- Department of Infection Control, Second Affiliated Hospital of Zhejiang University School of Medicine, Hangzhou, Zhejiang, China
Background: Traditional observational studies exploring the association between air pollution and infections have been limited by small sample sizes and potential confounding factors. To address these limitations, we applied Mendelian randomization (MR) to investigate the potential causal relationships between particulate matter (PM2.5, PM2.5–10, and PM10), nitrogen dioxide, and nitrogen oxide and the risks of infections.
Methods: Single nucleotide polymorphisms (SNPs) related to air pollution were selected from the genome-wide association study (GWAS) of the UK Biobank. Publicly available summary data for infections were obtained from the FinnGen Biobank and the COVID-19 Host Genetics Initiative. The inverse variance weighted (IVW) meta-analysis was used as the primary method for obtaining the Mendelian randomization (MR) estimates. Complementary analyses were performed using the weighted median method, MR-Egger method, and MR Pleiotropy Residual Sum and Outlier (MR-PRESSO) test.
Results: The fixed-effect IVW estimate showed that PM2.5, PM2.5–10 and Nitrogen oxides were suggestively associated with COVID-19 [for PM2.5: IVW (fe): OR 3.573(1.218,5.288), PIVW(fe) = 0.021; for PM2.5–10: IVW (fe): OR 2.940(1.385,6.239), PIVW(fe) = 0.005; for Nitrogen oxides, IVW (fe): OR 1.898(1.318,2.472), PIVW(fe) = 0.010]. PM2.5, PM2.5–10, PM10, and Nitrogen oxides were suggestively associated with bacterial pneumonia [for PM2.5: IVW(fe): OR 1.720 (1.007, 2.937), PIVW(fe) = 0.047; for PM2.5–10: IVW(fe): OR 1.752 (1.111, 2.767), P IVW(fe) = 0.016; for PM10: IVW(fe): OR 2.097 (1.045, 4.208), PIVW(fe) = 0.037; for Nitrogen oxides, IVW(fe): OR 3.907 (1.209, 5.987), PIVW(fe) = 0.023]. Furthermore, Nitrogen dioxide was suggestively associated with the risk of acute upper respiratory infections, while all air pollution were not associated with intestinal infections.
Conclusions: Our results support a role of related air pollution in the Corona Virus Disease 2019, bacterial pneumonia and acute upper respiratory infections. More work is need for policy formulation to reduce the air pollution and the emission of toxic and of harmful gas.
1 Background
Bacterial pneumonia, Corona Virus Disease 2019 (COVID-19), acute upper respiratory infections, and intestinal infections are common causes of hospital admission and important contributors to death (1). Among respiratory infectious diseases, lower respiratory tract infections (LRTIs) and pneumonia, in particular, rank in highest in terms of mortality (2). Several factors are implicated in heightening the risk of infection, including age, vaccination coverage, antibiotic therapy, seasonal fluctuations, and air pollution (3).
Notably, the persistently rising levels of air pollution worldwide have yielded dire consequences, leading to an alarming number of premature deaths. This increase in pollution is linked to a higher incidence of infections, especially respiratory infections (4, 5). Consequently, the role of respiratory infections as a driver of human mortality has assumed a position of paramount concern and it is now more crucial than ever to gain a comprehensive understanding of the intricate interplay between air pollution and infections. Particulate matter (PM) serves as a key indicator of air pollution, resulting from a variety of natural and human activities (6). Traditional observational studies have explored the association between PM and infections. Evidence from China by Yongjian Zhu indicated significantly positive associations of PM2.5, PM10, and Nitrogen dioxide (NO2) over the past 2 weeks with newly confirmed COVID-19 cases (7). However, this study did not include gender- or age-specific cases. Another study in Italy found that long-term air quality data with cases of COVID-19 in up to 71 Italian provinces, further indicating that chronic exposure to air pollution could facilitate virus spread (8). A systematic review with 15 studies by Chiara Copat indicated PM2.5 and NO2 are more closely correlated to COVID-19 than PM10 (9). Notably, these studies included limited sample sizes with potential confounders.
Investigating the causal association between air pollution and the risk of infections is challenging due to reverse causation and confounding. Mendelian randomization (MR) has emerged as a potent method for determining causation between risk factors and diseases by using genetic variants as instrument variables (IVs) (10). MR analysis can largely overcome the confounders with random assignment of an individual's genetic variants at conception. Moreover, it minimizes the risk of reverse causation, since the presence of a disease cannot affect individuals' genotypes (11).
In our study, we applied a two-sample MR analysis to explore the potential causal association between air pollution (including PM2.5, PM2.5–10, PM10, nitrogen dioxide, and nitrogen oxides) and risk of infections (intestinal infections, acute upper respiratory infections, bacterial pneumonia, and Corona Virus Disease 2019) using the summary statistics derived from the publicly available GWAS data.
2 Materials and methods
2.1 Study design
This study is reported according to the STROBE-MR guidelines (12). Our study is based on the Mendelian randomization design to explore the causal relationship between Air pollution and infections using publicly available summary datasets from two genome-wide association studies. In this study, air pollution indicators (PM2.5, PM2.5–10, PM10, nitrogen dioxide, and nitrogen oxides) were selected as exposures, while various infections (Intestinal infections, acute upper respiratory infections, bacterial pneumonia, and Corona Virus Disease 2019) served as outcomes. The design of this MR study is presented in Figure 1.
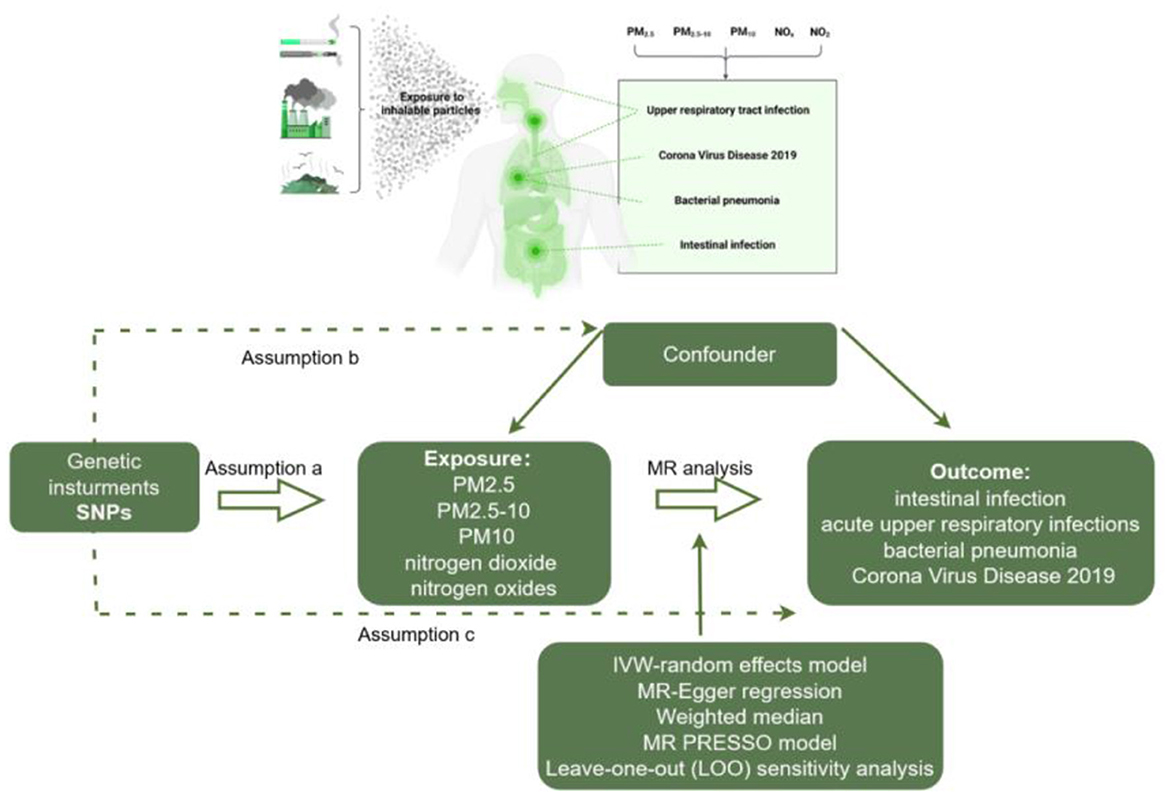
Figure 1. Workflow of two-sample MR for causal effect between air pollution and infection risks. PM, Particulate matter.
2.2 Data retrieval for MR analyses
We collected summary data on single nucleotide polymorphism (SNP)–phenotype associations from different Genome-wide Association Studies (GWAS). Publicly available summary data for PM2.5, PM2.5–10, PM10, nitrogen dioxide, and nitrogen oxides were obtained from UK Biobank, including more than 400,000 participants with European ancestors (13). UK Biobank is a large-scale biomedical database and research resource, containing in-depth genetic and health information from half a million UK participants (https://www.ukbiobank.ac.uk/). Air pollution-related indicators were measured by land use regression (LUR) models (14).
Publicly available summary data for Corona Virus Disease 2019 was from COVID-19 Host Genetics Initiative including 1,887,658 European participants (15). One hundred and five studies have joined the initiative, and participation is still expanding. The majority of studies are conducted in Europe (55%) and the US (28%) (15). Summary data for bacterial pneumonia, acute upper respiratory infections and intestinal infections were both from FinnGen Biobank with European ancestors (215,268 for Bacterial pneumonia, 218,792 for acute upper respiratory infections, 200,006 for intestinal infections). The GWASs conducted on the FinnGen dataset were analyzed using SAIGE and were adjusted for sex, age, first ten principal components, and genotyping batch (16). The detailed information was presented in Table 1.
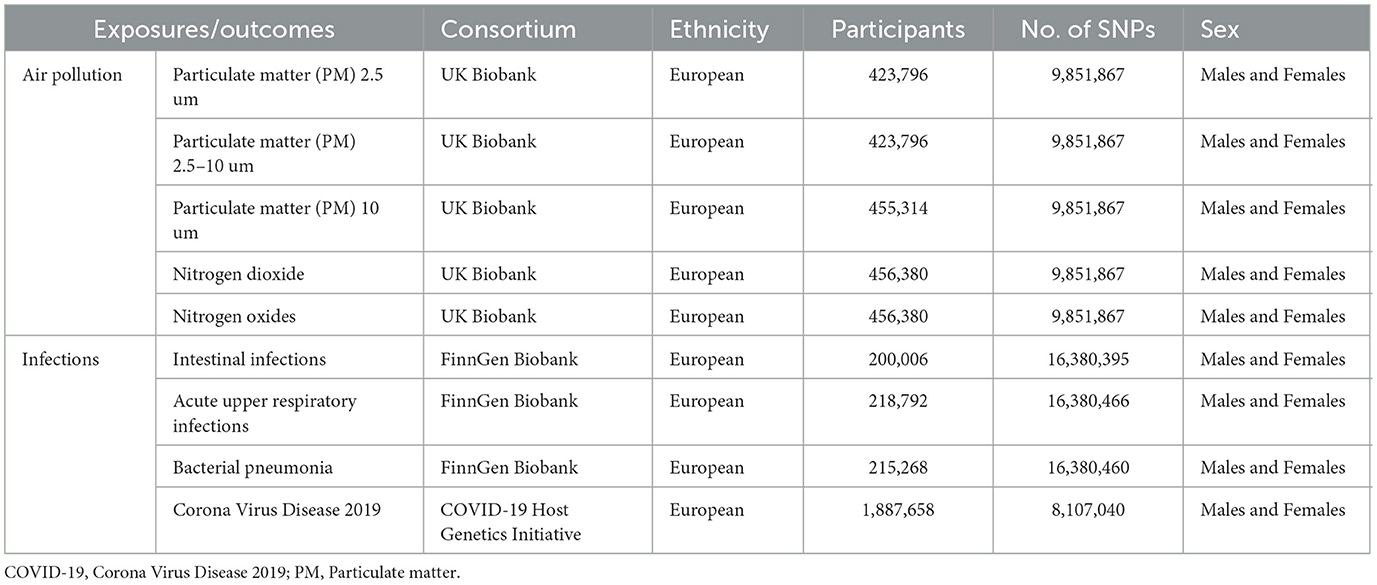
Table 1. Details of the genome-wide association studies included in this Mendelian randomization analysis.
2.3 Genetic instrumental variables selection
All genetic variants reaching genome-wide significance (P < 5 × 10−8) were selected as instruments for the MR analysis. To minimize the potential for weak instrumental variable bias, we employed a screening criterion of P < 5 × 10−6 for linear regression of each genetic variant on the respective risk factor (17–19).
Additionally, we lowered the genome-wide significance threshold of the PM2.5–10 to P < 5 × 10−6 to select enough SNPs as IVs associated with this significance level (17–19). The corresponding linkage disequilibrium was tested to confirm that there were any SNPs in a linkage disequilibrium state and the SNPs were independent by pruning SNPs within a 10,000 kb window with an R2 < 0.001 threshold (20). Furthermore, we identified SNPs associated with potential confounders of the outcomes. In this study, obesity, pregnancy, cardiovascular disease, and Parkinson's disease were considered confounding factors when COVID-19 was identified as the outcome (21–24). BMI, alcohol intake, asthma and coronary artery disease were considered confounding factors when Bacterial pneumonia and acute upper respiratory infections were identified as the outcomes (25, 26). Furthermore, Proton pump inhibitor (PPI) was considered confounding factors when Intestinal infections were identified as the outcome (http://www.phenoscanner.medschl.cam.ac.uk/) (27–29). SNP harmonization was conducted to correct the orientation of the alleles. We used the F statistic and R2 to evaluate the strength of the association between SNP and exposure and were also conducted to further assess weak instrument (30, 31). A strong correlation between SNP and exposure with sufficient statistical power was confirmed when the F statistic is >10 (Table 2).

Table 2. The F statistic, heterogeneity and horizontal pleiotropy test between the exposures and outcomes of this MR.
2.4 Two-sample MR analysis
Two-sample MR analysis were performed to explore the potential causal associations between air pollution and infections in two populations, respectively. For a genetic variant to be qualified as a valid instrument for causal inference in a MR study, it must meet three core assumptions (32):
a. The genetic variant must be truly associated with the exposure;
b. The genetic variant should not be associated with confounders of the exposure-outcome relationship; and
c. The genetic variant should only be related to the outcome of interest through the exposure under study.
The inverse variance weighted (IVW) meta-analysis was used as the main method for obtaining the MR estimate (33). Complementary analyses were performed using the weighted median method (34) and MR-egger method (34). Cochran's Q test and I2 was applied to assess heterogeneity between individual genetic variants estimates, by which random-effects model or fixed-effects model of IVW was determined (35).
Furthermore, we have performed another one-sample MR analysis as Replicative analysis. Summary statistics of air pollution and COVID-19 were all obtained from the (GWAS) UK Biobank.
2.5 Sensitivity analysis
To examine the possibility of violation of the main MR assumptions due to directional pleiotropy, the MR-Egger test for directional pleiotropy was performed (34). In this test, the intercept estimates the average pleiotropic effect across the genetic variants (36). Additionally, the MR pleiotropy residual sum and outlier test (MR-PRESSO) was performed to detect and correct the effects from outliers (37). To further assess the independent potential of each IV, a leave-one-out (LOO) sensitivity analysis was also performed. A forest plot was generated to evaluate the robustness of our results.
2.6 Statistical analysis
All analyses were performed using the package “Two-Sample-MR” (version 0.5.6) and “MR-PRESSO” (version 1.0) in R (version 4.0.5). A two-sided P value of < 0.05 was considered a potential causal relationship. To account for multiple testing in our primary analyses, a Bonferroni corrected threshold of P was applied.
3 Results
3.1 Genetic instrumental variables selected
After genetic Instrumental Variables Selections, the detailed information of the IVs was presented in Supplementary Table 1. F statistics for every instrument-exposure association were >10 in our study, demonstrating the small possibility of weak instrumental variable bias. Furthermore, the excluded SNPs associated with confounder risks were presented in Supplementary Tables 1, 2.
3.2 PM2.5, PM2.5–10, and nitrogen oxides will increase the risk of Corona Virus Disease 2019
After genetic instrumental variables selected, 6, 21, 20, 7, and 4 SNPs for PM2.5, PM2.5–10, PM10, nitrogen dioxide, and nitrogen oxides were identified after removal of chained unbalanced IVs. Table 3 reported the MR estimated for the association between air pollution and Corona Virus Disease 2019. The fixed-effect IVW estimate showed that PM2.5 and PM2.5–10 were significantly associated with Corona Virus Disease 2019 (for PM2.5: PIVW(fe) = 0.021; for PM2.5–10, PIVW(fe) = 0.005). However, we did not observe evidence of causal association in Bonferroni correction. To ensure the robustness of our results, MR-PRESSO was also conducted which showed the similar results (for PM2.5: PMR − PRESSO = 0.037; for PM2.5–10: PMR − PRESSO = 0.013). Furthermore, The IVW method showed that Nitrogen oxides was significantly associated with Corona Virus Disease 2019 (PIVW(fe) = 0.010), while PM10 and Nitrogen dioxide were not the risk factor of it (for PM10: PIVW(fe) = 0.873; for Nitrogen dioxide: P IVW(fe) = 0.533). Figure 2 also presented the MR results by IVW estimate. And we also found that the genetically predicted PM2.5 um was positively associated with increased risk of COVID-19 with IVW method by replicative analysis (Supplementary Tables 4, 5). There was no evidence of heterogeneity or directional pleiotropy for the analysis of air pollution on COVID-19 (Table 2, Supplementary Table 6). The forest plots were displayed in Figure 3. The Leave-one-out sensitivity analysis of MR estimate were presented in Supplementary Figures 1–3.
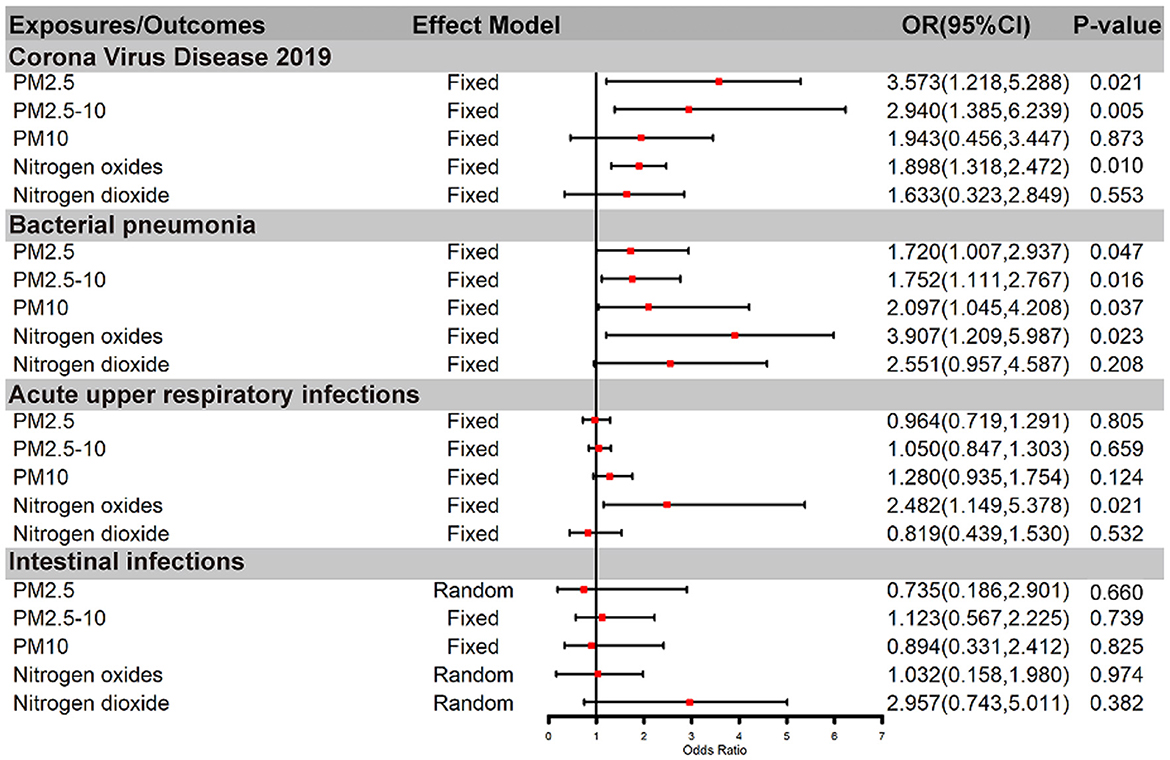
Figure 2. Causal relationship between air pollution and infections by IVW method. OR, odds ratio; PM, Particulate matter.
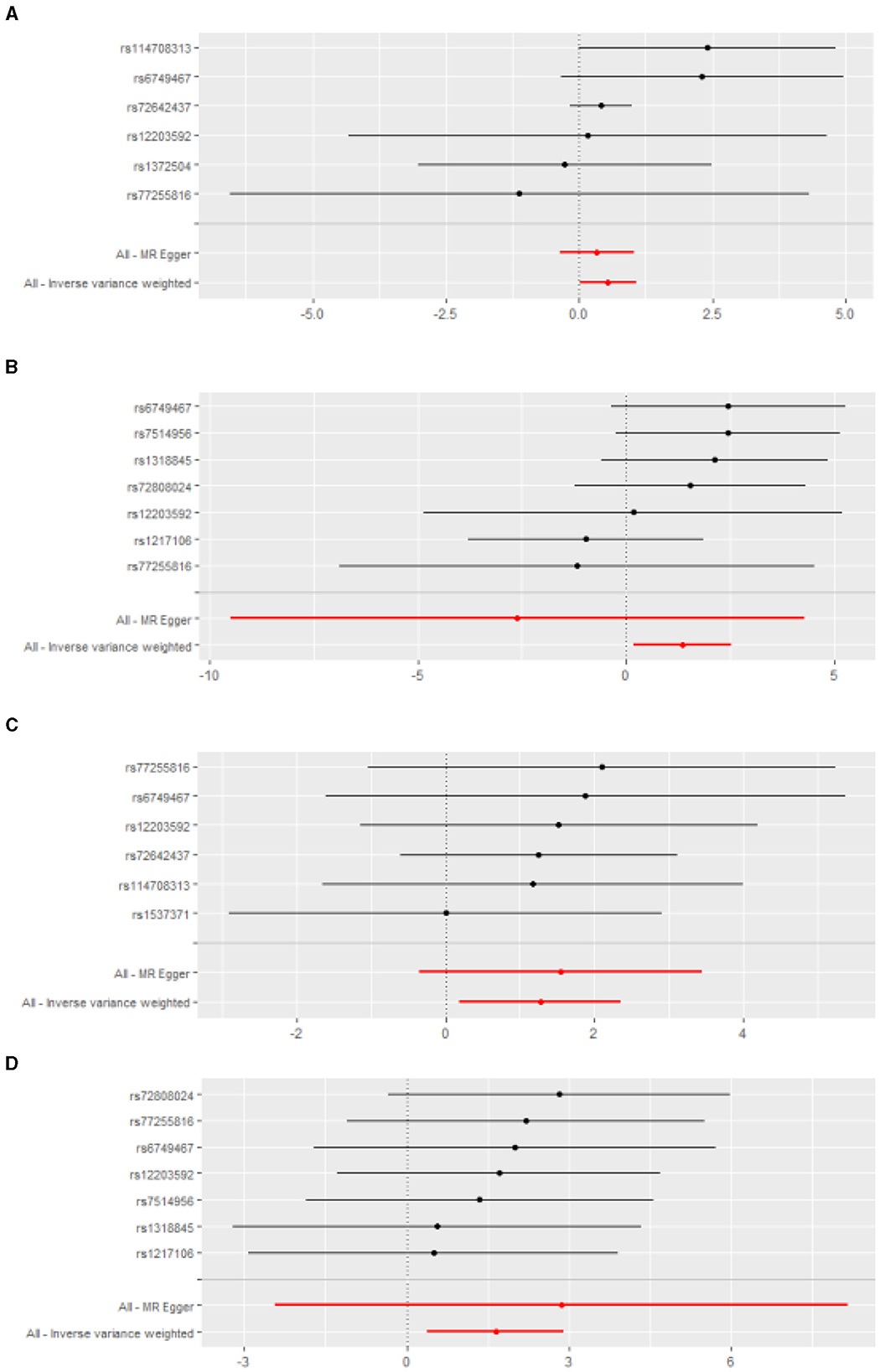
Figure 3. The forest plots for the effect of air pollution on the infections. (A) PM2.5 and bacterial pheumonia; (B) Nitrogen oxides and bacterial pheumonia; (C) PM2.5 and COVID-19; (D) Nitrogen oxides and COVID-19.
3.3 PM2.5, PM2.5–10, PM10, and Nitrogen oxides will increase the risk of bacterial pneumonia
After genetic instrumental variables selected, 6, 21, 17, 7 and 5 SNPs for PM2.5, PM2.5–10, PM10, nitrogen dioxide, and nitrogen oxides were identified after removal of chained unbalanced IVs. The fixed-effect IVW estimate showed that PM2.5, PM2.5–10, PM10, and Nitrogen oxides were significantly associated with bacterial pneumonia (for PM2.5: PIVW(fe) = 0.047; for PM2.5–10: P IVW(fe) = 0.016; for PM10: P IVW(fe) = 0.037; for Nitrogen oxides: P IVW(fe) = 0.023, Table 3, Figure 2, Supplementary Table 3). However, we did not observe evidence of causal association in Bonferroni correction. In addition, the IVW method showed that Nitrogen dioxide was not significantly associated with bacterial pneumonia (P IVW(fe) = 0.208). The results were consistent in complementary analyses (PMR − Egger = 0.858, PWeightedmedian = 0.518, PMR − PRESSO = 0.518). There was no evidence of heterogeneity or directional pleiotropy for the analysis (Table 2, Supplementary Table 6). And the forest plots were displayed in Figure 3.
3.4 Air pollution was not associated with the risk of acute upper respiratory infections and Intestinal infections except Nitrogen dioxide
The IVW estimate support that Nitrogen dioxide was associated with the risk of acute upper respiratory infections which were consistent with the result by MR-PRESSO method (PIVW(fe) = 0.021, PMR − PRESSO = 0.044, Table 3, Figure 2, Supplementary Table 3). However, we did not observe evidence of causal association in Bonferroni correction. All method did not support that PM2.5, PM2.5–10, PM10, and nitrogen dioxide were associated with Acute upper respiratory infections and intestinal infections. There was no evidence of heterogeneity or directional pleiotropy for the analysis of air pollution on acute upper respiratory infections (Table 2, Supplementary Table 6). However, Cochran's Q test showed that there was heterogeneity for the analysis of PM2.5, nitrogen oxides, and nitrogen dioxide on intestinal infections. And the forest plots were displayed in Figure 3.
4 Discussion
In this two-sample MR study, we found that PM2.5, PM2.5–10, and nitrogen oxides increase the risk of Coronavirus Disease 2019. Furthermore, PM2.5, PM2.5–10, PM10, and nitrogen oxides are associated with an increased risk of bacterial pneumonia. However, our analysis did not reveal a suggestively association between air pollution and the risk of acute upper respiratory infections and intestinal infections, except for nitrogen dioxide.
As mentioned, exposure to PM is associated with upper and lower respiratory tract infections. The COVID-19 pandemic, caused by the novel severe acute respiratory syndrome coronavirus 2 (SARS-CoV-2), has resulted in historic numbers of infections and deaths worldwide over the last 3 years (2022). Consequently, an increasing number of studies have investigated associations between PM and COVID-19 infection in China, Italy, and the USA (7–9, 38). The mechanism of COVID-19 infection related to PM can be summarized as follows. (1) PM may be involved in different life cycle stages of COVID-19, including alteration of SARS-CoV-2 viral receptors and proteases required for entry (e.g., angiotensin-converting enzyme 2 (ACE2) and transmembrane protease serine type 2 (TMPRSS2), proteins and protease critical to SARS-CoV-2 entry into host cells) (39), inhibition of mucosal ciliary clearance, alteration of antiviral interferon production and viral replication (40). (2) PM may impair the immune system. PM-induced pro-inflammatory cytokine production, oxidative stress, and impaired airway immune function may lead to increased susceptibility to respiratory pathogens, which can increase the risk of COVID-19 pneumonia (41). For example, exposure to chemicals in PM damages lung epithelial cells, interferes with tight junctions between epithelial cells, increases permeability of airway and lung epithelial cells, and decreases protection against viral infections (42). Furthermore, substances such as heavy metals and polycyclic aromatic hydrocarbons promote the production of reactive oxygen species in lung cells (40), increasing susceptibility to further oxidative damage due to oxidative stress. Interestingly, our results, consistent with findings by Bontempi (43), revealed a closer correlation between PM2.5 and COVID-19 than with PM10. This may be because PM10 larger than 5 μm is unlikely to reach type II alveolar cells, where the ACE2 receptor for cellular entry is primarily located (44).
The relationship between NO2 and COVID-19 has been reported as positive in China, Europe, and the United States (9). However, contrasting evidence suggesting a negative or insignificant association between NO2 and COVID-19 also exists (45, 46). The potential effect of NO2 exposure remains uncertain. Studies on nitrogen oxides (NOx) and COVID-19 are scarce. We demonstrated that there is a significant association between NOx and COVID-19. This may be determined by the next mentioned physicochemical properties of NOx that are not readily soluble in water. In addition, a study by Pfeffer et al. discovered that higher environmental NOx levels were linked to exacerbated viral lung infections, which increases the susceptibility to concurrent bacterial infections and enhances its severity (47).
Pneumonia has been a cause of morbidity and mortality throughout human history (48). Bacterial pneumonia represents the most prevalent manifestation of pneumonia, characterized by inflammation affecting the terminal airways, alveoli, or interstitial spaces because of pathogenic microorganism infections (49). Pathogenetic investigations have indicated Streptococcus pneumoniae as potentially the most widespread bacterial agent responsible for pneumonia on a global scale (50). Over the past few decades, the rise in mortality rates associated with bacterial pneumonia has been attributed, in part, to worsening air quality and the proliferation of environmental hazards. These factors facilitate pathogen transmission, often synergizing with particulate matter (PM) (51). Air pollution poses a significant challenge to public health, exacerbating the potential for pathogen epidemics.
Our study establishes a potential causal relationship between PM2.5, PM2.5–10, PM10, and nitrogen oxides (mainly nitric oxide), all of which exhibit associations with bacterial pneumonia. It is widely acknowledged that respirable particles encompass a complex amalgamation of ions, organic compounds, metals, carbonaceous matter, and other constituents (52). The composition and relative abundance of these components are primarily contingent upon factors such as source origin, climatic conditions, topography, and other environmental variables (53). Consequently, respirable particles may exhibit varying degrees of solubility, ranging from partial solubility to complete insolubility (54). Furthermore, nitrogen oxides (mainly nitric oxide) possess limited solubility and does not undergo reactions with water. These physical properties, to some extent, contribute to the characteristics of these air pollutants in the context of lower respiratory tract infections. Currently, a plethora of epidemiological and mechanistic studies lends support to our conclusion. Notably, a meta-analysis has demonstrated a causal relationship between PM2.5 exposure and acute lower respiratory tract infections (55).
In the case of Chile, respirable particles have been associated with an elevated frequency of emergency room visits among children under the age of 2 experiencing lower respiratory symptoms (56). Conversely, no significant association has been observed between indoor NO2 concentrations and the incidence or severity of respiratory illnesses in infants (57). Furthermore, urban PM exposure can promote bacterial adherence to human respiratory epithelial cells by impairing mucus cilia activity in airway mucosa (58, 59), as well as promoting bacterial adherence to human respiratory epithelial cells (60) to enhance bacterial colonization of the host lower respiratory tract.
On the other hand, our findings indicate that only NO2 suggestively constitutes a risk factor for upper respiratory tract infections, with its soluble nature dictating the characteristics of this air pollutant, primarily impacting the upper respiratory tract (54). In a study conducted by Arbex et al. (61), it was observed that a mere 10 μg/m3 rise in NO2 concentration correlated with a 0.63% increase in visits for upper respiratory tract infections. Likewise, two researches conducted in China (62, 63) reported that each 10 μg/m3 increment in NO2 levels corresponded to a 1.00% increase in emergency room visits for upper respiratory tract infections, along with a substantial 11.27% surge in pediatric emergency room visits related to upper respiratory tract infections. However, it is important to note that while NO2 may not directly enter the pulmonary system, its potent oxidizing properties trigger an inflammatory response, subsequently activating the body's immune system and affecting the entire respiratory system (64).
The SNP rs12203592, located in the IRF4 gene intron, is linked to pigmentation traits, hematological traits, squamous cell carcinoma, and smoking cessation. A multi-ethnic GWAS identified it as a novel lung cancer locus. It also increases the risk of invasive aspergillosis post-hematopoietic stem cell transplantation by modulating IRF4 mRNA expression and immune responses (65, 66). Another SNP, rs1537371, in the CDKN2B-AS1 gene, is linked to cardiovascular diseases like coronary artery disease (67, 68). SNPs in the 9p21 region, such as rs1537371 and rs1333049, are significantly associated with an increased risk of cardiovascular disease (69). This study finds rs1537371 and rs12203592 significantly associated with air pollution-related COVID-19 and bacterial pneumonia risks, suggesting pleiotropic effects (70).
These findings offer potential causal relationship between air pollutant exposure and the incidence of respiratory infections. Notably, it is of concern that a staggering 91% of the world's population resides in regions where air pollution exceeds the limits recommended by the World Health Organization (WHO) (source: https://www.who.int/news-room/fact-sheets/detail/ambient-(outdoor)-air-quality-and-health). Therefore, taking steps to curtail air pollution has the potential to yield significant reductions in the global burden of respiratory infectious diseases. Consequently, our data underscore the importance of advocating for worldwide initiatives aimed at reducing air pollution and transitioning to more sustainable energy sources that yield cleaner air.
There are some strengths should be mentioned as following: Firstly, this is the first MR analysis to examine the casual effect of air pollution on infections using large-scale GWAS data. Comparing to the observational studies, MR analysis can largely overcome the confounders with random assignment of an individual's genetic variants at conception. Moreover, the risk of reverse causation could also be minimized. Secondly, we conducted sensitivity analysis both with and without outliers to detect any coincidental effects, and all models exhibited no directional pleiotropy. Finally, F statistics were consistently >10, indicating a robust correlation between SNPs and exposures with the sufficient statistical power and minimizing the possibility of weak instrumental variable bias.
There are some shortcomings in this MR study. Firstly, there was heterogeneity among our results. Due to the GWAS data, any potential non-linear relationships or stratification effects which varies by health status, age or sex cannot be examined which may be the resource of heterogeneity. Secondly, our study could not rule out the effect of canalization (i.e., dilution of the gene-exposure association) and thus the estimate might be inflated (71). Thirdly, the association between air pollution and different infections subtypes was not explored. Additionally, instances where air pollution levels exceed the standard cannot be entirely discounted, as such occurrences may introduce confounding factors that could bias our MR estimates. Finally, our datasets included the European populations, limiting the applicability of our results to non-European populations. Further studies are needed to verify the applicability of these results in other populations and ethnicities.
5 Conclusion
In conclusion, we demonstrated a causal association between PM2.5, PM2.5–10, and nitrogen oxides on Corona Virus Disease 2019. Additionally, PM2.5, PM2.5–10, PM10, and Nitrogen oxides will increase the risk of bacterial pneumonia. However, air pollution, except for nitrogen dioxide, was not associated with the risk of acute upper respiratory infections. Overall, more work is needed for policy formulation to reduce air pollution and the emission of toxic and harmful gases.
Data availability statement
The original contributions presented in the study are included in the article/Supplementary material, further inquiries can be directed to the corresponding author.
Author contributions
SY: Conceptualization, Data curation, Formal analysis, Investigation, Methodology, Project administration, Resources, Software, Supervision, Validation, Visualization, Writing – original draft, Writing – review & editing. TT: Conceptualization, Data curation, Formal analysis, Investigation, Project administration, Resources, Supervision, Validation, Writing – original draft, Writing – review & editing. HW: Conceptualization, Data curation, Formal analysis, Investigation, Project administration, Resources, Supervision, Validation, Writing – original draft, Writing – review & editing. ZL: Data curation, Resources, Supervision, Validation, Writing – review & editing. MW: Data curation, Resources, Supervision, Validation, Writing – review & editing. KN: Conceptualization, Data curation, Formal analysis, Investigation, Methodology, Project administration, Resources, Software, Supervision, Validation, Visualization, Writing – review & editing.
Funding
The author(s) declare financial support was received for the research, authorship, and/or publication of this article. This research was supported by the Zhejiang Provincial Natural Science Foundation of China under Grant No. LQ23G030008.
Conflict of interest
The authors declare that the research was conducted in the absence of any commercial or financial relationships that could be construed as a potential conflict of interest.
Publisher's note
All claims expressed in this article are solely those of the authors and do not necessarily represent those of their affiliated organizations, or those of the publisher, the editors and the reviewers. Any product that may be evaluated in this article, or claim that may be made by its manufacturer, is not guaranteed or endorsed by the publisher.
Supplementary material
The Supplementary Material for this article can be found online at: https://www.frontiersin.org/articles/10.3389/fpubh.2024.1409640/full#supplementary-material
References
1. Naghavi M, Abajobir AA, Abbafati C, Abbas KM, Abd-Allah F, Abera SF, et al. Global, regional, and national age-sex specific mortality for 264 causes of death, 1980–2016: a systematic analysis for the Global Burden of Disease Study 2016. Lancet. (2017) 390:1151–210. doi: 10.1016/S0140-6736(17)32152-9
2. Vlieg-Boerstra B, de Jong N, Meyer R, Agostoni C, De Cosmi V, Grimshaw K, et al. Nutrient supplementation for prevention of viral respiratory tract infections in healthy subjects: a systematic review and meta-analysis. Allergy. (2022) 77:1373–88. doi: 10.1111/all.15136
3. Li S, Tong J, Li H, Mao C, Shen W, Lei Y, et al. L. pneumophila infection diagnosed by tNGS in a lady with lymphadenopathy. Infect Drug Resist. (2023) 16:4435–42. doi: 10.2147/IDR.S417495
4. Glencross DA, Ho TR, Camiña N, Hawrylowicz CM, Pfeffer PE. Air pollution and its effects on the immune system. Free Radic Biol Med. (2020) 151:56–68. doi: 10.1016/j.freeradbiomed.2020.01.179
5. Rentschler J, Leonova N. Global air pollution exposure and poverty. Nat Commun. (2023) 14:4432. doi: 10.1038/s41467-023-39797-4
6. Kim KH, Kabir E, Kabir S. A review on the human health impact of airborne particulate matter. Environ Int. (2015) 74:136–43. doi: 10.1016/j.envint.2014.10.005
7. Zhu Y, Xie J, Huang F, Cao L. Association between short-term exposure to air pollution and COVID-19 infection: evidence from China. Sci Total Environ. (2020) 727:138704. doi: 10.1016/j.scitotenv.2020.138704
8. Fattorini D, Regoli F. Role of the chronic air pollution levels in the Covid-19 outbreak risk in Italy. Environ Pollut. (2020) 264:114732. doi: 10.1016/j.envpol.2020.114732
9. Copat C, Cristaldi A, Fiore M, Grasso A, Zuccarello P, Signorelli SS, et al. The role of air pollution (PM and NO(2)) in COVID-19 spread and lethality: a systematic review. Environ Res. (2020) 191:110129. doi: 10.1016/j.envres.2020.110129
10. Emdin CA, Khera AV, Kathiresan S. Mendelian randomization. Jama. (2017) 318:1925–6. doi: 10.1001/jama.2017.17219
11. Sheehan NA, Didelez V, Burton PR, Tobin MD. Mendelian randomisation and causal inference in observational epidemiology. PLoS Med. (2008) 5:e177. doi: 10.1371/journal.pmed.0050177
12. Skrivankova VW, Richmond RC, Woolf BAR, Davies NM, Swanson SA, VanderWeele TJ, et al. Strengthening the reporting of observational studies in epidemiology using mendelian randomisation (STROBE-MR): explanation and elaboration. BMJ. (2021) 375:n2233. doi: 10.1136/bmj.n2233
13. Bycroft C, Freeman C, Petkova D, Band G, Elliott LT, Sharp K, et al. The UK Biobank resource with deep phenotyping and genomic data. Nature. (2018) 562:203–9. doi: 10.1038/s41586-018-0579-z
14. Eeftens M, Beelen R, de Hoogh K, Bellander T, Cesaroni G, Cirach M, et al. Development of Land Use Regression models for PM(25), PM(25) absorbance, PM(10) and PM(coarse) in 20 European study areas; results of the ESCAPE project. Environ Sci Technol. (2012) 46:11195–205. doi: 10.1021/es301948k
15. COVID-19 Host Genetics Initiative. The COVID-19 Host Genetics Initiative, a global initiative to elucidate the role of host genetic factors in susceptibility and severity of the SARS-CoV-2 virus pandemic. Eur J Hum Genet. (2020) 28:715–718. doi: 10.1038/s41431-020-0636-6
16. Flatby HM, Ravi A, Damås JK, Solligård E, Rogne T. Circulating levels of micronutrients and risk of infections: a Mendelian randomization study. BMC Med. (2023) 21:84. doi: 10.1186/s12916-023-02780-3
17. Zou XL, Wang S, Wang LY, Xiao LX, Yao TX, Zeng Y, et al. Childhood obesity and risk of stroke: a mendelian randomisation analysis. Front Genet. (2021) 12:727475. doi: 10.3389/fgene.2021.727475
18. Burgess S, Butterworth A, Thompson SG. Mendelian randomization analysis with multiple genetic variants using summarized data. Genet Epidemiol. (2013) 37:658–65. doi: 10.1002/gepi.21758
19. Sun M, Gao M, Luo M, Wang T, Zhong T, Qin J. Association between air pollution and primary liver cancer in European and east Asian populations: a Mendelian randomization study. Frontiers in public health. (2023) 11:1212301. doi: 10.3389/fpubh.2023.1212301
20. Park S, Lee S, Kim Y, Lee Y, Kang MW, Kim K, et al. Atrial fibrillation and kidney function: a bidirectional Mendelian randomization study. Eur Heart J. (2021) 42:2816–23. doi: 10.1093/eurheartj/ehab291
21. Stefan N. Metabolic disorders, COVID-19 and vaccine-breakthrough infections. Nat Rev Endocrinol. (2022) 18:75–6. doi: 10.1038/s41574-021-00608-9
22. Alberca RW, Oliveira LM, Branco A, Pereira NZ, Sato MN. Obesity as a risk factor for COVID-19: an overview. Crit Rev Food Sci Nutr. (2021) 61:2262–76. doi: 10.1080/10408398.2020.1775546
23. Chen Y, Klein SL, Garibaldi BT Li H, Wu C, Osevala NM Li T, Margolick JB, et al. Aging in COVID-19: vulnerability, immunity and intervention. Ageing Res Rev. (2021) 65:101205. doi: 10.1016/j.arr.2020.101205
24. Badell ML, Dude CM, Rasmussen SA, Jamieson DJ. Covid-19 vaccination in pregnancy. BMJ. (2022) 378:e069741. doi: 10.1136/bmj-2021-069741
25. Mandell LA, Niederman MS. Aspiration pneumonia. N Engl J Med. (2019) 380:651–63. doi: 10.1056/NEJMra1714562
26. Aliberti S, Dela Cruz CS, Amati F, Sotgiu G, Restrepo MI. Community-acquired pneumonia. Lancet. (2021) 398:906–19. doi: 10.1016/S0140-6736(21)00630-9
27. Lai CC, Wu FT Ji DD, Mu JJ, Yang JR, Chiu KT, Lin WY Li CY, et al. Gastroenteritis in a Taipei emergency department: aetiology and risk factors. Clin Microbiol Infect. (2011) 17:1071–7. doi: 10.1111/j.1469-0691.2010.03377.x
28. García Rodríguez LA, Ruigómez A, Panés J. Use of acid-suppressing drugs and the risk of bacterial gastroenteritis. Clin Gastroenterol Hepatol. (2007) 5:1418–23. doi: 10.1016/j.cgh.2007.09.010
29. Staley JR, Blackshaw J, Kamat MA, Ellis S, Surendran P, Sun BB, et al. PhenoScanner: a database of human genotype-phenotype associations. Bioinformatics. (2016) 32:3207–9. doi: 10.1093/bioinformatics/btw373
30. Levin MG, Judy R, Gill D, Vujkovic M, Verma SS, Bradford Y, et al. Genetics of height and risk of atrial fibrillation: A Mendelian randomization study. PLoS Med. (2020) 17:e1003288. doi: 10.1371/journal.pmed.1003288
31. Palmer TM, Lawlor DA, Harbord RM, Sheehan NA, Tobias JH, Timpson NJ, et al. Using multiple genetic variants as instrumental variables for modifiable risk factors. Stat Methods Med Res. (2012) 21:223–42. doi: 10.1177/0962280210394459
32. Zheng J, Baird D, Borges MC, Bowden J, Hemani G, Haycock P, et al. Recent developments in Mendelian randomization studies. Curr Epidemiol Rep. (2017) 4:330–45. doi: 10.1007/s40471-017-0128-6
33. Yang J, Ferreira T, Morris AP, Medland SE, Madden PA, Heath AC, et al. Conditional and joint multiple-SNP analysis of GWAS summary statistics identifies additional variants influencing complex traits. Nat Genet. (2012) 44:369–375, s361–363. doi: 10.1038/ng.2213
34. Bowden J, Davey Smith G, Haycock PC, Burgess S. Consistent estimation in mendelian randomization with some invalid instruments using a weighted median estimator. Genet Epidemiol. (2016) 40:304–14. doi: 10.1002/gepi.21965
35. Bowden J, Del Greco MF, Minelli C, Davey Smith G, Sheehan N, Thompson J, et al. A framework for the investigation of pleiotropy in two-sample summary data Mendelian randomization. Stat Med. (2017) 36:1783–802. doi: 10.1002/sim.7221
36. Bowden J, Davey Smith G, Burgess S. Mendelian randomization with invalid instruments: effect estimation and bias detection through Egger regression. Int J Epidemiol. (2015) 44:512–25. doi: 10.1093/ije/dyv080
37. Verbanck M, Chen CY, Neale B, Do R. Detection of widespread horizontal pleiotropy in causal relationships inferred from Mendelian randomization between complex traits and diseases. Nat Genet. (2018) 50:693–8. doi: 10.1038/s41588-018-0099-7
38. Wu X, Nethery RC, Sabath BM, Braun D, Dominici F. Exposure to air pollution and COVID-19 mortality in the United States: a nationwide cross-sectional study. medRxiv. (2020). doi: 10.1101/2020.04.05.20054502
39. Hoffmann M, Kleine-Weber H, Schroeder S, Krüger N, Herrler T, Erichsen S, et al. SARS-CoV-2 cell entry depends on ACE2 and TMPRSS2 and is blocked by a clinically proven protease inhibitor. Cell. (2020) 181:271–280.e278. doi: 10.1016/j.cell.2020.02.052
40. Woodby B, Arnold MM, Valacchi G. SARS-CoV-2 infection, COVID-19 pathogenesis, and exposure to air pollution: what is the connection? Ann N Y Acad Sci. (2021) 1486:15–38. doi: 10.1111/nyas.14512
41. Lei J, Chen R, Liu C, Zhu Y, Xue X, Jiang Y, et al. Fine and coarse particulate air pollution and hospital admissions for a wide range of respiratory diseases: a nationwide case-crossover study. Int J Epidemiol. (2023) 52:715–26. doi: 10.1093/ije/dyad056
42. Kokavec J, Min SH, Tan MH, Gilhotra JS, Newland HS, Durkin SR, et al. Biochemical analysis of the living human vitreous. Clin Exp Ophthalmol. (2016) 44:597–609. doi: 10.1111/ceo.12732
43. Bontempi E. Commercial exchanges instead of air pollution as possible origin of COVID-19 initial diffusion phase in Italy: more efforts are necessary to address interdisciplinary research. Environ Res. (2020) 188:109775. doi: 10.1016/j.envres.2020.109775
44. Hou YJ, Okuda K, Edwards CE, Martinez DR, Asakura T, Dinnon KH, et al. SARS-CoV-2 reverse genetics reveals a variable infection gradient in the respiratory tract. Cell. (2020) 182:429–446.e414. doi: 10.1016/j.cell.2020.05.042
45. Bashir MF, Ma BJ, Bilal, Komal B, Bashir MA, Farooq TH, et al. Correlation between environmental pollution indicators and COVID-19 pandemic: a brief study in Californian context. Environ Res. (2020) 187:109652. doi: 10.1016/j.envres.2020.109652
46. Zoran MA, Savastru RS, Savastru DM, Tautan MN. Assessing the relationship between ground levels of ozone (O(3)) and nitrogen dioxide (NO(2)) with coronavirus (COVID-19) in Milan, Italy. Sci Total Environ. (2020) 740:140005. doi: 10.1016/j.scitotenv.2020.140005
47. Pfeffer PE, Donaldson GC, Mackay AJ, Wedzicha JA. Increased chronic obstructive pulmonary disease exacerbations of likely viral etiology follow elevated ambient nitrogen oxides. Am J Respir Crit Care Med. (2019) 199:581–91. doi: 10.1164/rccm.201712-2506OC
48. Grousd JA, Rich HE, Alcorn JF. Host-pathogen interactions in gram-positive bacterial pneumonia. Clin Microbiol Rev. (2019) 32:10-128. doi: 10.1128/CMR.00107-18
49. Torres A, Cilloniz C, Niederman MS, Menéndez R, Chalmers JD, Wunderink RG, et al. Pneumonia. Nat Rev Dis Primers. (2021) 7:25. doi: 10.1038/s41572-021-00259-0
50. Shann F, Gratten M, Germer S, Linnemann V, Hazlett D, Payne R. Aetiology of pneumonia in children in Goroka Hospital, Papua New Guinea. Lancet. (1984) 2:537–41. doi: 10.1016/S0140-6736(84)90764-5
51. Zhang F, Zhou Y, Ding J. The current landscape of microRNAs (miRNAs) in bacterial pneumonia: opportunities and challenges. Cell Mol Biol Lett. (2022) 27:70. doi: 10.1186/s11658-022-00368-y
52. Aryal A, Harmon AC, Dugas TR. Particulate matter air pollutants and cardiovascular disease: strategies for intervention. Pharmacol Ther. (2021) 223:107890. doi: 10.1016/j.pharmthera.2021.107890
53. Fu C, Kuang D, Zhang H, Ren J, Chen J. Different components of air pollutants and neurological disorders. Front Public Health. (2022) 10:959921. doi: 10.3389/fpubh.2022.959921
54. Schraufnagel DE, Balmes JR, Cowl CT, De Matteis S, Jung SH, Mortimer K, et al. Air pollution and noncommunicable diseases: a review by the forum of international respiratory societies' environmental committee, part 1: the damaging effects of air pollution. Chest. (2019) 155:409–16. doi: 10.1016/j.chest.2018.10.042
55. Mehta S, Shin H, Burnett R, North T, Cohen AJ. Ambient particulate air pollution and acute lower respiratory infections: a systematic review and implications for estimating the global burden of disease. Air Qual Atmos Health. (2013) 6:69–83. doi: 10.1007/s11869-011-0146-3
56. Ostro BD, Eskeland GS, Sanchez JM, Feyzioglu T. Air pollution and health effects: a study of medical visits among children in Santiago, Chile. Environ Health Perspect. (1999) 107:69–73. doi: 10.1289/ehp.9910769
57. Fox NL, Sexton M, Hebel JR. Prenatal exposure to tobacco: I. Effects on physical growth at age three. Int J Epidemiol. (1990) 19:66–71. doi: 10.1093/ije/19.1.66
58. Alanin MC, Nielsen KG, von Buchwald C, Skov M, Aanaes K, Høiby N, et al. A longitudinal study of lung bacterial pathogens in patients with primary ciliary dyskinesia. Clin Microbiol Infect. (2015) 21:1093.e1091–1097. doi: 10.1016/j.cmi.2015.08.020
59. Carvalho-Oliveira R, Pires-Neto RC, Bustillos JO, Macchione M, Dolhnikoff M, Saldiva PH, et al. Chemical composition modulates the adverse effects of particles on the mucociliary epithelium. Clinics. (2015) 70:706–13. doi: 10.6061/clinics/2015(10)09
60. Sacks D, Baxter B, Campbell BCV, Carpenter JS, Cognard C, Dippel D, et al. Multisociety consensus quality improvement revised consensus statement for endovascular therapy of acute ischemic stroke. Int J Stroke. (2018) 13:612–32. doi: 10.3174/ajnr.A5638
61. Arbex MA, Pereira LA, Carvalho-Oliveira R, Saldiva PH, Braga AL. The effect of air pollution on pneumonia-related emergency department visits in a region of extensive sugar cane plantations: a 30-month time-series study. J Epidemiol Community Health. (2014) 68:66974. doi: 10.1136/jech-2013-203709
62. Zheng PW, Wang JB, Zhang ZY, Shen P, Chai PF Li D, Jin MJ, et al. Air pollution and hospital visits for acute upper and lower respiratory infections among children in Ningbo, China: a time-series analysis. Environ Sci Pollut Res Int. (2017) 24:18860–9. doi: 10.1007/s11356-017-9279-8
63. Tam WW, Wong TW, Ng L, Wong SY, Kung KK, Wong AH. Association between air pollution and general outpatient clinic consultations for upper respiratory tract infections in Hong Kong. PLoS ONE. (2014) 9:e86913. doi: 10.1371/journal.pone.0086913
64. Manisalidis I, Stavropoulou E, Stavropoulos A, Bezirtzoglou E. Environmental and health impacts of air pollution: a review. Front Public Health. (2020) 8:14. doi: 10.3389/fpubh.2020.00014
65. Lupiañez CB, Villaescusa MT, Carvalho A, Springer J, Lackner M, Sánchez-Maldonado JM, et al. Common genetic polymorphisms within NFκB-related genes and the risk of developing invasive aspergillosis. Front Microbiol. (2016) 7:1243. doi: 10.3389/fmicb.2016.01243
66. Boddicker RL, Kip NS, Xing X, Zeng Y, Yang ZZ, Lee JH, et al. The oncogenic transcription factor IRF4 is regulated by a novel CD30/NF-κB positive feedback loop in peripheral T-cell lymphoma. Blood. (2015) 125:3118–27. doi: 10.1182/blood-2014-05-578575
67. Vargas JD, Manichaikul A, Wang XQ, Rich SS, Rotter JI, Post WS, et al. Detailed analysis of association between common single nucleotide polymorphisms and subclinical atherosclerosis: the multi-ethnic study of atherosclerosis. Data in brief. (2016) 7:229–42. doi: 10.1016/j.dib.2016.01.048
68. O'Donnell CJ, Cupples LA, D'Agostino RB, Fox CS, Hoffmann U, Hwang SJ, et al. Genome-wide association study for subclinical atherosclerosis in major arterial territories in the NHLBI's framingham heart study. BMC Med Genet. (2007) 8 Suppl 1:S4. doi: 10.1186/1471-2350-8-S1-S4
69. Cao XL, Yin RX, Huang F, Wu JZ, Chen WX. Chromosome 9p21 and ABCA1 genetic variants and their interactions on coronary heart disease and ischemic stroke in a Chinese han population. Int J Mol Sci. (2016) 17:586. doi: 10.3390/ijms17040586
70. Zhang EB, Kong R, Yin DD, You LH, Sun M, Han L, et al. Long noncoding RNA ANRIL indicates a poor prognosis of gastric cancer and promotes tumor growth by epigenetically silencing of miR-99a/miR-449a. Oncotarget. (2014) 5:2276–92. doi: 10.18632/oncotarget.1902
Keywords: air pollution, infections, pneumonia, Mendelian randomization, casual effect
Citation: Yang S, Tong T, Wang H, Li Z, Wang M and Ni K (2024) Causal relationship between air pollution and infections: a two-sample Mendelian randomization study. Front. Public Health 12:1409640. doi: 10.3389/fpubh.2024.1409640
Received: 30 March 2024; Accepted: 16 July 2024;
Published: 01 August 2024.
Edited by:
Dimirios Nikolopoulos, University of West Attica, GreeceReviewed by:
Sofina Begum, Harvard Medical School, United StatesBangjian He, Zhejiang Chinese Medical University, China
Copyright © 2024 Yang, Tong, Wang, Li, Wang and Ni. This is an open-access article distributed under the terms of the Creative Commons Attribution License (CC BY). The use, distribution or reproduction in other forums is permitted, provided the original author(s) and the copyright owner(s) are credited and that the original publication in this journal is cited, in accordance with accepted academic practice. No use, distribution or reproduction is permitted which does not comply with these terms.
*Correspondence: Kaiwen Ni, nkw721@zju.edu.cn
†These authors have contributed equally to this work