- 1Department of Psychology, College of Education and Behavorial Sciences, Jimma University, Jimma, Ethiopia
- 2REVAL Rehabilitation Research Center, Faculty of Rehabilitation Sciences, Hasselt University, Diepenbeek, Belgium
- 3Department of Physiotherapy, Faculty of Medical Sciences, Institute of Health, Jimma University, Jimma, Ethiopia
- 4Department of Special Needs and Inclusive Education, Jimma University, Jimma, Ethiopia
- 5Technology-Supported and Data-Driven Rehabilitation, Data Sciences Institute, Hasselt University, Diepenbeek, Belgium
- 6Centre of Expertise in Care Innovation, Department of PXL–Healthcare, PXL University of Applied Sciences and Arts, Hasselt, Belgium
1 Introduction
Cognitive assessment plays a crucial role in understanding cognitive functioning and detecting impairments or changes in cognitive abilities (1). It serves as an invaluable tool in evaluating an individual's cognitive status, aiding in clinical diagnoses, monitoring treatment progress, and informing intervention strategies (2). In low- and middle-income countries (LMICs), where a significant portion of the global population resides, access to reliable cognitive assessment tools is essential for identifying cognitive impairments and promoting cognitive wellbeing (3, 4).
However, the existing cognitive assessment tools used in LMICs are often limited by their heavy reliance on language, education and cultural factors (5). Traditional assessment methods typically involve standardized tests and questionnaires that are influenced by the educational background and cultural context of the individuals being assessed. These tools may inadvertently introduce bias and hinder accurate evaluation, particularly in populations with limited educational opportunities or diverse cultural backgrounds (6). This is particularly concerning given that a low level of education is associated with an increased risk of dementia, especially in LMICs (7).
The reliance on education and culture as prerequisites for cognitive assessment poses significant challenges in LMICs. Limited access to quality education and variations in cultural norms and practices can greatly impact individuals' performance on cognitive tests (8, 9). This dependence exacerbates disparities in assessing cognitive abilities, further worsening health inequalities (10, 11).
Another potential problem arises from the disparities in medical resources and access to healthcare workers in various countries, particularly in relation to mental health. There is an unequal distribution of professionally trained health workers globally, with countries facing higher disease burdens having significantly fewer resources. For instance, the African continent, experiencing 24% of the world's disease burden, struggles with access to merely 3% of trained health workers and < 1% of global financial resources (12). While the allocation of resources primarily favors infectious diseases, minimal attention is given to mental health, including cognitive disorders like dementia. Furthermore, considering mental health, other factors may interfere the diagnosis and management such as stigma and awareness (13), the limited infrastructure (associated with lack of human resources as already mentioned) (14), and the low funding and policy priority (15). African civilizations stigmatize mental health disorders, resulting in low awareness, therefore, mental health services are underutilized because stigma hinders people from seeking help (16). The lack of comprehensive policies and frameworks hinders mental health care development resulting in very limited hospitals and community centers tackling mental health issues. Furthermore, these services are often concentrated in cities, making rural access problematic. To address this health inequality, technological advancements, particularly mobile phone penetration, present an opportunity. Even in LMICs, mobile phone usage is prevalent, which can facilitate access to diagnostic and treatment applications for various physical and mental health concerns, including dementia (17).
Therefore, the objective of this paper is to emphasize the urgent need for the development of automated cognitive assessment methods that are culture-fair and demand only limited education in LMICs. By exploring alternative approaches that overcome the limitations of existing assessment tools, we aim to promote equitable access to accurate cognitive assessment, leading to more effective interventions and improved cognitive health outcomes for individuals in LMIC settings.
In the following sections, we will discuss the limitations of current assessment tools in more detail, examine the potential benefits of culture-fair and demanding only limited education automated cognitive assessment, and propose a framework for developing such tools in LMICs. Ultimately, our goal is to empower healthcare practitioners, researchers, and policymakers in LMICs to address the pressing cognitive health needs of their populations effectively.
2 Challenges in implementing traditional cognitive assessment in LMICs
LMICs face several challenges when implementing traditional cognitive assessment tools, impairing their ability to accurately evaluate cognitive functioning and identify impairments. These challenges include education disparities, cultural bias, language and communication barriers, and disparities in healthcare and resource availability.
2.1 Educational disparities
In many LMICs, there are significant educational disparities, with limited access to quality education, particularly in rural or marginalized communities (18). Traditional cognitive assessment tools often rely on literacy, numeracy, and educational background, making them inadequate for individuals with limited education. This creates a barrier to accurate assessment and leads to underdiagnosis or misdiagnosis of cognitive impairments (19).
2.2 Cultural bias
Cultural factors strongly influence cognitive processes and behavior. Traditional cognitive assessment tools developed in Western settings may not adequately account for the cultural diversity present in LMICs (20). Culturally biased test items and assessment procedures can lead to unfair evaluations, failing to accurately reflect individuals' cognitive abilities and impairments. For example, western examinations use English and use idioms and references recognizable to native English speakers. The Mini-Mental State Examination (MMSE) may use idioms like “a rolling stone gathers no moss,” which may confuse non-Westerners. Reading comprehension and numerical literacy are needed for tests like the Montreal Cognitive Assessment (MoCA) and MMSE. In areas with low literacy rates or educational backgrounds that differ from Western norms, test-takers may fail with these activities due to a lack of formal education. Some examinations use visual stimuli that may not be culturally meaningful across varied groups. Pattern recognition tasks containing Western symbols like clocks or animals may be alien to those from other cultures, resulting in inferior performance. Other limitations included the use of culturally specific terms or lists for memory tests. Words associated with Western food or daily objects may not resonate with people from different cultures, causing misunderstandings or poor memory performance without cognitive deficiencies.
This may undermine the validity and reliability of cognitive assessments in diverse populations.
2.3 Language and communication barriers
Many traditional cognitive assessment tools are administered in specific languages that might not be the primary language spoken by individuals in LMICs (21). Language barriers limit effective communication during assessments, resulting in compromised test performance and inaccurate cognitive evaluations (22).
2.4 Disparities in healthcare and resource availability
Healthcare disparities in LMICs, including limited funding, inadequate infrastructure, and a shortage of trained healthcare professionals, significantly impact the availability and accessibility of reliable cognitive assessment tools. The lack of resources hinders the implementation of comprehensive cognitive assessments, leaving many individuals without access to proper evaluation and subsequent interventions.
Furthermore, the availability of standardized assessment tools is often limited in LMICs due to cost, licensing, and language barriers. Many traditional cognitive assessments are developed and marketed primarily for high-income countries, making them less accessible and affordable for LMICs. This further widens the gap in cognitive healthcare services, depriving vulnerable populations of essential cognitive assessments.
The combination of educational disparities, cultural bias, and limited healthcare resources in LMICs creates a complex environment that poses substantial challenges to the implementation of traditional cognitive assessment tools. Addressing these challenges is critical to ensure equitable access to reliable cognitive assessments and improve cognitive health outcomes in LMIC populations.
3 Existing automated cognitive assessment tools
Several promising tools are already available, or currently under validation for assessment of cognitive functions in LMICs.
3.1 Technology-based assessments
Digital platforms, such as smartphone applications and computer-based tests, offer the advantage of flexibility and scalability. These tools utilize interactive tasks, games, and simulations to assess cognitive abilities (23, 24), minimizing the dependence on educational and cultural background. Mobile technologies offer significant advantages in the realm of cognitive impairment assessment, enabling repeated and continuous evaluations while discreetly collecting additional behavioral markers. This enhanced approach allows users to observe patterns over time and promptly detect acute changes that have historically posed challenges for identification. The potential opportunities in this domain encompass the utilization of cutting-edge mobile sensors and wearable devices, ensuring enhanced reliability and validity of mobile assessments. Moreover, there is a need to establish appropriate clinical applications for mobile assessment data and integrate person-centered assessment principles alongside digital phenotyping methodologies. Embracing these advancements holds the potential to revolutionize cognitive impairment assessment and support more personalized and effective healthcare approaches (25).
3.2 Non-verbal assessments
Non-verbal assessments, such as visual-spatial tasks, pattern recognition, and cognitive games, provide a means of evaluating cognitive functioning without relying heavily on language or literacy skills (26). These assessments can be particularly useful in populations with limited education or diverse linguistic backgrounds.
3.3 Performance-based measures
Performance-based measures focus on evaluating an individual's actual abilities and skills rather than relying on self-reported or subjective measures. These measures include objective performance tasks that assess cognitive domains such as attention, memory, executive function, and processing speed (27).
3.4 Limitations and applicability of tools in LMICs
While these culture-fair and limited education demanding assessment tools show promise, their applicability in LMICs is not without limitations and need consideration of several factors.
In the context of administering assessment tools, particularly in LMICs, it is crucial to consider the role of evaluators. The person responsible for evaluating individuals using these tools is often a trained health care worker from the community, rather than a professional healthcare worker specialized in cognition.
The widespread implementation of technology-based assessments relies on the availability of reliable internet connectivity and access to appropriate devices. In resource-constrained settings, particularly in rural areas of LMICs, limited technological infrastructure may hinder the widespread adoption of these tools (28–30).
Adapting assessment tools to different cultural contexts is essential to ensure their validity and reliability. The tools should be sensitive to cultural nuances, norms, and values has these parameters significantly influence cognitive assessment Some of the most important parameters included language and interpretation, conceptual understanding, time perception, and social desirability bias (31). For example, even with literal translations, language interpretation can vary. Verbal-based examinations may misread Western words, phrases, and idioms because they may not have equivalents in other languages (20). Secondly, colors, shapes, and items can have different meanings and various symbolic meanings throughout cultures, which may affect cognitive evaluation responses to visual stimuli. Another important aspect is that some indigenous and African civilizations view time cyclically or focus on the present, while Western societies view time linearly and forward. Planning, time estimation, and sequencing assessments can be affected by time perception. Finally, many Asian cultures respect authority, which might show in subtle or indirect communication. This may cause people to give answers they think the assessor wants, skewing cognitive testing results (32).
Efforts should be made to validate and adapt these tools across diverse populations, establishing their applicability in different cultural settings. This includes the development of normative data specific to various LMIC populations.
While non-verbal assessments reduce the reliance on language, literacy skills may still play a role in some tools. Adapting assessments to different languages and ensuring they are accessible to individuals with varying literacy levels is crucial for accurate cognitive evaluations.
3.5 Gaps in the current landscape of automated cognitive assessment for LMICs
The current landscape of automated cognitive assessment tools for LMICs reveals several gaps.
First, many of the tools reviewed lack extensive validation in LMIC settings. Hence, robust validation studies are needed to establish the reliability, validity, and cross-cultural applicability of these new informatics tools in diverse populations within LMICs. For example, even if digital health solutions have shown promising results in improving health outcomes through enhanced patient engagement and adherence to prescribed therapies (33), several factors limiting the implementation of such solutions have been identified. Challenges include technological infrastructure, cultural acceptance, and the need for training among healthcare providers. Second, normative data specific to LMICs are lacking and need to be developed. This is crucial for accurate interpretation of assessment results. Population-specific norms accounting for cultural, educational, and socioeconomic factors need to be established to ensure appropriate comparisons and interpretation of cognitive assessment outcomes. Finally, the scalability and affordability of these tools in LMICs need to be considered. Ensuring that the tools are cost-effective, easily accessible, and compatible with the available resources is vital for their implementation and sustainability in LMIC settings.
4 Strategies for developing automated cognitive assessment tools
Developing assessment tools that are culture-fair and demand only limited education requires careful consideration and collaboration among researchers, practitioners, and stakeholders (34, 35). Table 1 presents potential strategies for achieving this goal. By employing these strategies, we can develop culture-fair and limited education-demanding assessment tools that accurately evaluate cognitive functioning across diverse populations in LMICs. These tools will promote equitable access to reliable cognitive assessments, leading to improved healthcare outcomes, early detection of cognitive impairments, and targeted interventions tailored to the needs of individuals in LMIC settings (36).
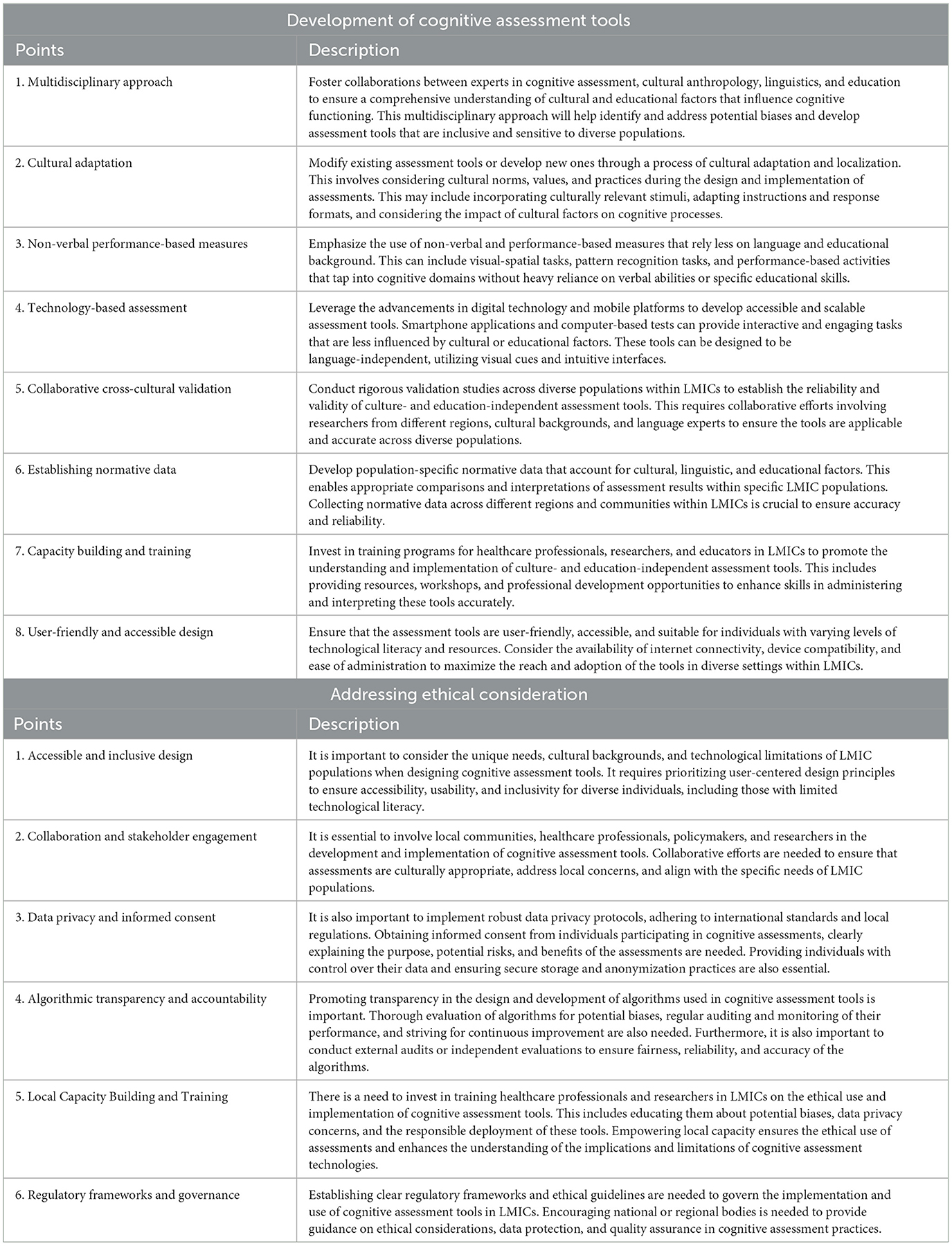
Table 1. Various strategies for (1) developing automated cognitive assessment tools in LMICs and (2) address ethical and implementation challenges.
5 Ethical considerations
The ethical considerations associated with creating and using cognitive assessment tools that are fair across different cultures and need minimal instruction are of utmost importance in LMICs due to several reasons if we consider that health is a human right, therefore according to Ooms et al. “all people, regardless of where they live, should be entitled to the same collective efforts that can protect or improve their health” (37). The current dependence on conventional assessment techniques, which are frequently impacted by cultural and educational variables, maintains inequalities and undermines the precision of cognitive evaluations, giving rise to ethical problems regarding impartiality and fairness. The ethical obligation to ensure fair and consistent access to dependable cognitive evaluations is highlighted as a fundamental human entitlement, in line with the principle that every person, regardless of their location, should have access to collaborative endeavors that safeguard or enhance their wellbeing. Utilizing technology-based examinations provides a chance to tackle these ethical concerns, fostering equity by mitigating cultural bias and reliance on educational factors. Nevertheless, the use of these tools presents actual ethical dilemmas, encompassing concerns of infrastructure, connection, data privacy, and potential biases in algorithmic design. When implementing these tools in LMICs, it is crucial to prioritize ethical measures such as providing fair access, obtaining informed consent, being culturally sensitive, and protecting data privacy. The ethical aspects of this undertaking emphasize the requirement for a deliberate and cooperative strategy, giving importance to impartiality, inclusiveness, and regard for the varied populations being evaluated.
5.1 Equity in healthcare
Culture-fair and limited education-demanding assessments promote equitable access to reliable cognitive evaluations across diverse populations. By reducing the influence of cultural and educational factors, these tools aim to minimize disparities and ensure that individuals from different backgrounds receive fair and accurate cognitive assessments (38).
5.2 Early detection and intervention
Timely identification of cognitive impairments is crucial for implementing effective targeted preventive measurements or interventions (39, 40). Digital assessments can enable early detection of cognitive changes, allowing for targeted interventions to mitigate the impact of cognitive impairments on individuals' lives, enhance quality of life and decrease family and healthcare costs, making it ethically important, as highlighted by the WHO (41). Furthermore, cognitive tests should be accessible and fair regardless of cultural background or academic level to uphold healthcare equity. All people have the same chance of early diagnosis and treatment.
5.3 Monitoring cognitive health
Ethically, care should be constant and continuous, including cognitive health monitoring (42, 43). Regular assessment of cognitive functioning can aid in monitoring the progression of cognitive disorders, tracking treatment effectiveness, and adjusting interventions accordingly. Regular assessments provide a standardized and objective means of monitoring cognitive health across diverse populations in LMICs (44, 45).
5.4 Potential biases in algorithmic design
Algorithmic bias can emerge if cognitive assessment tools are developed using datasets that are not representative of the diverse populations in LMICs. Biased algorithms may perpetuate inequalities, lead to misdiagnoses, or result in differential access to resources and interventions (46). Additionally, cultural biases in assessment tasks or scoring mechanisms can unfairly disadvantage certain groups.
6 Implementation challenges
The implementation of automated cognitive assessment tools in LMICs raises important practical considerations. Addressing these challenges is crucial to ensure the ethical and responsible deployment of cognitive assessment tools in LMICs.
6.1 Infrastructure and connectivity challenges
LMICs often face limitations in technological infrastructure and internet connectivity, particularly in rural or underserved areas. Implementing technology-based assessment tools requires reliable access to devices, internet connectivity, and a power supply (47). Unequal access to technology can exacerbate existing health disparities and impede the equitable distribution of cognitive assessments (48).
6.2 Data privacy and security
The collection, storage, and management of sensitive personal data during cognitive assessments raises concerns about data privacy and security. Safeguarding individuals' confidentiality and protecting their personal information are of paramount importance (49). Additionally, informed consent procedures should be robust, ensuring that individuals understand the purpose, risks, and benefits of cognitive assessments and have control over their data.
7 Measures to address ethical and implementation challenges
When developing novel solutions for cognitive assessment in LMICs, several crucial points must be taken into consideration. These points include accessible and inclusive design, collaboration and stakeholder engagement, data privacy and informed consent, algorithmic transparency and accountability, local capacity building and training, and the establishment of regulatory frameworks and governance, all of which are presented in Table 1. These considerations are essential to overcome challenges related to cultural, technological, and ethical factors in LMICs while ensuring the reliability and fairness of cognitive assessment tools.
8 Conclusion
Developing culture-fair and limited education demanding assessment tools for LMICs is vital for equitable access to reliable cognitive evaluations. Although promising tools have been identified, further research, validation, and adaptation are needed to address limitations and enable widespread implementation in LMIC settings.
These tools have the potential to significantly impact public health, enabling early detection and intervention for cognitive impairments. Timely identification allows for appropriate care, slowing cognitive decline, and easing the burden on healthcare systems.
Culture-fair and limited education demanding assessments also enhance accurate diagnosis and monitoring of cognitive disorders, tailoring interventions to individual needs in LMICs. This personalized approach leads to improved cognitive health outcomes.
To fully harness their potential, rigorous validation and collaboration between stakeholders are necessary. Adapting to diverse cultural contexts and developing population-specific norms ensures reliability and validity. Overcoming resource constraints and implementation challenges will ensure scalability and sustainability.
By embracing these tools, we advance public health, reduce disparities, and empower individuals to lead fulfilling lives in LMICs. Bridging the gap in cognitive assessment accessibility requires concerted efforts and a commitment to equity in healthcare.
Author contributions
DG: Writing – review & editing, Writing – original draft, Conceptualization. DL: Writing – review & editing, Supervision. TA: Writing – review & editing, Supervision. BB: Writing – review & editing, Writing – original draft, Supervision, Funding acquisition, Conceptualization.
Funding
The author(s) declare financial support was received for the research, authorship, and/or publication of this article. This study was supported by the NASCERE program.
Conflict of interest
The authors declare that the research was conducted in the absence of any commercial or financial relationships that could be construed as a potential conflict of interest.
The author(s) declared that they were an editorial board member of Frontiers, at the time of submission. This had no impact on the peer review process and the final decision.
Publisher's note
All claims expressed in this article are solely those of the authors and do not necessarily represent those of their affiliated organizations, or those of the publisher, the editors and the reviewers. Any product that may be evaluated in this article, or claim that may be made by its manufacturer, is not guaranteed or endorsed by the publisher.
References
1. Cleveland ML. Preserving cognition, preventing dementia. Clin Geriatr Med. (2020) 36:585–99. doi: 10.1016/j.cger.2020.06.003
2. Bu Z, Huang A, Xue M, Li Q, Bai Y, Xu G. Cognitive frailty as a predictor of adverse outcomes among older adults: a systematic review and meta-analysis. Brain Behav. (2021) 11:e01926. doi: 10.1002/brb3.1926
3. Hartle L, Charchat-Fichman H. Mild cognitive impairment history and current procedures in low- and middle-income countries: a brief review. Dement Neuropsychol. (2021) 15:155–63. doi: 10.1590/1980-57642021dn15-020001
4. Haile YG, Habatmu K, Derese A, Gouse H, Lawrie SM, Cella M, et al. Assessing cognition in people with severe mental disorders in low- and middle-income countries: a systematic review of assessment measures. Soc Psychiatry Psychiatr Epidemiol. (2022) 57:435–60. doi: 10.1007/s00127-021-02120-x
5. Ozawa S, Laing SK, Higgins CR, Yemeke TT, Park CC, Carlson R, et al. Educational and economic returns to cognitive ability in low- and middle-income countries: a systematic review. World Dev. (2022) 149:105668. doi: 10.1016/j.worlddev.2021.105668
6. Sania A, Sudfeld CR, Danaei G, Fink G, McCoy DC, Zhu Z, et al. Early life risk factors of motor, cognitive and language development: a pooled analysis of studies from low/middle-income countries. BMJ Open. (2019) 9:e026449. doi: 10.1136/bmjopen-2018-026449
7. Suemoto CK, Bertola L, Grinberg LT, Leite REP, Rodriguez RD, Santana PH, et al. Education, but not occupation, is associated with cognitive impairment: the role of cognitive reserve in a sample from a low-to-middle-income country. Alzheimers Dement. (2022) 18:2079–87. doi: 10.1002/alz.12542
8. Alsan M, Xing A, Wise P, Darmstadt GL, Bendavid E. Childhood illness and the gender gap in adolescent education in low- and middle-income countries. Pediatrics. (2017) 140:e20163175. doi: 10.1542/peds.2016-3175
9. Grande AJ, Hoffmann MS, Evans-Lacko S, Ziebold C, de Miranda CT, Mcdaid D, et al. Efficacy of school-based interventions for mental health problems in children and adolescents in low and middle-income countries: a systematic review and meta-analysis. Front Psychiatry. (2022) 13:1012257. doi: 10.3389/fpsyt.2022.1012257
10. Neves PAR, Barros AJD, Gatica-Domínguez G, Vaz JS, Baker P, Lutter CK. Maternal education and equity in breastfeeding: trends and patterns in 81 low- and middle-income countries between 2000 and 2019. Int J Equity Health. (2021) 20:20. doi: 10.1186/s12939-020-01357-3
11. Bornstein MH, Rothenberg WA, Lansford JE, Bradley RH, Deater-Deckard K, Bizzego A, et al. Child development in low- and middle-income countries. Pediatrics. (2021) 148:e2021053180. doi: 10.1542/peds.2021-053180
12. Global Health Observatory. Density of physicians (total number per 1000 population, latest available year). (2018). Available online at: https://www.who.int/gho/health_workforce/physicians_density_text/en/ (accessed June 5, 2019).
13. Thornicroft G, Sunkel C, Alikhon Aliev A, Baker S, Brohan E, El Chammay R, et al. The Lancet Commission on ending stigma and discrimination in mental health. Lancet. (2022) 400:1438–80. doi: 10.1016/S0140-6736(22)01470-2
14. Kleinitz P, Sabariego C, Llewellyn G, Taloafiri E, Mangar A, Baskota R, et al. Integrating rehabilitation into health systems: a comparative study of nine middle-income countries using WHO's systematic assessment of rehabilitation situation (STARS). PLoS One. (2024) 19:e0297109. doi: 10.1371/journal.pone.0297109
15. Wondimagegn D, Pain C, Seifu N, Cartmill C, Alemu AA, Whitehead CR. Reimagining global mental health in Africa. BMJ Glob Health. (2023) 8:e013232. doi: 10.1136/bmjgh-2023-013232
16. Daar AS, Jacobs M, Wall S, Groenewald J, Eaton J, Patel V, et al. Declaration on mental health in Africa: moving to implementation. Glob Health Action. (2014) 7:24589. doi: 10.3402/gha.v7.24589
17. Bonnechère B, Sahakian BJ. Can mobile technology help prevent the burden of dementia in low- and mid-income countries? Front Public Health. (2020) 8:554938. doi: 10.3389/fpubh.2020.554938
18. Local Burden of Disease Educational Attainment Collaborators. Mapping disparities in education across low- and middle-income countries. Nature. (2020) 577:235–238. doi: 10.1038/s41586-019-1872-1
19. Borda MG, Reyes-Ortiz C, Pérez-Zepeda MU, Patino-Hernandez D, Gómez-Arteaga C, Cano-Gutiérrez CA. Educational level and its Association with the domains of the Montreal Cognitive Assessment Test. Aging Ment Health. (2019) 23:1300–6. doi: 10.1080/13607863.2018.1488940
20. Khan G, Mirza N, Waheed W. Developing guidelines for the translation and cultural adaptation of the Montreal Cognitive Assessment: scoping review and qualitative synthesis. BJPsych Open. (2022) 8:e21. doi: 10.1192/bjo.2021.1067
21. Naqvi RM, Haider S, Tomlinson G, Alibhai S. Cognitive assessments in multicultural populations using the Rowland Universal Dementia Assessment Scale: a systematic review and meta-analysis. CMAJ. (2015) 187:E169–175. doi: 10.1503/cmaj.140802
22. Smith L, Leung WG, Crane B, Parkinson B, Toulopoulou T, Yiend J. Bilingual comparison of Mandarin and English cognitive bias tasks. Behav Res Methods. (2018) 50:302–12. doi: 10.3758/s13428-017-0871-0
23. Bonnechère B, Van Vooren M, Bier J-C, De Breucker S, Van Hove O, Van Sint Jan S, et al. The use of mobile games to assess cognitive function of elderly with and without cognitive impairment. J Alzheimers Dis. (2018) 64:1285–93. doi: 10.3233/JAD-180224
24. Bonnechère B, Bier J-C, Van Hove O, Sheldon S, Samadoulougou S, Kirakoya-Samadoulougou F, et al. Age-associated capacity to progress when playing cognitive mobile games: ecological retrospective observational study. JMIR Serious Games. (2020) 8:e17121. doi: 10.2196/17121
25. Koo BM, Vizer LM. Mobile technology for cognitive assessment of older adults: a scoping review. Innov Aging. (2019) 3:igy038. doi: 10.1093/geroni/igy038
26. Schumacher R, Halai AD, Lambon Ralph MA. Assessing and mapping language, attention and executive multidimensional deficits in stroke aphasia. Brain. (2019) 142:3202–16. doi: 10.1093/brain/awz258
27. Bonnechère B. Evaluation of processing speed of different cognitive functions across the life span using cognitive mobile games. Games Health J. (2022) 11:132–40. doi: 10.1089/g4h.2021.0144
28. Meherali S, Rahim KA, Campbell S, Lassi ZS. Does digital literacy empower adolescent girls in low- and middle-income countries: a systematic review. Front Public Health. (2021) 9:761394. doi: 10.3389/fpubh.2021.761394
29. Zhang M, Doi L, Awua J, Asare H, Stenhouse R. Challenges and possible solutions for accessing scholarly literature among medical and nursing professionals and students in low-and-middle income countries: a systematic review. Nurse Educ Today. (2023) 123:105737. doi: 10.1016/j.nedt.2023.105737
30. Guillaume D, Troncoso E, Duroseau B, Bluestone J, Fullerton J. Mobile-social learning for continuing professional development in low- and middle-income countries: integrative review. JMIR Med Educ. (2022) 8:e32614. doi: 10.2196/32614
31. Mungas D, Shaw C, Hayes-Larson E, DeCarli C, Farias ST, Olichney J, et al. Cognitive impairment in racially/ethnically diverse older adults: accounting for sources of diagnostic bias. Alzheimers Dement (Amst). (2021) 13:e12265. doi: 10.1002/dad2.12265
32. Rosli R, Tan MP, Gray WK, Subramanian P, Chin A-V. Cognitive assessment tools in Asia: a systematic review. Int Psychogeriatr. (2016) 28:189–210. doi: 10.1017/S1041610215001635
33. Bonnechère B, Kossi O, Mapinduzi J, Panda J, Rintala A, Guidetti S, et al. Mobile health solutions: an opportunity for rehabilitation in low- and middle income countries? Front Public Health. (2022) 10:1072322. doi: 10.3389/fpubh.2022.1072322
34. Sawatzky R, Kwon J-Y, Barclay R, Chauhan C, Frank L, van den Hout WB, et al. Implications of response shift for micro-, meso-, and macro-level healthcare decision-making using results of patient-reported outcome measures. Qual Life Res. (2021) 30:3343–57. doi: 10.1007/s11136-021-02766-9
35. Smith T, McNeil K, Mitchell R, Boyle B, Ries N. A study of macro-, meso- and micro-barriers and enablers affecting extended scopes of practice: the case of rural nurse practitioners in Australia. BMC Nurs. (2019) 18:14. doi: 10.1186/s12912-019-0337-z
36. Bates DW, Landman A, Levine DM. Health apps and health policy: what is needed? JAMA. (2018) 320:1975. doi: 10.1001/jama.2018.14378
37. Ooms G, Keygnaert I, Hammonds R. The right to health: from citizen's right to human right (and back). Public Health. (2019) 172:99–104. doi: 10.1016/j.puhe.2019.01.019
38. Chopra S, Kaur H, Pandey RM, Nehra A. Development of neuropsychological evaluation screening tool: an education-free cognitive screening instrument. Neurol India. (2018) 66:391–9. doi: 10.4103/0028-3886.227304
39. Carlsson CM. Management of dementia. Continuum (Minneap Minn). (2022) 28:885–900. doi: 10.1212/CON.0000000000001132
40. Livingston G, Huntley J, Sommerlad A, Ames D, Ballard C, Banerjee S, et al. Dementia prevention, intervention, and care: 2020 report of the Lancet Commission. Lancet. (2020) 396:413–46. doi: 10.1016/S0140-6736(20)30367-6
41. WHO. WHO Guidelines on Ethical Issues in Public Health Surveillance. (2017). Available online at: https://iris.who.int/bitstream/handle/10665/255721/9789241512657-eng.pdf?sequence=1 (accessed February 3, 2024)
42. Keenan AJ, Tsourtos G, Tieman J. Promise and peril-defining ethical telehealth practice from the clinician and patient perspective: a qualitative study. Digit Health. (2022) 8:20552076211070394. doi: 10.1177/20552076211070394
43. Gilmartin C, Arbe-Barnes EH, Diamond M, Fretwell S, McGivern E, Vlazaki M, et al. Varsity medical ethics debate 2018: constant health monitoring - the advance of technology into healthcare. Philos Ethics Humanit Med. (2018) 13:12. doi: 10.1186/s13010-018-0065-0
44. Chowdhary N, Barbui C, Anstey KJ, Kivipelto M, Barbera M, Peters R, et al. Reducing the risk of cognitive decline and dementia: WHO recommendations. Front Neurol. (2021) 12:765584. doi: 10.3389/fneur.2021.765584
45. Wang C, Song P, Niu Y. The management of dementia worldwide: A review on policy practices, clinical guidelines, end-of-life care, and challenge along with aging population. Biosci Trends. (2022) 16:119–29. doi: 10.5582/bst.2022.01042
46. Norori N, Hu Q, Aellen FM, Faraci FD, Tzovara A. Addressing bias in big data and AI for health care: A call for open science. Patterns. (2021) 2:100347. doi: 10.1016/j.patter.2021.100347
47. Byambasuren O, Sanders S, Beller E, Glasziou P. Prescribable mHealth apps identified from an overview of systematic reviews. NPJ Digital Med. (2018) 1:12. doi: 10.1038/s41746-018-0021-9
48. Saeed SA, Masters RM. Disparities in health care and the digital divide. Curr Psychiatry Rep. (2021) 23:61. doi: 10.1007/s11920-021-01274-4
Keywords: cognition, LMIC, assessment, digital health, public health
Citation: Garuma D, Lamba D, Abessa TG and Bonnechère B (2024) Advancing public health: enabling culture-fair and education-independent automated cognitive assessment in low- and middle-income countries. Front. Public Health 12:1377482. doi: 10.3389/fpubh.2024.1377482
Received: 27 January 2024; Accepted: 10 June 2024;
Published: 28 June 2024.
Edited by:
Edmund Howe, Uniformed Services University of the Health Sciences, United StatesReviewed by:
Adam Poulsen, The University of Sydney, AustraliaCopyright © 2024 Garuma, Lamba, Abessa and Bonnechère. This is an open-access article distributed under the terms of the Creative Commons Attribution License (CC BY). The use, distribution or reproduction in other forums is permitted, provided the original author(s) and the copyright owner(s) are credited and that the original publication in this journal is cited, in accordance with accepted academic practice. No use, distribution or reproduction is permitted which does not comply with these terms.
*Correspondence: Bruno Bonnechère, YnJ1bm8uYm9ubmVjaGVyZSYjeDAwMDQwO3VoYXNzZWx0LmJl