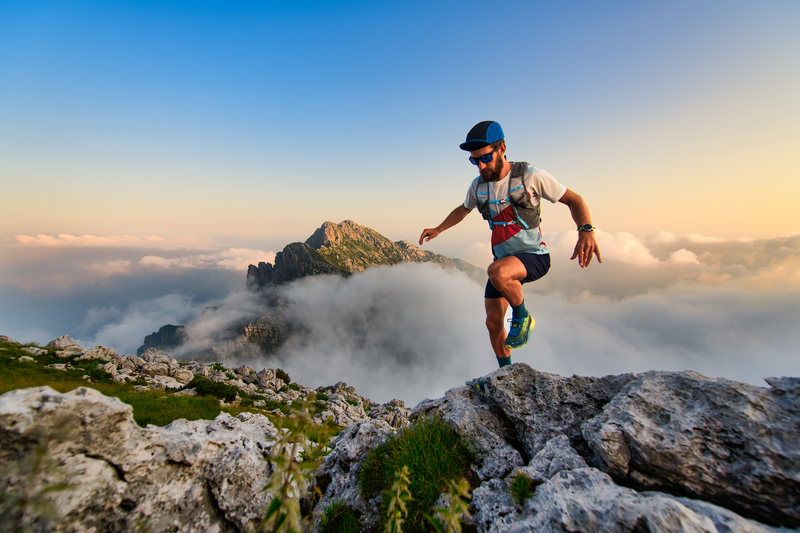
95% of researchers rate our articles as excellent or good
Learn more about the work of our research integrity team to safeguard the quality of each article we publish.
Find out more
ORIGINAL RESEARCH article
Front. Public Health , 20 June 2024
Sec. Substance Use Disorders and Behavioral Addictions
Volume 12 - 2024 | https://doi.org/10.3389/fpubh.2024.1386500
This article is part of the Research Topic Excessive Internet Use and its Impact on Mental Health View all 21 articles
Background: The aim of this study was to classify distinct subgroups of adolescents based on the severity levels of their mobile phone addiction and to investigate how these groups differed in terms of their psychosocial characteristics. We surveyed a total of 2,230 adolescents using three different questionnaires to assess the severity of their mobile phone addiction, stress, anxiety, depression, psychological resilience, and personality. Latent class analysis was employed to identify the subgroups, and we utilized Receiver Operating Characteristic (ROC) curves and multinomial logistic regression for statistical analysis. All data analyses were conducted using SPSS 26.0 and Mplus 8.5.
Methods: We classified the subjects into subgroups based on their mobile phone addiction severity, and the results revealed a clear pattern with a three-class model based on the likelihood level of mobile phone addiction (p < 0.05). We examined common trends in psychosocial traits such as age, grade at school, parental education level, anxiety levels, and resilience. ROC analysis of sensitivity versus 1-specificity for various mobile phone addiction index (MPAI) scores yielded an area under the curve (AUC) of 0.893 (95% CI, 0.879 to 0.905, p < 0.001). We also determined diagnostic value indices for potential cutoff points ranging from 8 to 40. The optimal cutoff value for MPAI was found to be >14, which corresponded to the maximum Youden index (Youden index = 0.751).
Results: The latent classification process in this research confirmed the existence of three distinct mobile phone user groups. We also examined the psychosocial characteristics that varied in relation to the severity levels of addiction.
Conclusion: This study provides valuable insights into the categorization of adolescents based on the severity of mobile phone addiction and sheds light on the psychosocial characteristics associated with different addiction levels. These findings are expected to enhance our understanding of mobile phone addiction traits and stimulate further research in this area.
In the past decade, the number of adolescents that use the internet has grown enormously. Latest figures show that approximately 5.3 billion of the Earth’s 8 billion people used the internet in 2022, or roughly 66% of the world’s population. At the same time, three quarters of the population aged 10 years and over owned a mobile phone. The statistics further show that young people are the driving force of connectivity, with 75% of 15–24 year olds now regularly online (1). According to the 51st Statistical Report on Internet Development in China published in December 2022, the proportion of individuals using the internet via mobile phones reached 99.8%. Among them, users aged 10–19 now account for 14.3% of the total number of users (2). In mainland China, around 80% of teenagers possess mobile phones, with nearly 40% of them using mobile phones without restrictions (3). While advances in the availability of the internet have brought many benefits to its users, its widespread adoption has also led to various types of harm, particularly to adolescents who are in a rapid stage of physical and psychological development and are susceptible to external influences. Therefore, excessive mobile phone usage may lead to unhealthy physical and psychological outcomes in teenagers.
Many studies have identified the predictors of mobile phone addiction: excessive use of mobile phones can contribute to negative physiological and psychological consequences, such as headaches and sleep disorders (4), neuroticism (5), trouble concentrating (6), and stress, anxiety, and depression (7). Although some studies have established the relationship between mental health and mobile phone addiction (8–10), all have ignored the within-class correlation between mobile phone addiction and other phenotypes. Therefore, the present study used latent class analysis to narrow the research gap in the field of psychological resilience and mobile phone addiction.
Mobile phone addiction can be classified as a behavioral addiction, exhibiting four typical features: compulsion, functional impairment, tolerance, and withdrawal (11). The addictive nature of mobile phones aligns with behavioral addiction, a disorder characterized by behavioral symptoms linked to pleasure and craving (12). Mobile phone addiction has been referred to as one of the most important social problems of the modern era (13). Problematic mobile phone use has been related to cognitive impairments (14) and depression (15). Addiction to mobile phones can be described as a secretive disease that as it results in damaging psychological health and impacting the quality of life, it is only natural to try to understand the behaviors associated with it (16).
Psychological distress includes symptoms of stress, anxiety, and depression. Significant psychological distress indicates impaired mental health and may reflect common mental disorders such as depression and anxiety disorders (17).
Severe psychological distress is connected with problematic health behaviors such as extreme alcohol consumption, smoking, and a propensity to commit (or an attempt to commit) suicide (18). Psychological distress among students is influenced by sociodemographic and contextual factors, academic-related factors such as performance and achievement at school or college, as well as personality and individual differences (18). Many studies have reported a high prevalence of psychological distress among adolescents. Prevalence rates of 40% have been recorded in China (19), 54% in Saudi Arabia (20), and 20.6% in Tanzania (21). Academic stress and adverse life events may account for much of this high prevalence of psychological distress among adolescents (22). However, a growing number of adolescents now spend a good deal of their time on their mobile phones, leading to an increasing incidence of mobile phone addiction. Mobile phone addiction has been regarded as an important cause of a variety of psychological and behavioral problems (23). Adolescents immersed in the content of their mobile phones may experience added psychological distress due to poor sleep quality and a tendency to procrastinate resulting from excessive or uncontrolled phone usage. The extent of disruption caused by mobile phone usage on anxiety and sleep quality exacerbates the deterioration of mental health, increasing levels of psychological tension and physiological agitation. This can have negative repercussions, leading to stress and poor health outcomes.
“Defense mechanisms” are a psychological process, typically accompanied by a behavioral response, used to cope with challenging situations, to manage conflicts, and to safeguard the basic functioning from disturbances caused by distressing, painful, and unacceptable thoughts, feelings, and experiences (24). Psychological protection plays a crucial role in the process of forming the individual and the individual’s needs. These mechanisms shield individuals from negative emotional experiences, facilitate the maintenance of psychological equilibrium, resolve interpersonal conflicts, and occur at the unconscious and subconscious levels of the psyche. Adolescent crisis is one of the most intricate issues within individual development. Adolescents are in a continuous state of adapting to physical and physiological changes, undergoing what is often termed a “hormonal storm.” In its most general form, it can be said that adolescents appear to be consistently under stress, and attempts at restoring personal equilibrium should be considered a central feature of adolescence.
The psychological resilience of adolescents, as one of the psychological defenses, has been confirmed by numerous researchers (25–27).
Cong et al. (28) verified that heightened psychological resilience may reduce the rate of suicide ideation in adolescents. Resilience has been related to decreased psychological distress and to increased positive experiences (29). Resilience can enhance self-assessment and recognized social support and thus favor the high level of emotional regulation skills in adolescents (30). Moreover, psychological resilience serves as a protective factor against smartphone addiction. Zhao et al. (7) revealed that psychological resilience has a significant negative impact on mobile phone addiction, that is, the stronger the psychological resilience, the less likely the mobile phone addiction. Resilience appears to negatively predict mobile phone addiction among minority preparatory students (31).
Based on our literature review, the present study focused on the relationship between mobile phone addiction and the unique characteristics of addicted mobile phone users. This study performed Latent Class Analysis (LCA) within the adolescent sample to identify subgroups representing mobile phone addiction and high-risk usage based on the severity of an individual’s mobile phone usage. Then, cross-sectional comparisons were conducted on the psychological characteristics of each group, leading to an estimate of the ratios of these groups in the population.
In this study, 2,300 adolescents from middle schools and high schools in Henan province of China were asked to complete the questionnaires from June 1 to August 30, 2022. In total, 2,230 valid questionnaires were procured, with an effective rate of 96.96%. Among the respondents, the mean age was 14.92 years (SD = 1.867, range 12–19 years), including 962 boys (43.1%) and 1,268 girls (56.9%). Voluntary participation was highlighted in an announcement. The questions of the survey covered the background and confidentiality information of this study, and participants’ privacy was protected. The participants completed the questionnaires at their own school and no incentives were provided. Incomplete questionnaires were excluded from this study.
The DASS-21 was used to evaluate the key symptoms of depression, anxiety, and stress (32) and collected information referred to the week prior to completing the questionnaire. The DASS-21 has been proven to have competent psychometric properties and is equivalent to other accurate questionnaires. This scale is classified into a 4-Likert reply from 0 to 3, where 0 = Nothing and 3 = Most of the time. This self-report instrument was designed to assess DASS, and includes three subscales: (1) the stress subscale, which measures tension, agitation, difficulty relaxing, and negative effect; (2) the anxiety subscale, which assesses autonomic arousal, skeletal musculature effects, situational anxiety, and subjective experience of anxiety arousal; and (3) the depression subscale, which measures hopelessness, dysphoria, lack of interest, self-deprecation, and inertia. The reliability coefficients of depression, anxiety and stress were 0.82, 0.82 and 0.79, respectively. The Cronbach’s alpha of the total scale was 0.89.
The MPAI has been used to identify the symptoms of mobile phone addiction among adolescents in Hong Kong (33). The scale includes 17 items and 4 subscales answered on a five-point Likert scale (5 = Always; 4 = Often; 3 = Occasionally; 2 = Rarely; and 1 = Not at all). The 4 subscales included “Inability to control craving”; “Anxiety and feeling lost”; “Productivity loss”; “Withdrawal and escape.” The Chinese version of the MPAI has had good reliability and validity when used with students (34, 35). The higher the score indicates a higher degree of mobile phone addiction. The Cronbach’s alpha of scale was 0.886. In addition, items 3, 4, 5, 6, 8, 9, 14, and 15 were designed to be mobile phone addiction screening questions. If participants answered five or more questions with a three or above score, they were regarded as mobile phone addicts. The others were categorized as non-phone addicts.
The RSCA was developed by Chinese scholars Hu and Gan (36) according to the process model of the resilience concept and applied to Chinese adolescents. There are 27 items divided into five factors: goal focus, emotion control, positive cognition, family support and interpersonal assistance. This scale is a five-point Likert-type scale from 1 = Completely disagree to 5 = Completely agree. This scale is especially appropriate in the evaluation of Chinese adolescents’ resilience, and is widely used in China under various situations. The reliability of the total scale was 0.85.
All data analyses were performed using SPSS 26.0 and Mplus 8.5 (37). First, descriptive data were received using SPSS 26.0, and correlation variables were calculated using Pearson’s correlations. Second, the present study used Latent Class Analysis (LCA) to identify latent mobile phone addiction class among Chinese adolescents, and the LCA performed by Mplus 8.5 to discover subgroups that displayed similar patterns of individual characteristics (i.e., class homogeneity) and diverse patterns across subgroups (i.e., class separation). LCA is a form of finite mixture modeling which involves clustering, density estimation, and random-effects modeling. The data analysis used fit indices to evaluate the best fitting model, including maximum log likelihood (LL), Akaike information criterion (AIC), the Bayesian information criteria (BIC), the sample-size adjusted Bayesian information criteria (SABIC), entropy (an indication of classification uncertainty), the Lo Mendell and Rubin likelihood ratio test (LMR), and the bootstrap likelihood ratio test (BLRT). Higher entropy values suggest a model that better divides the data into profiles, with values of >0.80 encouraging the minimal uncertainty of the profile classification of adolescents, and a significant BLRT, which compares the fit of the k-profile model with the k-1 profile model. The means of each class were stabilized using the logit values procured from the initial mix model, thus the latent class categorization was not influenced by subsequently added covariates. Finally, the ultimate class model was subsequently returned on the covariates.
We included 2,230 participants, including 962 boys and 1,268 girls in the final analysis. The proportion of girls was slightly higher than that of boys (56.9% vs. 43.1%, respectively). The mean age was 14.92 years (SD = 1.867, range 12–19 years). There were 1,223 (54.84%) participants from middle school, 1,007 (45.16%) from high school, 161 (7.2%) from one-child families, and 2,069 (92.8%) from multi-child families. The other results are shown in Table 1.
The variables correlated with the constructs in Table 2 were less than 0.85. The discriminant validity value (<0.85) was met in the construct correlation (38). We found that some demographic characteristics were related to the participants’ mobile phone addiction. The results of the analysis showed that academic performance, the primary caregiver, parenting style, family style, and financial status were positively correlated with the adolescents’ mobile phone addiction. Conversely, the education level of the parents, the educational level of the primary caregiver, and family history of psychosis were negatively correlated with the adolescents’ mobile phone addiction.
The major study variables correlated with the constructs in the present study. Stress, anxiety, depression, and psychological resilience were correlated with the adolescents’ mobile phone addiction (Table 3). Furthermore, the correlations suggest that there is an association between the psychological variables and mobile phone addiction.
This study involves five different model fit statistics that were used to assess which LCA model fits the data best. We detected that AIC values decreased gradually from the 2- to 5-class models, BIC values decreased gradually from the 2- to 4-class models, while BIC did not decrease from the 4- to 5-class model, which the 3-class model had SABIC as well as significant LMR and BLRT. The 3-class model also had the highest entropy score, reflecting the best classification accuracy among other models, suggesting that participants were ranged into three mutually exclusive classes. To sum up, the 3-class model was determined the optimal latent class, therefore we selected the 3-class model as the best fitting model (Table 4). Figure 1 shows the different classes and their means for each mobile phone use subscale. The first profile is called the “normal mobile phone use class” (n = 482, 21.50%), and included the least number of adolescents in all subscales of mobile phone use. The opposite class, namely the class in which mobile phone adoption was highest for all subscales of mobile phone use, is called the “mobile phone addiction class” (46.65%). The third class included the most adolescents (n = 1,058) and is called the “high-risk mobile phone use class.” Adolescents in this class are characterized by a moderate population in all mobile phone use subscales.
Using the binary outcomes (“non-case” and “case”) procured from LCA as the reference standard, the receiver operating characteristic (ROC) plot of the sensitivity versus 1-specificity of various MPAI scores revealed the area under the curve (AUC) of 0.893 (95% CI, 0.879 to 0.905, p < 0.001) (Figure 2). The diagnostic value indices for potential generated cut-off points from 8 to 40. The optimal cut-off value of MPAI was >14, which corresponded to the maximum Youden index (Youden index = 0.751). In this case, the sensitivity was 96.5%, specificity was 78.64%, PPV and NPV were 86.7 and 93.9%, respectively (Table 5). The positive group (defined as MPAI score > 14) included 59.13% of the participants.
Figure 2. ROC curve of the MPAI using the high-risk class derived from latent class model as gold standard.
Table 5. ROC analysis of the MPAI using the high-risk class derived from latent class model as gold standard.
A multinomial logistic regression is conducted with goal focus, interpersonal support emotional control, positive cognition, and family support as possible psychological resilience predictors of LCA membership as well as age, grade, academic performance, and family financial status as possible background characteristic predictors of LCA membership. As shown in Table 6, the multinomial logistic regression was employed by us to analyze Cluster normal mobile phone use, high-risk mobile phone use and mobile phone addiction as outcomes. Academic performance and grade negatively predicted memberships in cluster high-risk mobile phone use vs. normal mobile phone use and mobile phone addiction vs. normal mobile phone use. Goal focus, interpersonal support emotional control, positive cognition, and family support positively predicted memberships in cluster high-risk mobile phone use vs. normal mobile phone use, mobile phone addiction vs. normal mobile phone use, and mobile phone addiction vs. high-risk mobile phone use. Family financial status negatively predicted membership in cluster high-risk mobile phone use vs. normal mobile phone use, in contrast to cluster mobile phone addiction vs. high-risk mobile phone use.
Despite the growth in the incidence of mobile phone addiction among adolescents, many researchers tend to focus on potential risk factors contributing to mobile phone addiction, but few empirical studies have examined typologies of mobile phone addiction in a sample of Chinese adolescents. The current study empirically showed that a three-class model most effectively classified Chinese adolescents giving different degrees of problematic mobile phone usage. The three classes affected by the LCA were as follows: normal mobile phone use, high-risk mobile phone use, and mobile phone addiction. 21.25% of the mobile phone users fell into the category of normal, 32.1% of the mobile phone users were categorized as high-risk, and the rest (46.65%) were placed into the addiction group. When compared with the normal group, participants in the other two groups manifested more severe mobile phone-related problems. They also revealed a poorer psychosocial profile in the matter of stress, anxiety, depression, goal focus, interpersonal support, emotional control, and positive cognition.
The present study proposes that different classes of mobile phone users may be recognized through LCA, supplying a comprehensive view of the patterns of adolescents’ mobile phone use. This result was consistent with the findings of previous studies (39, 40). Given the fact that a mobile phone can provide more media resources than just a few years ago, mobile phone addiction may have more underlying groups. For this reason, personal characteristics may be a possible interpretation. Participants categorized in the LCA of mobile phone addiction were adolescents. The physical and psychological growth of adolescents is easily affected by many factors. The current study showed that academic performance, the education level of the mother, the educational level of the primary caregiver, the type of family education, family history of psychosis, stress, anxiety, depression, and psychological resilience were all related to mobile phone addiction of the adolescent participants. This result is consistent with findings of previous studies (10, 41, 42).
When compared with the high-risk mobile phone use group and the normal mobile phone use group, the result demonstrated that age, grade, psychological resilience and academic performance were negatively correlated with problematic mobile phone use. In other words, introducing mobile phones to children at a young age can lead to problematic mobile phone usage; the better the academic performance, the less likely an individual will develop problematic mobile phone usage. In this study, although grade was related to high-risk mobile phone use, middle school (9th grade) was not associated with high-risk mobile phone use. Due to the transition to higher education, 9th grade students have to deal with academic pressure, leading them to invest more time and energy into their studies.
Fischer-Grote et al. (43) suggested that gender, age, family, and personality might be risk factors for problematic smartphone use. Comparison between the mobile phone addiction group and the normal mobile phone use group revealed that psychological resilience and school grade were significantly negatively related to mobile phone addiction. There was a negative correlation between moderate academic performance and smartphone addiction. This study was consistent with previous research findings (7, 10, 44). Abd Rashid et al. (45) considered that an individual’s attention on academic study may be diverted if they have a severe addiction to their mobile phone. Bai et al. (46) regarded the effect of mobile phone addiction on academic rank was significant, pointing to a strong association between these two variables. When mobile phone addiction vs. high-risk mobile phone use, psychological resilience, gender, school grade (except for the 9th grade and the freshman year of high school), and family financial status had an effect on mobile phone addiction.
Liu et al. (47) considered that gender moderated the association between peer victimization and mobile phone addiction, with this association being stronger in girls than in boys. Korean girls were likely to more use their mobile phones and were at higher risk of mobile phone addiction (48). Borislav and Dijana (49) thought that age had a negative correlation with mobile phone addiction, while family risk factors were positively associated with disorder of early psychological development and mobile phone addiction. The subjective economic level, academic stress, and parental support were associated with a tendency to become addicted to the smartphone (50). In another study, Lee et al. (51) revealed that mobile phone addiction was related to higher levels of depression among low-income boys.
Based on the selected cut-off value, the prevalence of MPA among our sampled adolescents of Henan province in China was 59.13%. Alavi et al. (52) used the Mobile phone abuse questionnaire (MPA) to measure mobile phone usage of students, which showed that the cut-off point was 46 participants (31.2%) who were identified as having mobile phone addiction. The Smartphone Addition Inventory in Spanish (SPAI-Spain) was employed to investigate mobile addiction in a number of university communities in Spain. The result revealed that 23.67% of the participants were classified as dependent on the smartphone. As for gender, the percentage of women who suffered smartphone addiction accounted for 53.88% of the total (53). The literature review also revealed that the prevalence of smartphone addiction was 32% in Iran (54), 30.9% of middle school students in South Korea were categorized as a high-risk group (55), and 33.1% of secondary school students (56). Elsewhere, the rate of smartphone addiction among children and adolescents was 40.4% in 31 provinces of China (57), 46.1% of adolescents in Nigeria had moderate to high problematic phone usage (58), 71.9% of Saudi Arabian dental students had a mobile phone addiction (59), as did 85.4% of medical students in India (60). These variances could be partially assigned to the differences in participants and measurement tools. In this study, excessive use of mobile phone was due to the utilization of the virtual environment that was prevalent during the COVID-19 pandemic. At this time, many schools adopted online formats for teaching and various other educational activities, leading adolescents to primarily use smartphones or computers for remote learning. As a result, they spent more time on screens. Moreover, in China, socializing, shopping, recreation, and payment is now often done using social media such as TikTok, Wechat, QQ, interactive computer games, and so on, and this has become a principal factor of mobile phone addiction.
Based on these results, we suggest these cutoff point scores should be used as a threshold for normal mobile phone users as distinct from addicted mobile phone users. In research or diagnosis of mobile phone addiction, these results should be illustrated with care to sustain the validity of the questionnaire. Some consideration should be given when reporting cutoff value in interpretation, utilization of the cutoff value will rely on the purpose of the questionnaire and the popularity of mobile phone usage in the population under study. Moreover, no cutoff value is 100% accurate, so diagnostic errors will exist (61).
In sum, the current study used a sophisticated latent class analysis, and it highlights the differential associations between heterogeneous classes of multidimensional mental health and psychological resilience indicators for addictive mobile phone use in adolescents. Our discovery of the individual differences in factors that cause mobile phone addiction emphasizes the need to focus more on mobile phone users’ discrete psychological resilience and family status in future research. Further, the consideration of multidimensional pointers in our research spreads understanding of the united operation of various individual difference components in connection with varying degrees of risk for mobile phone addiction. Moreover, our results carry important real-world implications, in that suitable prevention or intervention methods for mobile phone addiction should target the particular psychological dimensions that are associated with mobile phone addiction, especially those that indicate a personal uneven psychological profile. For example, those whose latent classes are characterized by poor psychological resilience and high psychological distress will require stronger social support to improve their ability to regulate themselves psychologically. This appears to be a valid intervention device to reduce psychological dilemma with regards to addictive mobile phone use.
This report must address several limitations of the study. The research used cross-sectional data. Given the nature of cross-sectional data, this study was unable to infer temporal sequence relationships, limiting its capacity for causal inferences and instead focused on correlation. The data were self-reported and may have been influenced by social desirability bias, despite efforts in this study to reduce bias through measures such as anonymity and absence of teachers. As this study drew on a relatively small number of schools in two cities in Henan province, the generalizations should be approached with caution. Therefore, in the future, more complicated longitudinal studies will be needed to establish the directionality of the relationship between these latent classes and mobile phone addiction. Future research should include more representative and diverse samples, as well as investigate the relationship between psychological characteristics and mobile phone addiction in other populations.
The current study discovered different patterns of problematic mobile phone use among adolescents living in Henan province. Three latent classes were recognized among the whole population. This is particularly important, as misuse of mobile phones is prevalent among adolescents, and ever-developing mobile phone technology may be perceived as a risk for increasing prevalence rates. Individual academic performance, parental education level, low psychological resilience, and a family history of mental illness appear to contribute to smartphone addiction amongst adolescents. In our study, we found that in the high-risk mobile phone addiction vs. normal mobile phone use, age, psychological resilience, school grade, and academic performance play a role in problematic phone usage. In the mobile phone addiction vs. normal smartphone use, psychological resilience, school grade, and academic performance influence mobile phone addiction. In the high-risk smartphone addiction vs. mobile phone addiction, psychological resilience, gender, and school grade contribute to smartphone addiction. The family’s economic status seems to have minimal impact on an adolescent’s mobile phone addiction. These results give a reliable basis to evaluate a lot of problems emanating from excessive mobile phone use. The increase in the proportion of mobile phone addiction among adolescents provides a rationale for implementing preventive measures and conducting further research.
The raw data supporting the conclusions of this article will be made available by the authors, without undue reservation.
The studies involving humans were approved by Ethics Committee of Nanyang Medical College (number: NYYZ20240001). The studies were conducted in accordance with the local legislation and institutional requirements. Written informed consent for participation in this study was provided by the participants’ legal guardians/next of kin.
JW: Conceptualization, Writing – original draft. LJ: Investigation, Writing – review & editing. YL: Data curation, Investigation, Writing – review & editing. QL: Investigation, Writing – review & editing. YZ: Data curation, Investigation, Writing – review & editing. JZ: Data curation, Writing – review & editing. YJ: Data curation, Writing – review & editing. ZF: Conceptualization, Investigation, Supervision, Writing – review & editing.
The author(s) declare that financial support was received for the research, authorship, and/or publication of this article. This work was supported by the Vocational education teaching reform research and practice project, Henan Provincial Department of Education (Grant Number: (2024) 05842); Vocational education teaching reform research and practice project, Nanyang Medical College (Grant Number: JGXM202409).
Major thanks to YL at the Nanyang Medical College for scientific guidance and advice and for data collection support, respectively. We would also like to thank all the adolescents who took part in this study.
The authors declare that the research was conducted in the absence of any commercial or financial relationships that could be construed as a potential conflict of interest.
All claims expressed in this article are solely those of the authors and do not necessarily represent those of their affiliated organizations, or those of the publisher, the editors and the reviewers. Any product that may be evaluated in this article, or claim that may be made by its manufacturer, is not guaranteed or endorsed by the publisher.
DASS, depression, anxiety, and stress; MPAI, mobile phone addiction index; RSCA, the Resilience Scale for Chinese Adolescents; ROC, receiver operating characteristic; LCA, Latent Class Analysis; AUC, area under the curve
1. Committed to connecting the world (2023). Available at: https://www.itu.int/en/Pages/default.aspx (accessed August 9, 2023).
2. China Internet Network Information Center (2023). Available at: https://cnnic.cn/n4/2023/0302/c199-10755.html (accessed August 9, 2023).
3. Lu, G-L, Ding, Y-M, Zhang, Y-M, Huang, H-T, Liang, Y-P, and Chen, C-R. The correlation between mobile phone addiction and coping style among Chinese adolescents: a meta-analysis. Child Adolesc Psychiatry Ment Health. (2021) 15:60. doi: 10.1186/s13034-021-00413-2
4. Liu, QQ . Mobile phone addiction and sleep quality among Chinese adolescents: a moderated mediation model. Comput. Hum Behav. (2017) 72:108–14. doi: 10.1016/j.chb.2017.02.042
5. Lei, LYC, Ismail, MAA, Mohammad, JAM, and Yusoff, MSB. The relationship of smartphone addiction with psychological distress and neuroticism among university medical students. BMC Psychol. (2020) 8:97. doi: 10.1186/s40359-020-00466-6
6. Lepp, A, Barkley, JE, and Karpinski, AC. The relationship between cell phone use, academic performance, anxiety, and satisfaction with life in college students. Comput Hum Behav. (2014) 31:343–50. doi: 10.1016/j.chb.2013.10.049
7. Zhao, Z, Zhao, S, Wang, Q, Zhang, Y, and Chen, C. Effects of physical exercise on Mobile phone addiction in college students: the chain mediation effect of psychological resilience and perceived stress. Int J Environ Res Public Health. (2022) 19:15679. doi: 10.3390/ijerph192315679
8. Mei, S, Hu, Y, Wu, X, Cao, R, Kong, Y, Zhang, L, et al. Health risks of mobile phone addiction among college students in China. Int J Ment Heal Addict. (2022) 21:1–16. doi: 10.1007/s11469-021-00744-3
9. Patel, SASAW, and Puri, PA. A study of mobile phone addiction and mental health among adolescent girls studying in various streams. Int JInd Psychȯl. (2017) 5:99. doi: 10.25215/0501.099
10. Shen, X . Is psychological resilience a protective factor between motivations and excessive smartphone use? J Pac Rim Psychol. (2020) 14:e17. doi: 10.1017/prp.2020.10
11. Lin, YH, Chang, LR, Lee, YH, Tseng, HW, Kuo, TB, and Chen, SH. Development and validation of the smartphone addiction inventory (SPAI). PLoS One. (2014) 9:e98312. doi: 10.1371/journal.pone.0098312
12. Black, DW . Behavioural addictions as a way to classify behaviours. Can J Psychiatry. (2013) 58:249–51. doi: 10.1177/070674371305800501
13. Askarizadeh, G, Poormirzaei, M, and Hajmohammadi, R. Identity processing styles and cell phone addiction: the mediating role of religious coping. J Res Religion Health. (2017) 3:18–29.
14. Wilmer, HH, Sherman, LE, and Chein, JM. Smartphones and cognition: a review of research exploring the links between mobile technology habits and cognitive functioning. Front Psychol. (2017) 8:1–16. doi: 10.3389/fpsyg.2017.00605
15. Geng, Y, Gu, J, Wang, J, and Zhang, R. Smartphone addiction and depression, anxiety: the role of bedtime procrastination and self-control. J Affect Disord. (2021) 293:415–21. doi: 10.1016/j.jad.2021.06.062
16. Kim, K, Milne, G, and Bahl, S. Smartphone addiction and mindfulness: an intergenerational comparison. Int J Pharm Healthc Mark. (2018) 12:25–43. doi: 10.1108/IJPHM-08-2016-0044
17. Cuijpers, P, Smits, N, Donker, T, ten Have, M, and de Graaf, R. Screening for mood and anxiety disorders with the five-item, the three-item, and the two-item mental health inventory. Psychiatry Res. (2009) 168:250–5. doi: 10.1016/j.psychres.2008.05.012
18. Sharp, J, and Theiler, S. A review of psychological distress among university students: pervasiveness, implications and potential points of intervention. Int J Adv Couns. (2018) 40:193–212. doi: 10.1007/s10447-018-9321-7
19. Jaisoorya, T, Desai, G, Beena, K, Beena, M, Ellangovan, K, and Thennarasu, K. Prevalence and correlates of psychological distress in adolescent students from India. East Asian Arch Psychiatr. (2017) 27:56–62.
20. Bukuluki, P, Kisaakye, P, Wandiembe, SP, and Besigwa, S. Suicide ideation and psychosocial distress among refugee adolescents in Bidibidi settlement in West Nile, Uganda. Discov Psychol. (2021) 1:1–9. doi: 10.1007/s44202-021-00003-5
21. Pengpid, S, and Peltzer, K. Psychological distress and its associated factors among school-going adolescents in Tanzania. Psychol Stud. (2020) 65:174–81. doi: 10.1007/s12646-020-00550-2
22. Anyanwu, MU . Psychological distress in adolescents: prevalence and its relation to high-risk behaviors among secondary school students in Mbarara municipality, Uganda. BMC Psychol. (2023) 11:5. doi: 10.1186/s40359-023-01039-z
23. Yang, X, Zhou, Z, Liu, Q, and Fan, C. Mobile phone addiction and adolescents’ anxiety and depression: the moderating role of mindfulness. J Child Fam Stud. (2019) 28:822–30. doi: 10.1007/s10826-018-01323-2
24. Perrotta, G . Human mechanisms of psychological defence: definition, historical and psychodynamic contexts, classifications and clinical profiles. Int J Neurorehabilit Eng. (2020) 7:1000360.
25. Yavuz, K . Psychological resilience in children and adolescents: the power of self-recovery. Currt Approac Psychiatry. (2023) 15:112–31. doi: 10.18863/pgy.1054060
26. Vanderley, ICS, Vanderley, MDAS, Santana, ADDS, Scorsolini-Comin, F, Brandão Neto, W, and Monteiro, EMLM. Factors related to the resilience of adolescents in contexts of social vulnerability: integrative review. Enferm Global. (2020) 19:612–25.
27. Dias, PC, and Cadime, I. Protective factors and resilience in adolescents: the mediating role of self-regulation. Psicol Educ. (2017) 23:37–43. doi: 10.1016/j.pse.2016.09.003
28. Cong, EZ, Wu, Y, Cai, YY, Chen, HY, and Xu, YF. Association of suicidal ideation with family environment and psychological resilience in adolescents. Chin J Contemp Pediat. (2019) 21:479–84. doi: 10.7499/j.issn.1008-8830.2019.05.016
29. Beames, JR, Li, SH, Newby, JM, Maston, K, Christensen, H, and Werner-Seidler, A. The upside: coping and psychological resilience in Australian adolescents during the COVID-19 pandemic. Child Adolesc Psychiatry Ment Health. (2021) 15:77. doi: 10.1186/s13034-021-00432-z
30. Surzykiewicz, J, Skalski, SB, Sołbut, A, Rutkowski, S, and Konaszewski, K. Resilience and regulation of emotions in adolescents: serial mediation analysis through self-esteem and the perceived social support. Int J Environ Res Public Health. (2022) 19:8007. doi: 10.3390/ijerph19138007
31. Liao, Y, Ye, B, Jin, P, Xu, Q, and Li, A. The effect of resilience on Mobile phone addiction among minority preparatory students in Han District: moderated mediating effect. Psychol Dev Educ. (2017) 33:487–95.
32. Lovibond, SH, and Lovibond, PF. (1995). Manual for the depression anxiety and stress scales (DASS21) Second. Sydney, NSW: Psychology Foundation of Australia, 1–3.
33. Leung, L . Linking psychological attributes to addiction and improper use of the mobile phone among adolescents in Hong Kong. J Child Media. (2008) 2:93–113. doi: 10.1080/17482790802078565
34. Han, L, Geng, J, Jou, M, Gao, F, and Yang, H. Relationship between shyness and mobile phone addiction in Chinese young adults: mediating roles of self-control and attachment anxiety. Comput Hum Behav. (2017) 76:363–71. doi: 10.1016/j.chb.2017.07.036
35. Hao, Z, Jin, L, Li, Y, Akram, HR, Saeed, MF, Ma, J, et al. Alexithymia and mobile phone addiction in Chinese undergraduate students: the roles of mobile phone use patterns. Comput Hum Behav. (2019) 97:51–9. doi: 10.1016/j.chb.2019.03.001
36. Hu, YQ, and Gan, YQ. Development and psychometric validity of the resilience scale for Chinese adolescents. Acad Psychol Sin. (2008) 40:902–12. doi: 10.3724/SP.J.1041.2008.00902
37. Muth´en, L. K., and Muth´en, B. O. (1998–2012). Mplus Users Guide. Seventh Edition. Los Angeles, CA: Muth´en & Muth´en.
38. Kline, R. B. (2023). Principles and practice of structural equation modeling. Guilford publications.
39. Li, JB, Wu, AM, Feng, LF, Deng, Y, Li, JH, Chen, YX, et al. Classification of probable online social networking addiction: a latent profile analysis from a large-scale survey among Chinese adolescents. J Behav Addict. (2020) 9:698–708. doi: 10.1556/2006.2020.00047
40. Elhai, JD, Rozgonjuk, D, Yildirim, C, Alghraibeh, AM, and Alafnan, AA. Worry and anger are associated with latent classes of problematic smartphone use severity among college students. J Affect Disord. (2019) 246:209–16. doi: 10.1016/j.jad.2018.12.047
41. Ma, A, Yang, Y, Guo, S, Li, X, Zhang, S, and Chang, H. The impact of adolescent resilience on Mobile phone addiction during COVID-19 normalization and flooding in China: a chain mediating. Front Psychol. (2022) 13:865306. doi: 10.3389/fpsyg.2022.865306
42. Shoukat, S . Cell phone addiction and psychological and physiological health in adolescents. EXCLI J. (2019) 18:47–50.
43. Fischer-Grote, L, Kothgassner, OD, and Felnhofer, A. Risk factors for problematic smartphone use in children and adolescents: a review of existing literature. Psychiater. (2019) 33:179–90. doi: 10.1007/s40211-019-00319-8
44. Xie, G, Wu, Q, Guo, X, Zhang, J, and Yin, D. Psychological resilience buffers the association between cell phone addiction and sleep quality among college students in Jiangsu Province, China. Front Psych. (2023) 14:1105840. doi: 10.3389/fpsyt.2023.1105840
45. Abd Rashid, JA, Aziz, AA, Rahman, AA, Saaid, SA, and Ahmad, Z. The influence of mobile phone addiction on academic performance among teenagers. Malays J Commun Jilid. (2020) 36:408–24. doi: 10.17576/JKMJC-2020-3603-25
46. Bai, C, Chen, X, and Han, K. Mobile phone addiction and school performance among Chinese adolescents from low-income families: a moderated mediation model. Child Youth Serv Rev. (2020) 118:105406. doi: 10.1016/j.childyouth.2020.105406
47. Liu, QQ, Yang, XJ, Hu, YT, and Zhang, CY. Peer victimization, self-compassion, gender and adolescent mobile phone addiction: unique and interactive effects. Child Youth Serv Rev. (2020) 118:105397. doi: 10.1016/j.childyouth.2020.105397
48. Park, S-Y, Yang, S, Shin, C-S, Jang, H, and Park, S-Y. Long-term symptoms of Mobile phone use on Mobile phone addiction and depression among Korean adolescents. Int J Environ Res Public Health. (2019) 16:3584. doi: 10.3390/ijerph16193584
49. Borislav, Đ, and Dijana, K. The impact of adolescent resilience on mobile phone addiction in MONTENEGRO and Serbia. J Entrepren Bus Resil. (2022) 5:77–85.
50. Kim, J-H . Factors associated with smartphone addiction tendency in Korean adolescents. Int J Environ Res Public Health. (2021) 18:11668. doi: 10.3390/ijerph182111668
51. Lee, J, Lim, H, Allen, J, Choi, G, and Jung, J. Smartphone addiction and depression among low-income boys since COVID-19: the moderating effect of being an only child. Healthcare. (2021) 9:1350. doi: 10.3390/healthcare9101350
52. Alavi, SS, Ghanizadeh, M, Mohammadi, MR, Jannatifard, F, Salehi, M, Farahani, M, et al. Psychometric properties of Mobile phone abuse (MPA): exploratory and confirmatory factor analysis in student Mobile users. Iran J Psychiatry. (2021) 16:187–97. doi: 10.18502/ijps.v16i2.5820
53. Ballestar-Tarín, ML, Simó-Sanz, C, Chover-Sierra, E, Saus-Ortega, C, Casal-Angulo, C, and Martínez-Sabater, A. Self-perception of dependence as an indicator of smartphone addiction—establishment of a cutoff point in the SPAI–Spain inventory. Int J Environ Res Public Health. (2020) 17:3838. doi: 10.3390/ijerph17113838
54. Kheradmand, A, Amirlatifi, ES, Sohrabi, MR, and Meybodi, AM. Validation of the Persian smartphone addiction scale among Tehran university students, Iran. Int J High Risk Behav Addict. (2019) 8:e81176. doi: 10.5812/ijhrba.81176
55. Cha, SS, and Seo, BK. Smartphone use and smartphone addiction in middle school students in Korea: prevalence, social networking service, and game use. Health Psychol Open. (2018) 5:2055102918755046. doi: 10.1177/2055102918755046
56. Esmaeilpour, F, Letafatkar, A, Baker, JS, Dutheil, F, Khazaei, O, Rabiei, P, et al. Reliability and construct validity of the smartphone addiction scale short version (SAS-SV) in Iranian students. J Public Health. (2021) 31:1–9.
57. Zhou, N, Wang, SF, Zhu, XC, Wang, Y, Chen, L, Cao, HJ, et al. Analysis of mobile phone usage, smartphone addiction behavior, and related factors among children and adolescents in China. Chin J School Health. (2022) 8:1179–84. doi: 10.16835/j.cnki.1000-9817.2022.08.014
58. Balogun, FM, and Olatunde, OE. Prevalence and predictors of problematic smart phone use among pre-varsity young people in Ibadan, Nigeria. Pan Afr Med J. (2020) 36:285. doi: 10.11604/pamj.2020.36.285.18858
59. Venkatesh, E, Al Jemal, MY, and Al Samani, AS. Smart phone usage and addiction among dental students in Saudi Arabia: a cross sectional study. Int J Adolesc Med Health. (2017) 31:20160133. doi: 10.1515/ijamh-2016-0133
60. Sethuraman, AR, Rao, S, Charlette, L, Thatkar, PV, and Vincent, V. Smartphone addiction among medical college students in the Andaman and Nicobar Island. Int J Commun Med Public Health. (2018) 5:4273–7.
Keywords: adolescent, resilience, mobile phone addiction, DASS-21, ROC curve, cut-off point, latent class analysis
Citation: Wu JX, Jia L, Li Y, Liu Q, Zhang YY, Zhang J, Jia YR and Fan Z (2024) The interplay of psychological resilience and adolescent mobile phone addiction in Henan province, China: insights from latent class analysis. Front. Public Health. 12:1386500. doi: 10.3389/fpubh.2024.1386500
Received: 15 February 2024; Accepted: 17 April 2024;
Published: 20 June 2024.
Edited by:
Aleksandar Višnjić, University of Niš, SerbiaReviewed by:
Snezana Miodraga Radovanovic, University of Kragujevac, SerbiaCopyright © 2024 Wu, Jia, Li, Liu, Zhang, Zhang, Jia and Fan. This is an open-access article distributed under the terms of the Creative Commons Attribution License (CC BY). The use, distribution or reproduction in other forums is permitted, provided the original author(s) and the copyright owner(s) are credited and that the original publication in this journal is cited, in accordance with accepted academic practice. No use, distribution or reproduction is permitted which does not comply with these terms.
*Correspondence: Zhen Fan, eXpmZjYwMTFAMTYzLmNvbQ==
†These authors have contributed equally to this work and share first authorship
Disclaimer: All claims expressed in this article are solely those of the authors and do not necessarily represent those of their affiliated organizations, or those of the publisher, the editors and the reviewers. Any product that may be evaluated in this article or claim that may be made by its manufacturer is not guaranteed or endorsed by the publisher.
Research integrity at Frontiers
Learn more about the work of our research integrity team to safeguard the quality of each article we publish.