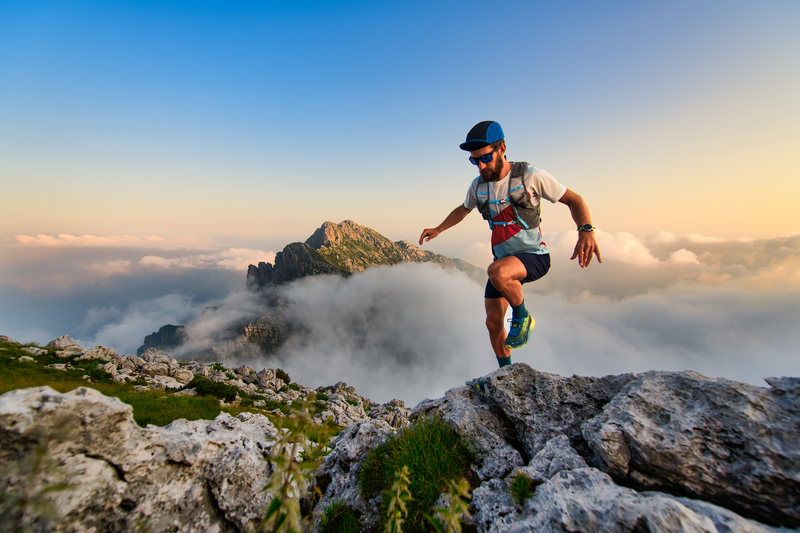
94% of researchers rate our articles as excellent or good
Learn more about the work of our research integrity team to safeguard the quality of each article we publish.
Find out more
ORIGINAL RESEARCH article
Front. Public Health , 03 June 2024
Sec. Digital Public Health
Volume 12 - 2024 | https://doi.org/10.3389/fpubh.2024.1386394
Objectives: To investigate the factor structure and verify the validity and reliability of the Korean version of the Digital Burnout Scale (DBS) among Generation Z university students.
Methodology: The World Health Organization guidelines were employed in the forward and back translation, synthesis, cross-cultural adaptation, and pre-survey phases to result in the Korean version of the DBS. The Korean version was then used to collect data from 330 university students online. Construct, convergent, discriminant, and concurrent validity, and internal consistency were assessed.
Findings: The Korean version of the DBS had three subscales (digital aging, digital deprivation, and emotional exhaustion) and included 24 items. The results of the confirmatory factor analysis indicated adequate model fit indices. Convergent, discriminant, and concurrent validity were satisfactory. The Cronbach’s ⍺ for the overall scale was 0.95.
Conclusion: The Korean version of the DBS has good reliability and validity and can be used as a tool to assess the level of risk of digital burnout and provide appropriate support and intervention.
Implications: The Korean version of the DBS will serve as a framework for developing healthy digital education by grasping individual characteristics. Longitudinal research is recommended to identify factors that cause digital usage and burnout for various age groups.
The development and widespread use of information and communication technologies have normalized the everyday use of various forms and functions of digital devices in all aspects of daily life (1, 2). The Coronavirus Disease 2019 (COVID-19) pandemic has been a catalyst for the digital transformation of daily life, changing traditional face-to-face interactions to non-face-to-face approaches through the application of digital technology (3). Consequently, many aspects of daily life depend on digital technology (4). The ease and speed with which digital technology can be used affects the quality of life; however, the digitization of daily life has various effects due to excessive time spent on digital devices, such as mental and behavioral health issues, academic performance problems, sleep issues, and musculoskeletal health problems (1, 5, 6).
The current generation of university students, Generation Z, is referred to as “Digital Natives” (7, 8). Born between the mid-1990s and early 2000s, they have grown up surrounded by digital devices since birth (9, 10). Having never experienced life before the Internet, they are sensitive to and active users of digital technology, with the smartphone being the most commonly used device regardless of gender (7). They prefer digital media for receiving diverse types of information and favor communication through digital technology over direct contact with people (11). Since there are various ways to express oneself online and form relationships, individuals can enjoy more freedom in interacting with others by building an image that differs from reality (12). Consequently, people become attendants who present an edited version of themselves that they believe others will best accept and often manage other people’s perceptions about them through what they post (13). Furthermore, online self-expression can differ from offline self-expression (14). This difference is confusing and may create a conflict between one’s online image and who one really is (15).
It is estimated that Generation Z individuals watch their smartphone screens for an average of 4–5 h a day (11). This generation has grown up with digital technology, is familiar with it, is greatly influenced by it, and has a strong presence on various digital platforms (16). They use it for various tasks such as checking emails, finding directions, taking pictures, buying, and entertainment (10). Of the Generation Z population, 98% have a smartphone and 97% have a social media account (17). However, problematic digital use can lead to feelings of isolation, which can lead to other chronic psychological disorders (9). Digital devices can affect the social, physical, and psychological functions of Generation Z and can cause neurological impairments including emotional, mental, and physical health problems such as insomnia, irritability, depression, social isolation, and musculoskeletal problems (8, 9, 11, 16, 17).
The excessive use of digital devices negatively impacts the academic performance, achievement, and learning adaptation of university students (2). Using digital devices until bedtime can lead to insomnia, decreased concentration, and less participation in academic activities, resulting in lower academic performance (18, 19). Therefore, the use of digital devices could disrupt learning and reduce motivation to learn (20). In addition, the improper and excessive use of digital education and learning technologies may lead to academic exhaustion (21). Excessive use of smartphones for non-academic purposes can hinder concentration on studies and lead to procrastination in completing assignments (21, 22). Several studies found a correlation between academic exhaustion and mobile phone use (19–24). Academic burnout is a common issue today, given that most learning takes place on computers and the boundary between school and the online world is blurred.
Academic burnout originates from the concept of job burnout and is usually characterized by three dimensions: emotional exhaustion, cynicism, and reduced academic efficacy (21, 25, 26). In the academic context, these dimensions translate to feeling exhausted because of study demands, having a cynical and detached attitude toward one’s studies, and feeling incompetent as a student (26, 27). Academic burnout can overwhelm students and lead to a loss of interest in activities they once enjoyed (19, 21, 24). Students experiencing academic exhaustion tend to lack self-control and use digital devices excessively (21, 24). Furthermore, phenomena such as nomophobia (fear of being without a mobile phone) (5), sleep disorders (18, 19), eye dryness (28), musculoskeletal health problems (29), reduced activity (30), and obesity may occur when smartphones are not available (1). Therefore, it is imperative to promptly assess and implement preventive measures to effectively address these issues.
Erten and Özdemir (31) developed the Digital Burnout Scale (DBS) in English and Turkish to measure the extent of digital burnout resulting from the use of digital devices. This scale facilitates proper assessment of and finding solutions to health issues. Even in the post-COVID-19 era, the digitalization of daily life has accelerated with the expansion of non-face-to-face and online stores, diversification of EdTech learning contents, and growth of the remote work collaboration tools market (16, 32, 33). Recognizing the increased risk of health issues related to digital exposure and overuse as daily patterns change owing to digitization, systematic prevention and management measures are needed (31). As such, using tools that can appropriately assess the physical, mental, and psychological issues of an individual is crucial (31).
During the COVID-19 pandemic, the concept of digital burnout emerged owing to the use of complementary and accessible technologies (31, 33–36). In our daily lives, we are constantly exposed to various digital devices, and without realizing it, may experience digital burnout from spending too much time on these (31, 34). However, individuals may be unaware of their situation, which makes it challenging to find solutions (3). Thus, the concept of digital burnout, a comprehensive psychological and physical problem caused by excessive exposure to digital culture, should be measured and evaluated. Therefore, this study aims to find individual levels of digital burnout in the digitalization era to enable careful observation and awareness of one’s digital usage patterns. Furthermore, it aims to evaluate the Korean version of the DBS through rigorous tool translation and validity and reliability surveys.
The next section of this paper provides a review of the current literature. Then, the methodology and findings of the study are presented, followed by the discussion, theoretical and managerial implications, and conclusion. Finally, the limitations of the study are delineated.
Burnout syndrome is an individual response to chronic work stress that develops progressively and can eventually become chronic and negatively impact the individual’s health (33, 37). Maslach (38) introduced and defined the concept of burnout as a gradual process of fatigue, cynicism, and reduced commitment among social care professionals. After several empirical studies, Maslach in collaborated with other authors (39, 40) reformulated the concept and elaborated a more rigorous and operational definition of burnout as a psychological syndrome characterized by emotional exhaustion, cynicism or depersonalization, and a reduced sense of professional efficacy (41). Burnout is a syndrome characterized by long-term physical and emotional fatigue and low work performance, which can lead to unproductive, inactive, and negative attitudes toward work (15, 34). Burnout negatively affects quality of life as it decreases mental, physical, and emotional health (42). Some signs and symptoms of digitally induced burnout are similar to those of normal burnout. The main difference between the two is evident in the long-term use of digital devices to the extent that individuals cannot be completely separated from their digital lives even during breaks (31, 33). Digital burnout is characterized by sleep deprivation and reduced efficiency at work, family issues, fatigue, stress, loss of interest, depersonalization, difficulty managing emotions, and physical and mental problems (3, 6, 31, 33, 34). Since burnout is both physical and psychological, people may experience different forms of burnout in their lives (31).
Erten and Özdemir (31) refined the concept of digital burnout by identifying three characteristics: digital aging, digital deprivation, and emotional exhaustion. Digital aging is one’s inability to strike a balance between the real world and the virtual world as a result of spending excessive time on digital platforms. Digital deprivation refers to the state in which one feels bad, physically or psychologically, when staying away from digital platforms. Emotional exhaustion refers to the depletion or draining of emotional resources (31, 34).
Excessive time spent online incurs a major risk of digital addiction and promotes digital exhaustion (43). Digital technologies lead individuals to experience a “fear of missing out,” and the anxiety it provokes is similar to that experienced with other addictions (33, 44). When this digital dependency is normalized and accepted, individuals experience a reduced ability to carry out activities that encourage them to disconnect from their digital devices (33). Consequently, digital burnout may occur (31). During these instances, individuals may experience a range of emotions and exhibit corresponding behaviors including tension, anxiety, dread, feelings of inadequacy, a sense of constriction, and difficulties concentrating (6). Therefore, it can be useful to consider digital addiction when analyzing digital burnout. This approach will help better understand and manage health and well-being in a digital environment (33).
Although digital tools offer practicality and convenience, their excessive use can act as a double-edged sword, contributing to digital burnout (3, 43). This phenomenon, as explained by demands–resources theory, suggests that burnout originates from an imbalance between work demands and available resources, with insufficient recovery exacerbating physical and mental exhaustion (41). When demands surpass resources, fatigue ensues, potentially leading to chronic fatigue and eventual burnout (41).
As the time spent using digital devices in daily life rapidly increases, university students find themselves continuously exposed to and utilizing these devices for their studies (16, 21, 45). While such use of digital devices can revolutionize the academic environment and enhance efficiency (22), it also heightens the risk of digital burnout (3, 34). Through online lectures, learning management systems, and online materials, students can easily access course materials, but this ease of access can also hinder their ability to concentrate on their studies (21). Factors such as technical issues and education quality from online lectures can cause stress among students regarding their academic performance (21). Additionally, students may allocate a significant amount of time to activities such as social networking, online video watching, and gaming (10, 11), which can reduce the time and energy they have available for their studies, ultimately leading to digital burnout (3, 34).
In conclusion, the continuous use of and exposure to digital devices in both academic and daily life settings can elevate the risk of digital burnout among university students (3). As such, the purpose of this study is to confirm the negative effects of digital use on individuals, including various internal and external stimuli, as well as emotional and cognitive overload. Specifically, we aim to evaluate the impact of digitalization on burnout and, consequently, the digital burnout experienced by Generation Z college students.
However, evaluations conducted in a specific context without considering the suitability of the measurement tools within that context may compromise the validity of the measurement and research results (28). The classical method for verifying a scale is factor analysis (46), and the DBS currently in use is based on the results of such an analysis confirming its validity among a population of 707 participants in Turkey (31). However, it needs to be determined whether the proposed factor structure in the initial population remains consistent among Generation Z university students in Korea to determine whether the DBS is a valid tool for measuring their level of digital burnout. Thus, the purpose of this study is to investigate the factor structure and to verify the validity and reliability of the Korean version of the DBS among Generation Z university students. Research on the validity of the Korean version of the DBS will contribute to establishing the concept of digital burnout and fostering a healthy digital culture in Korea.
This study was a methodological study with a cross-sectional design to investigate the factor structure in Korean Generation Z university students and verify the validity and reliability of the DBS. A cross-sectional design is an appropriate method for determining the prevalence of a disease, attribute, or phenomenon in a study sample, enabling investigators to describe their study sample and review associations between collected variables (47). It is effective to analyze each item when verifying the appropriateness of a tool, as the item is the most basic unit constituting that tool (48–50).
The participants in this study were first-, second-, third-, and fourth-year undergraduate students who resided in Korea, as well as individuals who had experience using digital devices (smartphones, tablets, wearables, etc.). Participants belonged to Generation Z, meaning they were born in or after 1995 (9, 10). Participants residing abroad or foreign nationals attending the universities were excluded. The survey was conducted online. A questionnaire was posted on various online communities frequently visited by Korean students to prepare for exams, find jobs, and learn languages. Detailed information on the study was provided on the first screen of the online survey. Subjects could only participate in the survey if they voluntarily agreed to do so. Filtering was set so that people who did not meet the inclusion criteria could not respond. Anonymity and confidentiality of the data were maintained to protect the rights of the research participants. Data were collected between July 26 and August 2, 2023, and participants were allowed to withdraw from the study at any time. All participants who completed the survey were given a small gift in return. To determine the validity of the tool, a sample size of at least five or 10 times the number of items is required (46). Since the original tool consisted of 24 items, a sample size of 240 was considered appropriate. However, considering that a minimum of 300 participants is suitable for confirmatory factor analysis (CFA) (51), and accounting for a 10% incomplete response rate, the required sample size was set at 330 participants. We collected 330 questionnaires, and the analysis was performed using the final dataset of 330 participants with no missing data.
The DBS was developed to measure burnout resulting from the use of digital devices (31). It consists of 24 items across 3 subscales, including content related to digital aging (12 items), digital deprivation (6 items), and emotional exhaustion (6 items). Each item in the DBS is measured on a 5-point Likert scale ranging from “strongly disagree” (1 point) to “entirely agree” (5 points). The total score ranges from 24 to 120, with high scores indicating high levels of digital burnout. During its development, this tool was validated with 286 high school students, 288 university students, and 133 graduates in Turkey, and its reliability was established based on Cronbach’s α = 0.94 (31). In this study, Cronbach’s α = 0.96.
The Korean version of the Digital Addiction Scale (K-DAS), developed by Kesici and Tunç (52) and adapted for Korean by Kim et al. (53), was used to verify concurrent validity. The K-DAS consists of 19 items measuring compulsive use (8 items) and the negative consequences thereof (11 items). Each item is scored on a 5-point Likert scale, with a total score ranging from 19 to 95. A higher total score indicates a higher level of digital addiction. In this study, the reliability was Cronbach’s α = 0.94.
The Korean version of the Maslach Burnout Inventory-Student Survey (MBI-SS), developed by Schaufeli et al. (27) and adapted for Korean by Lee and Lee (54), was used to verify concurrent validity. The Korean version of the MBI-SS consists of 14 items related to exhaustion (5 items), inefficacy (5 items, reverse-coded), and cynicism (4 items). Each item is rated on a 7-point Likert scale, with a total score ranging from 14 to 98. A higher total score indicates a higher level of academic burnout. In this study, the reliability was confirmed with Cronbach’s α = 0.87.
The Korean version of the digital burnout measurement tool was translated according to the World Health Organization guidelines (55). In the primary translation stage, two professional translators proficient in both English and Korean independently translated the measurement items from the original tool, which was written in English. Thereafter, in the expert panel stage, two nursing professors with experience in tool development and proficiency in both Korean and English reviewed the tool translated in the primary stage. During this stage, they confirmed and completed the draft items in Korean, ensured the translated items accurately represented the measurement concepts and had appropriate expressions, and addressed any items that required modification owing to cultural differences. Afterward, in the back-translation stage, a bilingual English-speaking professor was commissioned to translate the reviewed version. The questionnaire, completed through translation and back-translation, was constituted as a preliminary tool through discussions among a linguistics professor with experience in tool development, two nursing professors, and the back-translator. They confirmed and revised the discrepancies between the back-translated and original tools, expressions affected by cultural differences, and any distortions of meaning. Finally, after receiving grammatical corrections from a linguistics scholar specializing in Korean that did not alter the meaning, the Korean version of the preliminary tool was confirmed.
For the preliminary investigation, samples were selected using the same criteria as for the main investigation. Ten university students participated voluntarily and were instructed to complete a self-report questionnaire. The preliminary investigation aimed to identify confusing expressions or difficult questions, and measure response time. The results of the preliminary investigation indicated that the response time was approximately 7–10 min and that no expressions or questions posed difficulties for the respondents. Consequently, the final tool was completed without any modifications to the questions.
This study was conducted after obtaining approval from the Institutional Review Board (IRB No. MC23QASI0061) of the institution with which the researchers are affiliated to protect and respect the rights of research participants.
Data were analyzed using IBM SPSS Statistics 22 and Amos 18 software (IBM Corp., Armonk, NY, United States). IBM SPSS Amos is a powerful structural equation modeling software that enables researchers to support their studies and hypotheses by extending standard multivariate analysis methods including regression, factor analysis, correlation, and an analysis of variance. With SPSS Amos, one can build attitudinal and behavioral models that reflect complex relationships more accurately than with standard multivariate statistics techniques using either an intuitive graphical or programmatic user interface (56).
The CFA was conducted to assess construct validity, and principal component factor analysis using the Varimax method was employed for parameter estimation. Model fit was evaluated based on various fit indices including the absolute fit indices (χ2), χ2/degree of freedom, root mean square error of approximation (RMSEA), standardized root mean residual (SRMR), comparative fit index (CFI), and Tucker–Lewis index (TLI) (46, 56). As χ2 can be influenced by sample size or model complexity, fit indices other than χ2 (p > 0.05), including χ2/df ≤ 3, RMSEA ≤0.08, SRMR ≤0.08, CFI ≥ 0.90 and TLI ≥ 0.90, were considered to assess overall fit (46, 57–59).
Convergent and discriminant validity were assessed using standardized estimates (β), construct reliability, average variance extracted (AVE), and inter-factor correlation coefficients (46). Convergent validity of the items was confirmed through standardized factor loadings (β) ≥ 0.50, AVE ≥ 0.50, and CR ≥ 0.70. Discriminant validity was assessed based on the criterion of not including 1 within the range of r ± 2 × Standard Error (46). The normality of each item was evaluated based on absolute skewness values of less than 3 and absolute kurtosis values of less than 10 (57). Concurrent validity was assessed by assuming significant positive correlations between the Korean versions of the DBS and K-DAS, and between the Korean versions of the DBS and MBI-SS. Pearson’s correlation analysis was performed for this purpose. Finally, internal consistency reliability was verified using Cronbach’s α.
The mean age of participants was 21.27 ± 1.70 years, with 42.7% males and 57.3% females. The majority were second-year students (45.8%) and humanities majors (30.3%). The average daily usage of digital devices was 5.88 ± 2.54 h, and academic grade was predominantly categorized as “Middle” (69.4%), “Low” (17.0%), and “High” (13.6%). Devices were primarily used for social network services (SNS; 39.1%), instant messenger (26.7%), and video-sharing platforms (20.9%) (Table 1).
The mean score for each item ranged from 2.27 ± 1.24 to 3.36 ± 1.13. The skewness for each item ranged from −0.55 to 0.75, and kurtosis ranged from −1.16 to −0.40, which met the criteria. The correlation coefficients between each item and the overall items ranged from 0.54 to 0.81, indicating that each item contributed significantly to its respective subscale (Table 2).
The CFA results showed that χ2 = 804.94 (df = 249, p > 0.001), χ2/df = 3.23, RMSEA = 0.08, SRMR = 0.07, TLI = 0.89, and CFI = 0.90, indicating that some fit indices did not meet the criteria. Thus, adjustments were made to the model by sequentially using item paths within factors, starting with items with modification indices greater than 10, to avoid influencing the relationships between factors [(46, 58); Figure 1]. The adjusted model fit, excluding the sample size-sensitive χ2 = 732.53 (df = 246, p > 0.001), met the criteria with χ2/df = 2.98, RMSEA = 0.08, SRMR = 0.07, TLI = 0.90, and CFI = 0.91 (Table 3). The fit indices provided evidence confirming the three-factor Korean version of the DBS model.
Table 3. Factor structure of the Korean version of the DBS using confirmatory factor analysis (N = 330).
The results for convergent validity showed that the β values for all items ranged from 0.56 to 0.85. The AVE ranged from 0.56 to 0.58, and the CR from 0.88 to 0.94, satisfying all three criteria to verify convergent validity. Discriminant validity was confirmed as the range of r ± 2 × Standard Error was from 0.10 to 0.24, not including 1, which aligns with the criteria (Table 3).
Concurrent validity was analyzed in relation to digital addiction and academic burnout. Digital burnout showed significant positive correlations with digital addiction (r = 0.73, p < 0.001) and academic burnout (r = 0.66, p < 0.001), indicating concurrent validity (Table 4).
The Cronbach’s α for the Korean version of the DBS was 0.95. The internal consistency range for the three factors was between 0.88 and 0.94 (Table 2).
The purpose of this study was to investigate the factor structure of Korean Generation Z university students and to verify the validity and reliability of the DBS. The three-factor measurement model of the Korean DBS was deemed appropriate. These results are consistent with the measurement model developed by Erten and Özdemir (31) for Turkish high school students, university students, and graduates, indicating similarity with the original model.
The first factor, “digital aging,” consists of 12 items. Digital aging refers to the imbalance between the real and virtual worlds caused by excessive time spent on digital platforms (31). When digital activities negatively affect individuals’ abilities and energy, they induce mental and physical fatigue, resembling the aging process (3). Participants in this study spent 5.88 ± 2.54 h per day using digital devices. Increased exposure to digital devices and content may result in adverse non-specific symptoms and physiological issues. Previous studies indicated that using digital devices for >2 h can increase eye fatigue and lead to dry eye syndrome (28, 60).
The use of digital devices often involves sitting in a forward-leaning posture, which leads to constant changes in posture and muscle activity (29). This increases the mechanical load on the spine, causing muscle fatigue and potentially resulting in neck and back pain (29). In previous studies investigating musculoskeletal pain among university students, the use of digital devices was associated with frequent pain in the neck, shoulders, lower back, wrists, and hands (29, 61). Prolonged use of digital devices has also been linked to a reduction in overall physical activity (30). Several studies have indicated an elevated risk of obesity and higher body mass index among individuals who spend considerable time on computers or watching television (62, 63). In addition, using smartphones has been associated with decreased walking speed and impaired balance (64). A causal link has also been found between mental health symptoms and issues stemming from excessive digital use (33, 45). For this, studies have emphasized the elevated risk of emotional vulnerabilities associated with excessive digital device use, such as heightened depression, diminished self-esteem, and increased stress perception (3, 31). Consequently, prolonged and excessive use of digital devices can contribute to physical problems, encompassing musculoskeletal and ocular issues, as mentioned, regardless of age. The symptoms related to digital aging may accrue over time, underscoring the importance of prevention and early intervention.
The second factor, “digital deprivation,” consists of six items that measure how negatively individuals feel physically and psychologically when they distance themselves from digital platforms. In this regard, on a 5-point scale, the statement scoring the highest (3.17 points) was: “I am most afraid of losing or forgetting my phone. This thought bothers me.” This indicates that the high portability of digital devices can lead to obsessive use, resulting in negative outcomes (31, 53). This corresponds to nomophobia (5, 7), where individuals feel fear without their smartphone, emphasizing the contextual aspect.
Generation Z students have incorporated communication through social media and online platforms into their daily routines; it has become a natural habit that requires little conscious effort (10, 11). Their main use of digital platforms, such as SNS, revolves around communicating with friends, sustaining connections, or gaining additional information. However, the lack of digital devices can evoke feelings of anxiety and loss if communication via digital platforms is interrupted (9). Generation Z students receive education in a learning environment that utilizes digital technologies such as videos, simulations, online quizzes, case studies, and social media (16). Therefore, the effect of digital deprivation is presumed to be pronounced when they do not have access to digital devices. Given the overall digitization of living and learning environments, in which digital device usage is prevalent (6), appropriate use of digital devices provides convenience and familiarity in daily life. However, excessive usage can lead to mental and physical health problems (3, 31, 33, 34). Therefore, understanding an individual’s digital usage patterns and quantity is crucial. Furthermore, considering the potential decrease in real-world communication and the risk of social weakening and isolation associated with increased activity in the digital world (13, 14), it is essential to assess digital deprivation due to individual digital usage and to implement measures to minimize the negative impact on social relationships.
The third factor, “emotional exhaustion,” comprises six items measuring emotional fatigue resulting from the use of digital devices. Efforts to learn and apply new technologies can induce stress and lead to emotional exhaustion (65). Emotional exhaustion is a contemporary adaptation disorder resulting from an unhealthy coping mechanism involving the use of new technologies, often referred to as “Technostress” (45). It involves a broad spectrum of emotional stress experienced through using information systems (65). As students widely use mobile technology, social media, and various educational technologies (e.g., e-learning systems, online resources, PowerPoint slides, and podcasts), some are prone to experiencing high levels of technostress (21, 45). Consequently, the diverse use of digital devices can trigger emotional exhaustion, thereby depleting emotional resources and causing mental and physical fatigue. This depletion may result in overload, inefficient information processing, confusion, loss of control, psychological stress, increased depressive symptoms, and other personality changes and emotional issues (3, 6, 33). The interaction between emotional exhaustion and the use of digital devices is complex and can vary based on user characteristics, usage patterns, living environments, and other factors (31, 34).
The concurrent validity of the Korean version of the DBS was confirmed by analyzing its correlation with characteristics similar to those of the tool of this study, such as digital addiction and academic burnout. The results indicated a significant positive correlation. Thus, universities should provide education and support regarding academic burnout in the digital environment and ensure that students have access to appropriate support at all times. In a sustainable digital learning environment, students can overcome academic burnout and develop healthy study habits.
This study had three aims. The first was to evaluate the Korean version of the DBS, which comprises 24 items divided into 3 sub-factors, through the rigorous translation of the tool and verification of its validity and reliability. The Korean version of the DBS was theoretically and statistically proven to have high validity and reliability. The second aim was to contribute to understanding the concept of digital burnout through factor analysis. This clarification may contribute to the development of early detection and intervention programs in educational and clinical settings. The third aim was to measure individual levels of digital burnout in the era of digitalization, allowing for careful observation and awareness of university students’ digital usage patterns.
Students are constantly exposed to various digital devices, and without realizing it, may experience digital burnout from spending too much time thereon. However, these individuals may be unaware of their situation, making it challenging to find solutions. Early signs of mental and physical fatigue can be detected by identifying frequently used digital platforms, usage times, and emotional states, emphasizing the importance of assessing digital aging, digital deprivation, and emotional exhaustion. Following this study, subsequent diagnostic research is required to accurately evaluate and distinguish digital burnout among university students. Through such research, the level of risk of digital burnout can be accurately assessed and appropriate support and intervention provided.
Based on the results of this study, it is recommended that the characteristics of individuals based on sub-factors be identified to propose diverse and personalized strategies to promote healthy digital usage education. A program could be developed that encourages regular breaks from digital devices, which especially limits screen time before sleeping and promotes physical and mental rest through offline activities such as exercise. In addition, enhancing digital control through digital addiction programs and effective time management education can reduce digital deprivation. Finally, incorporating leisure activities such as meditation and yoga can alleviate emotional exhaustion and contribute to overall health and an improved quality of life.
The Korean version of the DBS has good reliability and validity and can be used as a tool to assess the level of risk of digital burnout and provide appropriate support and intervention.
Since data for the Korean version of the DBS were collected online, possibly, only individuals with high Internet accessibility participated. Moreover, the study focused on Generation Z university students, who are characterized by high digital usage, which limits the representativeness of the sample and generalizability of the results. In future, several considerations are necessary. First, an analysis of different age groups such as working professionals, middle-aged individuals, and the older adult is needed to reconfirm the factor structure of digital burnout based on age and occupational group. Second, using self-reported survey methods may lead to overestimation or underestimation of one’s behavior or habits. Therefore, in future research, the incorporation of objective data collection techniques such as vitality indicators or stress measurement technologies is essential. Third, this study employed a cross-sectional design, collecting data at a single point in time, which limited the ability to track the dynamic nature of digital burnout. Future research should employ a longitudinal study design to establish the causal relationship between digital usage and burnout, and to identify factors that trigger digital burnout.
The original contributions presented in the study are included in the article/supplementary material, further inquiries can be directed to the corresponding author.
S-YC: Conceptualization, Formal analysis, Methodology, Writing – original draft, Writing – review & editing. J-HK: Writing – original draft, Writing – review & editing, Conceptualization, Funding acquisition, Methodology.
The author(s) declare that financial support was received for the research, authorship, and/or publication of this article. This work was supported by a Korea Research Foundation grant funded by the Korean Government MOEHRD, Basic Research Promotion Fund [NRF-2021R1A2C2006327].
We are grateful to all participants who took part in the survey for this study.
The authors declare that the research was conducted in the absence of any commercial or financial relationships that could be construed as a potential conflict of interest.
All claims expressed in this article are solely those of the authors and do not necessarily represent those of their affiliated organizations, or those of the publisher, the editors and the reviewers. Any product that may be evaluated in this article, or claim that may be made by its manufacturer, is not guaranteed or endorsed by the publisher.
1. Lee, HK, Lim, LH, Lee, JJ, Shin, YM, Lee, SY, and Jung, SH. Development of preventive intervention recommendation for health problem related to excessive use of digital contents/devices. Seoul, Korea: Korean Medical Association (2020).
2. Tülübaş, T, Karakose, T, and Papadakis, S. A holistic investigation of the relationship between digital addiction and academic achievement among students. Eur J Investig Health Psychol Educ. (2023) 13:2006–34. doi: 10.3390/ejihpe13100143
3. Göldağ, B . An investigation of the relationship between university students’ digital burnout levels and perceived stress levels. J Learn Teach Digit Age. (2022) 7:90–8. doi: 10.53850/joltida.958039
4. Hoehe, MR, and Thibaut, F. Going digital: how technology use may influence human brains and behavior. Dialog Clin Neurosci. (2022) 22:93–7. doi: 10.31887/DCNS.2020.22.2/mhoehe
5. Daraj, LR, AlGhareeb, M, Almutawa, YM, Trabelsi, K, and Jahrami, H. Systematic review and meta-analysis of the correlation coefficients between nomophobia and anxiety, smartphone addiction, and insomnia symptoms. Healthcare. (2023) 11:2066. doi: 10.3390/healthcare11142066
6. Karataşlioğlu, RE, and Özkanal, B. Digital burnout in distance education process: investigation of secondary school teachers’ opinions. J Adv Educ Stud. (2023) 5:319–41. doi: 10.48166/ejaes.1353517
7. Hasmawati, F, Samiha, YT, Razzaq, A, and Anshari, M. Understanding nomophobia among digital natives: characteristics and challenges. J Crit Rev. (2020) 7:122. doi: 10.31838/jcr.07.13.22
8. Shorey, S, Chan, V, Rajendran, P, and Ang, E. Learning styles, preferences and needs of generation Z healthcare students: scoping review. Nurse Educ Pract. (2021) 57:103247. doi: 10.1016/j.nepr.2021.103247
9. Liu, H, Liu, W, Yoganathan, V, and Osburg, V-S. COVID-19 information overload and generation Z’s social media discontinuance intention during the pandemic lockdown. Technol Forecast Soc Chang. (2021) 166:120600. doi: 10.1016/j.techfore.2021.120600
10. Cervi, L . Tik Tok and generation Z. Theatre, Dance Perform Train. (2021) 12:198–204. doi: 10.1080/19443927.2021.1915617
11. Mason, MC, Zamparo, G, Marini, A, and Ameen, N. Glued to your phone? Generation Z’s smartphone addiction and online compulsive buying. Comput Hum Behav. (2022) 136:107404. doi: 10.1016/j.chb.2022.107404
12. Bojic, L . Metaverse through the prism of power and addiction: what will happen when the virtual world becomes more attractive than reality? Eur J Futures Res. (2022) 10:22. doi: 10.1186/s40309-022-00208-4
13. Schlosser, AE . Self-disclosure versus self-presentation on social media. Curr Opin Psychol. (2020) 31:1–6. doi: 10.1016/j.copsyc.2019.06.025
14. Michikyan, M . Linking online self-presentation to identity coherence, identity confusion, and social anxiety in emerging adulthood. Br J Dev Psychol. (2020) 38:543–65. doi: 10.1111/bjdp.12337
15. Maslach, C, Schaufeli, WB, and Leiter, MP. Job burnout. Annu Rev Psychol. (2001) 52:397–422. doi: 10.1146/annurev.psych.52.1.397
16. Szymkowiak, A, Melović, B, Dabić, M, Jeganathan, K, and Kundi, GS. Information technology and gen Z: the role of teachers, the internet, and technology in the education of young people. Technol Soc. (2021) 65:101565. doi: 10.1016/j.techsoc.2021.101565
17. Priporas, CV, Hussain, S, Khaneja, S, and Rahman, H. Technology distraction in generation Z: the effects on consumer responses, sensory overload, and discomfort. Int J Inf Manag. (2024) 75:102751. doi: 10.1016/j.ijinfomgt.2023.102751
18. Qanash, S, Al-Husayni, F, Falata, H, Halawani, O, Jahra, E, Murshed, B, et al. Effect of electronic device addiction on sleep quality and academic performance among health care students: cross-sectional study. JMIR Med Educ. (2021) 7:e25662. doi: 10.2196/25662
19. Rathakrishnan, B, Bikar Singh, SS, Kamaluddin, MR, Yahaya, A, Mohd Nasir, MA, Ibrahim, F, et al. Smartphone addiction and sleep quality on academic performance of university students: an exploratory research. Int J Environ Res Public Health. (2021) 18:8291. doi: 10.3390/ijerph18168291
20. Dontre, AJ . The influence of technology on academic distraction: a review. Hum Behav Emerg Technol. (2021) 3:379–90. doi: 10.1002/hbe2.229
21. Song, W, Wang, Z, and Zhang, R. Classroom digital teaching and college students’ academic burnout in the post COVID-19 era: a cross-sectional study. Int J Environ Res Public Health. (2022) 19:13403. doi: 10.3390/ijerph192013403
22. Mädamürk, K, Tuominen, H, Hietajärvi, L, and Salmela-Aro, K. Adolescent students’ digital engagement and achievement goal orientation profiles. Comput Educ. (2021) 161:104058. doi: 10.1016/j.compedu.2020.104058
23. Gundogan, S . The relationship of COVID-19 student stress with school burnout, depression and subjective well-being: adaptation of the COVID-19 student stress scale into Turkish. Asia Pac Educ Res. (2023) 32:165–76. doi: 10.1007/s40299-021-00641-2
24. Hao, Z, Jin, L, Huang, J, and Wu, H. Stress, academic burnout, smartphone use types and problematic smartphone use: the moderation effects of resilience. J Psychiatr Res. (2022) 150:324–31. doi: 10.1016/j.jpsychires.2022.03.019
25. Hao, Z, Jin, L, Huang, J, Lyu, R, and Cui, Q. Academic burnout and problematic smartphone use during the Covid-19 pandemic: the effects of anxiety and resilience. Front Psych. (2021) 12:725740. doi: 10.3389/fpsyt.2021.725740
26. Kilic, R, Nasello, JA, Melchior, V, and Triffaux, J-M. Academic burnout among medical students: respective importance of risk and protective factors. Public Health. (2021) 198:187–95. doi: 10.1016/j.puhe.2021.07.025
27. Schaufeli, WB, Martínez, IM, Pinto, AM, Salanova, M, and Bakker, AB. Burnout and engagement in university students: a cross-national study. J Cross-Cult Psychol. (2002) 33:464–81. doi: 10.1177/0022022102033005003
28. Al-Marri, K, Al-Qashoti, M, Al-Zoqari, H, Elshaikh, U, Naqadan, A, Saeed, R, et al. The relationship between smartphone use and dry eye disease: a systematic review with a narrative synthesis. Medicine. (2021) 100:e27311. doi: 10.1097/MD.0000000000027311
29. Mustafaoglu, R, Yasaci, Z, Zirek, E, Griffiths, MD, and Ozdincler, AR. The relationship between smartphone addiction and musculoskeletal pain prevalence among young population: a cross-sectional study. Korean J Pain. (2021) 34:72–81. doi: 10.3344/kjp.2021.34.1.72
30. Grimaldi-Puyana, M, Fernández-Batanero, JM, Fennell, C, and Sañudo, B. Associations of objectively-assessed smartphone use with physical activity, sedentary behavior, mood, and sleep quality in young adults: a cross-sectional study. Int J Environ Res Public Health. (2020) 17:3499. doi: 10.3390/ijerph17103499
31. Erten, P, and Özdemir, O. The digital burnout scale. İnönü Üniv Eğitim Fakültesi Dergisi. (2020) 21:668–83. doi: 10.17679/inuefd.597890
32. Sá, MJ, Santos, AI, Serpa, S, and Miguel, FC. Digitainability—digital competences post-COVID-19 for a sustainable society. Sustain For. (2021) 13:9564. doi: 10.3390/su13179564
33. da Silva, FP, Jerónimo, HM, Henriques, PL, and Ribeiro, J. Impact of digital burnout on the use of digital consumer platforms. Technol Forecast Soc Chang. (2024) 200:123172. doi: 10.1016/j.techfore.2023.123172
34. Durmuş, SÇ, Gülnar, E, and Özveren, H. Determining digital burnout in nursing students: a descriptive research study. Nurse Educ Today. (2022) 111:105300. doi: 10.1016/j.nedt.2022.105300
35. Oja, LA . Unplugging for inner peace: fostering digital wellness in an overconnected world. J Hosp Librariansh. (2023) 23:284–90. doi: 10.1080/15323269.2023.2253132
36. Yang, X, and Du, J. The effect of teacher self-efficacy, online pedagogical and content knowledge, and emotion regulation on teacher digital burnout: a mediation model. BMC Psychol. (2024) 12:51. doi: 10.1186/s40359-024-01540-z
37. World Health Organization . Burn-out an “occupational phenomenon”: international classification of diseases; (2019). Available at: https://www.who.int/news/item/28-05-2019-burn-out-an-occupational-phenomenon-international-classification-of-diseases (accessed May 18, 2024).
39. Maslach, C, Jackson, SE, and Leiter, MP. Maslach burnout inventory. Lanham: Scarecrow Education (1997).
40. Maslach, C, and Jackson, SE. The measurement of experienced burnout. J Organ Behav. (1981) 2:99–113. doi: 10.1002/job.4030020205
41. Edú-Valsania, S, Laguía, A, and Moriano, JA. Burnout: a review of theory and measurement. Int J Environ Res Public Health. (2022) 19:1780. doi: 10.3390/ijerph19031780
42. Zis, P, Artemiadis, A, Bargiotas, P, Nteveros, A, and Hadjigeorgiou, GM. Medical studies during the COVID-19 pandemic: the impact of digital learning on medical students’ burnout and mental health. Int J Environ Res Public Health. (2021) 18:349. doi: 10.3390/ijerph18010349
43. Coyne, P, and Woodruff, SJ. Taking a break: the effects of partaking in a two-week social media digital detox on problematic smartphone and social media use, and other health-related outcomes among young adults. Behav Sci. (2023) 13:1004. doi: 10.3390/bs13121004
44. Brown, L, and Kuss, DJ. Fear of missing out, mental wellbeing, and social connectedness: a seven-day social media abstinence trial. Int J Environ Res Public Health. (2020) 17:4566. doi: 10.3390/ijerph17124566
45. Vallone, F, Galvin, J, Cattaneo Della Volta, MF, Akhtar, A, Chua, S, Ghio, E, et al. Technostress and academic motivation: direct and indirect effects on university students’ psychological health. Front Psychol. (2023) 14:1211134. doi: 10.3389/fpsyg.2023.1211134
46. JP Y . The concept and understanding of structural equation modeling. Rev. ed. Seoul: Hannare Publishing (2022).
47. Wang, X, and Cheng, Z. Cross-sectional studies: strengths, weaknesses, and recommendations. Chest. (2020) 158:S65–71. doi: 10.1016/j.chest.2020.03.012
48. Gómez-Salgado, J, Allande-Cussó, R, Rodríguez-Domínguez, C, Domínguez-Salas, S, Camacho-Martín, S, Romero Ruiz, A, et al. Development and criterion validity of the COVID-19 anxiety and fear assessment scale: a cross sectional study. Sci Prog. (2021) 104:00368504211050291. doi: 10.1177/00368504211050291
49. Lorini, C, Velasco, V, Bonaccorsi, G, Dadaczynski, K, Okan, O, Zanobini, P, et al. Validation of the COVID-19 digital health literacy instrument in the Italian language: a cross-sectional study of Italian university students. Int J Environ Res Public Health. (2022) 19:6247. doi: 10.3390/ijerph19106247
50. Kakemam, E, Navvabi, E, Albelbeisi, AH, Saeedikia, F, Rouhi, A, and Majidi, S. Psychometric properties of the Persian version of depression anxiety stress Scale-21 items (DASS-21) in a sample of health professionals: a cross-sectional study. BMC Health Serv Res. (2022) 22:111. doi: 10.1186/s12913-022-07514-4
51. Devellis, RF, and Thorpe, CT. Scale development: theory and applications. Thousand Oaks (CA): Sage Publications (2021).
52. Kesici, A, and Tunç, NF. The development of the digital addiction scale for the university students: reliability and validity study. Univ J Educ Res. (2018) 6:91–8. doi: 10.13189/ujer.2018.060108
53. Kim, Y, Seo, Y, Yoon, S, Lee, H, and Choi, E. Exploratory factor analysis of the Korean version of the digital addiction scale. Health Soc Welfare Rev. (2021) 41:39. doi: 10.15709/hswr.2021.41.3.39
54. Lee, SH, and Lee, DY. Validation of the MBI-SS scales – based on medical school students. Asian J Educ. (2013) 14:165. doi: 10.15753/aje.2013.14.2.007
55. World Health Organization . Process of translation and adaptation of instruments Geneva (2018). Available at: https://www.coursehero.com/file/30372721/WHO-Process-of-translation-and-adaptation-of-instrumentspdf/ (accessed May 08, 2024).
56. Dash, G, and Paul, J. CB-SEM vs. PLS-SEM methods for research in social sciences and technology forecasting. Technol Forecast Soc Chang. (2021) 173:121092. doi: 10.1016/j.techfore.2021.121092
57. Kline, RB . Principles and practice of structural equation modeling. New York: Guilford Press (2023).
58. McDonald, RP, and Ho, M-HR. Principles and practice in reporting structural equation analyses. Psychol Methods. (2002) 7:64–82. doi: 10.1037/1082-989X.7.1.64
60. Alabdulkader, B . Effect of digital device use during COVID-19 on digital eye strain. Clin Exp Optom. (2021) 104:698–704. doi: 10.1080/08164622.2021.1878843
61. Walankar, PP, Kemkar, M, Govekar, A, and Dhanwada, A. Musculoskeletal pain and risk factors associated with smartphone use in university students. Indian J Occup Environ Med. (2021) 25:220–4. doi: 10.4103/ijoem.ijoem_351_20
62. Alghadir, AH, Iqbal, ZA, and Gabr, SA. The relationships of watching television, computer use, physical activity, and food preferences to body mass index: gender and nativity differences among adolescents in Saudi Arabia. Int J Environ Res Public Health. (2021) 18:9915. doi: 10.3390/ijerph18189915
63. Gülü, M, Yagin, FH, Gocer, I, Yapici, H, Ayyildiz, E, Clemente, FM, et al. Exploring obesity, physical activity, and digital game addiction levels among adolescents: a study on machine learning-based prediction of digital game addiction. Front Psychol. (2023) 14:1097145. doi: 10.3389/fpsyg.2023.1097145
64. Mourra, GN, Sénécal, S, Fredette, M, Lepore, F, Faubert, J, Bellavance, F, et al. Using a smartphone while walking: the cost of smartphone-addiction proneness. Addict Behav. (2020) 106:106346. doi: 10.1016/j.addbeh.2020.106346
Keywords: digital burnout, digital aging, digital deprivation, emotional exhaustion, university students
Citation: Choi S-Y and Kim J-H (2024) Validity and reliability of the Korean version of the Digital Burnout Scale. Front. Public Health. 12:1386394. doi: 10.3389/fpubh.2024.1386394
Received: 15 February 2024; Accepted: 23 May 2024;
Published: 03 June 2024.
Edited by:
Monika Fleischhauer, Medical School Berlin, GermanyReviewed by:
Rizwan Raheem Ahmed, Indus University, PakistanCopyright © 2024 Choi and Kim. This is an open-access article distributed under the terms of the Creative Commons Attribution License (CC BY). The use, distribution or reproduction in other forums is permitted, provided the original author(s) and the copyright owner(s) are credited and that the original publication in this journal is cited, in accordance with accepted academic practice. No use, distribution or reproduction is permitted which does not comply with these terms.
*Correspondence: Jung-Hee Kim, amhlZTkwQGNhdGhvbGljLmFjLmty
Disclaimer: All claims expressed in this article are solely those of the authors and do not necessarily represent those of their affiliated organizations, or those of the publisher, the editors and the reviewers. Any product that may be evaluated in this article or claim that may be made by its manufacturer is not guaranteed or endorsed by the publisher.
Research integrity at Frontiers
Learn more about the work of our research integrity team to safeguard the quality of each article we publish.