ZW: Data curation, Methodology, Software, Writing – review & editing. YiL: Data curation, Investigation, Software, Writing – review & editing. XL: Methodology, Resources, Supervision, Writing – review & editing. QZ: Conceptualization, Resources, Supervision, Validation, Visualization, Writing – review & editing. DL: Conceptualization, Supervision, Writing – original draft, Writing – review & editing.
Funding
The author(s) declare that financial support was received for the research, authorship, and/or publication of this article. This work was supported financially by grants from Sichuan Science and Technology Program (No. 2023YFS0027, 2023YFS0240, 2023YFS0074, 2023NSFSC1652), Sichuan Provincial Health Commission (No. 2023-101, 2024-102), Postdoctor Research Fund of West China Hospital, Sichuan University (No. 2024HXBH067), CDHT Health Bureau (No. 2024004, 2024005), and Health Commission of Chengdu (No. 2019012).
Acknowledgments
The authors appreciate the participants for their participation and contribution to this research in the UK Biobank study. This research has been conducted using the UK Biobank resource under application number 112111.
Conflict of interest
The authors declare that the research was conducted in the absence of any commercial or financial relationships that could be construed as a potential conflict of interest.
Publisher’s note
All claims expressed in this article are solely those of the authors and do not necessarily represent those of their affiliated organizations, or those of the publisher, the editors and the reviewers. Any product that may be evaluated in this article, or claim that may be made by its manufacturer, is not guaranteed or endorsed by the publisher.
Supplementary material
The Supplementary material for this article can be found online at: https://www.frontiersin.org/articles/10.3389/fpubh.2024.1423016/full#supplementary-material
Footnotes
References
1. Di Angelantonio, E, Kaptoge, S, Wormser, D, Willeit, P, Butterworth, AS, Bansal, N, et al. Association of Cardiometabolic Multimorbidity with Mortality. JAMA. (2015) 314:52–60. doi: 10.1001/jama.2015.7008
2. Roth, GA, Mensah, GA, Johnson, CO, and Addolorato, G. Global burden of cardiovascular diseases and risk factors, 1990-2019: update from the GBD 2019 study. J Am Coll Cardiol. (2020) 76:2982–3021. doi: 10.1016/j.jacc.2020.11.010
3. Han, Y, Hu, Y, Yu, C, Guo, Y, Pei, P, Yang, L, et al. Lifestyle, cardiometabolic disease, and multimorbidity in a prospective Chinese study. Eur Heart J. (2021) 42:3374–84. doi: 10.1093/eurheartj/ehab413
4. Singh-Manoux, A, Fayosse, A, Sabia, S, Tabak, A, Shipley, M, Dugravot, A, et al. Clinical, socioeconomic, and behavioural factors at age 50 years and risk of cardiometabolic multimorbidity and mortality: a cohort study. PLoS Med. (2018) 15:e1002571. doi: 10.1371/journal.pmed.1002571
5. Han, Y, Hu, Y, Yu, C, Sun, D, Pang, Y, Pei, P, et al. Duration-dependent impact of cardiometabolic diseases and multimorbidity on all-cause and cause-specific mortality: a prospective cohort study of 0.5 million participants. Cardiovasc Diabetol. (2023) 22:135. doi: 10.1186/s12933-023-01858-9
6. Zhang, D, Tang, X, Shen, P, Si, Y, Liu, X, Xu, Z, et al. Multimorbidity of cardiometabolic diseases: prevalence and risk for mortality from one million Chinese adults in a longitudinal cohort study. BMJ Open. (2019) 9:e024476. doi: 10.1136/bmjopen-2018-024476
7. Gerdts, E, and Regitz-Zagrosek, V. Sex differences in cardiometabolic disorders. Nat Med. (2019) 25:1657–66. doi: 10.1038/s41591-019-0643-8
8. Wang, JC, and Bennett, M. Aging and atherosclerosis: mechanisms, functional consequences, and potential therapeutics for cellular senescence. Circ Res. (2012) 111:245–59. doi: 10.1161/CIRCRESAHA.111.261388
9. Zhernakova, DV, Sinha, T, Andreu-Sánchez, S, Prins, JR, Kurilshikov, A, Balder, JW, et al. Age-dependent sex differences in cardiometabolic risk factors. Nat Cardiovasc Res. (2022) 1:844–54. doi: 10.1038/s44161-022-00131-8
10. Kennedy, BK, Berger, SL, Brunet, A, Campisi, J, Cuervo, AM, Epel, ES, et al. Geroscience: linking aging to chronic disease. Cell. (2014) 159:709–13. doi: 10.1016/j.cell.2014.10.039
11. Hägg, S, Belsky, DW, and Cohen, AA. Developments in molecular epidemiology of aging. Emerg Top Life Sci. (2019) 3:411–21. doi: 10.1042/ETLS20180173
12. Klemera, P, and Doubal, S. A new approach to the concept and computation of biological age. Mech Ageing Dev. (2006) 127:240–8. doi: 10.1016/j.mad.2005.10.004
13. Levine, ME. Modeling the rate of senescence: can estimated biological age predict mortality more accurately than chronological age? J Gerontol A Biol Sci Med Sci. (2013) 68:667–74. doi: 10.1093/gerona/gls233
14. Liu, Z, Kuo, PL, Horvath, S, Crimmins, E, Ferrucci, L, and Levine, M. A new aging measure captures morbidity and mortality risk across diverse subpopulations from NHANES IV: a cohort study. PLoS Med. (2018) 15:e1002718. doi: 10.1371/journal.pmed.1002718
15. Graf, GH, Crowe, CL, Kothari, M, Kwon, D, Manly, JJ, Turney, IC, et al. Testing Black-White disparities in biological aging among older adults in the United States: analysis of DNA-methylation and blood-chemistry methods. Am J Epidemiol. (2022) 191:613–25. doi: 10.1093/aje/kwab281
16. Gonzales, MM, Garbarino, VR, Pollet, E, Palavicini, JP, Kellogg, DL Jr, Kraig, E, et al. Biological aging processes underlying cognitive decline and neurodegenerative disease. J Clin Invest. (2022) 132:e158453. doi: 10.1172/JCI158453
17. Harada, CN, Natelson Love, MC, and Triebel, KL. Normal cognitive aging. Clin Geriatr Med. (2013) 29:737–52. doi: 10.1016/j.cger.2013.07.002
18. Livingston, G, Huntley, J, Sommerlad, A, Ames, D, Ballard, C, Banerjee, S, et al. Dementia prevention, intervention, and care: 2020 report of the lancet commission. Lancet. (2020) 396:413–46. doi: 10.1016/S0140-6736(20)30367-6
19. Dove, A, Marseglia, A, Shang, Y, Grande, G, Vetrano, DL, Laukka, EJ, et al. Cardiometabolic multimorbidity accelerates cognitive decline and dementia progression. Alzheimers Dement. (2023) 19:821–830. doi: 10.1002/alz.050473
20. Tai, XY, Veldsman, M, Lyall, DM, Littlejohns, TJ, Langa, KM, Husain, M, et al. Cardiometabolic multimorbidity, genetic risk, and dementia: a prospective cohort study. Lancet Healthy Longev. (2022) 3:e428–36. doi: 10.1016/S2666-7568(22)00117-9
21. Kaeberlein, M, Rabinovitch, PS, and Martin, GM. Healthy aging: the ultimate preventative medicine. Science. (2015) 350:1191–3. doi: 10.1126/science.aad3267
22. Fontana, L, Kennedy, BK, Longo, VD, Seals, D, and Melov, S. Medical research: treat ageing. Nature. (2014) 511:405–7. doi: 10.1038/511405a
23. Dmitrieva, NI, Gagarin, A, Liu, D, Wu, CO, and Boehm, M. Middle-age high normal serum sodium as a risk factor for accelerated biological aging, chronic diseases, and premature mortality. EBioMedicine. (2023) 87:104404. doi: 10.1016/j.ebiom.2022.104404
24. Cao, X, Yang, G, Li, X, and Fu, J. Weight change across adulthood and accelerated biological aging in middle-aged and older adults. Am J Clin Nutr. (2023) 117:1–11. doi: 10.1016/j.ajcnut.2022.10.020
25. Braunwald, E. Will primordial prevention change cardiology? Eur Heart J. (2023) 44:3307–8. doi: 10.1093/eurheartj/ehad459
26. Lloyd-Jones, DM, Allen, NB, Anderson, CAM, Black, T, Brewer, LC, Foraker, RE, et al. Life’s essential 8: updating and enhancing the American Heart Association’s construct of cardiovascular health: a presidential advisory from the American Heart Association. Circulation. (2022) 146:e18–43. doi: 10.1161/CIR.0000000000001078
27. Sudlow, C, Gallacher, J, Allen, N, Beral, V, Burton, P, Danesh, J, et al. UK biobank: an open access resource for identifying the causes of a wide range of complex diseases of middle and old age. PLoS Med. (2015) 12:e1001779. doi: 10.1371/journal.pmed.1001779
28. Hastings, WJ, Shalev, I, and Belsky, DW. Comparability of biological aging measures in the National Health and nutrition examination study, 1999-2002. Psychoneuroendocrinology. (2019) 106:171–8. doi: 10.1016/j.psyneuen.2019.03.012
29. Parker, DC, Bartlett, BN, Cohen, HJ, Fillenbaum, G, Huebner, JL, Kraus, VB, et al. Association of Blood Chemistry Quantifications of biological aging with disability and mortality in older adults. J Gerontol A Biol Sci Med Sci. (2020) 75:1671–9. doi: 10.1093/gerona/glz219
30. White, IR, Royston, P, and Wood, AM. Multiple imputation using chained equations: issues and guidance for practice. Stat Med. (2011) 30:377–99. doi: 10.1002/sim.4067
31. Stein, CM, Morris, NJ, Hall, NB, and Nock, NL. Structural equation modeling. Methods Mol Biol. (2017) 1666:557–80. doi: 10.1007/978-1-4939-7274-6_28
32. Ammous, F, Zhao, W, Ratliff, SM, Mosley, TH, Bielak, LF, Zhou, X, et al. Epigenetic age acceleration is associated with cardiometabolic risk factors and clinical cardiovascular disease risk scores in African Americans. Clin Epigenetics. (2021) 13:55. doi: 10.1186/s13148-021-01035-3
33. Huang, RC, Lillycrop, KA, Beilin, LJ, Godfrey, KM, Anderson, D, Mori, TA, et al. Epigenetic age acceleration in adolescence associates with BMI, inflammation, and risk score for middle age cardiovascular disease. J Clin Endocrinol Metab. (2019) 104:3012–24. doi: 10.1210/jc.2018-02076
34. Roetker, NS, Pankow, JS, Bressler, J, Morrison, AC, and Boerwinkle, E. Prospective study of epigenetic age acceleration and incidence of cardiovascular disease outcomes in the ARIC study (atherosclerosis risk in communities). Circ Genom Precis Med. (2018) 11:e001937. doi: 10.1161/CIRCGEN.117.001937
35. Emami, M, Agbaedeng, TA, Thomas, G, Middeldorp, ME, Thiyagarajah, A, Wong, CX, et al. Accelerated biological aging secondary to Cardiometabolic risk factors is a predictor of cardiovascular mortality: a systematic review and Meta-analysis. Can J Cardiol. (2022) 38:365–75. doi: 10.1016/j.cjca.2021.10.012
36. Wei, Y, Zhang, YJ, and Cai, Y. Growth or longevity: the TOR’s decision on lifespan regulation. Biogerontology. (2013) 14:353–63. doi: 10.1007/s10522-013-9435-6
37. Lamming, DW, Ye, L, Katajisto, P, Goncalves, MD, Saitoh, M, Stevens, DM, et al. Rapamycin-induced insulin resistance is mediated by mTORC2 loss and uncoupled from longevity. Science. (2012) 335:1638–43. doi: 10.1126/science.1215135
38. Grande, G, Qiu, C, and Fratiglioni, L. Prevention of dementia in an ageing world: evidence and biological rationale. Ageing Res Rev. (2020) 64:101045. doi: 10.1016/j.arr.2020.101045
39. Mecocci, P, Boccardi, V, Cecchetti, R, Bastiani, P, Scamosci, M, Ruggiero, C, et al. A long journey into aging, brain aging, and Alzheimer’s disease following the oxidative stress tracks. J Alzheimers Dis. (2018) 62:1319–35. doi: 10.3233/JAD-170732
40. Raina, A, Zhao, X, Grove, ML, Bressler, J, Gottesman, RF, Guan, W, et al. Cerebral white matter hyperintensities on MRI and acceleration of epigenetic aging: the atherosclerosis risk in communities study. Clin Epigenetics. (2017) 9:21. doi: 10.1186/s13148-016-0302-6
41. Zhou, A, Wu, Z, Zaw Phyo, AZ, Torres, D, Vishwanath, S, and Ryan, J. Epigenetic aging as a biomarker of dementia and related outcomes: a systematic review. Epigenomics. (2022) 14:1125–38. doi: 10.2217/epi-2022-0209
42. Pietri, P, and Stefanadis, C. Cardiovascular aging and longevity: JACC state-of-the-art review. J Am Coll Cardiol. (2021) 77:189–204. doi: 10.1016/j.jacc.2020.11.023
43. Glass, CK, and Olefsky, JM. Inflammation and lipid signaling in the etiology of insulin resistance. Cell Metab. (2012) 15:635–45. doi: 10.1016/j.cmet.2012.04.001
44. Henriksen, EJ, Diamond-Stanic, MK, and Marchionne, EM. Oxidative stress and the etiology of insulin resistance and type 2 diabetes. Free Radic Biol Med. (2011) 51:993–9. doi: 10.1016/j.freeradbiomed.2010.12.005
45. Olefsky, JM, and Glass, CK. Macrophages, inflammation, and insulin resistance. Annu Rev Physiol. (2010) 72:219–46. doi: 10.1146/annurev-physiol-021909-135846
46. Ungvari, Z, Tarantini, S, Sorond, F, Merkely, B, and Csiszar, A. Mechanisms of vascular aging, a Geroscience perspective: JACC focus seminar. J Am Coll Cardiol. (2020) 75:931–41. doi: 10.1016/j.jacc.2019.11.061
47. la Torre, A, Lo Vecchio, F, and Greco, A. Epigenetic mechanisms of aging and aging-associated diseases. Cells. (2023) 12:12. doi: 10.3390/cells12081163
48. Hamczyk, MR, Nevado, RM, Barettino, A, Fuster, V, and Andrés, V. Biological versus chronological aging: JACC focus seminar. J Am Coll Cardiol. (2020) 75:919–30. doi: 10.1016/j.jacc.2019.11.062
49. Mozaffarian, D. Dietary and policy priorities for cardiovascular disease, diabetes, and obesity: a comprehensive review. Circulation. (2016) 133:187–225. doi: 10.1161/CIRCULATIONAHA.115.018585
50. Banks, E, Joshy, G, Korda, RJ, Stavreski, B, Soga, K, Egger, S, et al. Tobacco smoking and risk of 36 cardiovascular disease subtypes: fatal and non-fatal outcomes in a large prospective Australian study. BMC Med. (2019) 17:128. doi: 10.1186/s12916-019-1351-4
51. Lee, DC, Brellenthin, AG, Thompson, PD, Sui, X, Lee, IM, and Lavie, CJ. Running as a key lifestyle medicine for longevity. Prog Cardiovasc Dis. (2017) 60:45–55. doi: 10.1016/j.pcad.2017.03.005
52. Kong, L, Ye, C, Wang, Y, Hou, T, Zheng, J, Zhao, Z, et al. Genetic evidence for causal effects of socioeconomic, lifestyle, and Cardiometabolic factors on epigenetic-age acceleration. J Gerontol A Biol Sci Med Sci. (2023) 78:1083–91. doi: 10.1093/gerona/glad078
Keywords: biological aging, cardiometabolic multimorbidity, cardiometabolic diseases, mortality, dementia, disease trajectory
Citation: He Y, Jia Y, Li Y, Wan Z, Lei Y, Liao X, Zhao Q and Li D (2024) Accelerated biological aging: unveiling the path to cardiometabolic multimorbidity, dementia, and mortality. Front. Public Health. 12:1423016. doi: 10.3389/fpubh.2024.1423016
Edited by:
Ljiljana T. Majnaric, Josip Juraj Strossmayer University of Osijek, CroatiaReviewed by:
Lin Jiang, The University of Texas Rio Grande Valley, United StatesFrantišek Babič, Technical University of Košice, Slovakia
Copyright © 2024 He, Jia, Li, Wan, Lei, Liao, Zhao and Li. This is an open-access article distributed under the terms of the Creative Commons Attribution License (CC BY). The use, distribution or reproduction in other forums is permitted, provided the original author(s) and the copyright owner(s) are credited and that the original publication in this journal is cited, in accordance with accepted academic practice. No use, distribution or reproduction is permitted which does not comply with these terms.
*Correspondence: Qian Zhao, emhhb3FpYW5Ad2Noc2N1LmNu; Dongze Li, bGlkb25nemVAd2Noc2N1LmNu