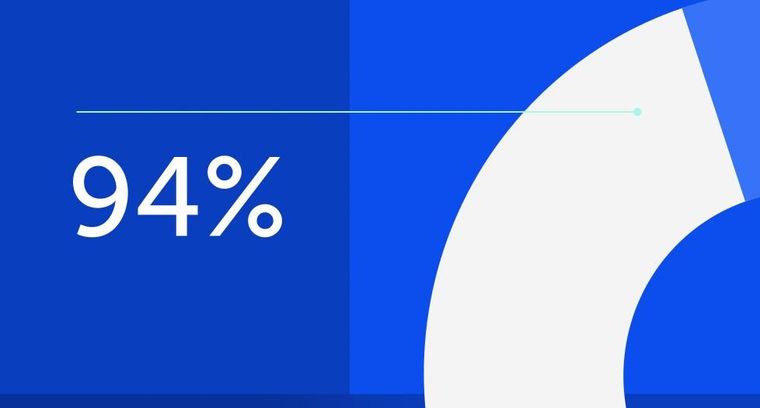
94% of researchers rate our articles as excellent or good
Learn more about the work of our research integrity team to safeguard the quality of each article we publish.
Find out more
ORIGINAL RESEARCH article
Front. Public Health, 24 July 2024
Sec. Life-Course Epidemiology and Social Inequalities in Health
Volume 12 - 2024 | https://doi.org/10.3389/fpubh.2024.1388093
Introduction: An educational mismatch is defined as the situation where the education qualifications of an employee do not match the qualifications required for the job they do. A mismatch can be vertical where the level of the employee's qualification is not the one required by the job. This study contributes to the literature on work-related social determinants of health, by carrying out the first assessment of the relationship between educational mismatch and health in Italy.
Methods: Data come from PLUS, a national survey of labor supply. The risk of suffering from bad or very bad health associated with educational mismatch is investigated through a logistic regression model accounting for the socioeconomic context and occupation.
Results: Our findings show women are at greater risk of suffering from bad or very bad health than men, especially if under-educated.
Discussion: Our results show the need to address more research on work-related social determinants of health, which can represent a barrier to achieving health equity.
An educational mismatch is defined as the situation where the education qualifications of an employee do not match the qualifications required for the job they do. A mismatch can be vertical where the level of the employee's qualification is not the one required by the job. Since Duncan and Hoffman's seminal work (1), educational mismatch has been extensively debated for the negative consequences that it may produce on labor markets. Originating from characteristics of both the supply and demand of labor (2), it affects wages (3–7), productivity (8), career mobility (9–11), and job satisfaction (12, 13). Educational mismatch is also viewed as an important public health concern. Several studies associate it with negative health outcomes, especially mental health conditions (14–21). This association pertains to what is called “status inconsistency” (22), i.e., “a discrepancy between the position a person holds in one domain of their social environment comparative to their position in another domain” (15). The remaining, scarce literature focuses on mortality, injuries, and self-assessed health (23–27).
Most existing empirical studies on educational mismatch concentrate on advanced economy countries, where this phenomenon is most evident, and investigate its effects both on the labor market and health outcomes. To our knowledge, studies regarding Italy are focused only on the labor market (10, 28), which suffers from long-term disequilibrium across regions, marked by the country's historic and persistent North-South developmental gap (29–32). The interactions between macroeconomic events and institutional configurations (33) result in high structural unemployment with age, gender, and sectoral disparity, further worsened by the Great Recession. In addition, Italy exhibits three other peculiar traits: the highest percentage of young people not in employment, education, or training (NEETs), the lowest attendance in tertiary education and the second lowest women's employment rate in Europe (34). However, the Italian situation bears particular interest with regard to the possible relationship between educational mismatch and health: high percentages of educational mismatch in the Italian labor market (35) coexist with long, and healthy life expectancy, which is one of the highest in the world, a low fertility rate and aging workers with increasing number of years lived with disability (36). Studies of educational mismatch and health have focused mainly on the over-educated for which investments in education do not match their occupational position (37, 38), clearly showing the “status inconsistency.” Educational mismatch comprise over-qualification, under-employment, and occupational downgrading (27) and can cause experience of relative deprivation and ultimately bad health (14, 16, 26, 27, 39). Similarly, the under-educated may be more likely to experience relative deprivation and associated stress or poor health effects when they compare themselves to others with similar levels of attained education but highest income or occupational status (27). This study aims at contributing to the literature on work-related social determinants of health, by carrying out the first ever assessment of the relationship between vertical educational mismatch and health for the over- and under-educated workers in Italy.
Data come from the Participation Labor Unemployment Survey (PLUS) (40) by the National Institute for Public Policy Analysis (INAPP). PLUS is an Italian national survey periodically conducted since 2005. It is included in the National Statistical Plan and is part of the official statistics network (SISTAN). The 2021 edition is based on a sample of 46,262 participants aged 18–74 years old and has been conducted between March and July 2021. The participation rate in the 2021 edition was 56.3%. PLUS adopted a stratified quota sampling with partially overlapping planned domains. Stratification was implemented considering the following conditions: region of residence, type of municipality, sex, age, and employment status. The questionnaire counts a total of about 200 questions. Individuals were interviewed by telephone through a Computer-Assisted Telephone Interviewing (CATI) technique and in the absence of proxy respondents, i.e., the answers were given directly and exclusively by the interviewee, thereby reducing measurement errors and partial non-responses. PLUS has a longitudinal structure, providing details about some social and economic indicators such as work and family income, education, and health and it is aimed mainly at investigating problematic aspects of the labor market through modules for each contractual typology and sub-modules dedicated to specific topics, allowing to read phenomena at different levels.
One of the specific modules available in PLUS concerns educational mismatch measured by worker's self-assessment, one of three methods generally used for quantifying educational mismatch together with job analysis and realized matched (41). The subjective definition of educational mismatch results from the “What level of education do you think is most appropriate for your job position?” question. Based on the worker's response, a vertical educational mismatch is noted by comparing the worker's actual education to that which they believe (self-reported) to be the appropriate education requirements for their job position (5). The term “over-educated” is used to describe individuals who have attained a level of education that is higher than that which they believe to be appropriate for their job role. Conversely, the term “under-educated” is used to describe individuals who have attained a level of education that is lower than that which they believe to be appropriate for their job role.
Additionally, PLUS dedicates a specific module on perceived health. The question is “How do you judge your overall health?” and the respondent has five possible answers corresponding to: very good, good, fair, bad, and very bad perceived health.
Occupation, coded according to ISCO-88 classification, is also investigated in PLUS and hence considered in the analysis. Finally, PLUS allows to detect the socioeconomic context, through the following variables: sex, age, marital status, having children, geographical location, and urbanization. As well-known, sex is one of the discriminating factors influencing the opportunities to obtain a job (42, 43). In Italy, despite the fact that women are often more educated than men they experience a higher percentage of unemployment, more frequent part-time jobs, and lower wage growth than males (44, 45). Women's participation depends on their partners' labor position, institutional context, welfare measures and relative position in the labor market (46, 47). Although, women live longer than men, their health perception is generally worse than men (48). Age is another key factor. Italy is experiencing two simultaneous phenomena: an aging workforce and a low rate of youth employment, one of the lowest in Europe (34). Typically, youth have better health than adults; however, given that youth employment is more sensitive to business cycles (49), a sense of frustration may arise, negatively affecting the perception of health. The more children's women have, the greater the gap in female and male employment rates in Italy (44). Furthermore, although some studies show that having children protects female health (50, 51), care for children may result in increasing stress both for men and women, and often lead them to neglect their health problems. Being married is recognized as a protective factor for health. It positively affects mental health (52), mortality (53, 54), and healthy behaviors (55, 56). Geographical location highlights Italy's historical North-South dualism regarding education, employment, and health. Urbanization is considered as well, since one third of the Italian territory is mountainous. Mountain municipalities, with a few thousand inhabitants, are almost half of the total of Italian municipalities (44). They suffer from marginality (57), shortage of local social and health services, and reduced hospital facilities (58).
The PLUS survey is representative of the Italian working-age population. Nineteen thousand and twenty-five subjects who declared to be occupied were included in the study. Data were analyzed applying weights to adjust the results for the sample design and to provide frequency distributions representing the target population (e.g., employed population). Such weights were calculated adopting methodologies based on generalized regression estimators (59). Descriptive statistics were used to analyse the distribution of age, sex, and area of residence of workers by education; age, sex, area of residence and education by perceived health status; age, sex, area of residence, education, urbanization level, marital status, and childbearing by type of mismatch. The crude and age standardized percentages of workers suffering from bad health by occupation, educational mismatch, and sex were also calculated. Data were analyzed according to a cross-sectional design.
The risk of suffering from bad or very bad health (the outcome variable) associated with educational mismatch (exposure variable) was investigated through a logistic regression model accounting for the socioeconomic context, considering sex (males/females), age (18–29/30–49/50–74 years), marital status (single/married/separated or divorced/widowed), having children (yes/no), geographical location (North/Center/South), urbanization (up to 5,000 residents/from 5,000 to 10,000 residents/from 10,000 to 30,000 residents/from 30,000 to 100,000 residents/from 100,000 to 250,000 residents/more than 250,000 residents), and nine macro-categories of occupation (administrative workers/intellectual scientific and highly skilled professionals/professionals/clericals and related workers/service workers/craft workers/production and related workers/unskilled workers/armed forces) as potential confounding factors. In the logistic models standardized weights, to sum to the sample size, were used to avoid spurious significance due to an “inflated” sample size. In order to analyse the role of EM in the relationship between sex and perceived health an interaction term was included in the regression models. Analyses were performed with “Stata 17” (60). All odds ratios (ORs) are given with 95% confidence intervals (CIs).
Almost 50% of all the respondents have a secondary level of education (high school) while about 30% have a degree (University degree). Women are better educated than men. Respondents with higher education are more frequent in the South of Italy and in the age class 30–49 years (Supplementary Table 1).
Regarding health, self-assessed health is high-grade overall. Only 6.3% of men and 9.4% of women perceived bad or very bad health. If only slightly, women feel worse than men, as we expected. There are no major differences between education levels, except for those who have primary schooling and report more feelings of bad or very bad health. There are no substantial dissimilarities between areas of the country, except for those residents of the South who report feeling bad health (Supplementary Table 2).
More than half of the sample respondents, corresponding to 69.6%, considered their level of education appropriate for their jobs, while 18.5% think they were over-educated and 11.9% under-educated (Supplementary Figure 1).
Men suffer from educational mismatch more than women and under-educated men are more than over-educated. Observing age, the majority of the over-educated, are aged 30–49 while the age range 50–75 prevails among the under-educated. Both over- and under-educated, 49.1 and 57.6% in that order, are prevalent in the North and live in a medium dimension of urbanization. Finally, the over-educated are single and without children, while the under-educated are married with children (Supplementary Table 3).
Relative to categories of occupation, the highest percentage of over-educated men is noted in “Industrial plant operators” and “Unskilled professions in manufacturing, mineral extraction and construction while over-educated women in “Farmers and skilled laborers in agriculture, forestry, animal husbandry, fishing, and hunting.” For under-educated men the highest percentage is in “Unskilled professions in agriculture, grounds maintenance, animal husbandry, forestry, and fishing” while under-educated women in “Semi-skilled workers of fixed machinery for mass production and assembly workers” (for data on other professions, see Supplementary Table 4). It is crucial to acknowledge that the holding a specific profession and experiencing an educational mismatch is influenced by the fact that the latter is self-reported and, most importantly, reflects expectations about one's life that may not be entirely realistic. We delve deeper into this in the Limitation section.
The under- and over-educated in Italy perceive a good health status overall. Only 7.6% of respondents say they suffer from bad or very bad health and the over-educated suffer a little less than the under-educated (Supplementary Figure 2). Among the 9-macro occupational macro-categories the perception of poor health (bad and very bad health) for over-educated men, is more frequent, compared to workers with adequate education, in “Clerical and Related Workers” and “Service Workers and Shop and Market Sales Workers” categories, even though not in a statistically significant way. While for under-educated is in “Production and Related Workers, Transport Equipment Operators and Laborers” and “Unskilled workers.” Regarding women, a gender disadvantage is confirmed: under-educated women suffer more than men in almost all occupational categories observed, significantly among “Administrative. Executive and Managerial Workers” and “Service workers” (Figures 1A, B).
Figure 1. (A) Prevalence of bad and very bad health perception by type of educational mismatch for macro professional categories: adjusted percentage by age with 95% Confidence Intervals (C.I.)—males. (B) Prevalence of bad and very bad health perception by type of educational mismatch for macro professional categories: adjusted percentage by age percentages with 95% Confidence Intervals (C.I.)—females.
A detailed analysis of the 38 occupations across the 9 categories reveals that overeducated men who report perceived poor health are employed in in “Secretarial and office machine clerks,” “Skilled professions in commercial activities” and “Unskilled occupations in domestic, recreational and cultural activities” while the under-educated in “Technical professions in public and personal services,” “Secretarial and office machine clerks” and “Unskilled occupations in trade and services.” On the other hand, the occupations in which women report more likely poor health if they are over-educated are “Engineers, architects and assimilated professions,” “Specialists in the life sciences” and “Education and research specialists” while those under-educated in “Secretarial and office machine clerks,” “Skilled professions in cultural, security and personal services” and “Unskilled occupations in domestic, recreational and cultural activities” (for details regarding perceived and self-reported ill health in all 38 occupations see Supplementary Tables 5, 6).
The analysis conducted through the logistic regression model showed different patterns for the under- and over-educated workers, with significantly higher risks for under-educated (OR = 1.41, 95% C.I. = 1.17–1.70) compared to workers with adequate education, and no significant difference for over-educated (OR = 0.89, 95% C.I. = 0.72–1.11). The probability of suffering from bad or very bad health was significantly higher for women (OR = 1.57, 95% C.I. = 1.38–1.79), adults and older, separated/divorced or widowed, and those residing in the South. The interaction between sex and EM revealed an increased risk among female workers, both under-educated and overeducated, even though not statistically significant. Among other adjusting factors, the level of urbanization showed an increased risk only for cities from 100,000 and 250,000 residents, as well as professions like “Service workers” (OR = 1.95, 95% C.I. = 1.33–2.87), “Production workers” (2.49, 95% C.I. = 1.56–3.97), and “Unskilled workers” (2.63; 95% C.I. = 1.77–3.91; Table 1).
Table 1. Risk of suffering, in terms of odds ratios (OR), from bad and very bad health associated with the educational mismatch (EM) for over-and under-education, adjusted for potential confounders with 95% confidence intervals (C.I.).
There are about 7 million Italian workers who perceive a mismatch between the educational qualification they attained and the one appropriate for their job. Of these, nearly 3 million are women, the majority of them are over-educated. The probability of suffering from bad health is significantly higher among under-educated workers confirming the well-known relationship between education and health (61).
Concerning the role of gender in the relationship between educational mismatch and health, this study showed an interaction effect, even though not statistical significant, between EM and gender, with a clear disadvantage for female workers, and a higher percentage of over-educated female workers in some professions, such as “Specialists in mathematical, computer, chemical, physical and natural sciences” “Engineers, architects, and assimilated professions” and “Specialists in life sciences,” suffering from poor health. These findings suggest the possibility of a higher susceptibility of female workers with EM to perceiving bad health. Even though the estimated risks did not reach the statistical significance, the pattern is quite evident: the risk of suffering from bad health, in terms of OR, of under-educated women is 2.43 (calculated as the combination of the two conditions—being a female and under educated worker—and the estimated interaction term, estimated using as the reference category that of men with no educational mismatch) and, similarly, the risk for over-educated is 1.73. Our results are entirely consistent with findings in the literature, particularly those that have highlighted inefficiencies and inequalities in labor market functioning in certain countries (26). They also are consistent with Italian labor market data on the gender disadvantage of women relative to men in the labor market (44), especially if they are separated/divorced or widowed.
Our results are also consistent with evidence in this regard concerning age (36). The risk of perceived bad or very bad health status increases if a woman is an adult or older adult. What is more, we confirm what is already known with respect to the Italian historic and persistent North-South developmental gap for health, education, and work (62–64) and for which Italian women residing in the South have a higher risk of perceiving bad or very bad health. The mechanisms through which educational mismatch can have repercussions on health can be multiple and trace back to the effects such a mismatch has on mental health, but this may also depend on the family, social and general context (20). Indeed, in the South, more than elsewhere, achieving effective universal health coverage with equity is compounded by social and economic deprivation, inefficient public services, environmental damage, unemployment and even crime and it is well-known how heavily socioeconomic context affects health (65).
Another related factor affecting workers' health is occupation. “Unskilled workers” have a higher risk of suffering from poor health than the other categories, as well as “Service Workers and Shop and Market Sales Workers” and “Production workers.” A gender health disadvantage is also confirmed in this: under-educated women suffer more than men in almost all occupational categories observed.
In contrast, no association was found for the other socioeconomic variables included in the analysis. In Italy, having children is not associated with bad or very bad health neither living in a city, with the sole exception of living in larger cities.
The first strength is that this study is on an issue never previously investigated for Italy. It makes an important contribution by providing new insights into work-related determinants of health, especially for women at greater risk of suffering from bad or very bad health than men. The second strength deals with the sample size that allows to analyze the occupational groups in details: the absolute number of several sampled workers by occupational groups is never <30 that constitutes a valid sample size.
Concerning the limitations, the first relies on self-assessed measures. They have often been criticized for their biases and misinterpretation due to various factors, including individual personality traits, which can lead to perceived mismatch and poor health (7, 46, 66, 67). The second limitation rests on the cross-sectional analysis, which, by nature, does not allow one to verify a causal relationship between educational mismatch and health. Finally, a possible selection bias could be happened because of a limited participation rate (56.3%), which is, on the other hand, in line with the participation rate of other similar national large survey (68).
This study has examined the relationship between educational mismatch and health in Italy. Both descriptive and empirical analysis, based on cross-sectional data from PLUS 2021 (40), seem to indicate a possible association between vertical educational mismatch and bad or vary bad health, especially for Italian under-educated women. Our study provides reliable hints on the health burden estimation of workers with EM suffering from bad health. Even though the study did not provide conclusive results, the findings constitute an important contribution by providing insights into an issue never investigated for Italy and emphasize the need to address more research on work-related social determinants of health which can represent a barrier to achieving health equity. Future developments may involve using the panel aspect of PLUS and investigating the relationship between skill mismatch and health considering several potential confounding factors.
Original datasets are available in a publicly accessible repository: INAPP, PLUS dataset: https://oa.inapp.org/xmlui/handle/20.500.12916/951.
CA: Conceptualization, Formal analysis, Funding acquisition, Investigation, Methodology, Project administration, Resources, Supervision, Validation, Visualization, Writing – original draft, Writing – review & editing. AR: Conceptualization, Data curation, Formal analysis, Investigation, Methodology, Software, Supervision, Validation, Visualization, Writing – original draft, Writing – review & editing.
The author(s) declare financial support was received for the research, authorship, and/or publication of this article. We acknowledge co-funding from Next Generation EU, in the context of the National Recovery and Resilience Plan, Investment PE8—Project Age-It: Aging Well in an Aging Society. This resource was co-financed by the Next Generation EU [DM 1557 11.10.2022].
The authors declare that the research was conducted in the absence of any commercial or financial relationships that could be construed as a potential conflict of interest.
The author(s) declared that they were an editorial board member of Frontiers, at the time of submission. This had no impact on the peer review process and the final decision.
All claims expressed in this article are solely those of the authors and do not necessarily represent those of their affiliated organizations, or those of the publisher, the editors and the reviewers. Any product that may be evaluated in this article, or claim that may be made by its manufacturer, is not guaranteed or endorsed by the publisher.
The views and opinions expressed are only those of the authors and do not necessarily reflect those of the European Union or the European Commission. Neither the European Union nor the European Commission can be held responsible for them.
The Supplementary Material for this article can be found online at: https://www.frontiersin.org/articles/10.3389/fpubh.2024.1388093/full#supplementary-material
1. Duncan GJ, Hoffman SD. The incidence and wage effects of over education. Econ Educ Rev. (1981) 1:75–86. doi: 10.1016/0272-7757(81)90028-5
2. Ghignoni E, Verashchagina A. Educational qualifications mismatch in Europe. Is it demand or supply driven? J Comp Econ. (2014) 42:670–92. doi: 10.1016/j.jce.2013.06.006
3. Bauer TK. Educational mismatch and wages: a panel analysis. Econ Educ Rev. (2002) 21:221–9. doi: 10.1016/S0272-7757(01)00004-8
4. Iriondo I, Pérez-Amaral T. The effect of educational mismatch on wages in Europe. J Policy Model. (2016) 38:304–23. doi: 10.1016/j.jpolmod.2015.12.008
5. Mahuteau S, Mavromaras K, Sloane P, Wei Z. Horizontal and vertical educational mismatch and wages. In: Paper Presented at 25th Australian Labour Market Research. Freemantle, Western Australia (2014).
6. Lu Y, Li X. Vertical education-occupation mismatch and wage inequality by race/ethnicity and nativity among highly educated US workers. Soc Forces. (2021) 100:706–37. doi: 10.1093/sf/soaa145
7. Sellami S, Verhaest D, Nonneman W, Van Trier W. The impact of educational mismatches on wages: the influence of measurement error and unobserved heterogeneity. BE J Econ Anal Policy. (2017) 17:20160055. doi: 10.1515/bejeap-2016-0055
8. Vermeylen G, Mahy B, Rycx F. Educational mismatch and productivity: a review. Dyn Reg. (2014) 1:17. doi: 10.3917/dyre.001.0017
9. Büchel F, Mertens A. Overeducation, undereducation, and the theory of career mobility. Appl Econ. (2004) 36:803–16. doi: 10.1080/0003684042000229532
10. Esposito P, Scicchitano S. Educational mismatch and labour market transitions in Italy: Is there an unemployment trap? Struct Change Econ Dyn. (2022) 61:138–55. doi: 10.1016/j.strueco.2022.02.011
11. Grunau P, Pecoraro M. Educational mismatch and promotions to managerial positions: a test of the career mobility theory. Appl Econ. (2017) 49:1226–40. doi: 10.1080/00036846.2016.1213369
13. Wang H, Abbas KM, Abbasifard M, Abbasi-Kangevari M, Abbastabar H, Abd-Allah F, et al. Global age-sex-specific fertility, mortality, healthy life expectancy (HALE), and population estimates in 204 countries and territories, 1950-2019: a comprehensive demographic analysis for the Global Burden of Disease Study 2019. Lancet. (2020) 396:1160–203. doi: 10.1016/S0140-6736(20)30977-6
14. Bracke P, Pattyn E, Von Dem Knesebeck O. Overeducation and depressive symptoms: diminishing mental health returns to education. Sociol Health Illn. (2013) 35:1242–59. doi: 10.1111/1467-9566.12039
15. Milner A, Aitken Z, Kavanagh A, LaMontagne AD, Petrie D. Status inconsistency and mental health: a random effects and instrumental variables analysis using 14 annual waves of cohort data. Soc Sci Med. (2017) 189:129–37. doi: 10.1016/j.socscimed.2017.08.001
16. Mossakowski KN. Unfulfilled expectations and symptoms of depression among young adults. Soc Sci Med. (2011) 73:729–36. doi: 10.1016/j.socscimed.2011.06.021
17. Zhu R, Chen L. Overeducation, overskilling and mental well-being. BE J Econ Anal Policy. (2016) 16:20150187. doi: 10.1515/bejeap-2015-0187
18. Lundberg O, Manderbacka K. Assessing reliability of a measure of self-rated health. Scand J Soc Med. (1996) 24:218–24. doi: 10.1177/140349489602400314
19. Artés J, Salinas-Jiménez MDM, Salinas-Jiménez J. Small fish in a big pond or big fish in a small pond? The effects of educational mismatch on subjective wellbeing. Soc Indic Res. (2014) 119:771–89. doi: 10.1007/s11205-013-0526-1
20. Friedland DS, Price RH. Underemployment: consequences for the health and well-being of workers. Am J Community Psychol. (2003) 32:33–45. doi: 10.1023/A:1025638705649
21. Frank K, Hou F. Over-education and well-being: how does education-occupation mismatch affect the life satisfaction of university-educated immigrant and non-immigrant workers? Ethn Health. (2018) 23:884–901. doi: 10.1080/13557858.2017.1316832
22. Vernon SW, Buffler PA. The status of status inconsistency. Epidemiol Rev. (1988) 10:65–86. doi: 10.1093/oxfordjournals.epirev.a036029
23. Garcy AM. Educational mismatch and mortality among native-born workers in Sweden. A 19-year longitudinal study of 2.5 million over-educated, matched and under-educated individuals, 1990-2008. Sociol Health Illn. (2015) 37:1314–36. doi: 10.1111/1467-9566.12312
24. Premji S, Smith PM. Education-to-job mismatch and the risk of work injury. Inj Prev. (2013) 19:106–11. doi: 10.1136/injuryprev-2011-040314
25. Smith P, Frank J. When aspirations and achievements don't meet. A longitudinal examination of the differential effect of education and occupational attainment on declines in self-rated health among Canadian labour force participants. Int J Epidemiol. (2005) 34:827–34. doi: 10.1093/ije/dyi047
26. Vasiakina M, Robone S. Education-job mismatch as a determinant of health: evidence from the Russian Federation. Riv Ital Econ Demogr E Stat—Ital J Econ Demogr Stat Stud. (2018) 72:101–11.
27. Dunlavy AC, Garcy AM, Rostila M. Educational mismatch and health status among foreign-born workers in Sweden. Soc Sci Med. (2016) 154:36–44. doi: 10.1016/j.socscimed.2016.02.018
28. Franzini M, Raitano M. Few and underutilized? Overeducation of Italian graduates. Labour Econ. (2012) 2012:17–52.
29. Eckaus RS. The north-south differential in Italian economic development. J Econ Hist. (1961) 21:285–317. doi: 10.1017/S002205070010292X
30. Iuzzolino G, Pellegrini G, Viesti G. Convergence among Italian Regions, 1861–2011. [Quaderni di storia economica (Economic History Working Papers)]. Bank of Italy, Economic Research and International Relations Area (2011). Available online at: https://ideas.repec.org/p/bdi/workqs/qse_22.html (accessed December 2, 2023).
31. Lagravinese R. Economic crisis and rising gaps North-South: evidence from the Italian regions. Camb J Reg Econ Soc. (2015) 8:331–42. doi: 10.1093/cjres/rsv006
32. Asso PF. New perspectives on old inequalities: Italy's north-south divide. Territ Polit Gov. (2021) 9:346–64. doi: 10.1080/21622671.2020.1805354
33. Bertola G, Garibaldi P. The structure and history of Italian unemployment. SSRN Electron J. (2003) 2003:395420. doi: 10.2139/ssrn.395420
34. Eurostat. Ageing Europe—Statistics on Population Developments. Eurostat (2024). Available online at: https://ec.europa.eu/eurostat/statistics-explained/index.php?title=Ageing_Europe_-_statistics_on_population_developments (accessed January 3, 2024).
35. Sgobbi F, Cainarca GC. Educational mismatch e skill mismatch: un'indagine empirica sui lavoratori italiani. Quad Econ Lav. (2005) 2005:57–82.
36. James SL, Abate D, Abate KH, Abay SM, Abbafati C, Abbasi N, et al. Global, regional, and national incidence, prevalence, and years lived with disability for 354 diseases and injuries for 195 countries and territories, 1990-2017: a systematic analysis for the Global Burden of Disease Study 2017. Lancet. (2018) 392:1789–858. doi: 10.1016/S0140-6736(18)32279-7
37. Korpi T, Tåhlin M. Educational mismatch, wages, and wage growth: overeducation in Sweden, 1974-2000. Labour Econ. (2009) 16:183–93. doi: 10.1016/j.labeco.2008.08.004
38. Verdugo RR, Verdugo NT. The impact of surplus schooling on earnings: some additional findings. J Hum Resour. (1989) 24:629–43. doi: 10.2307/145998
39. Åberg Yngwe M, Kondo N, Hägg S, Kawachi I. Relative deprivation and mortality—a longitudinal study in a Swedish population of 4,7 million, 1990-2006. BMC Publ Health. (2012) 12:664. doi: 10.1186/1471-2458-12-664
40. INAPP. PLUS, Participation, Labor, Unemployment, Survey. (2023). Available online at: https://inapp.org/it/dati/plus (accessed February 4, 2023).
41. Hartog J. Over-education and earnings: where are we, where should we go? Econ Educ Rev. (2000) 19:131–47. doi: 10.1016/S0272-7757(99)00050-3
42. Cuberes D, Teignier M. Aggregate effects of gender gaps in the labor market: a quantitative estimate. J Hum Cap. (2016) 10:1–32. doi: 10.1086/683847
43. Philippis MD. The Dynamics of the Italian Labour Force Participation Rate: Determinants and Implications for the Employment and Unemployment Rate. [Questioni di Economia e Finanza (Occasional Papers)]. Bank of Italy, Economic Research and International Relations Area (2017). Available online at: https://ideas.repec.org/p/bdi/opques/qef_396_17.html (accessed November 1, 2023).
44. Istat. Percorso lavorativo. (2019). Available online at: https://www.istat.it/donne-uomini/bloc-2b.html (accessed February 28, 2023).
45. Del Bono E, Vuri D. Job mobility and the gender wage gap in Italy. Labour Econ. (2011) 18:130–42. doi: 10.1016/j.labeco.2010.06.002
46. Muñoz De Bustillo Llorente R, Sarkar S, Sebastian R, Antón J-I. Educational mismatch in Europe at the turn of the century: measurement, intensity and evolution. Int J Manpow. (2018) 39:977–95. doi: 10.1108/IJM-10-2018-0327
47. Baussola M, Mussida C. Disadvantaged workers in the Italian labour market: gender and regional gaps. In:Malo MÁ, Sciulli D, , editors. Disadvantaged Workers. AIEL Series in Labour Economics. Cham: Springer International Publishing (2014). p. 231–56.
48. National Institute of Statistics (ISTAT). L'eccesso di mortalità in: Rapporto annuale 2020 - Lo shock da pandemia: impatto demografico e conseguenze sanitarie. Pisa: Istat (2021).
49. Caroleo F, Pastore F. The Youth Experience Gap: Explaining Differences across EU Countries. [Quaderni del Dipartimento di Economia, Finanza e Statistica]. Università di Perugia, Dipartimento Economia (2007). Available online at: https://EconPapers.repec.org/RePEc:pia:wpaper:41/2007 (accessed January 15, 2024).
50. Walker LO, Walker ML, Walker ME. Health and well-being of childbearing women in rural and urban contexts. J Rural Health. (1994) 10:168–72. doi: 10.1111/j.1748-0361.1994.tb00226.x
51. Beral V. Long term effects of childbearing on health. J Epidemiol Community Health. (1985) 39:343–6. doi: 10.1136/jech.39.4.343
52. William, K, Frech, A, Carlson, DL. Marital status and mental health. In:A A Handbook for the Study of Mental Health: Social Contexts Theories and Systems 2nd, ed. Brown TN, Scheid TL, editors. New York, NY: Cambridge University Press (2010). p. 306–20.
53. Zueras P, Rutigliano R, Trias-Llimós S. Marital status, living arrangements, and mortality in middle and older age in Europe. Int J Publ Health. (2020) 65:627–36. doi: 10.1007/s00038-020-01371-w
54. Roth AR, Peng S. Non-spousal support, marital status, and mortality risk. J Aging Health. (2022) 34:41–50. doi: 10.1177/08982643211025381
55. Umberson D. Gender, marital status and the social control of health behavior. Soc Sci Med. (1992) 34:907–17. doi: 10.1016/0277-9536(92)90259-S
56. Kim A, Lee JA, Park HS. Health behaviors and illness according to marital status in middle-aged Koreans. J Public Health. (2018) 40:e99–e106. doi: 10.1093/pubmed/fdx071
57. Vendemmia B, Pucci P, Beria P. An institutional periphery in discussion. Rethinking the inner areas in Italy. Appl Geogr. (2021) 135:102537. doi: 10.1016/j.apgeog.2021.102537
58. Giulio de Belvis A, Meregaglia M, Morsella A, Adduci A, Perilli A, Cascini F, et al. Italy: health system review. Health Syst Transit. (2022) 24:1–236.
59. Giammatteo M. L'indagine campionaria Isfol-PLUS: contenuti metodologici e implementazione. Conenaghen: Studi Isfol (2009).
61. Cutler DM, Lleras-Muney A. Education and Health: Insights from International Comparisons. [NBER Working Papers]. National Bureau of Economic Research, Inc (2012). Available online at: https://ideas.repec.org/p/nbr/nberwo/17738.html (accessed February 6, 2024).
62. Toth F. How health care regionalisation in Italy is widening the North-South gap. Health Econ Policy Law. (2014) 9:231–49. doi: 10.1017/S1744133114000012
63. Abramo G, D'Angelo CA, Rosati F. The north-south divide in the Italian higher education system. Scientometrics. (2016) 109:2093–117. doi: 10.1007/s11192-016-2141-9
64. Basile R, Mantuano M, Girardi A, Russo G. Interregional migration of human capital and unemployment dynamics: evidence from Italian Provinces. Ger Econ Rev. (2019) 20:e385–414. doi: 10.1111/geer.12172
65. Stringhini S, Carmeli C, Jokela M, Avendaño M, Muennig P, Guida F, et al. Socioeconomic status and the 25 × 25 risk factors as determinants of premature mortality: a multicohort study and meta-analysis of 1.7 million men and women. Lancet. (2017) 389:1229–37. doi: 10.1016/S0140-6736(16)32380-7
66. Lundberg J, Kristenson M, Starrin B. Status incongruence revisited: associations with shame and mental wellbeing. Sociol Health Illn. (2009) 31:478–93. doi: 10.1111/j.1467-9566.2008.01148.x
67. Wu S, Wang R, Zhao Y, Ma X, Wu M, Yan X, et al. The relationship between self-rated health and objective health status: a population-based study. BMC Publ Health. (2013) 13:320. doi: 10.1186/1471-2458-13-320
Keywords: educational mismatch, health equity, gender gap, work related socioeconomic determinant, occupation
Citation: Abbafati C and Rosano A (2024) Work-related socioeconomic determinants of health: evidence from educational mismatch in Italy. Front. Public Health 12:1388093. doi: 10.3389/fpubh.2024.1388093
Received: 19 February 2024; Accepted: 08 July 2024;
Published: 24 July 2024.
Edited by:
Angelo d'Errico, Azienda Sanitaria Locale TO3, ItalyReviewed by:
Guoxi Jin, The First Affiliated Hospital of Bengbu Medical College, ChinaCopyright © 2024 Abbafati and Rosano. This is an open-access article distributed under the terms of the Creative Commons Attribution License (CC BY). The use, distribution or reproduction in other forums is permitted, provided the original author(s) and the copyright owner(s) are credited and that the original publication in this journal is cited, in accordance with accepted academic practice. No use, distribution or reproduction is permitted which does not comply with these terms.
*Correspondence: Cristiana Abbafati, Y3Jpc3RpYW5hLmFiYmFmYXRpQHVuaXJvbWExLml0
Disclaimer: All claims expressed in this article are solely those of the authors and do not necessarily represent those of their affiliated organizations, or those of the publisher, the editors and the reviewers. Any product that may be evaluated in this article or claim that may be made by its manufacturer is not guaranteed or endorsed by the publisher.
Research integrity at Frontiers
Learn more about the work of our research integrity team to safeguard the quality of each article we publish.