- 1National Center for Respiratory Medicine, State Key Laboratory of Respiratory Health and Multimorbidity, Department of Pediatrics, China-Japan Friendship Hospital, National Clinical Research Center for Respiratory Diseases, Institute of Respiratory Medicine, Chinese Academy of Medical Sciences, Beijing, China
- 2Precision and Smart Imaging Laboratory, Beijing Friendship Hospital, Capital Medical University, Beijing, China
- 3Graduate School of Peking Union Medical College, Chinese Academy of Medical Sciences, Beijing, China
Objective: This study aims to explore the association between outdoor artificial light at night (ALAN) exposure and gestational diabetes mellitus (GDM).
Methods: This study is a retrospective case–control study. According with quantiles, ALAN has been classified into three categories (Q1-Q3). GDM was diagnosed through oral glucose tolerance tests. Conditional logistic regression models were used to evaluate the association between ALAN exposure and GDM risk. The odds ratio (OR) with 95% confidence interval (CI) was used to assess the association. Restricted cubic spline analysis (RCS) was utilized to investigate the no liner association between ALAN and GDM.
Results: A total of 5,720 participants were included, comprising 1,430 individuals with GDM and 4,290 matched controls. Pregnant women exposed to higher levels of ALAN during the first trimester exhibited an elevated risk of GDM compared to those with lower exposure levels (Q2 OR = 1.39, 95% CI 1.20–1.63, p < 0.001); (Q3 OR = 1.70, 95% CI 1.44–2.00, p < 0.001). Similarly, elevated ALAN exposure during the second trimester also conferred an increased risk of GDM (second trimester: Q2 OR = 1.70, 95% CI 1.45–1.98, p < 0.001; Q3 OR = 2.08, 95% CI 1.77–2.44, p < 0.001). RCS showed a nonlinear association between ALAN exposure and GDM risk in second trimester pregnancy, with a threshold value of 4.235.
Conclusion: Outdoor ALAN exposure during pregnancy is associated with an increased risk of GDM.
1 Introduction
Exposure to artificial light at night (ALAN) has emerged as a progressively ubiquitous environmental hazard within contemporary society (1). Over the past several decades, urbanization and shifts in modern lifestyle have led to a continuous escalation of ALAN in our daily lives (2). While ALAN offers convenience and safety, it also brings forth an array of potential health concerns (3).
It is worth noting that recent research has employed satellite remote sensing data to validate the correlations between ALAN and a range of human health issues, including obesity (4), metabolic syndrome (5), sleep disorder (6, 7), and cancer (8). Furthermore, emerging evidence suggests an association between ALAN and the risk of type 2 diabetes (Minjee (9–11)). However, the relationship between outdoor ALAN exposure and gestational diabetes mellitus (GDM) remains poorly understood.
The mechanisms through which ALAN impacts human health remain unclear; however, research indicates that ALAN can disrupt circadian rhythms in humans and other organisms, thereby influencing various physiological processes and behavioral patterns (12, 13). Exposure to ALAN may even lead to suppressed secretion of melatonin, a hormone that plays a crucial role in regulating sleep and other physiological functions (14). Furthermore, ALAN may impact the functioning of other endocrine systems, such as the secretion of adrenal corticosteroids and insulin regulation (15).
GDM is a condition characterized by abnormal blood glucose levels during pregnancy (16). Reports indicate that the prevalence of GDM varies across different countries and regions, with a notably higher incidence of 14.8% reported in China, making it a noteworthy public health concern in the country (17). This increased prevalence can primarily be attributed to behavioral and environmental risk factors (18). For mothers, having GDM can lead to heightened risks of pregnancy complications such as hypertension (19) and preterm birth (20), along with an elevated risk of developing type 2 diabetes later in life (21). Additionally, GDM can have enduring consequences for the newborn, including neonatal cardiovascular health (22) and respiratory distress syndrome (23). Consequently, the identification of potential risk factors for gestational diabetes is of paramount importance in mitigating the risks posed to both mothers and their offspring.
Pregnant women constitute a unique population group, as they are more susceptible to the influence of environmental factors during pregnancy due to hormonal effects (24). Current research suggests that exposure to ALAN may have adverse effects on fetal size and the metabolism of offspring (25, 26). Hence, this study postulates that ALAN among pregnant women may is the risk of GDM through alterations in circadian rhythms and metabolism. The primary objective of this study is to investigate the association between outdoor ALAN exposure and gestational diabetes, aiming to address existing knowledge gaps and offer pertinent public health recommendations.
2 Materials and methods
2.1 Study population
This retrospective case–control study was conducted at the China-Japan Friendship Hospital. The geographic distribution of the study participants is illustrated in Figure 1. Participants were selected based on specific inclusion criteria, which included: (1) residence in Beijing; (2) delivery at the China-Japan Friendship Hospital; (3) maternal age ≥ 18 years; (4) singleton pregnancies; (5) live-born infants. Exclusion criteria encompassed: (1) missing residential address (n = 1,122); (2) presence of complications during pregnancy, such as gestational hypertension, placental abruption, etc. (n = 320); (3) missing information on age, delivery date, last menstrual period (LMP) date, and other related data (n = 670). A 1:3 propensity score matching was performed based on nation and offspring sex to select the control group. The final study comprised 5,720 participants, and the workflow is depicted in Figure 2.
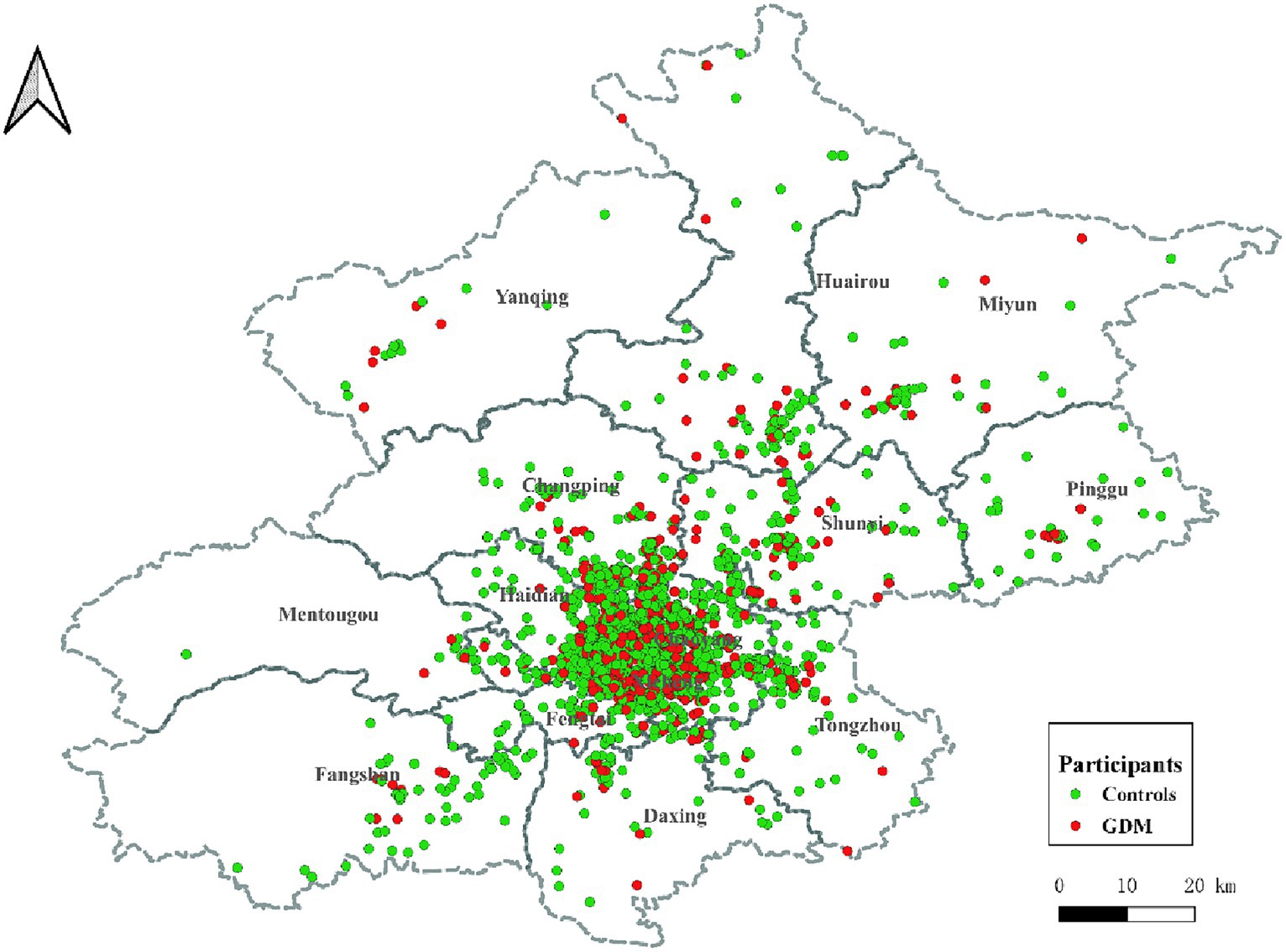
Figure 1. Geographical distribution of participants in Beijing. ALAN: artificial light at night; Red dots represent GDMs, and green dots represent controls. GDM, gestational diabetes mellitus.
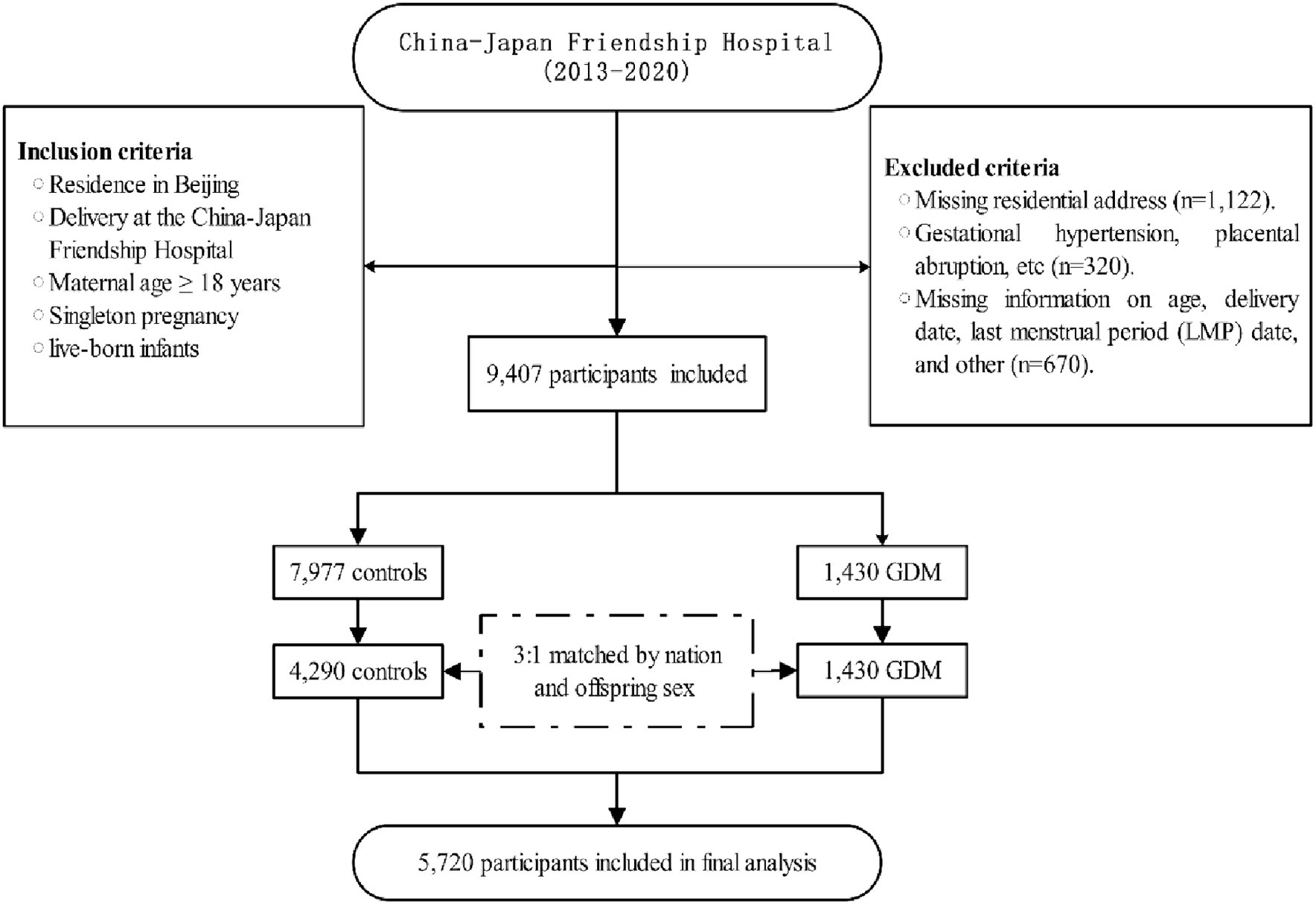
Figure 2. Flowchart of the study. LMP, Last Menstrual Period; GDM, Gestational diabetes mellitus; NDVI, normalized difference vegetation index; PM2.5, ambient fine particulate matter; PM10, ambient inhalable particulate matter.
The retrospective case–control study design precluded the acquisition of informed consent from the participants. Nevertheless, this approach aligns with the ethical review approved by the Ethics Committee of the China-Japan Friendship Hospital (Ethics Review Number: 2023-KY-137), which acknowledges the impracticality of obtaining informed consent in retrospective research studies.
2.2 Assessment of outdoor ALAN
In this study, ALAN measurements were obtained using the Suomi National Polar-Orbiting Partnership Visible Infrared Imaging Radiometer Suite (NPP-VIIRS), which offers superior spatial resolution, enhanced temporal resolution, an extended spectral range, and advanced calibration and correction when compared to the Operational Linescan System of Defense Meteorological Satellite Program (OLS-DMSP) (27). Commencing in April 2012, NPP-VIIRS captures data within the wavelength range of 500 nm to 900 nm, with a spatial resolution of 500 m × 500 m at the Equator (28). Monthly NPP-VIIRS nighttime light data for the period from 2013 to 2020 were obtained from the Earth Observation Group.1 The unit of measurement is nanowatts per square centimeter per steradian (nW/cm2/sr), which quantifies the radiative intensity per unit area, accounting for solid angles in all directions.
2.3 Outcomes and covariates
In this study, we directly acquired the diagnosis of GDM in participants from electronic health records. This diagnosis was based on the results of the 75 g oral glucose tolerance test (75 g OGTT) conducted on participants between gestational weeks 24–28. Participants were diagnosed with GDM if they met any of the following diagnostic criteria: fasting blood glucose level ≥ 5.1 mmol/L (92 mg/dL); 1-h blood glucose level ≥ 10.0 mmol/L (180 mg/dL); 2-h blood glucose level ≥ 8.5 mmol/L (153 mg/dL) (29). This study concurrently collected data on fetal sex and birth weight. Additionally, information on the following covariates was gathered: maternal race (Han, non-Han), age (years), parity (primiparous, multiparous), gravidity (1, 2, >2 times), pre-pregnancy body mass index (BMI, kg/m2), and conception season (Spring, Summer, Autumn, and Winter).
2.4 Other environmental variables
Given the role of environmental factors in GDM, we incorporated environmental covariates including inhalable particulate matter (PM10) and fine particulate matter (PM2.5), as well as green space, into the study. The data for PM2.5 and PM10 were sourced from the China High-resolution Air Pollutants (CHAP) database. PM2.5 and PM10 data were obtained using a spatiotemporal extreme random tree model that leveraged model data to fill spatial gaps in Moderate Resolution Imaging Spectroradiometer Multi-Angle Implementation of Atmospheric Correction Aerosol Optical Depth satellite products. This approach integrated ground observations, atmospheric reanalysis, emissions inventories, and other large-scale data sources, generating seamless nationwide surface PM2.5 and PM10 data from 2000 to 2021. The ten-fold cross-validation coefficient of determination (R2) for PM2.5 data was 0.92, with a root mean square error (RMSE) of 10.76 μg/m3 (30). For the PM10 data, the ten-fold cross-validation yielded an R2 of 0.9 and an RMSE of 21.12 μg/m3 (31). The Normalized Difference Vegetation Index (NDVI) was employed as a surrogate indicator for residential greenness. NDVI is a widely utilized metric in environmental research for quantifying the density and health status of vegetation in various regions (32). This index ranges from 0 to 1, where higher NDVI values indicate denser and healthier vegetation, while lower values suggest sparse or stressed vegetation (33). In our study, NDVI was estimated based on 16-day composite images from the NASA Terra Moderate Resolution Imaging Spectroradiometer satellite.2 After obtaining annual data for PM2.5, PM10, and NDVI, we performed weighting matching for the residential locations of pregnant women and computed annual prenatal environmental pollution exposures.
2.5 Exposure time window
Participants’ residential addresses were geocoded using Baidu Maps.3 Subsequently, we proceeded to estimate the average exposure levels during the first and second trimesters of pregnancy to investigate potential heterogeneity in the association between ALAN and GDM across different exposure windows. These exposure windows corresponded to the first and second trimesters of pregnancy, corresponding to 3 and 6 months after the last menstrual period, respectively.
2.6 Statistical analysis
Continuous variables, normally distributed, are presented as mean ± standard deviation, while categorical variables are presented as counts (percentages). Differences between groups for continuous variables were compared using t-tests or Wilcoxon tests. Differences between groups for categorical variables were compared using chi-square tests or Fisher’s exact tests.
We employed conditional logistic regression to assess the link between ALAN exposure and GDM, calculating odds ratios (ORs) with 95% confidence intervals (CIs). Initially, we established an unadjusted model, without considering any potential confounding factors. Subsequently, we adjusted for potential confounders including age, ethnicity, gravidity, parity, pre-pregnancy body mass index, and conception season. Covariate selection guided by Directed Acyclic Graph Analysis (Supplementary Figure S1). Finally, while controlling for potential confounding, we further controlled for PM2.5, PM10, and NDVI. Employing Pearson correlation analysis, we identified a strong correlation between PM2.5 and PM10 (correlation coefficient = 0.97, p < 0.001). To mitigate issues of multicollinearity, principal component analysis was utilized to reduce the dimensionality of PM2.5 and PM10, incorporating the first principal component (PC1), which accounted for 71.65% of the variance, into the final model as a substitute for both PM10 and PM2.5.
To investigate the association between exposure to ALAN and GDM, restricted cubic spline (RCS) analysis was utilized in this study. The analysis was focused on ALAN exposure in first and second trimester pregnancy, assessing its nonlinear relationship with the risk of GDM. Additionally, we conducted a stratified analysis by infant sex to examine potential effect modification and assessed the interaction between ALAN and infant sex. The inclusion of interaction terms in the model was employed to assess whether fetal sex modifies the effect of exposure on the risk of GDM.
All statistical analyses were performed using R (version 4.1.0, available at https://www.r-project.org/).
2.7 Sensitivity analyses
This study conducted multiple sensitivity analyses: (1) ALAN per SD increase was employed to assess the relationship with GDM (Supplementary Tables S1, S2). (2) Evaluation of Han ethnicity participants was performed to assess potential influences related to ethnicity (Supplementary Table S3). (3) Similar analyses were conducted within the primiparous population to assess potential differences that might arise from multiple pregnancies (Supplementary Table S4). (4) Excluding participants with pre-existing diabetes prior to pregnancy (Supplementary Table S5). (5) Using linear regression to investigate the effect of ALAN exposure on participants’ fasting blood glucose levels (Supplementary Table S6).
3 Results
3.1 Characteristics of the study population
Table 1 provides an overview of the characteristics of pregnant women and newborns in the control group (n = 4,290) and GDM group (n = 1,430). While there were no significant differences in Han Chinese ethnicity between the group, the GDM group had a slightly higher mean age (GDM: 31.85 ± 3.96 years; Controls: 30.69 ± 3.41 years, p < 0.001). Furthermore, the GDM group showed a higher proportion of multiparous women (23.92% compared to 19.91% in the control group, p = 0.001). Gravidity distribution also significantly differed between the groups (p < 0.001). The distribution of neonatal sex was similar, with 51.40% males in the control group and 51.89% males in the GDM group. Additionally, there were slight differences in neonatal length (Control: 50.67 ± 2.39 cm; GDM: 50.47 ± 2.51 cm, p = 0.007), birth weight (Control: 3302.70 ± 479.89 g; GDM: 3270.36 ± 510.18 g, p = 0.030), and gestation duration (Control: 276.77 ± 12.90 days; GDM: 274.92 ± 33.59 days, p = 0.003) between the groups.
3.2 Distribution of environmental factors in different trimesters
Table 2 presents the differences in outdoor ALAN levels between the GDM and Control groups. There were no statistically significant differences in PM10 levels (Control: 102.85 ± 21.33 μg/m3; Case: 103.41 ± 20.70 μg/m3, p = 0.391) or PM2.5 levels (Control: 64.87 ± 17.72 μg/m3; Case: 65.90 ± 17.47 μg/m3, p = 0.054) between the two groups. Similarly, the NDVI showed no significant difference (Control: 0.32 ± 0.07; Case: 0.31 ± 0.07, p = 0.216). However, there were substantial differences in ALAN levels between the groups. In the first trimester (T1), ALAN levels were significantly higher in the GDM group (27.46 ± 16.86 nW/cm2/sr) compared to the Control group (24.42 ± 16.64 nW/cm2/sr, p < 0.001). This trend was consistent in the second trimester (T2) (Control: 24.69 ± 16.81 nW/cm2/sr; Case: 27.34 ± 16.61 nW/cm2/sr, p < 0.001).
3.3 Association of outdoor ALAN exposure in different trimesters with GDM
In Table 3, we present the results of conditional logistic regression models examining the association between outdoor ALAN exposure and the risk of GDM across various trimesters (T1 and T2). In the initial unadjusted model (Model 1), participants in the second (Q2) and third (Q3) quartiles of ALAN exposure exhibited significantly elevated odds of developing GDM compared to those in the first quartile (Q1) during all trimesters (all p-values <0.001). These results remained consistent after accounting for potential confounders. Specifically, for the first trimester, the ORs were as follows: Q2 OR = 1.39 (95%CI 1.20–1.63, p < 0.001), Q3 OR = 1.70 (95%CI 1.44, 2.00, p < 0.001). In the second trimester, the ORs were: Q2 OR = 1.70 (95%CI 1.45–1.98, p < 0.001), Q3 OR = 2.08 (95%CI 1.77–2.44, p < 0.001). No significant interaction between ALAN exposure and sex was observed across all models. Table 4 presents the sex-specific associations of ALAN exposure with the risk of GDM across different trimesters, along with tests for interaction. ALAN exposure exhibited consistent associations with GDM risk across trimesters, particularly among females. In our study, RCS analysis showed no significant nonlinear relationship between ALAN exposure and GDM risk in first trimester pregnancy. However, a significant nonlinear association was found in second trimester pregnancy, with a threshold value of 4.235 (Figure 3).
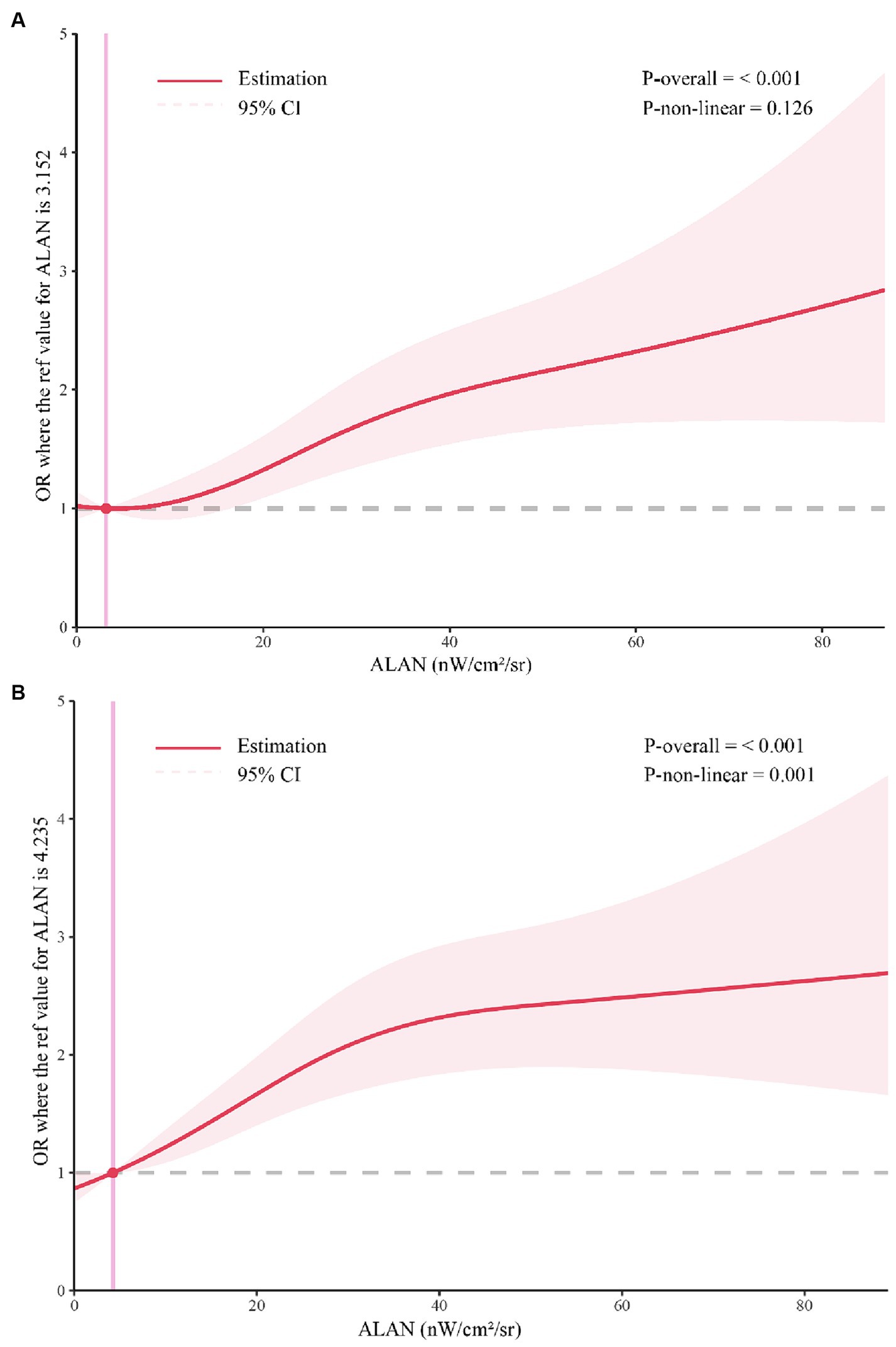
Figure 3. Restricted cubic spline analysis. (A) The association between first-trimester ALAN and GDM; (B) The relationship between second trimester ALAN and GDM; ALAN, Artificial Light at Night; GDM, Gestational Diabetes Mellitus.
4 Discussion
To investigate the association between outdoor ALAN exposure and GDM, we conducted a retrospective case–control study. Our study found a significant association between exposure to outdoor ALAN during pregnancy and an increased risk of GDM after adjusting for confounding factors. Furthermore, the association between outdoor ALAN and the risk of GDM did not differ between male and female infants. Our findings provide evidence supporting the role of outdoor ALAN in the risk of GDM among pregnant women.
In recent decades, the impact of ALAN on human health has gained global attention. Numerous studies have investigated the associations between ALAN exposure and chronic conditions such as cardiovascular diseases (34), obesity (35), and mental disorders (36). Recent research has suggested that exposure to outdoor ALAN may increase the risk of type 2 diabetes mellitus (T2DM) (Minjee (9, 10)). Furthermore, a cross-sectional study has shown a significant association between long-term exposure to higher-intensity outdoor ALAN and an increased risk of impaired glucose metabolism (11). Recent studies have elucidated the relationship between ALAN and GDM. In the United States, the risk associated with GDM has been correlated with pre-sleep exposure to light, as measured by wrist-worn activity monitors (37). Consistent with our findings, a prospective cohort study in Sichuan Province, China, utilizing satellite data to estimate outdoor ALAN exposure, offered a broader perspective on environmental exposure (38). Furthermore, a study conducted in Hefei City revealed that outdoor ALAN was associated with elevated early-pregnancy glucose homeostasis markers, yet it did not correlate with GDM risk (39). The variability in these findings may be attributed to differences in study populations and geographical locations. Our research, conducted in Beijing, a major metropolitan area, underscores the significant public health implications of addressing light pollution in densely populated urban environments. Moreover, our study surpassed traditional methods by thoroughly adjusting for critical environmental variables, including PM2.5, PM10, and NDVI, thereby reinforcing the robustness and credibility of our findings.
Exploring the critical windows of association between maternal ALAN exposure and the risk of GDM is of paramount importance for devising targeted intervention measures. The early and mid-stages of pregnancy are crucial periods for embryonic and fetal development, being particularly susceptible to external environmental influences (40). In our study, we observed that pregnant women exposed to higher levels of ALAN during the first and second trimesters exhibited an increased risk of GDM. However, considering the timing of GDM diagnosis (41), the relationship between ALAN exposure during the second trimester of pregnancy and GDM may be subject to constraints, necessitating further investigation.
The mechanisms underlying the relationship between ALAN exposure during pregnancy and the risk of GDM remain poorly understood. Several potential mechanisms may be involved. Firstly, ALAN exposure could potentially impact the risk of GDM by disrupting the circadian rhythms of pregnant women. Circadian rhythm regulation during pregnancy is critical for normal fetal and maternal physiological processes (42). ALAN may induce circadian rhythm disruption (43), leading to sleep disturbances and reduced sleep quality among pregnant women, consequently increasing the risk of GDM. Secondly, hormonal changes may play a significant role. ALAN exposure may influence hormone levels in pregnant women (44), particularly melatonin, a hormone crucial for regulating circadian rhythms during pregnancy (45). ALAN exposure might suppress melatonin secretion, potentially affecting maternal physiology and fetal development negatively. Lastly, ALAN exposure may contribute to an elevated risk of GDM by provoking alterations in inflammation and immune responses. Animal experiments have demonstrated that prolonged illumination can lead to changes in both the immune system and inflammatory processes (46). Although these mechanisms remain multifaceted and not fully elucidated, further research is needed to unravel these intricate pathways. In-depth investigations in both laboratory and epidemiological settings will contribute to a better understanding of the relationship between ALAN exposure and GDM, offering more precise directions for future intervention strategies.
This study has several limitations that warrant discussion. Firstly, in our research, we estimated outdoor ALAN exposure during pregnancy using high-resolution satellite images. However, we lacked data on indoor light exposure and whether participants used blackout curtains during the night, which could potentially lead to exposure misclassification. Future studies should consider collecting information on both indoor and outdoor light exposure. Secondly, while we adjusted for environmental confounders related to GDM, such as environmental particulate matter (47) and greenness (48) at the residential area, we did not account for other potential confounding factors, such as temperature (49), household income and education level. The absence of this information needs to be addressed and improved in future research. Thirdly, our study adopted a retrospective case–control study design, limiting the ability to establish causality between ALAN exposure and GDM. Therefore, the relationship between ALAN and GDM needs further confirmation through prospective study designs. Fourthly, the annual inclusion of study participants was not uniform (Supplementary Table S7), which was due to the COVID-19 pandemic. Although the ratio of cases to controls remained consistent, this could potentially introduce a certain degree of bias. Finally, our single-center study involved participants from the Beijing area with relatively higher socioeconomic status. Caution is advised when extending the study results to regions with lower economic development. Future research should validate these findings in diverse socioeconomic contexts.
Despite these limitations, our study possesses several strengths. Firstly, we elucidated the association between ALAN exposure during pregnancy and GDM, identifying the critical exposure window for this relationship. This finding provides valuable reference for targeted intervention measures during the identified exposure window. Additionally, we conducted a series of sensitivity analyses and performed stratified analyses by newborn sex to assess the consistency and robustness of this relationship.
5 Conclusion
In summary, our study reveals that higher outdoor ALAN exposure during pregnancy is associated with an elevated risk of GDM. These findings emphasize the need for targeted interventions and further research to better understand the mechanisms underlying this relationship and mitigate the health risks associated with light pollution during pregnancy.
Data availability statement
The raw data supporting the conclusions of this article will be made available by the authors, without undue reservation.
Ethics statement
The studies involving humans were approved by Ethics Committee of the China-Japan Friendship Hospital. The studies were conducted in accordance with the local legislation and institutional requirements. The ethics committee/institutional review board waived the requirement of written informed consent for participation from the participants or the participants' legal guardians/next of kin because this was a retrospective study and the ethics committee waived informed consent.
Author contributions
QS: Methodology, Writing – original draft, Writing – review & editing. FY: Investigation, Visualization, Writing – original draft, Writing – review & editing. JL: Investigation, Writing – original draft, Writing – review & editing. YY: Investigation, Writing – original draft, Writing – review & editing. QH: Software, Writing – original draft, Writing – review & editing YC: Data curation, Resources, Writing – original draft, Writing – review & editing. DLi: Software, Writing – original draft, Writing – review & editing. JG: Data Curation, Writing – original draft, Writing – review & editing. CW: Software, Writing – original draft, Writing – review & editing. DLv: Visualization, Writing – original draft, Writing – review & editing. LT: Investigation, Writing – original draft, Writing – review & editing. QZ: Conceptualization, Supervision, Writing – original draft, Writing – review & editing.
Funding
The author(s) declare that financial support was received for the research, authorship, and/or publication of this article. This study was funded by MOE Key Laboratory of Population Health Across Life Cycle (No: JK20225), Chinese Academy of Medical Sciences Clinical and Translational Medicine Research Project (No: 2021-I2M-C&T-B-089), Chinese Academy of Medical Sciences Innovation Fund for Medical Sciences (No: 2021-I2M-1-049), and a grant from State Key Laboratory of Resources and Environmental Information System.
Acknowledgments
We thank all the participants in this study.
Conflict of interest
The authors declare that the research was conducted in the absence of any commercial or financial relationships that could be construed as a potential conflict of interest.
Publisher’s note
All claims expressed in this article are solely those of the authors and do not necessarily represent those of their affiliated organizations, or those of the publisher, the editors and the reviewers. Any product that may be evaluated in this article, or claim that may be made by its manufacturer, is not guaranteed or endorsed by the publisher.
Supplementary material
The Supplementary material for this article can be found online at: https://www.frontiersin.org/articles/10.3389/fpubh.2024.1396198/full#supplementary-material
Abbreviations
ALAN, artificial light at night; GDM, gestational diabetes mellitus; CI, confidence interval; OR, odds ratio; OLS-DMSP, Operational Linescan System of Defense Meteorological Satellite Program; NPP-VIIRS, Suomi National Polar-Orbiting Partnership Visible Infrared Imaging Radiometer Suite; PM10, ambient inhalable particulate matter; PM2.5, ambient fine particulate matter; CHAP, China High Air Pollutants; NDVI, normalized difference vegetation index; RMSE, root mean square error; R2, coefficient of determination.
Footnotes
References
1. Bożejko, M, Tarski, I, and Małodobra-Mazur, M. Outdoor artificial light at night and human health: a review of epidemiological studies. Environ Res. (2023) 218:115049. doi: 10.1016/j.envres.2022.115049
2. Wang, T, Kaida, N, and Kaida, K. Effects of outdoor artificial light at night on human health and behavior: a literature review. Environ Pollut. (2023) 323:121321. doi: 10.1016/j.envpol.2023.121321
3. Stanhope, J, Liddicoat, C, and Weinstein, P. Outdoor artificial light at night: a forgotten factor in green space and health research. Environ Res. (2021) 197:111012. doi: 10.1016/j.envres.2021.111012
4. Dang, J, Shi, D, Li, X, Ma, N, Liu, Y, Zhong, P, et al. Artificial light-at-night exposure and overweight and obesity across GDP levels among Chinese children and adolescents. Nutrients. (2023) 15:939. doi: 10.3390/nu15040939
5. Yi, W, Wang, W, Xu, Z, Liu, L, Wei, N, Pan, R, et al. Association of outdoor artificial light at night with metabolic syndrome and the modifying effect of tree and grass cover. Ecotoxicol Environ Saf. (2023) 264:115452. doi: 10.1016/j.ecoenv.2023.115452
6. Paksarian, D, Rudolph, KE, Stapp, EK, Dunster, GP, He, J, Mennitt, D, et al. Association of Outdoor Artificial Light at night with mental disorders and sleep patterns among US adolescents. JAMA Psychiatry. (2020) 77:1266–75. doi: 10.1001/jamapsychiatry.2020.1935
7. Wang, L-B, Gong, Y-C, Fang, Q-L, Cui, X-X, Dharmage, SC, Jalaludin, B, et al. Association between exposure to outdoor artificial light at night and sleep disorders among children in China. JAMA Netw Open. (2022) 5:e2213247–7. doi: 10.1001/jamanetworkopen.2022.13247
8. Muscogiuri, G, Poggiogalle, E, Barrea, L, Tarsitano, MG, Garifalos, F, Liccardi, A, et al. Exposure to artificial light at night: a common link for obesity and cancer? Eur J Cancer. (2022) 173:263–75. doi: 10.1016/j.ejca.2022.06.007
9. Kim, M, Facco, FL, Braun, RI, Wolf, MS, Garcia-Canga, B, Grobman, WA, et al. The association between light exposure before bedtime in pregnancy and the risk of developing gestational diabetes mellitus. Am J Obstet Gynecol MFM. (2023) 5:100922. doi: 10.1016/j.ajogmf.2023.100922
10. Xu, Z, Jin, J, Yang, T, Wang, Y, Huang, J, Pan, X, et al. Outdoor light at night, genetic predisposition and type 2 diabetes mellitus: a prospective cohort study. Environ Res. (2023) 219:115157. doi: 10.1016/j.envres.2022.115157
11. Zheng, R, Xin, Z, Li, M, Wang, T, Xu, M, Lu, J, et al. Outdoor light at night in relation to glucose homoeostasis and diabetes in Chinese adults: a national and cross-sectional study of 98, 658 participants from 162 study sites. Diabetologia. (2022) 66:336–45. doi: 10.1007/s00125-022-05819-x
12. Kumar, P, Ashawat, MS, Pandit, V, and Sharma, DK. Artificial light pollution at night: a risk for Normal circadian rhythm and physiological functions in humans. Curr Environ Eng. (2019) 6:111–25. doi: 10.2174/2212717806666190619120211
13. Meléndez-Fernández, OH, Liu, JA, and Nelson, RJ. Circadian rhythms disrupted by light at night and mistimed food intake Alter hormonal rhythms and metabolism. Int J Mol Sci. (2023) 24:3392. doi: 10.3390/ijms24043392
14. Hu, K, Li, W, Zhang, Y, Chen, H, Bai, C, Yang, Z, et al. Association between outdoor artificial light at night and sleep duration among older adults in China: a cross-sectional study. Environ Res. (2022) 212:113343. doi: 10.1016/j.envres.2022.113343
15. Grunst, ML, and Grunst, AS. Endocrine effects of exposure to artificial light at night: a review and synthesis of knowledge gaps. Mol Cell Endocrinol. (2023) 568-569:111927. doi: 10.1016/j.mce.2023.111927
16. Pathirana, MM, Ali, A, Lassi, ZS, Arstall, MA, Roberts, CT, and Andraweera, PH. Protective influence of breastfeeding on cardiovascular risk factors in women with previous gestational diabetes mellitus and their children: a systematic review and Meta-analysis. J Hum Lact. (2021) 38:501–12. doi: 10.1177/08903344211034779
17. Gao, C, Sun, X, Lu, L, Liu, F, and Yuan, J. Prevalence of gestational diabetes mellitus in mainland China: a systematic review and meta-analysis. J Diabetes Investig. (2018) 10:154–62. doi: 10.1111/jdi.12854
18. Feng, Y, Jiang, C-D, Chang, A-M, Shi, Y, Gao, J, Zhu, L, et al. Interactions among insulin resistance, inflammation factors, obesity-related gene polymorphisms, environmental risk factors, and diet in the development of gestational diabetes mellitus. J Matern Fetal Neonatal Med. (2018) 32:339–47. doi: 10.1080/14767058.2018.1446207
19. Diao, D, Diao, F, Xiao, B, Liu, N, Zheng, D, Li, F, et al. Bayes conditional probability-based causation analysis between gestational diabetes mellitus (GDM) and pregnancy-induced hypertension (PIH): a statistic case study in Harbin, China. J Diabetes Res. (2022) 2022:1–7. doi: 10.1155/2022/2590415
20. Su, W-J, Chen, Y-L, Huang, P-Y, Shi, X-L, Yan, F-F, Chen, Z, et al. Effects of Prepregnancy body mass index, weight gain, and gestational diabetes mellitus on pregnancy outcomes: a population-based study in Xiamen, China, 2011–2018. Ann Nutr Metab. (2019) 75:31–8. doi: 10.1159/000501710
21. Diaz-Santana, MV, O’Brien, KM, Park, Y-MM, Sandler, DP, and Weinberg, CR. Persistence of risk for type 2 diabetes after gestational diabetes mellitus. Diabetes Care. (2022) 45:864–70. doi: 10.2337/dc21-1430
22. Di Bernardo, SC, Lava, SAG, Epure, AM, Younes, SE, Chiolero, A, Sekarski, N, et al. Consequences of gestational diabetes mellitus on neonatal cardiovascular health: MySweetHeart cohort study. Pediatr Res. (2022) 94:231–8. doi: 10.1038/s41390-022-02390-4
23. Li, Y, Wang, W, and Zhang, D. Maternal diabetes mellitus and risk of neonatal respiratory distress syndrome: a meta-analysis. Acta Diabetol. (2019) 56:729–40. doi: 10.1007/s00592-019-01327-4
24. Fuhler, GM . The immune system and microbiome in pregnancy. Best Pract Res Clin Gastroenterol. (2020) 44-45:101671. doi: 10.1016/j.bpg.2020.101671
25. Dzirbíková, Z, Stebelová, K, Kováčová, K, Okuliarová, M, Olexová, L, and Zeman, M. Artificial dim light at night during pregnancy can affect hormonal and metabolic rhythms in rat offspring. Int J Mol Sci. (2022) 23:14544. doi: 10.3390/ijms232314544
26. Zhang, L, Yin, W, Yu, W, Wang, P, Wang, H, Zhang, X, et al. Environmental exposure to outdoor artificial light at night during pregnancy and fetal size: a prospective cohort study. Sci Total Environ. (2023) 883:163521. doi: 10.1016/j.scitotenv.2023.163521
27. Zheng, Q, Weng, Q, and Wang, K. Developing a new cross-sensor calibration model for DMSP-OLS and Suomi-NPP VIIRS night-light imageries. ISPRS J Photogramm Remote Sens. (2019) 153:36–47. doi: 10.1016/j.isprsjprs.2019.04.019
28. Chen, Z, Yu, B, Yang, C, Zhou, Y, Yao, S, Qian, X, et al. An extended time series (2000–2018) of global NPP-VIIRS-like nighttime light data from a cross-sensor calibration. Earth Syst Sci Data. (2021) 13:889–906. doi: 10.5194/essd-13-889-2021
29. Huhn, EA, Göbl, CS, Fischer, T, Todesco Bernasconi, M, Kreft, M, Kunze, M, et al. Sensitivity, specificity, and diagnostic accuracy of WHO 2013 criteria for diagnosis of gestational diabetes mellitus in low risk early pregnancies: international, prospective, multicentre cohort study. BMJ Med. (2023) 2:e000330. doi: 10.1136/bmjmed-2022-000330
30. Wei, J, Li, Z, Lyapustin, A, Sun, L, Peng, Y, Xue, W, et al. Reconstructing 1-km-resolution high-quality PM2.5 data records from 2000 to 2018 in China: spatiotemporal variations and policy implications. Remote Sens Environ. (2021) 252:112136. doi: 10.1016/j.rse.2020.112136
31. Wei, J, Li, Z, Xue, W, Sun, L, Fan, T, Liu, L, et al. The China high PM10 dataset: generation, validation, and spatiotemporal variations from 2015 to 2019 across China. Environ Int. (2021) 146:106290. doi: 10.1016/j.envint.2020.106290
32. Liu, X-X, Ma, X-L, Huang, W-Z, Luo, Y-N, He, C-J, Zhong, X-M, et al. Green space and cardiovascular disease: a systematic review with meta-analysis. Environ Pollut. (2022) 301:118990. doi: 10.1016/j.envpol.2022.118990
33. Rhew, IC, Vander Stoep, A, Kearney, A, Smith, NL, and Dunbar, MD. Validation of the normalized difference vegetation index as a measure of neighborhood greenness. Ann Epidemiol. (2011) 21:946–52. doi: 10.1016/j.annepidem.2011.09.001
34. Sorensen, TB, Wilson, R, Gregson, J, Shankar, B, Dangour, AD, and Kinra, S. Is night-time light intensity associated with cardiovascular disease risk factors among adults in early-stage urbanisation in South India? A cross-sectional study of the Andhra Pradesh children and parents study. BMJ Open. (2020) 10:e036213. doi: 10.1136/bmjopen-2019-036213
35. Park, Y-MM, White, AJ, Jackson, CL, Weinberg, CR, and Sandler, DP. Association of Exposure to artificial light at night while sleeping with risk of obesity in women. JAMA Intern Med. (2019) 179:1061–71. doi: 10.1001/jamainternmed.2019.0571
36. Yu, Z, Hu, N, Du, Y, Wang, H, Pu, L, Zhang, X, et al. Association of outdoor artificial light at night with mental health among China adults: a prospective ecology study. Environ Sci Pollut Res. (2022) 29:82286–96. doi: 10.1007/s11356-022-21587-y
37. Kim, M, Vu, T-H, Maas, MB, Braun, RI, Wolf, MS, Roenneberg, T, et al. Light at night in older age is associated with obesity, diabetes, and hypertension. Sleep. (2023) 46:zsac 130. doi: 10.1093/sleep/zsac130
38. Liao, J, Yu, C, Cai, J, Tian, R, Li, X, Wang, H, et al. The association between artificial light at night and gestational diabetes mellitus: a prospective cohort study from China. Sci Total Environ. (2024) 919:170849. doi: 10.1016/j.scitotenv.2024.170849
39. Zhang, L, Wang, H, Zu, P, Li, X, Ma, S, Zhu, Y, et al. Association between exposure to outdoor artificial light at night during pregnancy and glucose homeostasis: a prospective cohort study. Environ Res. (2024) 247:118178. doi: 10.1016/j.envres.2024.118178
40. Reynolds, LP, Borowicz, PP, Caton, JS, Crouse, MS, Dahlen, CR, and Ward, AK. Developmental programming of fetal growth and development. Vet Clin N Am Food Anim Pract. (2019) 35:229–47. doi: 10.1016/j.cvfa.2019.02.006
41. Quaresima, P, Visconti, F, Chiefari, E, Mirabelli, M, Borelli, M, Caroleo, P, et al. Appropriate timing of gestational diabetes mellitus diagnosis in medium-and low-risk women: effectiveness of the Italian NHS recommendations in preventing fetal macrosomia. J Diabetes Res. (2020) 2020:1–8. doi: 10.1155/2020/5393952
42. Escobar, C, Rojas-Granados, A, and Angeles-Castellanos, M. Development of the circadian system and relevance of periodic signals for neonatal development. Handb Clin Neurol. (2021) 179:249–58. doi: 10.1016/B978-0-12-819975-6.00015-7
43. Lee, E, and Kim, M. Light and life at night as circadian rhythm disruptors. Chronobiol Med. (2019) 1:95–102. doi: 10.33069/cim.2019.0016
44. Zeman, M, Okuliarova, M, and Rumanova, VS. Disturbances of hormonal circadian rhythms by light pollution. Int J Mol Sci. (2023) 24:7255. doi: 10.3390/ijms24087255
45. Verteramo, R, Pierdomenico, M, Greco, P, and Milano, C. The role of melatonin in pregnancy and the health benefits for the newborn. Biomedicines. (2022) 10:3252. doi: 10.3390/biomedicines10123252
46. Zhang, L, Liu, Y, Li, M, Zhu, X, and Shi, Y. Effect of a high-calorie diet and constant light exposure on female reproduction, metabolism and immune inflammation: a comparative study of different mouse models. Am J Reprod Immunol. (2021) 86:e13479. doi: 10.1111/aji.13479
47. Ren, Z, Yuan, J, Luo, Y, Wang, J, and Li, Y. Association of air pollution and fine particulate matter (PM2.5) exposure with gestational diabetes: a systematic review and meta-analysis. Ann Transl Med. (2023) 11:23–3. doi: 10.21037/atm-22-6306
48. Qu, Y, Yang, B, Lin, S, Bloom, MS, Nie, Z, Ou, Y, et al. Associations of greenness with gestational diabetes mellitus: the Guangdong registry of congenital heart disease (GRCHD) study. Environ Pollut. (2020) 266:115127. doi: 10.1016/j.envpol.2020.115127
Keywords: gestational diabetes mellitus, outdoor artificial light, pregnancy, risk factors, air pollution
Citation: Sun Q, Ye F, Liu J, Yang Y, Hui Q, Chen Y, Liu D, Guo J, Wang C, Lv D, Tang L and Zhang Q (2024) Outdoor artificial light at night exposure and gestational diabetes mellitus: a case–control study. Front. Public Health. 12:1396198. doi: 10.3389/fpubh.2024.1396198
Edited by:
Alberto Modenese, University of Modena and Reggio Emilia, ItalyCopyright © 2024 Sun, Ye, Liu, Yang, Hui, Chen, Liu, Guo, Wang, Lv, Tang and Zhang. This is an open-access article distributed under the terms of the Creative Commons Attribution License (CC BY). The use, distribution or reproduction in other forums is permitted, provided the original author(s) and the copyright owner(s) are credited and that the original publication in this journal is cited, in accordance with accepted academic practice. No use, distribution or reproduction is permitted which does not comply with these terms.
*Correspondence: Qi Zhang, emhhbmdxaWtleWFuQDE2My5jb20=