- 1Department of Medical Informatics, School of Public Health, Jilin University, Changchun, China
- 2Shenzhen Health Development Research and Data Management Center, Shenzhen, China
- 3Department of Software Technology, School of Computer Science and Software Engineering, Shenzhen Institute of Information Technology, Shenzhen, China
- 4Department of Management Science, College of Management, Shenzhen University, Shenzhen, China
Background: The early years of life are critical for gross motor development (GMD). This study utilized decision tree modeling to examine the influences on gross motor development in children under the age of 3 years and to rank the key factors affecting their development.
Methods: Based on randomized stratified sampling, 9,507 children aged 0–3 years in Shenzhen were included in this study. The Ages and Stages Questionnaires (ASQ) were utilized for the assessment of gross motor development. The chi-square test was used to compare groups, and variables were screened using univariable and multivariable regression analyses. Decision tree modeling was employed to rank the importance of statistically significant variables.
Results: The research found a prevalence of gross motor developmental delay of 1.41% among the respondents. The accuracy of the decision tree model is 70.96%. The results demonstrated a strong correlation between seven variables affecting the gross motor development of children, which were ranked based on importance: age, whether to provide supplementary food, average time spent interacting with children, family type, feeding method, mode of delivery, and birth order.
Conclusion: The risk of gross motor developmental delay increases with age. Furthermore, supplementary food and interacting with other children are critical factors in improving children’s GMD delay. It is therefore imperative to enhance the monitoring of children’s gross motor skills through regular developmental assessments that detect potential GMD delays. Moreover, family type, feeding method, mode of delivery, and birth order were also predictive factors of GMD delay.
1 Introduction
Building gross motor skills (GMS) is an essential component of the motor growth of the body. Gross movements result from the actions of large muscle groups, whereas fine movements happen through the actions of smaller muscles or muscle groups. The combination of gross and fine movements constitutes human behavior of practical significance. Important gross movements in childhood include various infant reflexes (e.g., stepping and crawling reflexes), autonomous mobility (e.g., creeping and rolling), basic motor skills (e.g., running and jumping), and object manipulation abilities (e.g., throwing and kicking) (1). The initial 1,000 days of life are crucial for children’s growth and development, significantly influencing their future (2). Moreover, GMS will facilitate experience, discovery, learning, and development during this life stage. In this regard, GMS is positively correlated with children’s physical development, cognitive development (3, 4), cardiometabolic risk (5), and social skills (6).
Among the typical gross motor evaluation instruments [e.g., Alberta Infant Motor Scale (AIMS) (7), Bruininks–Oseretsky Test of Motor Proficiency-2 (BOT-2), Bayley Scale of Infant and Toddler Development-III (Bayley-III), Movement Assessment Battery for Children-2 (MABC-2), McCarron Assessment of Neuromuscular Development (MAND), Neurological Sensory Motor Developmental Assessment (NSMDA), Peabody Developmental Motor Scales-2 (PDMS-2), and Test of Gross Motor Development-2 (TGMD-2)] that are widely employed in the detection, diagnosis, and assessment of pediatric movement disorders (8), the Bayley-III is the most commonly utilized instrument for diagnosing developmental delay in children under the age of 3 years. Nevertheless, several challenges exist in the implementation of this tool in regions and countries with limited resources. As an effective solution, the Ages and Stages Questionnaire (ASQ) serves as a simple and cost-effective screening tool that allows parents or caregivers to complete (9). The ASQ has been extensively used in various countries and has emerged as a commonly adopted screening instrument for child development globally (10–12). Using the ASQ, a Peruvian study assessed five major areas of child development: communication skills, gross motor abilities, fine motor skills, problem-solving capabilities, and personal–social competencies (13). A study from Argentina employed the Ages and Stages Questionnaires—Third Edition (ASQ-3) and found that 19.5% of the surveyed children were at risk for neurodevelopmental conditions. The ASQ-3 demonstrated congruence in psychometric characteristics when compared to the National Screening Test (14).
To devise effective strategies for preventing gross motor developmental delay, it is essential to comprehend the underlying risk factors. Research involving 205 toddlers indicates that encouraging extended and regular nighttime sleep, in conjunction with an earlier bedtime, could enhance the development of gross motor skills (15). Further research implies a positive correlation between gross motor skill proficiency and physical fitness in Chilean children aged 4–6 years (16). A cross-sectional descriptive analysis reveals that preschool children in South Africa, regardless of their economic backgrounds, exhibit high levels of gross motor skill proficiency (17). Furthermore, existing literature indicates that GMS is positively associated with physical activity and fitness (18), perceived competence (19), and body weight (20). Another cross-sectional investigation found no correlation between physical activity levels and Australian toddlers, highlighting the importance of a specific age for promoting physical activity (21).
Although several studies have probed the factors associated with developmental delay, each presents its own limitations. The majority of studies primarily examined cohorts before the year 2000, and the geographic scope of data collection was predominantly constrained, which may diminish the generalizability of their findings (22–26). Moreover, few studies have thoroughly investigated the current state of gross motor development in Shenzhen for children aged 0–3 years.
Bronfenbrenner’s ecological theory offers a framework for analyzing children’s gross motor developmental delays and their association with potential risk factors. It assumes that children’s development is the result of environmental influences and that their interaction with the environment is marked by complexity and multidimensionality (27). The environment in which children develop is divided into four levels: micro, mediated, outer, and macro. Each level contains positive or negative factors that influence children’s growing performance (28). Children are affected by direct interactions and the broader environment, including family, community, and society (29). The Dynamic Systems Theory provides a framework that defines behavior as an emergent outcome of a self-regulating, multifaceted system that evolves over time, underscoring the interplay among various elements as the impetus for behavioral and developmental changes (30). This study explored the influencing factors of GMS at the individual and family levels. A decision tree was used to analyze the importance of its influencing factors. The findings of our study offer an empirical reference for the prevention and control of gross motor developmental delay.
2 Methods
2.1 Study population and sample
The study involved 9,507 infants and toddlers aged 0–3 years who received health examinations at the Shenzhen Social Health Center’s Child Health Clinic between August 2021 and June 2022. The survey was conducted by pediatric healthcare professionals who had undergone specialized training and met the qualification criteria. The project personnel monitored the evaluation data throughout the entire process, while the questionnaire and data compiled by the data quality control personnel were reviewed on the same day. All participating parents provided basic information and completed the ASQ-3 questionnaire.
Exclusion criteria included the following: (1) children exhibiting developmental delays or severe hereditary conditions; (2) caregivers with cognitive deficits or emotional issues; (3) children over 36 months or those who refused informed consent; and (4) the involvement of non-primary caregivers during the evaluation.
2.2 Measures
We utilized electronic resources such as CNKI and PubMed to review the domestic and international literature on the developmental levels of children aged 0–3 years and their influencing factors and screened possible influencing factors for inclusion in the study based on previous reports in the literature.
2.2.1 Dependent variable
GMS of infants and toddlers aged 0–3 years were screened and assessed using the ASQ-3. The Ages and Stages Questionnaire—Third Edition—Gross Motor domain (ASQ-3-GM) score is valid to identify gross motor developmental delay in young children (12). Scholars introduced the ASQ-3 to China, created the ASQ-Chinese (ASQ-C), and collaborated with the authors of the ASQ system to examine the national norms and psychometric properties of Chinese children aged 1–66 months. The ASQ-C demonstrated a sensitivity of 87.50% and a specificity of 84.48% (31).
The ASQ-3 is a parent-completed developmental screening tool comprising 21 age-specific questionnaires for children aged 0–66 months. All questionnaires include six questions in each of five developmental domains: gross motor, fine motor, problem-solving, personal-social, and communication. Responses to each question are categorized as ‘yes’ (10 points), ‘sometimes’ (5 points), or ‘not yet’ (0 points) f, yielding a maximum score of 60 in each domain. Domain scores are compared to normative scores, with cut-off points categorizing development in that domain as ‘typical’ (<1SD below the mean), ‘monitor’ (≥1SD to <2SD below the mean), or ‘refer for further assessment’ (‘refer’) (≥2SD below the mean). According to ASQ-3 instructions, children with ASQ-3-GM scores below the ‘refer’ cutoff require further assessment for gross motor delay (12, 32).
2.2.2 Independent variables
2.2.2.1 Basic information
The items were composed of age (months), sex, gestational week, birth weight, and birth order. Age was divided into three categories (1 = 1–12 months, 2 = 13–24 months, and 3 = 25–36 months). Sex was categorized as male and female (0 = male and 1 = female). Using 37 weeks as the cut-off point, the gestation week was categorized into preterm and non-preterm infants (1 = <37, 2 = ≥37). Birth weight was categorized as low birth weight, normal weight, and macrosomia (1 = low birth weight, 2 = normal weight and 3 = macrosomia). The order of birth was categorized into two types depending on whether the child was the first birth (1 = first-born child and 2 = non-first-born child).
2.2.2.2 Feeding situation
By asking parents how their babies were fed within the age of 6 months, they were categorized as exclusively breastfeeding (fed only mother’s milk without any other dairy products or animal milk added), mixed feeding (breastfed with formula milk), and bottle-feeding (fed with formula milk only). The mode of delivery is categorized as natural delivery, cesarean section, and instrumental delivery (including forceps or suction). Feeding status was determined by asking parents “whether or not complementary foods have been added” and “Is the baby getting colostrum?”(0 = No; 1 = Yes).
2.2.2.3 Living habits
Average parent–child reading time per day (1 = less than 5 min, 2 = 5–15 min, 3 = 16–30 min, and 4 = more than 30 min). Average time spent interacting with other children per day (1 = less than 15 min, 2 = 15–30 min, 3 = 31–60 min, and 4 = more than 60 min). Average time spent outdoors per day (1 = less than 30 min, 2 = 30–60 min, 3 = 61–90 min, 4 = 91–120 min, and 5 = more than 60 min).
2.2.2.4 Parental information
Parental education level (1 = middle school and below, 2 = high school, and 3 = college), mother’s age at childbearing (1 = <35, 2 = ≥35), father’s age at childbearing (1 = ≤24, 24 < 2 ≤ 34, 3 > 34), maternal employment status (0 = unemployment, 1 = employment), career type of the parents (1 = government/institutional cadres/civil servants, 2 = professionals and technicians, 3 = clerks and service workers, 4 = production and manufacturing workers, 5 = others, and 6 = unemployment), and maternal health status during pregnancy (gestational diabetes mellitus, hypertension during pregnancy, anemia during pregnancy, bacterial vaginitis (BVI), placenta previa and prenatal depression) were collected. Family type was categorized as follows: nuclear family (consisting of married couples and unmarried or adopted children), a backbone family (consisting of three generations of parents, married children, and their offspring), and other family structures.
2.3 Decision tree
The decision tree model of machine learning was created using the open-source software R Studio. The decision tree has the following advantages: (1) Decision-tree algorithms are adept at generating straightforward and interpretable classification guidelines, displaying these rules in a clear, top-down graphical format. (2) These models are proficient at managing intricate interactions among predictor variables as evidenced by their capacity to accommodate high multicollinearity (33). In this study, a decision tree model was developed and the significant variables were ranked in order of importance.
2.4 Statistical analysis
Categorical variables are expressed as frequencies and percentages, and the difference between groups was tested using the chi-square test. The data set was randomly divided into two parts: 70% as the training set and 30% as the validation set. The variables were screened using univariable and multivariable regression analyses (p < 0.1); all tests were two-tailed. Odds ratios (ORs) and 95% confidence intervals (CIs) were used to quantify the associations between factors and gross motor developmental delay. Given the significant imbalance in this data set, the ROSE algorithm was used to address the issue, and a decision tree was constructed based on the processed data. The statistical analysis and decision tree analysis were performed using the R software package (version 4.3.1).
3 Results
3.1 Descriptive analysis
Table 1 presents the baseline characteristics of all participants grouped according to the presence or absence of developmental delay. Approximately 1.43% of children under 3 years of age have delayed gross motor development. One-half of respondents were under 12 months, of which 43.77% were female. The proportion of first-born infants reached 55.45%. Significant differences (p<0.05) were noted in variables such as age, mode of delivery, complementary food, mother’s education, father’s education, family type and average time spent interacting with others children when comparing the typical development group to the atypical development (Table 1).
3.2 Results of logistic regression
To investigate more deeply the relationship between various factors and gross motor retardation, we used logistics regression to screen for statistically significant variables. The results of the univariate regression analysis indicated that age, birth order, feeding method, mode of delivery, whether supplementary food was given, family type, and average time spent interacting with children were related to GMS (p < 0.1). The results of multivariate regression analysis indicated that with age, the likelihood of gross motor developmental delay increased relative to the probability of not occurring (OR = 2.8, 95% CI = [1.486, 5.274] and OR = 6.635, 95% CI = [1.486, 5.274]). Within the age of 6 months, mixed feeding had an increased risk of gross motor developmental delay compared to exclusive breastfeeding (OR = 1.684, 95% CI = [1.079, 2.629]). There was an increased prevalence of gross motor developmental delay in children with instrumental delivery compared to natural delivery (OR = 3.65, 95% CI = [1.489, 8.95]) (Table 2).
3.3 Predictive analysis of gross motor developmental delay
According to Figure 1, the predictor variable of gross motion retardation is age, supplementary food, playtime, family type, feeding method, mode of delivery, and birth order. According to Figure 2, a total of seven variables that were important for gross motor developmental delay of children under the age of 3 years were screened. These variables were prioritized based on their significance: age (importance = 233.66), supplementary food (153.23), playtime (116.43), family type (101.1), feeding method (93.54), mode of delivery (34.15), and birth order (5.41).
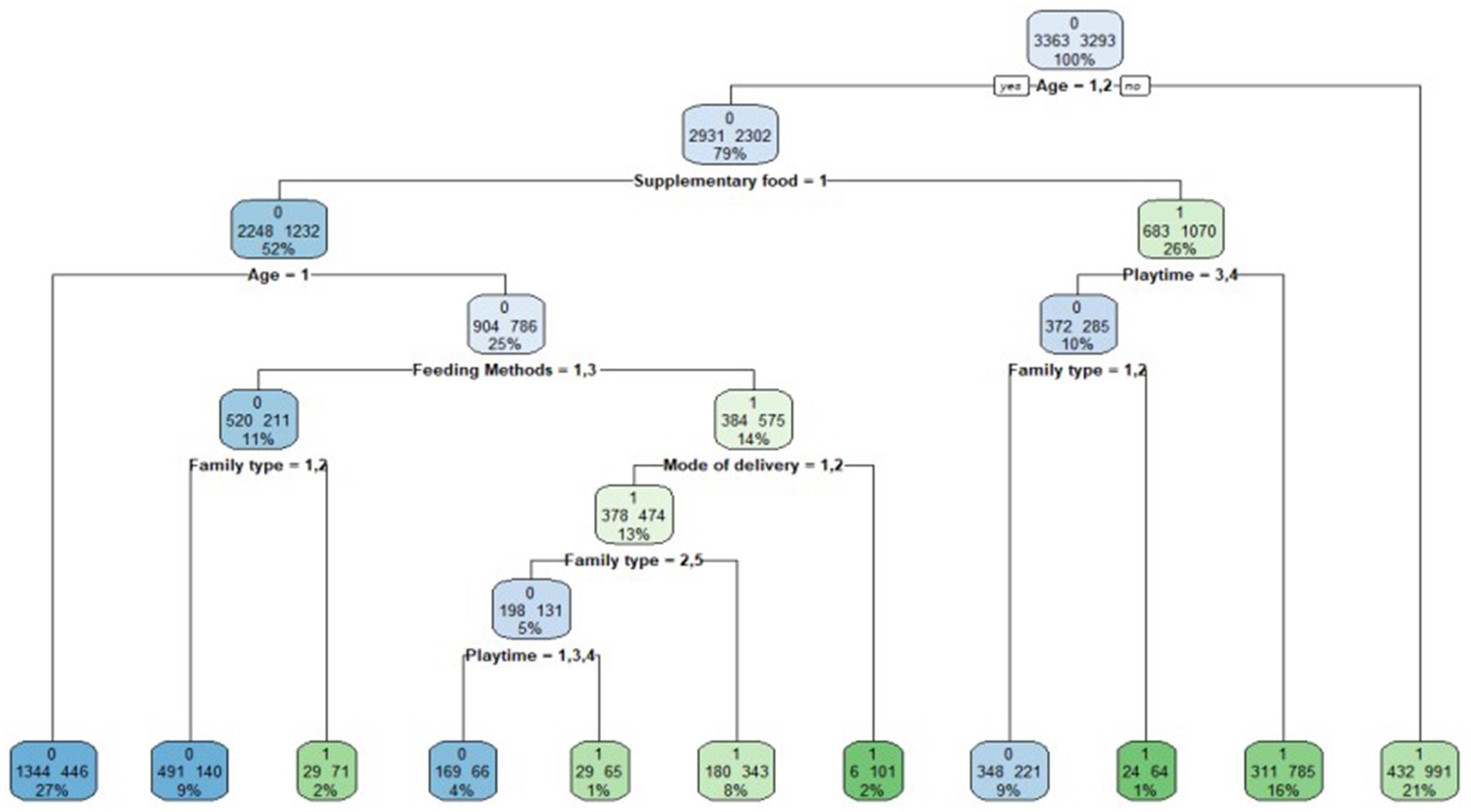
Figure 1. Decision tree for predicting gross motor development. The decision tree model shows that GMS are predicted to be typical development or atypical development depending on age, whether to provide supplementary food, average time spent interacting with children,family type, feeding method, mode of delivery and birth order.
3.4 Evaluation of decision tree model
The data set was divided into a training set and a test set in a 7:3 ratio. The accuracy of the test set was 70.96%, indicating that the model was effective (Table 3).
4 Discussion
This research endeavored to investigate the variances in the occurrence rates and associated risks of gross motor developmental lag in children below 3 years old. Concurrently, a decision-tree model was established to elucidate the importance of each factor causing gross motor developmental delay. The findings from this study furnished a solid foundation for an in-depth comprehension of the prevalence of motor developmental delays. Our findings showed that approximately 1.43% of children under the age of 3 years exhibited a low prevalence of gross motor developmental delay, consistent with our initial prediction. Moreover, the logistic regression analysis demonstrated that age, whether supplementary food was given, average time spent interacting with children, family type, feeding methods, mode of delivery, and birth order were the most significant predictors of gross motor developmental delay. The accuracy of the test set was 70.96%, indicating that the model was effective.
Utilizing the decision tree approach, our analysis revealed that age was the most significant factor affecting gross motor developmental delay among children under the age of 3 years. The likelihood of gross motor developmental delay increases with age. This finding has been confirmed in a number of studies (34, 35). Therefore, we should strengthen the monitoring of children’s GMS, with regular developmental assessments to identify potential gross motor delays. We found that there was no statistically significant difference between the sexes. However, there is no consensus on this conclusion (34, 36–38). Moreover, a child’s birth weight and gestation period are not associated with GMS. However, there is evidence that shorter gestational age is associated with gross motor developmental delays, and birth weight is significantly associated with motor development (39, 40). However, this study has a larger range of samples, and the results are more credible. To address this discrepancy, more research is needed in the future to explore it in depth.
This study established a positive link between exclusive breastfeeding and gross motor skills, with a multitude of studies affirming the clear-cut advantages of exclusive breastfeeding for child development (41–43). Exclusive breastfeeding duration as a factor was defined differently in all studies, and conflicting evidence was found regarding the role of exclusive breastfeeding (42, 43). Furthermore, we found that there was an increased prevalence of gross motor developmental delays in children with instrumental delivery compared to natural delivery. There are even studies showing that delivery circumstances had an association with attention-deficit hyperactivity disorder (44) and autism spectrum disorder (45). However, existing research findings are still uncertain regarding the precise impact of cesarean delivery on gross motor skills. For instance, certain studies have detected no substantial disparity in gross motor performance up to 12 months between children conceived through assisted reproductive technology and those in the control group (46–48).
This study did not find a correlation between maternal depression and children’s gross motor development. Overall, no studies have confirmed this association (49, 50). Furthermore, our research illuminated a positive correlation between GMS and average time spent interacting with children and no correlation between GMS and average parent–child reading time per day. A Canadian investigation determined that increased reading duration across various time points was considerably correlated with enhanced ASQ-3 scores in fine motor, gross motor, personal-social, and overall developmental domains over time (51). Therefore, more research is needed to explore this area in the future.
This research encountered several limitations. First, the cross-sectional methodology employed in this study restricted the ability to definitively infer causality. Second, the ASQ-3 was not designed for diagnosing gross motor developmental delays. Third, the data were obtained from the parents of the infants, who might lack comprehensive knowledge of their child’s precise condition, potentially leading to discrepancies between the reported data and the actual circumstances.
This research was endowed with multiple advantages. Initially, it boasted a substantial and representative participant pool. Moreover, it delved into the correlation between maternal gestational conditions and developmental delays.
5 Conclusion
Through the decision tree model, a total of seven critical influencing factors for gross motor developmental delay were screened. The top three factors in order of their importance were age, supplementary food, playtime, and family type, followed by feeding methods, mode of delivery, and birth order.
Data availability statement
The datasets presented in this article are not readily available because data is strictly confidential and cannot be shared. Requests to access the datasets should be directed to Yuxiang Xiong, eXV4aWFuZ3hpb25nMjAyMkAxNjMuY29t.
Ethics statement
The studies involving humans were approved by the Ethics Committee of Shenzhen Health Development and Data Management Center (No. 2021006). The studies were conducted in accordance with the local legislation and institutional requirements. The participants provided their written informed consent to participate in this study.
Author contributions
YX: Visualization, Writing – original draft, Formal analysis. XH: Data curation, Writing – original draft. JC: Conceptualization, Writing – review & editing. LS: Data curation, Writing – original draft. YY: Writing – original draft. BN: Funding acquisition, Writing – review & editing, Formal analysis, Supervision.
Funding
The author(s) declare that financial support was received for the research, authorship, and/or publication of this article. The study is supported by the Key Program of the National Natural Science Foundation of China (No. 72334004), the National Natural Science Foundation of China (No. 71971143), the Natural Science Foundation of Guangdong Province (No. 2024A1515011712), the Guangdong Provincial Philosophy and Social Sciences Planning Project (No. GD22CGL35), the Special Projects in Key Fields of Ordinary Colleges and Universities in Guangdong Province (No. 2022ZDZX2054), and the University Innovation Team Project of Guangdong Province (No. 2021WCXTD002).
Acknowledgments
We thank all the babies and their families for taking part in the study.
Conflict of interest
The authors declare that the research was conducted in the absence of any commercial or financial relationships that could be construed as a potential conflict of interest.
Publisher’s note
All claims expressed in this article are solely those of the authors and do not necessarily represent those of their affiliated organizations, or those of the publisher, the editors and the reviewers. Any product that may be evaluated in this article, or claim that may be made by its manufacturer, is not guaranteed or endorsed by the publisher.
Abbreviations
ASQ, Ages and Stages Questionnaires; ASQ-3, Ages and Stages Questionnaires—Third Edition; ORs, Odds ratios; Cis, Confidence intervals; GMS, Gross motor skill; AIMS, Alberta Infant Motor Scale; Bayley-III, Bayley Scale of Infant and Toddler Development-III; BOT-2, Bruininks–Oseretsky Test of Motor Proficiency-2; MABC-2, Movement Assessment Battery for Children-2; MAND, McCarron Assessment of Neuromuscular Development; NSMDA, Neurological Sensory Motor Developmental Assessment; PDMS-2, Peabody Developmental Motor Scales-2; TGMD-2, Test of Gross Motor Development-2.
References
1. Nagel, M, and Sharman, R. The encyclopedia of child and adolescent development. Biol Neurol Cogn Adolesc. (2019) 8. doi: 10.1002/9781119171492
2. Black, MM. Nurturing care framework and implementation science: promoting nutrition, health and development among infants and toddlers globally. Nestle Nutr Inst Workshop Ser. (2019) 92:53–64. doi: 10.1159/000501660
3. Veldman, SLC, Santos, R, Jones, RA, Sousa-Sá, E, and Okely, AD. Associations between gross motor skills and cognitive development in toddlers. Early Hum Dev. (2019) 132:39–44. doi: 10.1016/j.earlhumdev.2019.04.005
4. Viegas, ÂA, Mendonça, VA, Pontes Nobre, JN, Souza Morais, RL, Fernandes, AC, Oliveira Ferreira, F, et al. Associations of physical activity and cognitive function with gross motor skills in preschoolers: cross-sectional study. J Mot Behav. (2023) 55:564–79. doi: 10.1080/00222895.2021.1897508
5. Burns, RD, Brusseau, TA, Fu, Y, and Hannon, JC. Gross motor skills and cardiometabolic risk in children: a mediation analysis. Med Sci Sports Exerc. (2017) 49:746–51. doi: 10.1249/MSS.0000000000001147
6. Wang, LAL, Petrulla, V, Zampella, CJ, Waller, R, and Schultz, RT. Gross motor impairment and its relation to social skills in autism spectrum disorder: a systematic review and two meta-analyses. Psychol Bull. (2022) 148:273–300. doi: 10.1037/bul0000358
7. Spittle, AJ, Doyle, LW, and Boyd, RN. A systematic review of the clinimetric properties of neuromotor assessments for preterm infants during the first year of life. Dev Med Child Neurol. (2008) 50:254–66. doi: 10.1111/j.1469-8749.2008.02025.x
8. Griffiths, A, Toovey, R, Morgan, PE, and Spittle, AJ. Psychometric properties of gross motor assessment tools for children: a systematic review. BMJ Open. (2018) 8:e021734. doi: 10.1136/bmjopen-2018-021734
9. Manasyan, A, Salas, AA, Nolen, T, Chomba, E, Mazariegos, M, Tshefu Kitoto, A, et al. Diagnostic accuracy of ASQ for screening of neurodevelopmental delays in low resource countries. BMJ Open. (2023) 13:e065076. doi: 10.1136/bmjopen-2022-065076
10. Small, JW, Hix-Small, H, Vargas-Baron, E, and Marks, KP. Comparative use of the ages and stages questionnaires in low-and middle-income countries. Dev Med Child Neurol. (2019) 61:431–43. doi: 10.1111/dmcn.13938
11. Filgueiras, A, Pires, P, Maissonette, S, and Landeira-Fernandez, J. Psychometric properties of the Brazilian-adapted version of the ages and stages questionnaire in public child daycare centers. Early Hum Dev. (2013) 89:561–76. doi: 10.1016/j.earlhumdev.2013.02.005
12. Fauls, JR, Thompson, BL, and Johnston, LM. Validity of the ages and stages questionnaire to identify young children with gross motor difficulties who require physiotherapy assessment. Dev Med Child Neurol. (2020) 62:837–44. doi: 10.1111/dmcn.14480
13. Kyerematen, V, Hamb, A, Oberhelman, RA, Cabrera, L, Bernabe-Ortiz, A, and Berry, SJ. Exploratory application of the ages and stages (ASQ) child development screening test in a low-income Peruvian shantytown population. BMJ Open. (2014) 4:e004132. doi: 10.1136/bmjopen-2013-004132
14. Romero Otalvaro, AM, Grañana, N, Gaeto, N, Torres, M, Zamblera, MN, Vasconez, MA, et al. ASQ-3: validation of the ages and stages questionnaire for the detection of neurodevelopmental disorders in argentine children. Archivos Argentinos de Pediatria. (2018) 116:7–13. doi: 10.5546/aap.2018.eng.7
15. Zhang, Z, Okely, AD, Pereira, JR, Sousa-Sá, E, Veldman, SLC, and Santos, R. Associations of sleep characteristics with cognitive and gross motor development in toddlers. Sleep Health. (2022) 8:350–5. doi: 10.1016/j.sleh.2022.04.001
16. Godoy-Cumillaf, A, Fuentes-Merino, P, Giakoni-Ramírez, F, Duclos-Bastías, D, Bruneau-Chávez, J, Vergara-Ampuero, D, et al. Association between gross motor competence and physical fitness in Chilean children aged 4 to 6 years. Children. (2024) 11:561. doi: 10.3390/children11050561
17. Tomaz, SA, Jones, RA, Hinkley, T, Bernstein, SL, Twine, R, Kahn, K, et al. Gross motor skills of South African preschool-aged children across different income settings. J Sci Med Sport. (2019) 22:689–94. doi: 10.1016/j.jsams.2018.12.009
18. Lubans, DR, Morgan, PJ, Cliff, DP, Barnett, LM, and Okely, AD. Fundamental movement skills in children and adolescents: review of associated health benefits. Sports Med. (2010) 40:1019–35. doi: 10.2165/11536850-000000000-00000
19. Barnett, LM, Ridgers, ND, and Salmon, J. Associations between young children's perceived and actual ball skill competence and physical activity. J Sci Med Sport. (2015) 18:167–71. doi: 10.1016/j.jsams.2014.03.001
20. Logan, SW, Scrabis-Fletcher, K, Modlesky, C, and Getchell, N. The relationship between motor skill proficiency and body mass index in preschool children. Res Q Exerc Sport. (2011) 82:442–8. doi: 10.1080/02701367.2011.10599776
21. Veldman, SLC, Jones, RA, Santos, R, Sousa-Sá, E, Pereira, JR, Zhang, Z, et al. Associations between gross motor skills and physical activity in Australian toddlers. J Sci Med Sport. (2018) 21:817–21. doi: 10.1016/j.jsams.2017.12.007
22. Varsi, K, Torsvik, IK, Huber, S, Averina, M, Brox, J, and Bjørke-Monsen, AL. Impaired gross motor development in infants with higher PFAS concentrations. Environ Res. (2022) 204:112392. doi: 10.1016/j.envres.2021.112392
23. Burns, RD, Byun, W, and Brusseau, TA. Gross motor skills predict classroom behavior in lower-income children. Front Sports Act Living. (2019) 1:29. doi: 10.3389/fspor.2019.00029
24. Burns, RD, Fu, Y, Fang, Y, Hannon, JC, and Brusseau, TA. Effect of a 12-week physical activity program on gross motor skills in children. Percept Mot Skills. (2017) 124:1121–33. doi: 10.1177/0031512517720566
25. Burns, RD, Fu, Y, Hannon, JC, and Brusseau, TA. School physical activity programming and gross motor skills in children. Am J Health Behav. (2017) 41:591–8. doi: 10.5993/AJHB.41.5.8
26. Hu, X, Jiang, GP, Ji, ZQ, Pang, B, and Liu, J. Effect of novel rhythmic physical activities on fundamental movement skills in 3-to 5-year-old children. Biomed Res Int. (2020) 2020:1–10. doi: 10.1155/2020/8861379
27. Zhou, N, and Cheah, CS. Ecological risk model of childhood obesity in Chinese immigrant children. Appetite. (2015) 90:99–107. doi: 10.1016/j.appet.2015.02.028
28. Ma, Y, Song, J, Hu, M, Yang, R, and Yang, P. Risk factors of in-home unintentional injuries among 0-6-year-old children in Changsha city of China: a cross-sectional survey based on Bronfenbrenner's ecological system theory. BMC Pediatr. (2022) 22:598. doi: 10.1186/s12887-022-03661-z
29. Purkait, T. Farm to preschool programs and its impact on Children's dietary health: evaluation through Bronfenbrenner's socio-ecological model. Ecol Food Nutr. (2024) 63:191–203. doi: 10.1080/03670244.2024.2327619
30. Perone, S, and Simmering, VR. Applications of dynamic systems theory to cognition and development: new frontiers. Adv Child Dev Behav. (2017) 52:43–80. doi: 10.1016/bs.acdb.2016.10.002
31. Wei, M, Bian, X, Squires, J, Yao, G, Wang, X, Xie, H, et al. Studies of the norm and psychometrical properties of the ages and stages questionnaires, third edition, with a Chinese national sample. Chinese J Pediatr. (2015) 53:913–8. doi: 10.3760/cma.j.issn.0578-1310.2015.12.009
32. Squires, J, Bricker, DD, and Twombly, E. Ages & stages questionnaires. Baltimore: Paul H. Brookes (2009).
33. Wang, Q, Kou, Z, Sun, X, Wang, S, Wang, X, Jing, H, et al. Predictive analysis of the pro-environmental behaviour of college students using a decision-tree model. Int J Environ Res Public Health. (2022) 19:9407. doi: 10.3390/ijerph19159407
34. Veldman, SLC, Jones, RA, Santos, R, Sousa-Sá, E, and Okely, AD. Gross motor skills in toddlers: prevalence and socio-demographic differences. J Sci Med Sport. (2018) 21:1226–31. doi: 10.1016/j.jsams.2018.05.001
35. Sorgente, V, Cohen, EJ, Bravi, R, and Minciacchi, D. Crosstalk between gross and fine motor domains during late childhood: the influence of gross motor training on fine motor performances in primary school children. Int J Environ Res Public Health. (2021) 18:11387. doi: 10.3390/ijerph182111387
36. Aye, T, Kuramoto-Ahuja, T, Sato, T, Sadakiyo, K, Watanabe, M, and Maruyama, H. Gross motor skill development of kindergarten children in Japan. J Phys Ther Sci. (2018) 30:711–5. doi: 10.1589/jpts.30.711
37. Zhu, Y, Wang, S, Qian, Y, Hu, J, Zhou, H, Korivi, M, et al. The impact of birth season and sex on motor skills in 2-year-old children: a study in Jinhua, eastern China. Life. (2024) 14:836. doi: 10.3390/life14070836
38. Reyes, AC, Chaves, R, Baxter-Jones, ADG, Vasconcelos, O, Barnett, LM, Tani, G, et al. Modelling the dynamics of children's gross motor coordination. J Sports Sci. (2019) 37:2243–52. doi: 10.1080/02640414.2019.1626570
39. Flensborg-Madsen, T, and Mortensen, EL. Predictors of motor developmental milestones during the first year of life. Eur J Pediatr. (2017) 176:109–19. doi: 10.1007/s00431-016-2817-4
40. Scharf, RJ, Stroustrup, A, Conaway, MR, and DeBoer, MD. Growth and development in children born very low birthweight. Arch Dis Child Fetal Neonatal Ed. (2016) 101:F433–8. doi: 10.1136/archdischild-2015-309427
41. Jardí, C, Hernández-Martínez, C, Canals, J, Arija, V, Bedmar, C, Voltas, N, et al. Influence of breastfeeding and iron status on mental and psychomotor development during the first year of life. Infant Behav Dev. (2018) 50:300–10. doi: 10.1016/j.infbeh.2017.05.009
42. Michels, KA, Ghassabian, A, Mumford, SL, Sundaram, R, Bell, EM, Bello, SC, et al. Breastfeeding and motor development in term and preterm infants in a longitudinal US cohort. Am J Clin Nutr. (2017) 106:1456–62. doi: 10.3945/ajcn.116.144279
43. Bjarnadóttir, E, Stokholm, J, Chawes, B, Thorsen, J, Mora-Jensen, AC, Deleuran, M, et al. Determinants of neurodevelopment in early childhood - results from the Copenhagen prospective studies on asthma in childhood (COPSAC(2010)) mother-child cohort. Acta Paediatr. (2019) 108:1632–41. doi: 10.1111/apa.14753
44. Talge, NM, Allswede, DM, and Holzman, C. Gestational age at term, delivery circumstance, and their association with childhood attention deficit hyperactivity disorder symptoms. Paediatr Perinat Epidemiol. (2016) 30:171–80. doi: 10.1111/ppe.12274
45. Curran, EA, Dalman, C, Kearney, PM, Kenny, LC, Cryan, JF, Dinan, TG, et al. Association between obstetric mode of delivery and autism spectrum disorder: a population-based sibling design study. JAMA Psychiatry. (2015) 72:935–42. doi: 10.1001/jamapsychiatry.2015.0846
46. Hashimoto, K, Ogawa, K, Horikawa, R, Ikeda, N, Kato, K, Kamide, A, et al. Gross motor function and general development of babies born after assisted reproductive technology. J Obstet Gynaecol Res. (2016) 42:266–72. doi: 10.1111/jog.12898
47. Aoki, S, Hashimoto, K, Ogawa, K, Horikawa, R, and Sago, H. Developmental outcomes of Japanese children born through assisted reproductive technology (ART) in toddlerhood. J Obstet Gynaecol Res. (2018) 44:929–35. doi: 10.1111/jog.13613
48. Dekeunink, GM, Goossens, SM, Matthijs, V, Senden, RH, Beckers, CM, and Roumen, FJ. Neurodevelopmental outcome of twins at two years of age according to the planned mode of delivery. J Matern Fetal Neonatal Med. (2016) 29:303–8. doi: 10.3109/14767058.2014.999232
49. Smith-Nielsen, J, Tharner, A, Krogh, MT, and Vaever, MS. Effects of maternal postpartum depression in a well-resourced sample: early concurrent and long-term effects on infant cognitive, language, and motor development. Scand J Psychol. (2016) 57:571–83. doi: 10.1111/sjop.12321
50. Sutter-Dallay, AL, Murray, L, Dequae-Merchadou, L, Glatigny-Dallay, E, Bourgeois, ML, and Verdoux, H. A prospective longitudinal study of the impact of early postnatal vs. chronic maternal depressive symptoms on child development. Eur Psychiatry. (2011) 26:484–9. doi: 10.1016/j.eurpsy.2010.05.004
Keywords: gross motor, decision tree, under the age of 3 years, stratified random sampling, feature importance
Citation: Xiong Y, Hu X, Cao J, Shang L, Yao Y and Niu B (2024) Development of gross motor skills in children under the age of 3 years: a decision tree approach. Front. Public Health. 12:1421173. doi: 10.3389/fpubh.2024.1421173
Edited by:
Catherine M. Capio, Hong Kong Metropolitan University, Hong Kong SAR, ChinaReviewed by:
Aleksandra Đurić Zdravković, University of Belgrade, SerbiaRafaela Silva Moreira, Federal University of Santa Catarina, Brazil
Aymen Balikci, Kocaeli University, Türkiye
Copyright © 2024 Xiong, Hu, Cao, Shang, Yao and Niu. This is an open-access article distributed under the terms of the Creative Commons Attribution License (CC BY). The use, distribution or reproduction in other forums is permitted, provided the original author(s) and the copyright owner(s) are credited and that the original publication in this journal is cited, in accordance with accepted academic practice. No use, distribution or reproduction is permitted which does not comply with these terms.
*Correspondence: Ben Niu, ZHJuaXViZW5AZ21haWwuY29t
†These authors share first authorship
‡ORCID: Ben Niu, https://orcid.org/0000-0001-5822-8743