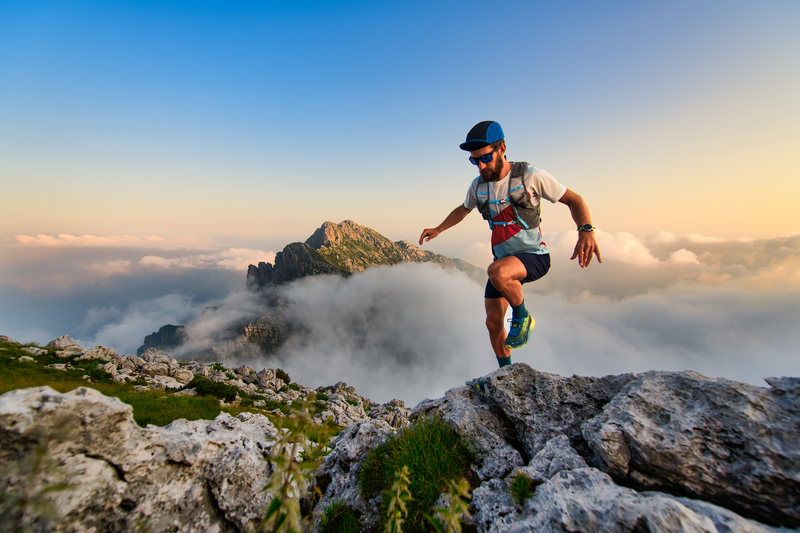
94% of researchers rate our articles as excellent or good
Learn more about the work of our research integrity team to safeguard the quality of each article we publish.
Find out more
CLINICAL TRIAL article
Front. Public Health , 12 August 2024
Sec. Children and Health
Volume 12 - 2024 | https://doi.org/10.3389/fpubh.2024.1380621
This article is part of the Research Topic Motivation for Physical Activity, volume II View all 11 articles
Introduction: The use of mobile apps to promote physical activity in adolescents can improve health-related parameters. However, previous studies have not evaluated whether the benefits depend on the users’ prior active or inactive status. Therefore, the main objective was to analyze differences in physical activity levels, adherence to the Mediterranean diet (AMD), anthropometry, body composition, and physical fitness between active and inactive adolescents.
Methods: The study was conducted through a randomized controlled trial (RCT) with 462 adolescents, divided into experimental (EG) and control groups (CG), further categorized as active and inactive. Variables of physical activity, kinanthropometry, body composition, and physical fitness were measured before (pre-test) and after (post-test) a 10-week intervention using step-tracking apps (Strava, Pacer, MapMyWalk, and PokémonGo) at least three times per week.
Results: The results showed that inactive EG adolescents significantly increased their physical activity levels, body mass, and muscle mass, and improved in all fitness variables except the countermovement jump (CMJ). The sum of three skinfolds also significantly decreased. Active EG adolescents increased body and muscle mass and improved in all fitness variables. Additionally, they significantly reduced fat mass and the sum of three skinfolds. All covariates, mainly gender and maturity, had significant effects on the study variables. Comparing changes between the active EG and CG groups, significant differences were found in body mass index (BMI) and CMJ in favor of the EG. However, while significant differences were observed in the study variables when analyzing each app individually, there were no differences between the changes produced by each app in these variables.
Conclusion: After a 10-week program of physical activity promoted through step-tracking apps, improvements were observed in fat variables, cardiorespiratory fitness, and curl-up performance. Furthermore, only inactive adolescents perceived an increase in their level of physical activity. The measurement protocol was registered prior to the start of the intervention at ClinicalTrials.gov (code: NCT04860128).
Physical inactivity, is a concept that tends to be confused with sedentary lifestyle (1). This term is defined as the failure to meet the minimum guidelines on physical activity practice established by organizations such as the World Health Organization (WHO) or the American College of Sports Medicine (ACSM) (2). Although the scientific literature on the health benefits of physical activity is numerous, physical inactivity has grown exponentially in recent decades in the world population (3, 4). Thus, a high percentage of the world’s population is currently considered inactive, with women accounting for a large part of this percentage (5, 6). As a consequence of the above, physical inactivity is currently the fourth leading cause of death in the world (2). In addition, is also one of the most determining factors in the prevalence of chronic diseases such as obesity, hypertension, diabetes, or metabolic syndrome, becoming one of the major public health problems (5, 7–9). Not surprisingly, it is estimated that given the consequences of physical inactivity on health, this factor generates a health expenditure of around 53.8 million euros/dollars worldwide (10).
This situation is especially relevant during adolescence, as it is a crucial stage for the establishment of physical activity, along with other healthy behaviors, preventing the onset of chronic diseases (7–9, 11). The adolescent population has been greatly affected by physical inactivity in recent years (12). In addition, puberty has become one of the critical stages in which a higher percentage of adolescents drop out of physical activity (13). The COVID-19 pandemic greatly contributed to this, as it drastically affected the period of movement of this population (14). The main consequences of the pandemic were the decrease in physical activity and the increase of screen time, resulting in an increase in physical inactivity and sedentary behaviors in adolescents (13, 15, 16). Although the confinement eventually ended, some of the unhealthy habits acquired by adolescents during the pandemic seem to persist (17). Thus, the pre-pandemic levels of physical activity have not been recovered in this population (18).
As a consequence of the above, 80% of adolescents currently do not meet the minimum physical activity recommendations established by the WHO (6). These recommendations include 60 min of moderate to vigorous intensity physical activity per day to promote physical fitness, a healthy anthropometry and body composition, and a greater adherence to the Mediterranean diet (AMD) (6, 19, 20). For children and adolescents, these recommendations can be undertaken as part of leisure and recreational activities, physical education, transport (cycling, walking and hiking) or household chores (21). Regarding transport, previous research has shown that walking between 11,500 and 14,000 steps per day is the optimal measure that corresponds to the recommendations for moderate to vigorous intensity physical activity (22). In addition, walking becomes relevant in this population because has shown that programs of 8 weeks or more can improve cardiorespiratory fitness, adherence to diet pattern and body mass index (BMI) in adolescents (21, 23, 24). This is because walking has been shown to increase physiological activity and the process of energy expenditure, with potential long-term weight control (25, 26).
Even with the wide variety of possibilities, the minimum levels of physical activity are not reached in the adolescent population, which is even more worrying for females and older adolescents, as this inactivity could affect their health status (27, 28). In this context, the subject of physical education during the compulsory education stage can contribute toward the acquisition of healthy lifestyle habits and the promotion of physical activity in the adolescent population (29–31). However, in Spain the educational curriculum only considers 2 h of physical education per week, which is insufficient to increase the time of motor engagement and comply with WHO recommendations (32, 33). For this reason, previous research has promoted the practice of school and out-of-school physical activity outside physical education hours. In this regard, active recess, physical activity programs in out-of-school leisure time, and active travel to school programs stand out (34–36). All of them have been shown to be effective strategies to increase the daily time dedicated to moderate to vigorous intensity physical activities in the adolescent population, allowing for a reduction in the rate of obesity (37, 38).
In recent years, the inclusion of mobile step-tracking apps has become a new trend in the interventions used to promote the practice of physical activity among adolescents (39, 40). These studies are characterized by two aspects: (a) the use of step tracker app through mobile phones in the classroom (41, 42); and (b) the use of these step tracker apps outside school hours (43). Regarding the former, the results have not been very encouraging, as they have shown the disadvantage that the step tracker mobile apps used are not designed for use in an educational center, limiting the possible benefits (44). Regarding the second aspect, it has shown significant benefits on the level of physical activity, physical fitness, kinanthropometry and body composition of adolescents, mainly when its use is promoted by the physical education subject (45, 46).
The usefulness of mobile apps for the promotion of physical activity in the adolescent population lies in the following reasons: (a) mobile phones are fully integrated in the lives of adolescents (47) and apps have proven to be useful for the generation of a healthy habit in which the mobile phone is a fundamental part (46); (b) one of the main reasons for the abandonment of physical activity programs is the lack of motivation of the participants (48), as many of them do not perceive themselves as sufficiently competent or autonomous during sport activities (49), so apps can solve this situation by enabling adolescents to practice autonomously and in activities of their own choice (50); (c) the possibility of interacting with other users through the app is another reason for using it (50), as the relationship with others is one of the main reasons for this population to practice physical activity (51); and (d) the use of the app promoted by the physical education subject in schools allows adolescents who take the intervention to obtain an academic reward, which provides greater motivation to continue using it due to the increase in external regulation (52), avoiding the initial abandonment of the intervention. In this way, mobile apps for the promotion of physical activity in adolescents could fulfill the key aspects of the theory of self-determination (53) by developing in adolescents a feeling of competent, autonomous and social relations, which could encourage adolescents to make more consistent use of these apps than other types of interventions that do not promote these three issues.
Despite the benefits found with the use of mobile applications promoted from the subject of physical education, this area of research has been under explored, and there are still many unknowns to be resolved (40, 45). Little is known about whether the previous level of physical activity of adolescents could be a determining factor in the use of step tracker mobile apps. This is because previous studies have shown differences in the effectiveness of physical activity intervention depending on the previous physical activity level of the adolescents (54). In this respect, a lower level of physical activity at the beginning of the intervention program, commonly measured by questionnaires recording physical activity in the last week, is related to higher effectiveness of intervention in adolescents (54–56). Only one previous study used step tracker mobile apps with inactive adolescents between 12 and 15 years of age, obtaining improvements in their cardiorespiratory fitness and decreasing their BMI (57). However, this research did not include a group of initially active adolescents to compare whether the benefits were truly superior in the initially inactive group.
Furthermore, although it has been found that the benefit of using this type of mobile application in physical education may be higher among females, and that it may depend on the number of steps taken, no studies have analyzed whether these factors may also differentiate between active and inactive students (58). In addition, one aspect that has not been considered in research conducted with mobile applications in adolescents is the effect that the maturational process may have on the changes observed. Thus, the duration of the interventions with mobile apps is not excessively long, varying between eight and 12 weeks, which leads to consider that the maturational process plays a fundamental role in the changes found in the adolescents, but there is no scientific evidence to contrast this (59, 60). On the other hand, it has been observed that the use of different mobile applications can lead to small changes in the different study variables. However, it is not known whether this could be affected by the level of physical activity of the adolescents who use them (61). Therefore, the objectives of the present research were: (a) to analyze the differences in the level of physical activity, AMD, anthropometry, body composition, and physical fitness of active and inactive adolescents achieved by a 10-week program of after-school use of step tracker mobile apps promoted from the physical education subject; and (b) to determine the influence of gender, maturity, the app used and the number of steps taken with the mobile apps on the study variables.
According to the aims of the present research, it is expected that the use of mobile step-tracking apps will be more effective on the study variables in inactive adolescents, since their starting level of physical activity is lower than that of active ones (H1); and that gender, maturity, app used and the number of steps taken with the mobile apps will influence the changes produced in the study variables after the use of the mobile apps (H2).
A randomized controlled trial (RCT) was conducted, consisting of a 10-week intervention in which step tracker mobile apps were used to promote physical activity in the adolescent population. An experimental group (EG) and a control group (CG) were used in the research. With the pre-test measurement of physical activity level, the EG and CG adolescents were divided into active and inactive according to the cut-off values of the “Physical Activity Questionnaire for Adolescents” (PAQ-A) (55). Participants were measured before starting the intervention (pre-test) and after finishing the intervention (post-test). In each measurement, the level of physical activity, kinanthropometric and body composition variables, AMD, and physical fitness were assessed. EG active and inactive participants used one of the step tracker mobile apps selected for the research (Strava, Pacer, MapMyWalk, and PokémonGo). The adolescents used the apps for 10 weeks, a minimum of three times per week, covering the proposed target distance for each week, which was incremental as the intervention progressed. The CG active and inactive adolescents did not use any step tracker mobile apps and continued to attend physical education classes as usual.
The study design followed the principles of the Declaration of Helsinki and was previously approved by the institutional committee of the Catholic University of Murcia (code: CE022102). In addition, the measurement protocol was registered prior to the start of the intervention at ClinicalTrials.gov (code: NCT04860128), and complied with the Consolidated Standards of Reporting Trials (CONSORT) guidelines (62).
The sampling was non-probabilistic by convenience. The intervention took place in two public centers of compulsory secondary education in the Region of Murcia. These centers had a high number of students enrolled in compulsory secondary education (more than 200 students per center). For their selection, the compulsory secondary school in the Region of Murcia with the largest sample of adolescents was contacted and, if the selected school did not wish to participate, the next public school with the largest number of students in compulsory secondary education would be chosen. However, the two centers initially contacted agreed to participate in the research. Once both centers were selected, a meeting was held with each management team to inform them of the research objectives and procedures. Once approval was received, a meeting was held with the teachers in charge of physical education and, subsequently, with the students and their parents. Participation was completely voluntary and the adolescents who were interested provided informed consent signed by themselves and their parents authorizing data collection and subsequent publication of the anonymous data.
To calculate the sample size, we used the methodology from previous studies based on the standard deviation (SD) (63). Thus, the SD of previous studies in which physical activity practice was promoted by means of step tracker mobile apps (SD = 0.66) was used (64). For an estimated error (d) of 0.06 and a confidence interval of 95% (95% CI), the minimum sample needed for conducting the study was 380 adolescents.
A total of 925 adolescents were potentially eligible between the two schools. The initial participation was 462 adolescents, with 430 adolescents participating in the research at the end (270 EG; 160 CG). The number of adolescents who were active and inactive at the beginning of the study was similar (209 active and 221 inactive). The participation of males and females was homogeneous (225 males and 205 females). The age of the adolescents was between 12 and 16 years (mean age: 13.76 ± 1.41 years). The sample selection flow diagram is shown in Figure 1.
The inclusion criteria were (a) age between 12 and 16 years old; (b) not presenting any disease or pathology that would hinder participation; and (c) attending compulsory secondary education. The exclusion criteria were (a) not regularly attending physical education classes during the school year (compulsory for more than 80% of the sessions); (b) not having a cell phone, among the adolescents in the EG; (c) changing educational center; (d) starting some type of systematic physical activity, understood as an activity performed more than three times a week for a duration of more than 60 min a day (such as a specific sport or going to the gym), but without considering the fact of starting to walk, since this was one of the objectives of the research; (e) abandoning the physical activities that were performed regularly before the beginning of the research; and (f) using one of the step tracker mobile apps designated for the research prior to the start of the research. Starting or abadoning any type of systematic physical activity was asked to adolescents in the post-test, together with the PAQ-A questionnaire. A specific question was included after the PAQ-A specifying what was meant by systematic physical activity, which adolescents were asked to answer dichotomously (yes or no).
The randomization process was carried out by the principal investigator in the presence of other investigators not involved in the study, using a computer-generated random number table. For this purpose, the adolescents from each of the schools were randomized into EG and CG, so that in both schools there were adolescents from both groups, thus avoiding that the teaching plans proposed in each school in the physical education subject could contaminate the data. In addition, randomization was done by class, so that all adolescents belonging to that class became part of the CG or one of the EG groups. A second randomization was carried out in the EG to establish the step tracker mobile apps to be used by each of the classes (Strava, Pacer, MapMyWalk, PokémonGo). Four classes were randomly assigned to each application, achieving a homogeneous number of adolescents per step tracker mobile apps at the beginning of the intervention (Figure 1).
Pre-test measurements were performed after randomization. The researchers involved in the measurements were blinded as to which group each adolescent belonged to. They also did not know whether they were active or inactive at baseline, as this was determined after measurements for both EG and CG. In the post-test measurements, the researchers were blinded to whether the adolescents belonged to the EG or CG, to whether they were active or inactive before the start of the investigation, and to the score obtained by each adolescent in the pre-test measurements. The researchers in charge of monitoring the EG intervention were also blinded to the scores obtained by the adolescents in the measurements.
The tests and instruments included are justified on the basis that previous research with mobile applications has shown significant changes in these variables (58, 61). However, the present study aims to determine whether there are differences in the effectiveness of the intervention in active and inactive adolescents. In addition, the inclusion of covariates in the present study will allow to determine whether gender, maturity status, app used and distance traveled with the app significantly influence the results, or whether the changes are the result of the intervention.
The “Physical Activity Questionnaire for Adolescents” (PAQ-A) was used to assess the level of physical activity in the adolescent population (65). This questionnaire is composed of 9 items. The arithmetic mean of the first eight items provides the final physical activity score. The ninth item allows to know if the adolescents had had any difficulty in performing physical activity during the week prior to completing the questionnaire. This questionnaire was used to determine active and inactive adolescents. Thus, adolescents were considered to be active when they scored 2.75 or higher on the PAQ-A, while they were considered inactive when they scored below 2.75 (55). This questionnaire was previously validated in an adolescent population and presents an intraclass correlation coefficient of 0.71 for the final score of the questionnaire (66).
AMD was assessed using the Mediterranean Diet Quality Index (KIDMED). This questionnaire is composed of 16 items that are completed with a 1 or 0 depending on whether the statement indicated is true (1) or not (0). Of the 16 items, 12 have a positive connotation (+1), favoring a good AMD, while four have a negative connotation (−1), hindering a good AMD. For this reason, the final score of the questionnaire ranges from 0 to 12 points, with a higher score referring to a better AMD. This questionnaire presents adequate reliability and reproducibility for use in the adolescent population (α = 0.79 and kappa: 0.66) (67).
Adolescent body composition and anthropometry were measured by anthropometrists accredited by the International Society for the Advancement of Kinanthropometry (ISAK) (levels 3 to 4). The measurement consisted of body mass and height; triceps, thigh and leg skinfolds; and relaxed arm, waist, mid-thigh and leg girths (68). Kinanthropometric measurements were used to calculate the following variables: BMI, muscle mass, fat mass, sum of 3 skinfolds (triceps, thigh, and leg), corrected arm, thigh and calf girths, and waist-to-height ratio (waist girth/height) (69, 70).
All the measurements were performed following the protocol established by ISAK (68). A skinfold caliper (Harpenden, Burgess Hill, United Kingdom) with an accuracy of 0.2 mm was used to measure skinfolds; a Lufkin W606PM inextensible tape (Lufkin Industries) with an accuracy of 0.1 cm was used to measure girths; a TANITA BC 418-MA segmental scale (TANITA, Tokyo, Japan) with an accuracy of 100 g was used to measure body mass; and a SECA 213 stadiometer (SECA, Hamburg) with an accuracy of 0.1 cm was used to measure height. All instruments were calibrated prior to the pre- and post-test measurements. In the pre and post measurements, each anthropometrist measured the same subjects to minimize inter-rater error. Intra- and inter-evaluator technical measurement error (TME) was calculated in a subsample. For the basic measurements, the intra- and inter-evaluator TME was 0.03 and 0.05%, respectively; 1.23 and 1.99% for skinfolds, respectively; and 0.03 and 0.05% for perimeters, respectively.
The maturity offset was calculated according to the procedure established by Mirwald et al. and using gender-specific formulas: −9.37 + 0.0001882 × ((height-sitting height) × sitting height) − 0.0022 × (age × (height − sitting height)) + 0.005841 × (age × sitting height) − 0.002658 × (age × weight) + 0.07693 × (weight/height) (71). The result of the maturity offset equation is expressed in years from the age at peak height velocity (APHV) when the result is positive, and in years to the APHV when the result is negative (71).
Five researchers with previous experience in the supervision of physical fitness testing were in charge of the pre and post measurements. To avoid inter-rater bias, each test was assigned to a researcher and all adolescents were familiarized with the test prior to measurement.
The 20-m shuttle run test was used to assess the cardiorespiratory capacity of the participants (72). This is a maximal incremental test with high validity and reliability for use with adolescents (73). The test ends when the subject reaches exhaustion or is unable to complete the 20-m distance two consecutive times before the beep sounds. The last stage at which the subject finishes the test is used to predict maximal oxygen uptake (VO2 max) using the formula by Léger et al. (72).
To measure upper limb strength, two tests were carried out. First, the manual handgrip strength, which is considered a valid and reliable measurement in adolescents (74). The test requires adolescents to apply a maximum manual grip force with the elbow fully extended on a Takei Tkk5401, as this is the optimal position to produce the maximum force (75). All adolescents performed the test with both hands separately. Secondly, the push-up test was carried out. The test had a duration of 1 min. The number of final repetitions in which the elbow was fully extended and flexed during execution was counted (76).
Lower body power was measured using the countermovement jump (CMJ). The test was carried out on a force platform with a sampling frequency of 200 Hz (MuscleLab, Stathelle, Norway). The objective was to reach the highest vertical jump height. According to the guidelines in Barker et al., the hands were to be kept on the hips throughout the flight phase, the knees and ankles were to be fully extended and the adolescents were to keep their backs fully straight (77).
The curl-up test was used to assess the strength-resistance of the abdominal musculature. The adolescents had to perform as many trunk flexions as possible until they reached exhaustion, or until the end of 1 min. Those repetitions in which the upper back was no longer in contact with the ground were considered valid (78).
First, the adolescents completed the PAQ-A and the KIDMED. Subsequently, the kinanthropometric assessment was performed. Once finished, the adolescents were provided an explanation corresponding to the physical fitness tests and were familiarized with the execution of the handgrip, push up, CMJ, and curl up tests. Once the familiarization was completed, a progressive warm-up consisting of running and joint mobility involved in the physical fitness tests was performed, and two measurements of each of the tests were taken. The best score obtained was selected as the final value. Between repetitions of the same test, 2 min of rest were allowed, while 5 min were allowed between the different tests. The order in which the tests were conducted was randomized for each adolescent. Once the handgrip, push-ups, CMJ, and curl up tests were completed, a single repetition of the 20-m shuttle run test was performed, as this is a maximal incremental test whose fatigue may influence the performance of the rest of the tests. The recommendations by the National Strength and Conditioning Association (NSCA) were followed to establish the physical fitness assessment protocol, as it is based on the metabolic demands of each test, as well as the fatigue generated by the tests and the time required for recovery (79).
To minimize interference from possible contaminating variables, the pre- and post-test measurements were performed under as similar conditions as possible. Thus, to complete the PAQ-A and KIDMED questionnaires, a classroom in the school was used in which there was no noise, and the researchers only answered the adolescents’ questions, but in no case did they influence their answers. The changing rooms of the sports hall were used for kinanthropometric measurements, and a stable temperature was maintained during all the measurements. The measurements were always taken between 8:30 a.m. and 2:30 p.m. using physical education class hours, so that each class group was measured in the pre- and post-test at the same time, to avoid the time of measurement inducing changes in kinanthropometric and body composition variables (80, 81). For the physical fitness tests, the covered sports pavilion was used to prevent the weather conditions from influencing the performance of the tests.
The initial sample (n = 462) was divided into EG (n = 280) and CG (n = 182). The apps used by the EG adolescents were: Pokémon Go®, Pacer®, Strava®, and MapMyWalk®. For the use of the mobile applications, the adolescents carried their mobile device with them while walking the agreed distance for each day. The selection of this device was based on the fact that a high percentage of adolescents have a mobile device, but not all have watches or other wearables that could be used for the same purpose (82, 83).
Four different mobile apps were included to find out if there were significant differences between these when used by active and inactive adolescents. This is because previous research has shown that there may be small differences in the study variables when used in the general adolescent population (61). Four apps that included a high number of behavior change techniques were selected, based on previous studies, and that were available for android and apple, with the aim of finding out if there were differences between “similar” apps (84). In addition, the study design was not compromised as it is a small number of groups and covariates, being small the amount of information that can be lost during the execution (85). Since each school class consisted of a different number of adolescents, there were small sampling differences in EG adolescents at the beginning of the research (Pokémon Go: n = 72; MapMyWalk: n = 67; Pacer: n = 70; Strava: n = 71).
Prior to the start of the intervention, the adolescents of the EG were given a brief explanation of the application to be used and were shown how it worked and how to record the distance traveled. The adolescents then began the 10-week intervention in which they used the corresponding step tracker mobile apps after school hours a minimum of three times per week. Ten weeks was chosen because previous research has shown that interventions of short (6–12 weeks) and medium (12–26 weeks) duration are more effective than those of longer duration (more than 26 weeks) (86). Therefore, taking into account the academic year of the adolescents and the possibility to perform the pre- and post-test measurements, a duration of 10 weeks was chosen. The distance to be completed in each session was increased by 600 steps each week, starting with 7,100 steps per day in the first week and ending with 12,500 steps per day in the tenth week. This distance was used in previous research with step tracker mobile apps and was chosen because the target daily distance of the tenth week is the minimum distance established to stop being considered inactive (58, 87). Since the applications recorded the distance in kilometers, the adolescents were given the distance to be covered in kilometers (week 1: 4.57 km per session; week 10: 8.00 km per session), considering that 1 km corresponds to approximately 1,565 steps in this population (88). Regarding step tracker mobile apps, Pokémon Go was selected because it has been shown to be an effective game for increasing the level of physical activity and the number of daily steps in children and adolescents (89, 90); while Pacer, Strava and MapMyWalk were chosen because they include between 8 and 10 techniques for behavior change (84, 89, 90). The CG continued to attend physical education classes as normal and to perform the physical activity they were doing prior to the start of the investigation, but did not use any step tracker mobile apps. After 10 weeks of intervention, physical activity level, anthropometry, body composition and physical fitness of the CG and EG were measured again.
The use of the mobile applications was encouraged in the physical education course, with the adolescents who completed the intervention receiving up to one extra point in their final grade. This is similar to previous research and is due to the fact that rewards have been shown to be a great incentive to motivate adolescents in physical activity interventions (91, 92). Even so, not all EG adolescents completed the required distance each week, but they were not excluded from the research. These adolescents were measured at post-test and continued to be part of the n of the research. The intervention completion rate was different between applications. Of the 462 adolescents who initiated the study (Pokémon Go: n = 72; Map My Walk: n = 67; Pacer: n = 70; Strava: n = 71; CG: n = 182), 430 completed the investigation (6.93% attrition). Of the adolescents who completed the research, 160 belonged to the control group (12.09% attrition), 69 to Pokémon Go (4.17% attrition), 65 to Map My Walk (2.99% attrition), 67 to Pacer (4.29% attrition) and 69 to Strava (2.82% attrition).
The normality of the variables was analyzed using the Kolmogorov–Smirnov test, and kurtosis and skewness analysis. As the data followed a normal distribution, parametric tests were used for analysis. Mean and standard deviation (M ± SD) were used as descriptive values for the sample. To analyze the differences in distance traveled depending on whether the adolescents were active or inactive, as well as on the mobile application used, a Student’s t-test for independent samples and an ANOVA were used, respectively. Two Student’s t-test for independent samples were performed to determine baseline differences in all the study variables between active and inactive adolescents, as well as between adolescents belonging to the EG and CG. A MANOVA was performed to analyze differences in the study variables between adolescents who were active and inactive and who belonged to the CG or EG. Subsequently, a MANCOVA was carried out including four covariates: gender (male or female), maturity (in years from the APHV), app used (Strava, Pacer, MapMyWalk, and Pokémon Go) and distance covered with the use of the app (based on steps taken during the study). An ANCOVA was performed to analyze whether the change observed in the EG between the pre- and post-test was significantly different from that observed in the CG, both in the active and inactive groups, including the covariate gender and maturity. And to analyze whether there were significant differences in the study variables between the different apps selected, firstly, a MANOVA was performed, taking into account the level of physical activity and the app used; and secondly, an ANOVA was performed to establish whether the change between pre-post in each app was significantly different from the change in the others. Partial Eta squared (η2) was used to determine the effect size, and was defined as small: ES ≥ 0.10; moderate: ES ≥ 0.30; large: ≥ 1.2; or very large: ES ≥ 2.0, with an error of p < 0.05 (93). Statistical significance was established at p < 0.05. The statistical analysis was performed using the SPSS statistical package (v. 25.0; SPSS Inc., IL).
The average distance traveled by the adolescents during the intervention was 146.73 ± 17.96 km. In the EG of active adolescents, the average distance traveled was 147.52 ± 19.24 km, while in the EG of inactive adolescents, the average distance traveled was 145.23 ± 16.78 km. Based on the application used, the average distance traveled by users was 146.91 ± 14.35 km on Pokémon Go, 148.27 ± 16.83 km on Strava, 148.64 ± 19.42 km on Pacer and 144.53 ± 17.65 km on MapMyWalk. There were no significant differences in distance traveled between the EG groups (p = 0.864), nor between the different applications used (p = 0.657).
At baseline, significant differences were found between active and inactive adolescents in physical activity level (p < 0.001), AMD (p = 0.004), corrected arm girth (p = 0.023), corrected thigh girth (p = 0.002), corrected calf girth (p = 0. 003), fat mass (p < 0.001), sum of 3 skinfolds (p < 0.001), muscle mass (p < 0.001), VO2 max (p < 0.001), handgrip (right: p = 0.003; left: p < 0.001), CMJ (p = 0.001), curl up (p = 0.003) and push up (p < 0.001). No differences were found in body mass (p = 0.849), height (p = 0.118), waist-height ratio (p = 0.964) or BMI (p = 0.430).
By randomly dividing the active and inactive sample between EG and CG, the comparison between EG and CG at baseline showed no differences in physical activity level (p = 0.209), body mass (p = 0.063), height (p = 0.058), BMI (p = 0.071), waist/height (p = 0.997), corrected arm girth (p = 0.512), corrected thigh girth (p = 0.946), corrected calf girth (p = 0.297), fat mass (p = 0.083), sum of 3 skinfolds (p = 0.065), muscle mass (p = 0.781), V02 max. (p = 0.144), handgrip (right: p = 0.902; left: p = 0.800), CMJ (p = 0.950), curl up (p = 0.893) nor push up (p = 0.339), but differences in AMD were found (p < 0.001), with EG adolescents showing higher AMD before the start of the research.
Table 1 shows the differences between the pre- and post-test in the EG and CG of active and inactive adolescents. Thus, the EG of inactive adolescents showed a significant increase in the level of physical activity, as well as an increase in body mass, height, BMI, corrected arm girth, corrected thigh girth, muscle mass, Vo2 max, handgrip right and left hand, curl up and push up; while significantly decreasing the sum of 3 skinfolds after the intervention. As for the CG of inactive adolescents, a significant increase in physical activity level, body mass, BMI, corrected arm girth, corrected leg girth, muscle mass, Vo2 max and push-up was found after the 10-week period.
Table 1. Pre and post-test differences in the physical activity level, adherence to Mediterranean diet, body composition and kinanthropometric variables, and physical fitness in active and inactive adolescents according to the group (intra-group differences).
In the EG of active adolescents, a significant increase in body mass, height, corrected arm girth, corrected thigh girth, corrected leg girth, muscle mass, Vo2 max, handgrip right and left hand, CMJ, curl-up and push up was found; but the percentage of fat mass and the sum of 3 skinfolds decreased significantly after the training. In the CG of active adolescents there was a significant increase in body mass, height, BMI, corrected arm girth, corrected leg girth, muscle mass and handgrip left hand; however, the level of physical activity decreased significantly after the 10-week period.
Table 2 shows the influence of the covariates on the differences between pre and post of the different groups. The inclusion of the covariate gender showed significant influence on the EG evolution of inactive adolescents in the variables of physical activity, body mass, height, BMI, corrected arm girth, corrected thigh girth, muscle mass, sum of 3 skinfolds and all physical fitness variables, except CMJ. In the CG of inactive adolescents, the influence of this covariate was found in the evolution of level of physical activity, body mass, height, BMI, corrected arm girth, corrected leg girth, muscle mass, VO2 max and push-ups. In the EG of active adolescents, influence was observed in the evolution of body mass, height, corrected arm girth, corrected thigh girth, corrected leg girth, fat mass, the sum of 3 skinfolds, muscle mass and all the physical fitness variables. And in the CG of active adolescents, this covariate caused significant changes in the evolution of physical activity level, body mass, height, BMI, corrected arm girth, corrected thigh girth, corrected leg girth, muscle mass and handgrip. This covariate showed no influence on AMD in any of the groups analyzed.
Table 2. Influence of the covariates gender, maturity, app used and distance covered with the app on the intra-group differences for active and inactive adolescents.
The maturity covariate showed significant influence in EG of inactive adolescents in the evolution of body mass, height, BMI, corrected arm and thigh girths, muscle mass, handgrip right and left hand and push-ups. In the CG inactive, this covariates influenced in the evolution of body mass, BMI, corrected arm girth, muscle mass, handgrip right and left hands and push-ups. In the EG active, the influence of this covariate was observed in the evolution of body mass, height, corrected arm and thigh girths, muscle mass, handgrip right and left hands and push-ups. And in the CG active, the influence was significant in the evolution of body mass, height, BMI, corrected arm and thigh girths, muscle mass, handgrip right and left hands, and push-ups. However, this covariate did not show influence in the evolution of physical activity, AMD, waist-height, corrected calf-girth, fat mass, sum of 3 skinfolds, VO2 max., CMJ nor curl up (Table 2).
The covariate app used showed influence in the EG inactive in the involution of physical activity, body mass, VO2 max. and curl up, while in the EG active the differences were significant in the evolution of body mass, VO2 max. and curl up. And the inclusion of the covariable distance covered with the app showed significant influence on the EG of inactive adolescents in the evolution of the variable physical activity, body mass, height, BMI, corrected arm girth, corrected thigh girth, sum of 3 skinfolds, muscle mass and all the physical fitness test, except CMJ. In the EG of active adolescents, influence was observed on the evolution of body mass, height, corrected arm girth, corrected thigh girth, corrected calf girth, muscle mass and all the physical fitness test. Neither of the two covariates showed any influence on AMD in any of the groups analyzed (Table 2).
Table 3 shows the differences in the change showed by EG and CG in both the active and inactive adolescent groups. The results showed significant differences in the group of active adolescents in BMI, with the change observed being greater in the CG, with a significant effect of the covariates gender and maturity. In the case of the CMJ test for active group, a greater change was found in EG compared to CG, without influence of the covariates. No significant differences were found in the rest of the variables analyzed. Thus, the difference between the intra-group change in EG and CG was not significant for physical activity level and AMD. Neither was it significant for kinanthropometric variables or body composition (body mass, height, waist-height, corrected girths, fat mass, sum of 3 skinfolds or muscle mass), nor for fitness variables (VO2 max, handgrip left and right hands, curl-up or push-up). The covariates gender and maturity also showed no effect on the variables for which the analysis of change was not statistically significant.
Table 3. Inter-group differences (EG vs. CG) in the intra-group differences for active and inactive groups.
Table 4 shows the pre-post differences for each of the variables according to the different mobile apps used by active and inactive adolescents. There was a significant increase in the level of physical activity in the inactive group with all the apps (p < 0.001–0.032). In body mass, there was an increase in Strava (p < 0.001), Pacer (p = 0.003) and MapMyWalk (p = 0.007) inactives, as well as in Pokémon Go (p = 0.003) and Strava (p < 0.001) actives. Significant increases occurred in all groups in height (p < 0.001–0.049), except in the MapMyWalk actives group (p = 0.105). In corrected arm girth there was a significant increase in Pacer (p = 0.009) and MapMyWalk (p = 0.011) inactives, as well as in Pokémon Go (p < 0.001), Strava (p = 0.006) and MapMyWalk (p = 0.004) actives. In the corrected thigh girth the significant increase occurred in the group of Strava (p = 0.004) and Pacer (p < 0.001) inactives, as well as in Strava actives (p = 0.025). And in corrected calf girth, a significant increase was recorded in the inactive Pacer (p = 0.024) and active Strava (p = 0.012). Only a significant decrease in fat mass (p = 0.011) and sum of 3 skinfolds (p < 0.001) was recorded in the inactive Pacer participants. In contrast, muscle mass increased significantly in all groups analyzed (p < 0.001–0.049). Regarding fitness tests, a significant increase in V02 max was found in Pacer inactive (p = 0.001) and Strava active (p < 0.001). Regarding the handgrip, there was an increase in the active and inactive Pacer (p < 0.001), as well as in the active Strava (p = 0.010). In curl-up, there was a significant increase in performance in those active and inactive on Strava (p < 0.001) and in those inactive on MapMyWalk (p = 0.014). And, in push-up, there was a significant improvement in those active (p = 0.013) and inactive (p = 0.028) from Pokémon Go. Despite the results obtained, Table 5 shows that the analysis of the change between the different applications did not show significant differences in any of the analyzed variables.
Table 4. Intra-group differences in the study variables in the groups of active and inactive adolescents according to the app used.
Table 5. Differences in the change between the pre and posttest in the study variables according to the app used.
The first objective of the present research was to analyze the differences in the level of physical activity, AMD, anthropometry, body composition, and physical fitness of active and inactive adolescents achieved by a 10-week program of after-school use of step tracker mobile apps promoted from the physical education subject. The results showed that there was no difference in the distance traveled by the adolescents with the use of the mobile application depending on whether they were active or inactive, nor depending on the app used. Regarding the perceived level of physical activity, there were significant increases in both inactive groups (EG and CG) and in the active CG, while in AMD there were no significant differences in either group between the pre- and post-test. In the kinanthropometric and body composition variables, the results showed significant increases in body mass, height, BMI, corrected girths and muscle mass in all the groups. Furthermore, in active and inactive adolescents, a decrease in fat mass and in the sum of 3 skinfolds was observed in the EGs. Regarding the physical fitness variables, the EGs of active and inactive adolescents showed improved performance in all the physical fitness tests, with the exception of the CMJ in the inactive group.
The second objective of the research was to determine the influence of gender, maturity, the app used, and the number of steps taken with the mobile apps on the study variables. Gender showed a significant effect on the kinanthropometric and body composition variables, physical fitness test and physical activity level of the active and inactive adolescents in CG and EG. The maturity status seemed to influence the body mass and height, the muscle mass variables and most physical fitness test in all the groups. According to the app used, this covariate influenced the level of physical activity of inactive adolescents, as well as the body mass, VO2 max and curl up of active and inactive adolescents. And the covariate distance covered with the app showed significant influence in the physical activity, body mass, height, BMI, corrected arm girth, corrected thigh girth, sum of 3 skinfolds, muscle mass and all the physical fitness test, except CMJ, on the inactive adolescents; as well as in the body mass, height, corrected arm girth, corrected thigh girth, corrected calf girth, muscle mass and all the physical fitness test on the active adolescents.
Despite the results obtained, the analysis of the change in the differences found in the study variables in the EG and CG in both the active and inactive groups showed that only the change in BMI was significantly greater in the CG than in the EG, with a significant influence of the covariates gender and maturity; and that the changes in CMJ were greater in the EG than in the CG, with a significant influence of the covariate maturity. It should also be noted that, although there were differences between the changes caused by the four apps when analyzing pre-post intra-group differences, there were no inter-group differences in the changes generated by the different apps.
According to the kinanthropometric and body composition variables, one of the main findings of the present investigation was the decrease in fat mass and in the sum of 3 skinfolds in active and inactive adolescents of the EGs, without significant changes in active and inactive adolescents of the CGs. This could lead to consider that the interventions with step tracker mobile apps can slightly reduce the accumulation of fat mass in the adolescent population, regardless of the initial level of physical activity of the adolescents. Thus, the increased physical activity performed by the adolescents in the EG would increase their energy expenditure, which would favor the loss of fat mass, as observed in previous research (94). However, it should be noted that the active adolescents in the EG did not report a significant increase in the physical activity performed. This could be due to the fact that when recording this variable by means of a self-completed questionnaire, these students did not perceive that going for a walk three times a week increased the daily physical activity performed.
In terms of muscle mass variables, both active and inactive EG and CG adolescents showed significant increases after the intervention, which leads to consider that the changes were not solely due to the intervention performed with the step tracker mobile apps. The fact that participants used aerobic applications where no strength-endurance training was performed leads one to believe that changes in muscle mass were not generated by the intervention (95). Therefore, a possible explanation for these results could be that the maturation process in which adolescents are immersed was the cause of the changes in muscle mass variables, which explains why changes in muscle mass variables also occurred in the CGs (96). Puberty is a key stage in adolescent musculoskeletal development, and is characterized by increases in the accumulation of sex steroid hormones such as testosterone or growth hormone (96–98). This increase in hormone concentration is highly correlated with increased muscle mass, as well as increased strength production (99–101).
Similarly, the observed changes in height and body mass in all the groups could be due to the maturational process (102). Previous research has shown that the maximum peak growth (APHV) occurs in girls between the ages of 11.4 and 12.2, and in boys between 13.8 and 14.4 years of age increasing in height during these ages at a rate of 9–10.3 cm per year (60, 103, 104). Body mass is also affected during this stage, increasing by 8.3 kg per year in girls and 9 kg per year in boys (105, 106). Furthermore, changes in height and body mass would be the cause of the changes in BMI, which would explain why this variable was modified in all groups. However, despite being a widely used index in previous scientific literature it does not allow distinguishing whether the changes are due to changes in fat mass or muscle mass (107, 108).
Therefore, based on the results concerning kinanthropometric and body composition variables, it seems that the 10-week intervention with step tracker mobile apps could generate changes only in fat variables. However, the changes in body mass, height, BMI, and muscle mass variables seem to be the result of changes caused by the maturation process in which adolescents are immersed. The results obtained in the present study support these assertions, since the inclusion of the covariate maturity showed influence on all the kinanthropometric and body composition variables, with the exception of fat mass and sum of 3 skinfolds.
Regarding the physical fitness variables, the EGs of active and inactive adolescents showed improved performance in all the physical fitness tests, with the exception of the CMJ in the inactive group. In this case, the covariate maturity was shown to be determinant in the physical fitness tests related to muscular strength (handgrip and push-ups), but not in those of cardiorespiratory capacity or curl-up in which the distance covered with the app were more relevant. A possible explanation for these results could be that walking programs favor the strength-endurance of the abdominal muscles, as walking activates the core-stabilizing muscles, and could improve it after 10 weeks of intervention (109, 110). In terms of cardiorespiratory capacity, the use of aerobic applications based on walking would help increase performance in this test in both active and inactive adolescents, by including in the intervention, a weekly increase in the volume of walking to end in a daily distance equivalent to what an active adolescent should do (57). Differences were found in CMJ performance in the group of active adolescents, but the absence of these differences in the group of inactive adolescents could be due to the fact that this test requires a correct technical execution, which is present in many sports when performing vertical jumps (111, 112). This could indicate that in athletes with previous experience with this type of test, the improvements in vertical jump performance after the mobile app-based intervention were due to an improvement in lower limb strength (61). However, in inactive subjects, this improvement could be diluted by the lack of proper technique during the execution of the test by the participants.
The fact that all groups in the study improved handgrip and push-up performance, together with the fact that the covariate maturity showed influence in these tests, and the fact that mobile apps were not included for strength work, lead us to consider that the changes in these variables would be more related to the increase in muscle mass and strength as a result of the maturation process, than to the 10-week intervention (113). Therefore, another finding of relevance of the present investigation was that the fitness variables that could be most affected by the 10-week intervention were VO2 max and curl-up, while the rest of the variables seem to be more affected by the maturational process and associated changes in muscle mass.
It is also worth mentioning that the level of physical activity only showed significant changes in inactive adolescents. This could be because the perception of active adolescents, regardless of the volume of training completed, is that walking is not a sporting activity in the way that practicing a particular sport might be, so their perception is that the use of apps does not increase the physical activity performed. However, in the group of inactive adolescents, whose level of practice was lower, the inclusion of walking three times a week would be perceived as a substantial increase in the physical activity practiced.
Similarly, the absence of differences in AMD in either group is a relevant finding. The fact that the selected step tracker mobile apps did not include any type of nutritional guidelines could explain the results found, as previous studies using mobile nutrition applications have shown them to be effective in controlling intake, and in achieving changes in the anthropometry and body composition of adolescents (57, 114).
However, despite the results obtained, it should be noted that the results of the analysis of the change observed between EG and CG adolescents were only significant in BMI and CMJ for active participants, and the effect sizes of these changes were small in all the variables analyzed. More specifically, there was a greater body mass gain in the active CG, and a greater CMJ gain in the active EG. Therefore, the evolution of most variables showed no significant change between the EG and the CG. On the one hand, this could be because longer interventions may be necessary to obtain differences in the changes between EG and CG (115). On the other hand, intensity, as opposed to distance, could be a stronger differentiating factor for generating changes in some of these variables (58, 116). Therefore, although some of the changes achieved during the research in the EG seem to be the result of the 10-week intervention with mobile applications, future research is needed to determine the real potential of this type of tool to increase physical activity and achieve substantial changes in kinanthropometric, body composition and physical fitness variables in the adolescent population.
The results obtained highlight the importance of the covariates included in the present study. The covariate gender seems to influence the level of physical activity, kinanthropometric and body composition variables, as well as physical fitness variables. Previous research has shown that the practice of physical activity of adolescent males tends to be higher than that of adolescent females (117). The results of the present research could indicate that step tracker mobile apps are a good strategy to increase physical activity practice in active and inactive females. These results are in line with previous research where it was observed that females used physical activity apps to a higher extent than males when their use was promoted in physical education classes (118). Regarding anthropometry and body composition, differences were observed in the muscle mass of all the groups analyzed, and in the fat mass of the EG. These results are consistent with those found in previous research, in which the muscle mass of adolescent males was higher than that of adolescent females (119). It is true that during the maturation process, steroid hormones increase in both males and females, but the accumulation is much greater in males, which generates greater increases in muscle mass (59, 120–122). In contrast, there is a greater accumulation of fat mass in females during puberty, which would be influencing the effects of the intervention program (59, 120, 121). Similarly, differences were significant in the majority of the physical fitness variables in the EG of active and inactive adolescents. It should also be noted that in the physical fitness tests related to body strength and cardiorespiratory capacity, the differences were significant according to gender in all groups. The greater development of muscle mass and strength of males with respect to females during puberty, as well as the greater cardiorespiratory capacity shown in previous research, could be the explanation for the results found (123). Another explanation could be that males generally practice more physical activity than females, so it is possible that this is the factor that causes a higher performance in all the fitness test in males (109).
The maturational process in which adolescents are immersed plays a very relevant role in the results of this research. As it has been observed, most of the changes in the variables of muscle mass, as well as in body mass, height and BMI are influenced by maturation. Similarly, the physical fitness variables related to strength, mainly handgrip and push-ups present a similar influence. This is due to hormonal changes and muscle mass development that occur during puberty (122). However, the fact that the changes in the level of physical activity, fat mass variables, VO2 max and curl up were not affected by this covariate, suggests that the intervention generates changes in these variables.
A significant finding was that the app used has been shown to influence the level of physical activity of inactive adolescents, as well as kinanthropometric and body composition, and physical fitness variables for both active and inactive adolescents. Specifically, the results showed differences in physical activity level, body mass, height, corrected perimeters, fat mass, sum of 3 skinfolds, muscle mass, V02 max, handgrip, curl up and push up when analyzing each of the mobile apps individually. However, the change in these variables between the different mobile apps was not significant, so there were no different effects between apps. These results show that the applications, even if they have different techniques for behavior change (84), can have similar effects. This is relevant because step-counting apps, such as Strava, Pacer and MapMyWalk, as well as gamified apps, such as Pokémon Go, can be effective with both active and inactive adolescents. These results are similar with previous research in which differences were seen in the study variables, mainly body mass, handgrip and curl up, depending on the application used (61), but bring the great novelty that the change produced between apps in these variables is not significant. Furthermore, the fact that the selected application shows different effects on the increase in physical activity depending on the level of physical activity is very relevant. That said, it would be important for future research to analyze the differences between interventions delivered with different apps in the longer term, to see if the fact that some of them are gamified may have an influence on the psychological variables that lead to greater use of them in the longer term, as has been seen in research in other fields (124, 125).
And, regarding the covariate distance covered with the use of the apps, the results showed a significant influence on the kinanthropometric and body composition variables both in active and inactive adolescents. This could indicate that the greater the distance covered with the application, the greater the changes achieved in the anthropometry and body composition of adolescents, regardless of whether or not they regularly practiced physical activity. These results are of great relevance, as they would indicate that the completion of a 10-week aerobic training program using step tracker mobile apps for its quantification would be effective in producing improvements in anthropometry and body composition in this population, at least for the reduction in fat mass, which would follow the line of previous research that used step tracker mobile apps (58). Similarly, the distance traveled with the use of the step tracker mobile apps showed an influence on all physical fitness variables, regardless of whether the adolescents were active or inactive before starting the research. It is logical to think that regardless of the previous level of practice, the improvements would be greater in adolescents who completed a longer distance with the use of step tracker mobile apps, as they would be more active, allowing them to obtain a greater physical fitness, as shown in previous research (58).
The main contribution of the present investigation with respect to previous scientific evidence is that active and inactive adolescents after the use of mobile applications for 10 weeks could show changes in fat mass, cardiorespiratory capacity and the strength-endurance of the abdominal muscles. It is true that previous research had shown the benefits of physical activity interventions, and more specifically of interventions with mobile applications, on these variables in the adolescent population (58). In this regard, previous research have shown a decrease in fat variables, as well as an improvement in performance in physical fitness tests, such as CMJ, curl-up, push-up or handgrip, after the interventions (46, 61). This is similar to the results obtained in the present research; however, the main contribution of this study and which had not been observed in any previous study is that the results are equally significant for previously active and inactive adolescents, with no significant differences between these groups, this study being novel in that sense. In addition, the fact that curl-up and cardiorespiratory capacity were not influenced by maturity is also new to this study and could indicate the effects of the intervention on these variables.
The improvement in other physical fitness variables as handgrip and push-up after intervention with step-tracking mobile apps also follows the line of previous research (61). However, this study provides two main novelties. The first is that both active and inactive adolescents belonging to the GE and the CG showed benefits in these tests. The second is that the maturity covariate was shown to significantly influence these variables. Therefore, this is the first study that shows the effect that maturity could play in the changes obtained in tests related to upper limb strength in this type of intervention. It is logical to think of the effect of maturity since this intervention did not include upper limb strength work, but in previous investigations this argument was used to justify the changes and in none of them was the influence statistically demonstrated.
Another novelty of the present study is that the increases in the level of physical activity occurred only in the group of inactive adolescents. Previous studies have shown that the implementation of step-tracker app-based interventions could increase self-perceived physical activity in adolescents (126). However, no previous study had examined the influence of adolescents’ previous activity level on this change. The present novel study is novel in this respect. Furthermore, the absence of differences in AMD after intervention with step tracker mobile apps had been also observed in previous research (115).
In addition, the inclusion of the covariates gender, maturity, app used and distance traveled with the app and their effect on the study variables allows us to learn more about the changes that can actually be caused by the 10-week intervention with step tracker mobile apps. Previous studies have suggested that the effects of step-tracker intervention could depend on gender, the app used or the distance traveled with it (61, 118). However, in the case of the app used, the changes in the study variables when comparing between the different mobile applications were not significant, so there was no greater effect of one app on the others in either the active or inactive group. For its part, it has been shown that maturation could have a significant influence on the effects of physical exercise on anthropometric variables, body composition or physical fitness (120). However, this is the first research to analyze the effects of these factors according to whether the adolescents were active or inactive.
According to the results obtained in the present investigation, H1 in which indicated that the use of mobile step-tracking apps will be more effective on the study variables in inactive adolescents, since their level of physical activity is lower than that of active ones, can be partially rejected. This is because the changes occurred in both active and inactive adolescents, with the exception of the level of physical activity, in which only inactive adolescents perceived a greater improvement after the intervention, and the CMJ test, where significant changes were found only in the active adolescents. Regarding H2 in which indicated that gender, maturity, app used and the number of steps taken with the mobile apps will influence the changes produced in the study variables after the use of the mobile apps, it can be accepted. This is because all four covariates were shown to influence the study variables, mainly gender and maturity, although in the case of the app used there were no significant differences when considering the change produced by each of the apps.
The present investigation is not without limitations. The sample was selected by convenience, by choosing the compulsory secondary schools with the largest number of adolescents in the geographical area of study. The level of physical activity was assessed using the PAQ-A questionnaire, which is a subjective measure of physical activity, which could influence the results obtained. It is also important to note that this questionnaire only records the physical activity of the last week, which might not give an overall picture of the subject’s level of physical activity. In addition, the PAQ-A classifies subjects as active or inactive, without providing an intermediate range between the two conditions of physical activity. In addition, although the results of the KIDMED questionnaire did not show changes in adolescent AMD, other instruments should be used to assess adolescent caloric intake, as it could significantly influence changes in the anthropometry and body composition in this population.
In view of the limitations present in the research, the following lines of future research are proposed: (a) it would be important to complement the subjective information on perceived physical activity obtained by means of questionnaires with an objective measure. This could be carried out by accelerometry, or by means of wearable devices that allow the collection of data on physical activity throughout the day; (b) the use of mobile applications that include strength-endurance exercises would allow to know whether the effect of the maturational process is the cause of changes in muscle mass variables and fitness tests, or whether these are also modified by appropriate training; (c) interventions that include nutritional and physical activity guidelines are more effective than those that isolate one of these behaviors (97). Considering the absence of changes in AMD with the present intervention, future studies should use mobile step-tracking apps that track both physical activity and nutrition, or that include recommendations in both areas, as the results could be superior to those found in the present investigation; (d) future research should address the effect of the step-tracker apps on the results found, as it could be very relevant to decrease the time of physical inactivity in adolescents; (e) should also be examined in depth as to whether the distance traveled may be a more important determinant factor in an inactive or active group; and (f) this is the first study using the mobile apps and dividing the results according to the previous physical activity level of the adolescents, so the results should be taken with caution and future research is needed to corroborate them.
The following practical applications are derived from this research: (a) the step tracker mobile apps can be a very useful tool to be used in physical education classes, or promoted in the field of health, to increase the level of physical activity of inactive adolescents; (b) the use of mobile app step trackers can be a useful tool to favor the perception of competence, autonomy and social relationships in relation to physical activity. This could increase the satisfaction of the basic psychological needs of adolescents, which could be especially important among inactive adolescents. Thus, the use of mobile apps for the promotion of physical activity could lead to an improvement in the basic psychological needs of adolescents and, therefore, to greater adherence to the practice of physical activity; (c) the improvement in the fat variables after the intervention, both in the group of active and inactive adolescents, leads to consider these interventions as effective in improving body composition, which is decisive due to the increase in the rate of overweight and obesity in the adolescent population, especially among inactive adolescents, and could be a tool of great interest for the promotion of public health; (d) the use of rewards from the physical education class seems to be a useful strategy to increase participation and gain benefits from the intervention. This could be due to the fact that this external factor influences external regulation, which would affect adolescents’ motivation. Therefore, if the use of apps is promoted from the physical education subject with an increase in the grade, initial adherence to the program may benefit; and (e) the present study demonstrates the benefits of the use of mobile applications in both active and inactive adolescents, which is fundamental to combat the negative perception that currently exists toward the use of mobile devices in this population. This leads to the view that it is not so much the time spent using the devices that is really harmful, but the apps used.
Regardless of the initial level of physical activity, the use of step tracker mobile apps in out-of-school hours, promoted from the subject of physical education, resulted in significant improvements in fat variables and in cardiorespiratory capacity and curl-up performance of adolescents aged 12–16 years old. The evolution of the rest of the kinanthropometric and body composition variables, as well as the physical fitness variables, seem to be more strongly influenced by gender and maturity status than by the physical exercise intervention. It should be noted that although both active and inactive adolescents in the EG used the step tracker mobile apps during the intervention period, only adolescents who were inactive before the start of the study perceived improvements in their level of physical activity. In addition, the app used and the distance covered with the apps seem to be influential in the results. Therefore, mobile step tracking apps may be a useful alternative to obtain improvements in fat variables and in some of the physical fitness variables, but the results obtained should be taken with caution because it is the first research to be carried out dividing adolescents according to their previous level of physical activity.
The raw data supporting the conclusions of this article will be made available by the authors, without undue reservation.
The studies involving humans were approved by Institutional committee of the Catholic University of Murcia (code: CE022102). The studies were conducted in accordance with the local legislation and institutional requirements. Written informed consent for participation in this study was provided by the participants’ legal guardians/next of kin.
NG-C: Formal analysis, Investigation, Writing – original draft, Writing – review & editing. AM-O: Conceptualization, Data curation, Formal analysis, Investigation, Methodology, Writing – original draft, Writing – review & editing. LM: Investigation, Writing – original draft, Writing – review & editing. LA-C: Conceptualization, Funding acquisition, Investigation, Project administration, Writing – original draft, Writing – review & editing. RV-C: Conceptualization, Data curation, Formal analysis, Funding acquisition, Investigation, Methodology, Project administration, Writing – original draft, Writing – review & editing.
The author(s) declare that financial support was received for the research, authorship, and/or publication of this article. This research was financed by grants from the Ministry of Science and Innovation of the Spanish Government, in the program knowledge generation (Title: “Promotion of Daily Physical Activity recommended for the Health of Adolescents through Mobile Apps, Wearables and a Gamified TAC”/Call: 2023/Reference code: PID2022-140245OA-I00). This work was part of the doctoral thesis of Nerea Gómez-Cuesta.
The authors would like to thank the schools, management teams, teachers, students, and research assistants who made it possible to carry out the measurements for this project.
The authors declare that the research was conducted in the absence of any commercial or financial relationships that could be construed as a potential conflict of interest.
All claims expressed in this article are solely those of the authors and do not necessarily represent those of their affiliated organizations, or those of the publisher, the editors and the reviewers. Any product that may be evaluated in this article, or claim that may be made by its manufacturer, is not guaranteed or endorsed by the publisher.
1. Cristi-Montero, C, Celis-Morales, C, Ramírez-Campillo, R, Aguilar-Farías, N, Álvarez, C, and Rodríguez-Rodríguez, F. ¡Sedentarismo e inactividad física no son lo mismo!: una actualización de conceptos orientada a la prescripción del ejercicio físico para la salud. Rev Med Chile. (2015) 143:1089–90. doi: 10.4067/S0034-98872015000800021
2. Kohl, HW, Craig, CL, Lambert, EV, Inoue, S, Alkandari, JR, Leetongin, G, et al. The pandemic of physical inactivity: global action for public health. Lancet. (2012) 380:294–305. doi: 10.1016/S0140-6736(12)60898-8
3. Tabacchi, G, Faigenbaum, A, Jemni, M, Thomas, E, Capranica, L, Palma, A, et al. Profiles of physical fitness risk behaviours in school adolescents from the ASSO project: a latent class analysis. Int J Environ Res Public Health. (2018) 15:1933. doi: 10.3390/ijerph15091933
4. García-Tascón, M, Mendaña-Cuervo, C, Sahelices-Pinto, C, and Magaz-González, A-M. Repercusión en la calidad de Vida, salud y práctica de actividad física del confinamiento por Covid-19 en España (effects on quality of life, health and practice of physical activity of Covid-19 confinement in Spain). Retos. (2022) 42:684–95. doi: 10.47197/retos.v42i0.88098
5. Guthold, R, Stevens, GA, Riley, LM, and Bull, FC. Global trends in insufficient physical activity among adolescents: a pooled analysis of 298 population-based surveys with 1·6 million participants. Lancet Child Adolesc Health. (2020) 4:23–35. doi: 10.1016/S2352-4642(19)30323-2
6. Pechtl, SML, Kim, LP, and Jacobsen, KH. Physical inactivity and sedentariness: languorous behavior among adolescents in 80 countries. J Adolesc Health. (2022) 70:950–60. doi: 10.1016/j.jadohealth.2021.12.017
7. Anderson, E, and Durstine, JL. Physical activity, exercise, and chronic diseases: a brief review. Sports Med Health Sci. (2019) 1:3–10. doi: 10.1016/j.smhs.2019.08.006
8. Wellman, RJ, Sylvestre, M, Abi Nader, P, Chiolero, A, Mesidor, M, Dugas, EN, et al. Intensity and frequency of physical activity and high blood pressure in adolescents: a longitudinal study. J Clin Hypertens. (2020) 22:283–90. doi: 10.1111/jch.13806
9. Myers, J, Kokkinos, P, and Nyelin, E. Physical activity, cardiorespiratory fitness, and the metabolic syndrome. Nutrients. (2019) 11:1652. doi: 10.3390/nu11071652
10. Santos, AC, Willumsen, J, Meheus, F, Ilbawi, A, and Bull, FC. The cost of inaction on physical inactivity to public health-care systems: a population-attributable fraction analysis. Lancet Glob Health. (2023) 11:e32–9. doi: 10.1016/S2214-109X(22)00464-8
11. Telama, R, Yang, X, Viikari, J, Välimäki, I, Wanne, O, and Raitakari, O. Physical activity from childhood to adulthood. Am J Prev Med. (2005) 28:267–73. doi: 10.1016/j.amepre.2004.12.003
12. Hu, D, Zhou, S, Crowley-McHattan, ZJ, and Liu, Z. Factors that influence participation in physical activity in school-aged children and adolescents: a systematic review from the social ecological model perspective. Int J Environ Res Public Health. (2021) 18:3147. doi: 10.3390/ijerph18063147
13. Musa, S, Elyamani, R, and Dergaa, I. COVID-19 and screen-based sedentary behaviour: systematic review of digital screen time and metabolic syndrome in adolescents. PLoS One. (2022) 17:e0265560. doi: 10.1371/journal.pone.0265560
14. Chen, Y, Osika, W, Henriksson, G, Dahlstrand, J, and Friberg, P. Impact of COVID-19 pandemic on mental health and health behaviors in Swedish adolescents. Scand J Public Health. (2022) 50:26–32. doi: 10.1177/14034948211021724
15. Zenic, N, Taiar, R, Gilic, B, Blazevic, M, Maric, D, Pojskic, H, et al. Levels and changes of physical activity in adolescents during the COVID-19 pandemic: contextualizing urban vs. Rural Liv Environ Appl Sci. (2020) 10:3997. doi: 10.3390/app10113997
16. Margaritis, I, Houdart, S, El Ouadrhiri, Y, Bigard, X, Vuillemin, A, and Duché, P. How to deal with COVID-19 epidemic-related lockdown physical inactivity and sedentary increase in youth? Adaptation of Anses’ benchmarks. Arch Public Health. (2020) 78:52. doi: 10.1186/s13690-020-00432-z
17. Castañeda-Babarro, A, Arbillaga-Etxarri, A, Gutiérrez-Santamaría, B, and Coca, A. Physical activity change during COVID-19 confinement. Int J Environ Res Public Health. (2020) 17:6878. doi: 10.3390/ijerph17186878
18. Tapia-Serrano, MA, Sánchez-Oliva, D, Sevil-Serrano, J, Marques, A, and Sánchez-Miguel, PA. 24-h movement behaviours in Spanish youth before and after 1-year into the covid-19 pandemic and its relationship to academic performance. Sci Rep. (2022) 12:16660–10. doi: 10.1038/s41598-022-21096-5
19. Tapia-Serrano, MA, Vaquero-Solís, M, López-Gajardo, MA, and Sánchez-Miguel, PA. Adherencia a la dieta mediterránea e importancia de la actividad física y el tiempo de pantalla en los adolescentes extremeños de enseñanza secundaria. Nutr Hosp. (2021) 38:236–44. doi: 10.20960/nh.03372
20. Ferrari Gl De, M, Kovalskys, I, Fisberg, M, Gómez, G, Rigotti, A, LYC, S, et al. Socio-demographic patterning of objectively measured physical activity and sedentary behaviours in eight Latin American countries: findings from the ELANS study. Eur J Sport Sci. (2020) 20:670–81. doi: 10.1080/17461391.2019.1678671
21. Chaput, J-P, Willumsen, J, Bull, F, Chou, R, Ekelund, U, Firth, J, et al. 2020 WHO guidelines on physical activity and sedentary behaviour for children and adolescents aged 5–17 years: summary of the evidence. Int J Behav Nutr Phys Act. (2020) 17:1–9. doi: 10.1186/s12966-020-01037-z
22. Adams, MA, Johnson, WD, and Tudor-Locke, C. Steps/day translation of the moderate-to-vigorous physical activity guideline for children and adolescents. Int J Behav Nutr Phys Act. (2013) 10:49–11. doi: 10.1186/1479-5868-10-49
23. Grace, J, Bosch, M, and Du Toit, J. The effect of a walking programme on the psychological well-being and selected physical and physiological parameters of overweight and obese adolescents. Afr J Phys Health Educ Recreat Dance. (2015) 21. Available at: https://hdl.handle.net/10520/EJC182294
24. Kantanista, A, Bronikowski, M, Laudańska-Krzemińska, I, Król-Zielińska, M, and Osiński, W. Positive effect of pedometer-based walking intervention on body image and physical activity enjoyment in adolescent girls. Biomed Hum Kinet. (2017) 9:34–42. doi: 10.1515/bhk-2017-0006
25. Murtagh, EM, Nichols, L, Mohammed, MA, Holder, R, Nevill, AM, and Murphy, MH. Walking to improve cardiovascular health: a meta-analysis of randomised control trials. Lancet. (2014) 384:S54. doi: 10.1016/S0140-6736(14)62180-2
26. Morris, JN, and Hardman, AE. Walking to health. Sports Med. (1997) 23:306–32. doi: 10.2165/00007256-199723050-00004
27. Carlsson, S, Andersson, T, Wolk, A, and Ahlbom, A. Low physical activity and mortality in women: baseline lifestyle and health as alternative explanations. Scand J Public Health. (2006) 34:480–7. doi: 10.1080/14034940600551293
28. Howie, EK, McVeigh, JA, Smith, AJ, Zabatiero, J, Bucks, RS, Mori, TA, et al. Physical activity trajectories from childhood to late adolescence and their implications for health in young adulthood. Prev Med. (2020) 139:106224. doi: 10.1016/j.ypmed.2020.106224
29. Martins, J, Marques, A, Gouveia, ÉR, Carvalho, F, Sarmento, H, and Valeiro, MG. Participation in physical education classes and health-related behaviours among adolescents from 67 countries. Int J Environ Res Public Health. (2022) 19:955. doi: 10.3390/ijerph19020955
30. Sierra-Díaz, MJ, González-Víllora, S, Pastor-Vicedo, JC, and López-Sánchez, GF. Can we motivate students to practice physical activities and sports through models-based practice? A systematic review and Meta-analysis of psychosocial factors related to physical education. Front Psychol. (2019) 10:2115. doi: 10.3389/fpsyg.2019.02115
31. Gea-García, GM, González-Gálvez, N, Espeso-García, A, Marcos-Pardo, PJ, González-Fernández, FT, and Martínez-Aranda, LM. Relationship between the practice of physical activity and physical fitness in physical education students: the integrated regulation as a mediating variable. Front Psychol. (2020) 11:1910. doi: 10.3389/fpsyg.2020.01910
33. Blasco, MJ, Puig, T, Balada, G, Gich, I, Hernández, H, Parra, M, et al. Perfil de salud, prevención del riesgo cardiovascular y ejercicio físico en adolescentes. Apunts Educ Física Deport. (2021) 2:18–24. doi: 10.5672/apunts.2014-0983.es.(2021/2).144.03
34. Pastor-Vicedo, JC, Martínez-Martinez, J, López-Polo, M, and Prieto-Ayuso, A. Recreos activos como estrategia de promoción de la actividad física: una revisión sistemática. Retos. (2021) 40:135–44. doi: 10.47197/retos.v1i40.82102
35. Barfield, PA, Ridder, K, Hughes, J, and Rice-McNeil, K. Get outside! Promoting adolescent health through outdoor after-school activity. Int J Environ Res Public Health. (2021) 18:7223. doi: 10.3390/ijerph18147223
36. Martin-Moraleda, E, Mandic, S, Queralt, A, Romero-Blanco, C, and Aznar, S. Associations among active commuting to school and prevalence of obesity in adolescents: a systematic review. Int J Environ Res Public Health. (2022) 19:1–13. doi: 10.3390/ijerph191710852
37. Mendoza, JA, Watson, K, Baranowski, T, Nicklas, TA, Uscanga, DK, and Hanfling, MJ. The walking school bus and Children’s physical activity: a pilot cluster randomized controlled trial. Pediatrics. (2011) 128:e537–44. doi: 10.1542/peds.2010-3486
38. Khodanazari, H, Choupani, A-A, and Aghayan, I. The contribution of walking to School in Students’ physical activity and its effect on being overweight. Biomed Res Int. (2022) 2022:1–15. doi: 10.1155/2022/2633109
39. Gil-Espinosa, FJ, Nielsen-Rodríguez, A, Romance, R, and Burgueño, R. Smartphone applications for physical activity promotion from physical education. Educ Inf Technol. (2022) 27:11759–79. doi: 10.1007/s10639-022-11108-2
40. He, Z, Wu, H, Yu, F, Fu, J, Sun, S, Huang, T, et al. Effects of smartphone-based interventions on physical activity in children and adolescents: systematic review and Meta-analysis. JMIR Mhealth Uhealth. (2021) 9:e22601–12. doi: 10.2196/22601
41. Vega-Ramírez, L, Notario, RO, and Ávalos-Ramos, MA. The relevance of Mobile applications in the learning of physical education. Educ Sci. (2020) 10:329. doi: 10.3390/educsci10110329
42. Gil-Espinosa, FJ, Merino-Marbán, R, and Mayorga-Vega, D. Aplicación móvil Endomondo para promocionar la actividad física en estudiantes de educación secundaria (Endomondo smartphone app to promote physical activity in high school students). Cult Cienc Depor. (2020) 15:465–73. doi: 10.12800/ccd.v15i46.1597
43. Direito, A, Jiang, Y, Whittaker, R, and Maddison, R. Smartphone apps to improve fitness and increase physical activity among young people: protocol of the apps for IMproving FITness (AIMFIT) randomized controlled trial. BMC Public Health. (2015) 15:635. doi: 10.1186/s12889-015-1968-y
44. Alonso-Fernández, D, Gutiérrez-Sánchez, Á, Portela-Pino, I, and Taboada-Iglesias, Y. Evaluation of applications for Mobile devices on the practice of physical exercise in adolescents. Appl Sci. (2022) 12:2784. doi: 10.3390/app12062784
45. Shin, Y, Kim, SK, and Lee, M. Mobile phone interventions to improve adolescents’ physical health: a systematic review and meta-analysis. Public Health Nurs. (2019) 36:787–99. doi: 10.1111/phn.12655
46. Mateo-Orcajada, A, Vaquero-Cristóbal, R, and Abenza-Cano, L. Influence of Pokémon go playing style on physical activity and its effect on Kinanthropometry variables and body composition in adolescents. J Phys Act Health. (2023) 20:934–47. doi: 10.1123/jpah.2023-0140
47. Nikhita, CS . Prevalence of Mobile phone dependence in secondary school adolescents. J Clin Diagn Res. (2015) 9:VC06–9. doi: 10.7860/JCDR/2015/14396.6803
48. Vieira, LL , Oliveira V de, Machado, AA, and Tertuliano, IW. Reasons for adherence and abandonment of physical activity. Man Ther Posturol Rehabil J (2020) 16:601. doi: 10.17784/mtprehabjournal.2018.16.601
49. Mateo-Orcajada, A, Vaquero-Cristóbal, R, Rey-López, JP, Martín-Campoy, R, and Abenza-Cano, L. The role of basic psychological needs in the adoption of healthy habits by adolescents. Behav Sci. (2023) 13:592. doi: 10.3390/bs13070592
50. Seah, MLC, and Koh, KT. The efficacy of using mobile applications in changing adolescent girls’ physical activity behaviour during weekends. Eur Phys Educ Rev. (2021) 27:113–31. doi: 10.1177/1356336X20930741
51. Mendonça, G, Cheng, LA, Mélo, EN, and de Farias Júnior, JC. Physical activity and social support in adolescents: a systematic review. Health Educ Res. (2014) 29:822–39. doi: 10.1093/her/cyu017
52. Ferriz-Valero, A, Østerlie, O, García Martínez, S, and García-Jaén, M. Gamification in physical education: evaluation of impact on motivation and academic performance within higher education. Int J Environ Res Public Health. (2020) 17:4465. doi: 10.3390/ijerph17124465
53. Ryan, R, and Deci, E. The darker and brighter sides of human existence: basic psychological needs as a unifying concept. Psychol Inq. (2000) 11:319–38. doi: 10.1207/S15327965PLI1104_03
54. Sirriyeh, R, Lawton, R, and Ward, J. Physical activity and adolescents: An exploratory randomized controlled trial investigating the influence of affective and instrumental text messages. Br J Health Psychol. (2010) 15:825–40. doi: 10.1348/135910710X486889
55. Benítez-Porres, J, Alvero-Cruz, JR, Sardinha, LB, López-Fernández, I, and Carnero, EA. Cut-off values for classifying active children and adolescentes using the physical activity questionnaire: PAQ-C and PAQ-A. Nutr Hosp. (2016) 33:1036–44. doi: 10.20960/nh.564
56. Janz, KF, Lutuchy, EM, Wenthe, P, and Levy, SM. Measuring activity in children and adolescents using self-report. Med Sci Sports Exerc. (2008) 40:767–72. doi: 10.1249/MSS.0b013e3181620ed1
57. Martínez-López, EJ, López-Serrano, S, De La Torre-Cruz, M, and Ruiz-Ariza, A. Effects of the augmented reality game Pokémon GO on fitness and fatness in secondary school students. Health Educ J. (2022) 81:54–68. doi: 10.1177/00178969211047800
58. Mateo-Orcajada, A, Vaquero-Cristóbal, R, and Abenza-Cano, L. Importance of training volume through the use of step trackers apps promoted from the subject of physical education to change body composition, physical fitness and physical activity in adolescents and the influence of gender. Physiol Behav. (2024) 273:114402. doi: 10.1016/j.physbeh.2023.114402
59. Albaladejo-Saura, M, Vaquero-Cristóbal, R, González-Gálvez, N, and Esparza-Ros, F. Relationship between biological maturation, physical fitness, and Kinanthropometric variables of young athletes: a systematic review and meta-analysis. Int J Environ Res Public Health. (2021) 18:328. doi: 10.3390/ijerph18010328
60. Malina, RM, and Bouchard, C. Growth, maturation, and physical activity. Med Sci Sports Exerc. (1991) 24:841. doi: 10.1249/00005768-199207000-00018
61. Mateo-Orcajada, A, Abenza-Cano, L, Albaladejo-Saura, MD, and Vaquero-Cristóbal, R. Mandatory after-school use of step tracker apps improves physical activity, body composition and fitness of adolescents. Educ Inf Technol. (2023) 28:10235–66. doi: 10.1007/s10639-023-11584-0
62. Schulz, KF, Altman, DG, and Moher, D. CONSORT 2010 statement: updated guidelines for reporting parallel group randomised trials. Int J Surg. (2011) 9:672–7. doi: 10.1016/j.ijsu.2011.09.004
63. Bhalerao, S, and Kadam, P. Sample size calculation. Int J Ayurveda Res. (2010) 1:55–7. doi: 10.4103/0974-7788.59946
64. Direito, A, Jiang, Y, Whittaker, R, and Maddison, R. Apps for IMproving FITness and increasing physical activity among young people: the AIMFIT pragmatic randomized controlled trial. J Med Internet Res. (2015) 17:e210–3. doi: 10.2196/jmir.4568
65. Kowalski, KC, Crocker, PRE, Columbia, B, and Donen, RM. The physical activity questionnaire for older children (PAQ-C) and adolescents (PAQ-A) manual. (2004). Available at: https://www.researchgate.net/publication/228441462
66. Martínez-Gómez, D, Martínez-de-Haro, V, Pozo, T, Welk, GJ, Villagra, A, Calle, ME, et al. Fiabilidad y validez del cuestionario de actividad física PAQ-A en adolescentes españoles. Rev Esp Salud Publica. (2009) 83:427–39. doi: 10.1590/s1135-57272009000300008
67. Serra-Majem, L, Ribas, L, Ngo, J, Ortega, RM, García, A, Pérez-Rodrigo, C, et al. Food, youth and the Mediterranean diet in Spain: development of KIDMED, Mediterranean diet quality index in children and adolescents. Public Health Nutr. (2004) 7:931–5. doi: 10.1079/PHN2004556
68. Esparza-Ros, F, Vaquero-Cristo, R, and Marfell-Jones, M. International standars for anthropometric assessment. International Society for the Advancement of Kinanthropometry. (2019). pp. 1–154.
69. Poortmans, JR, Boisseau, N, Moraine, J-J, Moreno-Reyes, R, and Goldman, S. Estimation of total-body skeletal muscle mass in children and adolescents. Med Sci Sports Exerc. (2005) 37:316–22. doi: 10.1249/01.MSS.0000152804.93039.CE
70. Slaughter, MH, Lohman, TG, Boileau, RA, Horswill, CA, Stillman, RJ, Van Loan, MD, et al. Skinfold equations for estimations of body fatness in children and youth. Hum Biol. (1988) 60:709–23.
71. Mirwald, RL, Baxter-Jones, AD, Bailey, DA, and Beunen, GP. An assessment of maturity from anthropometric measurements. Med Sci Sports Exerc. (2002) 34:689–94. doi: 10.1097/00005768-200204000-00020
72. Léger, LA, Mercier, D, Gadoury, C, and Lambert, J. The multistage 20 metre shuttle run test for aerobic fitness. J Sports Sci. (1988) 6:93–101. doi: 10.1080/02640418808729800
73. Tomkinson, GR, Lang, JJ, Blanchard, J, Léger, LA, and Tremblay, MS. The 20-m shuttle run: assessment and interpretation of data in relation to youth aerobic fitness and health. Pediatr Exerc Sci. (2019) 31:152–63. doi: 10.1123/pes.2018-0179
74. Matsudo, VKR, Matsudo, SM, LFM, R, and Raso, V. Força de preensão manual como preditor de aptidão física em crianças e adolescentes. Rev Bras Cineantrop Desemp Hum. (2014) 17:1–10. doi: 10.5007/1980-0037.2015v17n1p1
75. España-Romero, V, Ortega, FB, Vicente-Rodríguez, G, Artero, EG, Rey, JP, and Ruiz, JR. Elbow position affects handgrip strength in adolescents: validity and reliability of Jamar, DynEx, and TKK dynamometers. J Strength Cond Res. (2010) 24:272–7. doi: 10.1519/JSC.0b013e3181b296a5
76. Castro-Piñero, J, Ortega, FB, Artero, EG, Girela-Rejón, MJ, Mora, J, Sjöström, M, et al. Assessing muscular strength in youth: usefulness of standing long jump as a general index of muscular fitness. J Strength Cond Res. (2010) 24:1810–7. doi: 10.1519/JSC.0b013e3181ddb03d
77. Barker, LA, Harry, JR, and Mercer, JA. Relationships between countermovement jump ground reaction forces and jump height, reactive strength index, and jump time. J Strength Cond Res. (2018) 32:248–54. doi: 10.1519/JSC.0000000000002160
78. Garcia-Pastor, T, Salinero, JJ, Sanz-Frias, D, Pertusa, G, and Del Coso, J. Body fat percentage is more associated with low physical fitness than with sedentarism and diet in male and female adolescents. Physiol Behav. (2016) 165:166–72. doi: 10.1016/j.physbeh.2016.07.016
79. Coburn, JW, and Malek, MH. Manual NSCA Fundamentos del entrenamiento personal. Barcelona, Spain: Paidotribo (2016).
80. Siklar, Z, Sanli, E, Dallar, Y, and Tanyer, G. Diurnal variation of height in children. Pediatr Int. (2005) 47:645–8. doi: 10.1111/j.1442-200x.2005.02144.x
81. Lampl, M . Further observations on diurnal variation in standing height. Ann Hum Biol. (1992) 19:87–90. doi: 10.1080/03014469200001952
82. Lauricella, AR, Cingel, DP, Blackwell, C, Wartella, E, and Conway, A. The mobile generation: youth and adolescent ownership and use of new media. Commun Res Rep. (2014) 31:357–64. doi: 10.1080/08824096.2014.963221
83. Adamczyk, M, Adamczyk, A, and Tłuściak-Deliowska, A. Using mobile phones by young people: the trends and risk of addiction. Int J Psychoeduc Sci. (2018) 7:29–41.
84. Bondaronek, P, Alkhaldi, G, Slee, A, Hamilton, FL, and Murray, E. Quality of publicly available physical activity apps: review and content analysis. JMIR Mhealth Uhealth. (2018) 6:e53. doi: 10.2196/mhealth.9069
85. Schochet, PZ . Analyzing grouped administrative data for RCTs using design-based methods. J Educ Behav Stat. (2019) 45:32–57. doi: 10.3102/1076998619855350
86. van de Kop, JH, van Kernebeek, WG, Otten, RHJ, Toussaint, HM, and Verhoeff, AP. School-based physical activity interventions in prevocational adolescents: a systematic review and meta-analyses. J Adolesc Health. (2019) 65:185–94. doi: 10.1016/j.jadohealth.2019.02.022
87. Lubans, DR, Plotnikoff, RC, Miller, A, Scott, JJ, Thompson, D, and Tudor-Locke, C. Using pedometers for measuring and increasing physical activity in children and adolescents. Am J Lifestyle Med. (2015) 9:418–27. doi: 10.1177/1559827614537774
88. Morency, C, Demers, M, and Lapierre, L. How many steps do you have in reserve? Trans Res Rec. (2007) 2002:1–6. doi: 10.3141/2002-01
89. Lee, JE, Zeng, N, Oh, Y, Lee, D, and Gao, Z. Effects of Pokémon GO on physical activity and psychological and social outcomes: a systematic review. J Clin Med. (2021) 10:1860. doi: 10.3390/jcm10091860
90. Khamzina, M, Parab, KV, An, R, Bullard, T, and Grigsby-Toussaint, DS. Impact of Pokémon go on physical activity: a systematic review and Meta-analysis. Am J Prev Med. (2020) 58:270–82. doi: 10.1016/j.amepre.2019.09.005
91. Corder, K, Schiff, A, Kesten, JM, and van Sluijs, EMF. Development of a universal approach to increase physical activity among adolescents: the GoActive intervention. BMJ Open. (2015) 5:e008610. doi: 10.1136/bmjopen-2015-008610
92. Mateo-Orcajada, A . Promoting aerobic physical activity of adolescents aged 12 to 16 years old during after-school hours using mobile applications: a critical review. Cult Cien Depor. (2024) 19:3–15. doi: 10.12800/ccd.v19i59.2169
93. Hopkins, WG, Marshall, SW, Batterham, AM, and Hanin, J. Progressive statistics for studies in sports medicine and exercise science. Med Sci Sports Exerc. (2009) 41:3–12. doi: 10.1249/MSS.0b013e31818cb278
94. Rauner, A, Mess, F, and Woll, A. The relationship between physical activity, physical fitness and overweight in adolescents: a systematic review of studies published in or after 2000. BMC Pediatr. (2013) 13:1–9. doi: 10.1186/1471-2431-13-19
95. Kelley, GA, Kelley, KS, and Pate, RR. Exercise and adiposity in overweight and obese children and adolescents: a systematic review with network meta-analysis of randomised trials. BMJ Open. (2019) 9:e031220. doi: 10.1136/bmjopen-2019-031220
96. Fernhall, B . The young athlete with a mental disability. In: Hebestreit H, Bar-Or O, editors. Young Athlete. (2007) New Jersey: Wiley. pp. 403–12.
97. Clark, PA, and Rogol, AD. Growth hormones and sex steroid interactions at puberty. Endocrinol Metab Clin N Am. (1996) 25:665–81. doi: 10.1016/S0889-8529(05)70346-7
98. Swain, M, Kamper, SJ, Maher, CG, Broderick, C, McKay, D, and Henschke, N. Relationship between growth, maturation and musculoskeletal conditions in adolescents: a systematic review. Br J Sports Med. (2018) 52:1246–52. doi: 10.1136/bjsports-2017-098418
99. Vanderschueren, D, Laurent, MR, Claessens, F, Gielen, E, Lagerquist, MK, Vandenput, L, et al. Sex steroid actions in male bone. Endocr Rev. (2014) 35:906–60. doi: 10.1210/er.2014-1024
100. Xu, Y, Wen, Z, Deng, K, Li, R, Yu, Q, and Xiao, S-M. Relationships of sex hormones with muscle mass and muscle strength in male adolescents at different stages of puberty. PLoS One. (2021) 16:e0260521–14. doi: 10.1371/journal.pone.0260521
101. Mateo-Orcajada, A, Vaquero-Cristóbal, R, Esparza-Ros, F, and Abenza-Cano, L. Physical, psychological, and body composition differences between active and sedentary adolescents according to the “fat but fit” paradigm. Int J Environ Res Public Health. (2022) 19:10797. doi: 10.3390/ijerph191710797
102. Gerber, BP, Pienaar, AE, and Kruger, A. A longitudinal study of growth patterns in stature, mass, arm span, sitting height and sitting height ratio of boys during the mid-adolescence phase. S Afr J Res Sport Phys Educ Recreat. (2015) 37:13–30.
103. Beunen, G, and Malina, RM. Growth and physical performance relative to the timing of the adolescent spurt. Exerc Sport Sci Rev. (1988) 16:503???540. doi: 10.1249/00003677-198800160-00018
104. Beunen, GP, Rogol, AD, and Malina, RM. Indicators of biological maturation and secular changes in biological maturation. Food Nutr Bull. (2006) 27:S244–56. doi: 10.1177/15648265060274s508
105. Tanner, JM . Fetus into man: physical growth from conception to maturity. Cambridge, Massachusetts, Estados Unidos: Harvard University Press (1990).
106. Kelch, RP, and Beitins, IZ. Adolescent sexual development In: MS Kappy, RM Blizzard, and CJ Migeon, editors. The diagnosis and treatment of endocrine disorders in chilhood and adolescence. Springfield, Illinois: Charles C Thomas Pub Ltd. (1994). 193–234.
107. Al-Mashaqbah, SF, Al-Zyoud, W, and Al-Zaben, A. Utilizing upper arm images in determining body mass index and nutritional status. Eng Technol Appl Sci Res. (2019) 9:4392–6. doi: 10.48084/etasr.2858
108. Marra, M, Sammarco, R, De Lorenzo, A, Iellamo, F, Siervo, M, Pietrobelli, A, et al. Assessment of body composition in health and disease using bioelectrical impedance analysis (BIA) and dual energy X-ray absorptiometry (DXA): a critical overview. Contrast Media Mol Imaging. (2019) 2019:1–9. doi: 10.1155/2019/3548284
109. Soo Choi, J, Kim, H, Lim, J, and Seok, RJ. The facilitation of trunk muscles by abdominal bracing during walking in chronic low back pain patients. J Biomech. (2022) 143:111299. doi: 10.1016/j.jbiomech.2022.111299
110. Shnayderman, I, and Katz-Leurer, M. An aerobic walking programme versus muscle strengthening programme for chronic low back pain: a randomized controlled trial. Clin Rehabil. (2013) 27:207–14. doi: 10.1177/0269215512453353
111. Bartol, V, Vauhnik, R, and Rugelj, D. Influence of the sport specific training background on the symmetry of the single legged vertical counter movement jump among female ballet dancers and volleyball players. Heliyon. (2022) 8:e10669. doi: 10.1016/j.heliyon.2022.e10669
112. Bosco, C, Luhtanen, P, and Komi, PV. A simple method for measurement of mechanical power in jumping. Eur J Appl Physiol Occup Physiol. (1983) 50:273–82. doi: 10.1007/BF00422166
113. De Almeida-Neto, PF, de Matos, DG, Pinto, VCM, Dantas, PMS, Bulhões-Correia, A, Aidar, FJ, et al. Can the neuromuscular performance of young athletes be influenced by hormone levels and different stages of puberty? Int J Environ Res Public Health. (2020) 17:5637. doi: 10.3390/ijerph17165637
114. Ulfa, M, Setyonugroho, W, Lestari, T, Widiasih, E, and Nguyen, QA. Nutrition-related Mobile application for daily dietary self-monitoring. J Nutr Metab. (2022) 2022:1–11. doi: 10.1155/2022/2476367
115. Mateo-Orcajada, A, Vaquero-Cristóbal, R, and Abenza-Cano, L. Mobile application interventions to increase physical activity and their effect on kinanthropometrics, body composition and fitness variables in adolescent aged 12–16 years old: An umbrella review. Child Care Health Dev. (2023) 50:e13146. doi: 10.1111/cch.13146
116. Meng, C, Yucheng, T, Shu, L, and Yu, Z. Effects of school-based high-intensity interval training on body composition, cardiorespiratory fitness and cardiometabolic markers in adolescent boys with obesity: a randomized controlled trial. BMC Pediatr. (2022) 22:112. doi: 10.1186/s12887-021-03079-z
117. Peral-Suárez, Á, Cuadrado-Soto, E, Perea, JM, Navia, B, López-Sobaler, AM, and Ortega, RM. Physical activity practice and sports preferences in a group of Spanish schoolchildren depending on sex and parental care: a gender perspective. BMC Pediatr. (2020) 20:337. doi: 10.1186/s12887-020-02229-z
118. Mateo-Orcajada, A, Vaquero-Cristóbal, R, and Abenza-Cano, L. Gender and academic year as moderators of the efficacy of mobile app interventions to promote physical activity in adolescents: a randomized controlled trial. Humanit Soc Sci Commun. (2023) 10:980. doi: 10.1057/s41599-023-02502-3
119. Albaladejo-Saura, M, Vaquero-Cristóbal, R, García-Roca, JA, and Esparza-Ros, F. Influence of maturity status on Kinanthropometric and physical fitness variables in adolescent female volleyball players. Appl Sci. (2022) 12:4400. doi: 10.3390/app12094400
120. Albaladejo-Saura, M, Vaquero-Cristóbal, R, García-Roca, JA, and Esparza-Ros, F. Influence of biological maturation status on selected anthropometric and physical fitness variables in adolescent male volleyball players. PeerJ. (2022) 10:e13216. doi: 10.7717/peerj.13216
121. Albaladejo-Saura, M, Vaquero-Cristóbal, R, García-Roca, JA, and Esparza-Ros, F. The effect of age, biological maturation and birth quartile in the Kinanthropometric and physical fitness differences between male and female adolescent volleyball players. Children. (2022) 9:58. doi: 10.3390/children9010058
122. Handelsman, DJ, Hirschberg, AL, and Bermon, S. Circulating testosterone as the hormonal basis of sex differences in athletic performance. Endocr Rev. (2018) 39:803–29. doi: 10.1210/er.2018-00020
123. Mendoza-Muñoz, M, Adsuar, JC, Pérez-Gómez, J, Muñoz-Bermejo, L, Garcia-Gordillo, MÁ, and Carlos-Vivas, J. Influence of body composition on physical fitness in adolescents. Medicina. (2020) 56:328. doi: 10.3390/medicina56070328
124. Tran, S, Smith, L, El-Den, S, and Carter, S. The use of gamification and incentives in Mobile health apps to improve medication adherence: scoping review. JMIR Mhealth Uhealth. (2022) 10:e30671. doi: 10.2196/30671
125. Litvin, S, Saunders, R, Maier, MA, and Lüttke, S. Gamification as an approach to improve resilience and reduce attrition in mobile mental health interventions: a randomized controlled trial. PLoS One. (2020) 15:e0237220. doi: 10.1371/journal.pone.0237220
Keywords: adolescents, anthropometry, body composition, gender, healthy lifestyle, mobile phone, mobile application, physical activity
Citation: Gómez-Cuesta N, Mateo-Orcajada A, Meroño L, Abenza-Cano L and Vaquero-Cristóbal R (2024) A mobile app-based intervention improves anthropometry, body composition and fitness, regardless of previous active-inactive status: a randomized controlled trial. Front. Public Health. 12:1380621. doi: 10.3389/fpubh.2024.1380621
Received: 08 February 2024; Accepted: 31 July 2024;
Published: 12 August 2024.
Edited by:
Behzad Behzadnia, University of Tabriz, IranReviewed by:
Regien Biesma, University Medical Center Groningen, NetherlandsCopyright © 2024 Gómez-Cuesta, Mateo-Orcajada, Meroño, Abenza-Cano and Vaquero-Cristóbal. This is an open-access article distributed under the terms of the Creative Commons Attribution License (CC BY). The use, distribution or reproduction in other forums is permitted, provided the original author(s) and the copyright owner(s) are credited and that the original publication in this journal is cited, in accordance with accepted academic practice. No use, distribution or reproduction is permitted which does not comply with these terms.
*Correspondence: Adrián Mateo-Orcajada, YW1hdGVvNUB1Y2FtLmVkdQ==
†These authors have contributed equally to this work
Disclaimer: All claims expressed in this article are solely those of the authors and do not necessarily represent those of their affiliated organizations, or those of the publisher, the editors and the reviewers. Any product that may be evaluated in this article or claim that may be made by its manufacturer is not guaranteed or endorsed by the publisher.
Research integrity at Frontiers
Learn more about the work of our research integrity team to safeguard the quality of each article we publish.