- 1School of Information Management, Nanjing University, Nanjing, China
- 2School of Management, Xuzhou Medical University, Nanjing, China
Introduction: During the COVID-19 pandemic, older adults were facing more mental health issues that may cause complex impacts on pandemic prevention, and turning to the internet for health information is a double-edged sword for them. This study aimed to investigate the reciprocal relationship between negative emotions and prevention behaviors in older adults, as well as the direct and moderating effects of online health information seeking (OHIS) on negative emotions and prevention behaviors.
Methods: Based on the common-sense model of self-regulation (CSM) and a sample of more than 20,000 participants from the Survey of Health, Aging and Retirement in Europe (SHARE), this study first used an autoregressive cross-lagged panel model (CLPM) to analyze the longitudinal effect of negative emotions on prevention behaviors. Second, the study used ordinary least squares (OLS) regression to explore the influence of OHIS usage frequency changes on negative emotions and prevention behaviors. Third, the study used multigroup analysis to examine the moderating effect of OHIS usage frequency changes on the CLPM.
Results: The findings indicate a significant longitudinal association where initial negative emotions predicted later prevention behaviors (β = 0.038, p < 0.001), and increased OHIS frequency was linked to positive changes in prevention behavior (β = 0.109, p < 0.001). Multigroup analysis revealed that the connection between negative emotions or increased negative emotions and prevention behaviors remained significant for those with no change or an increase in OHIS frequency but not for those with a decrease.
Conclusion: This study suggested that negative emotions may drive older adults to engage more in prevention behaviors and that OHIS can augment this effect. These results underscore the importance of addressing mental health and providing reliable online health information to support older adults in managing infectious disease risks.
1 Introduction
The COVID-19 pandemic had a significant impact on individuals worldwide, particularly on older adults, because the age group suffered a higher prevalence, severity, and mortality rate (1, 2). Moreover, the pandemic could lead to deterioration in the mental health of older adults due to anxiety about the lethality of COVID-19, restricted access to healthcare, and disruptions to their daily routines, which may have had complex impacts on pandemic prevention and control (3–5). In addition, studies have shown that proactive actions might mitigate mental distress during the early stage of the pandemic, and the effect was greater in older adults (6). However, as the pandemic continued, the utility of this solution seemed to diminish among older adults (7), and the social isolation caused by prevention behaviors (e.g., staying distant from others outside the home) might increase the negative emotions (e.g., depression, loneliness) of older adults (8). It has also been found that the characteristics of emotional and mental health problems vary at different stages of the pandemic (9). Thus, the long-term relationship between negative emotions and pandemic prevention behaviors is complex and worthy of further study. There are already some studies focusing on negative emotions and pandemic prevention behaviors. However, current research is mostly cross-sectional and rarely considers the reciprocal relationship between negative emotions and pandemic prevention behaviors from a longitudinal perspective.
The internet is an important source of health information for older adults and plays an important role in their health decision-making and self-caring (10, 11). During the pandemic, the desire for health information increased rapidly among older adults (12). They were increasingly turning to the internet for information about the pandemic not only for meeting their information needs but also for maintaining mental connections in coping with isolation and loneliness (13). However, due to lower familiarity with information and communications technology usage, as well as worse sensory and cognitive capabilities, there are some obstacles during the health information seeking and use process that may cause negative results. First, older adults may encounter misinformation or conflicting information online, which can further increase their stress and confusion (14, 15). In addition, constant exposure to news about the pandemic and its impact can lead to information overload and information avoidance (16), which further increases negative emotions and reluctance to engage in prevention behaviors (17). Therefore, it is necessary to explore the long-term effects of OHIS on negative emotions and pandemic prevention behaviors.
For the effect of OHIS on negative emotions and pandemic prevention behaviors, Pluye et al. (18) proposed a framework of health outcomes of online consumer health information based on a systematic review that showed that OHIS affects health behaviors through cognitive impacts. Previous research has treated worry and anxiety as mediators between OHIS and prevention behaviors and has suggested that OHIS can help prevent infections but also promote anxiety and fear, which may lead to other inappropriate behaviors (19, 20). However, few studies have investigated the influence of older adults’ OHIS on other relationships, and there is a scarcity of literature exploring the change in OHIS usage frequency at different stages of the pandemic. Therefore, clarifying the reciprocal relationship between negative emotions and prevention behaviors in older adults and the long-term effect of OHIS on them has important implications for future research and public interventions. Thus, the following research questions are proposed:
Question 1: What is the long-term reciprocal relationship between older adults’ negative emotions and their pandemic prevention behaviors?
Question 2: How does changes in older adults’ OHIS usage frequency influence changes in their negative emotions and pandemic prevention behaviors?
Question 3: What is the moderating effect of OHIS usage frequency changes between negative emotions and pandemic prevention behaviors?
2 Related works
2.1 Negative emotions and prevention behaviors
The psychological function of emotion is to motivate people to take action, and negative emotions (such as depression, nervousness and loneliness) can significantly contribute to the development or exacerbation of mental health problems (21, 22). According to theories such as the Protective Motivation Theory (PMT) (23), the Health Belief Model (24), and the Common-Sense Model of Self-Regulation (CSM) (25), negative emotion is usually related to threat perceptions and is an essential antecedent of prevention behaviors (26). In particular, CSM posits that when people are facing threats to their health, they tend to return to equilibrium by resolving the threat guided by illness representations and emotion representations (27). Moreover, CSM contains a “feedback loop” framework that describes the dynamic process by which individuals modify their emotional representations through an appraisal of coping strategies (28). In this study, coping strategies are represented by OHIS and prevention behavior, illness representation can be represented by subjective health and COVID-19 involvement, and emotional representation, which is our primary concern, can be represented by negative emotions. This study explored the relationship between emotional representation and two coping strategies. Figure 1 shows the theoretical framework of the feedback loops. Emotional illness representation directly affected coping strategies in wave 1 and indirectly affected coping strategies in wave 2 through emotional outcomes and coping appraisals. Prevention behavior in wave 1 will have an effect on prevention behavior in wave 2. As another coping strategy, OHIS can affect both negative emotion change and prevention behavior change and can moderate the relationship between negative emotion and prevention behavior by affecting coping appraisal.
Some studies have explored the relationship between negative emotions and prevention behaviors in the context of the COVID-19 pandemic. Dixon et al. (29) combined constructs from CSM, PMT, and other theories to measure adherence to prevention behaviors for 6 weeks and found worry to be a significant antecedent. Based on the knowledge-attitudes-practices model, Ning et al. (30) found that negative emotions are positively associated with COVID-19 prevention behaviors. In addition, studies using CSM have shown that negative emotions are important predictors of vaccination willingness (31), safety-seeking behaviors (32), and high levels of psychological distress, which drive people to search for health-related information online (33). In contrast, others have drawn opposite conclusions. Based on the PMT, Ezati Rad et al. (34) reported that fear negatively predicts protective behaviors. Alegria et al. (35) reported that negative emotions improve risky behaviors rather than prevention behaviors. In terms of older adults, there are fewer studies on this topic. Therefore, given the mixed findings in the literature and the characteristics of older adults, there is a knowledge gap regarding the reciprocal relationship between older adults’ negative emotions and prevention behaviors. This research aims to resolve these discrepancies from a longitudinal perspective.
2.2 The effect of OHIS on health levels and behaviors
Online health information seeking (OHIS) refers to individuals seeking information about their health, illnesses, and other health-prevention behaviors through the internet (36). As mentioned before, OHIS can have both positive and negative effects on older adults’ health levels and behaviors. OHISs have drawn much attention during the pandemic. According to a systematic review of the COVID-19 information seeking behavior literature, OHIS behavior is a predictor of practising prevention behavior (37). Yang and Cao (38) found that OHIS can mediate the effects of age and education on the performance of protective behaviors, which means that it is important for older adults to actively look for health information from online sources. In contrast, some studies have reported negative results. In Thailand, Yodmai et al. (39) reported that health literacy and access to health information were not significant antecedents of older adults’ COVID-19 prevention behaviors. Vismara et al. (40) reported that OHIS contributes to psychological stress and has negative effects on health behaviors during the pandemic, especially for those who have cyberchondria (negative behavior characterized by excessive online health research combined with increasing levels of health anxiety or distress). Thus, the general effect of OHIS on older adults’ negative emotions and pandemic prevention behavior is still uncertain, and there is a need to investigate the long-term effect of OHIS on older adults’ negative emotions and prevention behavior.
To address the above three research questions, the study selected data from the SHARE project with older adults aged 55 or older in 26 European countries and Israel and used an autoregressive cross-lagged panel model (CLPM) and ordinary least squares (OLS) for data analysis.
3 Methods
3.1 Samples
The data used in this study are from the “Survey of Health, Aging and Retirement in Europe” (SHARE) project (41), which is the largest European social science panel study and has been widely used in gerontology. The sampling design for SHARE involves a complex multistage process that ensures the representativeness of the sample across participating countries, including random sampling and stratification, which considers different regions or population subgroups in the sample. Which is the largest European social science panel study. Specifically, we used data from the SHARE Wave 8 survey (October 2019–March 2020) (42, 43), the first SHARE Corona Survey (June–August 2020), and the second SHARE Corona Survey (June 2021–August 2021) (44, 45). The regular SHARE was a longitudinal survey fielded every 2 years via computer-assisted personal interviewing, and the SHARE Corona Survey was collected via computer-assisted telephone interviews on pandemic-related topics.
This study selected samples of people older than 55 years old who participated in both waves of the SHARE Corona Survey and removed those samples with missing values for key variables. The final samples are from 26 European countries and Israel. The first SHARE Corona Survey was treated as Wave 1, and the second SHARE Corona Survey was treated as Wave 2.
The surveys were finished at the end of two pandemic peaks. According to the Oxford Coronavirus Government Response Tracker (OxCGRT) (46), the average number of new deaths per million and the stringency index of governmental policy responses to the pandemic in the countries during the first survey were 0.290 and 48.824, respectively, and changed to 0.696 and 45.211, respectively, during the second survey, which indicates relatively similar pandemic severity.
3.2 Measures
Table 1 shows the descriptions of the variables used in our research. Prevention actions and negative emotions are measured in both Wave 1 and Wave 2. Increased Negative Emotions in Wave 2 The OHIS frequency change is measured in Wave 2. The study also included several covariates, including sex, age, education level, living alone, household income, and employment, as demographic variables and subjective health, chronic disease status, and COVID-19 status as health indicators. According to previous studies, these variables have a significant influence on older adults’ OHIS behavior and prevention behavior (47, 48). The study also considered the context regarding the evolution of the pandemic and associated public health guidance during the measurement period by adding two covariates: New Deaths per Million and Stringency Index, adapted from OxCGRT. The measurements of prevention actions and negative emotions and COVID-19 incidence were adapted from previous studies (7, 30, 49–51).
3.3 Data analysis
This study first used an autoregressive cross-lagged panel model (CLPM) (52) to analyze the relationship between negative emotions and prevention behaviors in older adults. In the CLPM, after controlling for reverse causality and the influence of auto regression, the estimated cross-lagged coefficient has a clear time-sequence relationship, which conforms to the causal inference principle of “the cause comes first and the result comes later” and excludes alternative explanations that may occur in cross-sectional studies (53).
Second, the study used ordinary least squares (OLS) regression to investigate the direct effect of changes in older adults’ OHIS behavior frequency on changes in negative emotions and prevention behaviors. The changes in negative emotions and prevention behaviors were measured by subtracting the value in wave 1 from the value in wave 2.
Third, to analyze the moderating effect of OHIS, a multiple-group approach was used in the CLPM (54, 55). The samples were divided into 4 groups according to their OHIS frequency changes. After checking the collinearity of all variables in the model, the CLPM was built in the structural equation model with robust standard error, and full information maximum likelihood estimation was used because of missing data for some covariates (56). All analyses were conducted using Stata 17.
4 Results
4.1 Demographic characteristics
The means, SDs, and Pearson correlation coefficients for the dependent variables and covariates are shown in Table 2. The final sample of this study comprised 20,894 individuals, with a mean age of 68 years and an average educational level of 3.5 years. Women constituted 56% of the participants. Only 24.7% of the participants lived alone, and 26.8% of the participants were employed. The subjective health of the participants was generally at a medium level. The participants had more than one chronic disease on average. Each sample had experience using the internet after the outbreak of the pandemic, indicating that everyone had basic IT skills.
For the dependent variables, each participant had an average of more than one prevention behavior and negative emotion. The average number of prevention behaviors decreased by 36.49% from wave 1 to wave 2, but the average number of negative emotions increased by 33.22%. There could be possible explanations for this finding. The initial wave of the pandemic engendered a heightened awareness and sense of urgency for older adults to engage in prevention behaviors, but as time went on, the constant stress and the decreasing stringency index of the pandemic may have led to a decline in the motivation to continue engaging in prevention behaviors. In addition, the increase in negative emotions could also be due to the prolonged impact of the pandemic, especially among those who were already vulnerable due to preexisting conditions or limited access to healthcare resources.
The correlations between negative emotions and prevention behaviors across the two waves were significant (0.52 and 0.20, respectively), which implied the consistency and variability of the dependent variables over time. There were also significant positive correlations between negative emotions and prevention behaviors across waves.
4.2 Cross-lagged models of negative emotions and prevention behaviors
Figure 2 illustrates the results of the CLPM. One-way arrows indicate the stability of constructs over time (i.e., autoregressive effects) and cross-lagged (i.e., reciprocal) effects, while two-way arrows indicate correlations. The relationship between older adults’ negative emotions and prevention behaviors was examined. First, the autoregressive coefficients of negative emotions and prevention behaviors were both significant (β = 0.430, p < 0.001 and β = 0.177, p < 0.001, respectively), but the autoregressive coefficient of prevention behaviors was less stable. Second, more negative emotions were significantly correlated with an increase in prevention behaviors in both Wave 1 and Wave 2 (β = 0.067, p < 001; β = 0.025, p < 0.001, respectively). Third, there was a significant cross-lagged effect of negative emotions in Wave 1 on prevention behaviors in Wave 2 (β = 0.038, p < 0.001), but prevention behaviors in Wave 1 had no significant effect on negative emotions in Wave 2 (β < 0.001, p = 0.823). Thus, for the first research question, it can be inferred that the negative emotions of older adults can positively predict their prevention behaviors, but not vice versa.
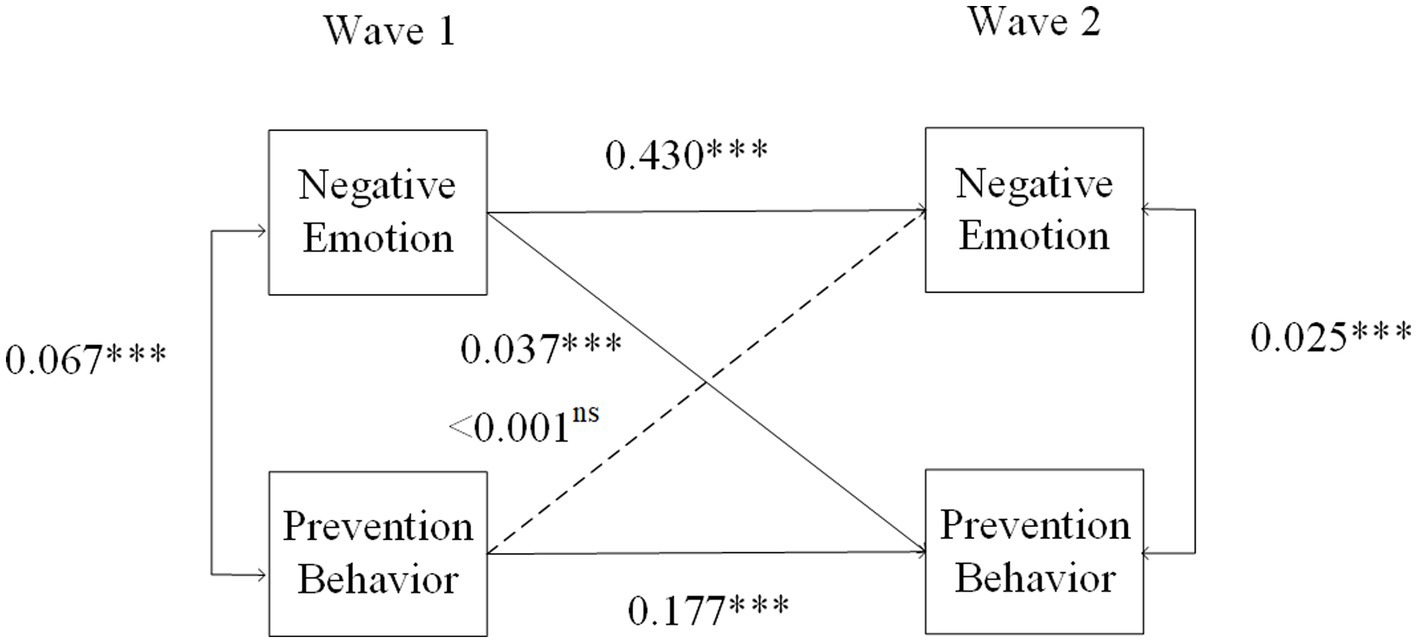
Figure 2. Autoregressive cross-lagged panel model of negative emotions and prevention behaviors. Covariates are not shown for clarity ns, nonsignificant; N = 20,894, *p < 0.05, **p < 0.01, ***p < 0.001.
The fit statistics and parameter estimates of the CLPM are reported in Supplementary material A1. The β and Z values of the relationship between negative emotions in wave 1 and prevention behaviors in wave 2 (0.038) are weaker than those between increased OHIS, subjective health, employment, gender and the stringency index but are greater than those between income, new deaths per million and other variables.
To check the validity of the results, the study further examined the relationship between fear of infection and prevention behavior to better explain the results. Fear of infection is also an important emotional representation in CSM (28). The measurement of “Fear of infection” was slightly different from that of other negative emotions, so it was tested in the CLPM separately. The question was “the outbreak of Corona, did you forgo medical treatment because you were afraid to become infected by the corona virus?” The results in Figure 3 show that there is a reciprocally significant relationship between fear of infection and prevention behaviors (β = 0.030, p < 0.001; β = 0.020, p = 0.002, respectively). Thus, the results prove that fear of infection can promote prevention behaviors, but prevention behaviors also increase fear of infection among older adults (57).
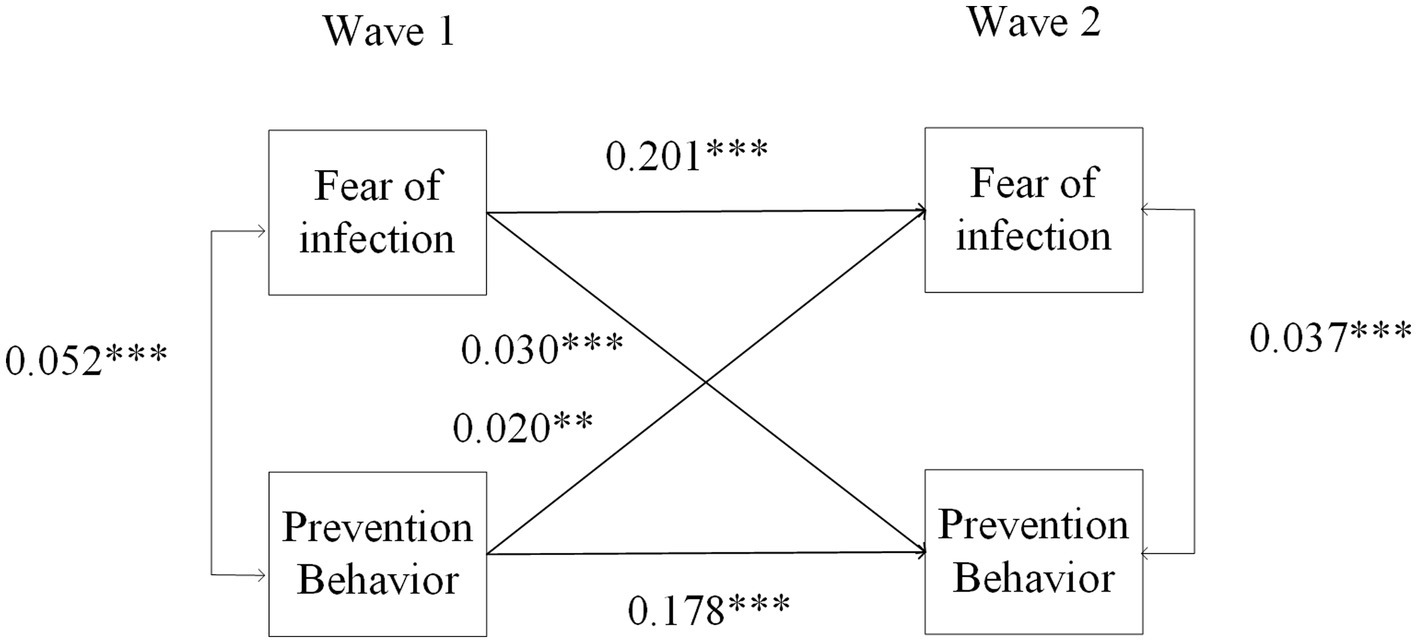
Figure 3. Autoregressive cross-lagged panel model of fear of infection and prevention behaviors. Covariates are not shown for clarity ns, nonsignificant, N = 20,894, *p < 0.05, **p < 0.01, ***p < 0.001.
In addition, to better explain the discrepancies in the previous literature, this study further divided prevention behaviors into two types: personal prevention behaviors (covering coughs and sneezes; taking drugs for coronavirus prevention) and socialized prevention behaviors (always or often staying distanced from others outside the home; not meeting with more than 5 people from outside the household). Figure 4 shows that there is no significant relationship between negative emotions and personal prevention behavior and vice versa (β = 0.007, p = 0.348; β = 0.004, p = 0.543, respectively), while there is a significant bidirectional relationship between negative emotions and socialized prevention behavior (β = 0.042, p < 0.001; β = 0.012, p = 0.035, respectively). Thus, the research reveals that socialized prevention behaviors are more strongly associated with negative emotions. In the early stage, there is a negative association, but it becomes positive in the later stage and in the long term. However, for personal prevention behavior, the association turned from positive to none. This finding is in line with previous studies showing that older adults do not suffer from the negative effect of confinement measures (58), but as the pandemic continues, low-cost and habituating behavior increases linearly, while adherence to high-cost and sensitizing behaviors decreases, which may be caused by the fatigue of the pandemic and restrictive policies (59). According to CSM, we can also infer that socialized behavior is more inclined to involve feedback on emotional representations and affect future prevention behaviors.
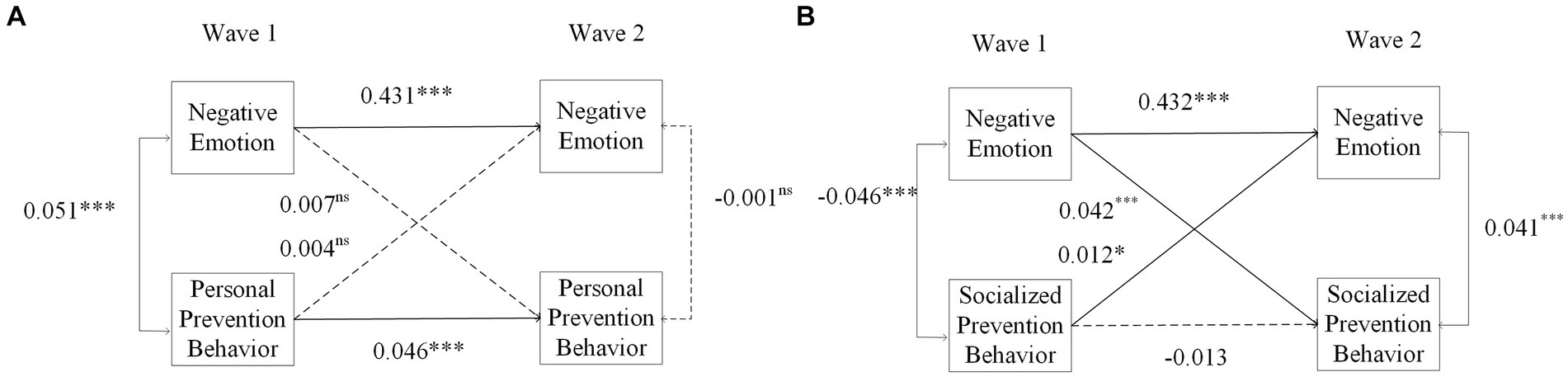
Figure 4. Autoregressive cross-lagged panel model of negative emotions and personal/socialized prevention behaviors. Covariates are not shown for clarity. ns, nonsignificant, N = 20,894, *p < 0.05, **p < 0.01, ***p < 0.001.(Model A: Personal Prevention Behavior, Model B: Social Prevention Behavior).
4.3 The direct effect of OHIS on prevention behaviors and negative emotion changes
Table 3 shows the results of the OLS estimates for the impact of OHIS on prevention behavior change and mental health change. Only increased OHIS had a significant positive impact on changes in prevention behavior (β = 0.109, p < 0.001). In addition, among the covariates, subjective health, education, COVID-19 severity and stringency index also had significant influences on negative emotion change, while age, subjective health, COVID-19 severity, marital status, stringency index and number of new deaths per million people had significant influences on prevention behavior change. The coefficient between increased OHIS and prevention behavior change (β = 0.0.109) was greater than that for other variables, and for negative emotion change, subjective health had the strongest influence (β = −0.132). The significance of COVID-19 involvement and subjective health are in accordance with CSM, in that illness representation can influence both emotional representation and coping strategies (25).
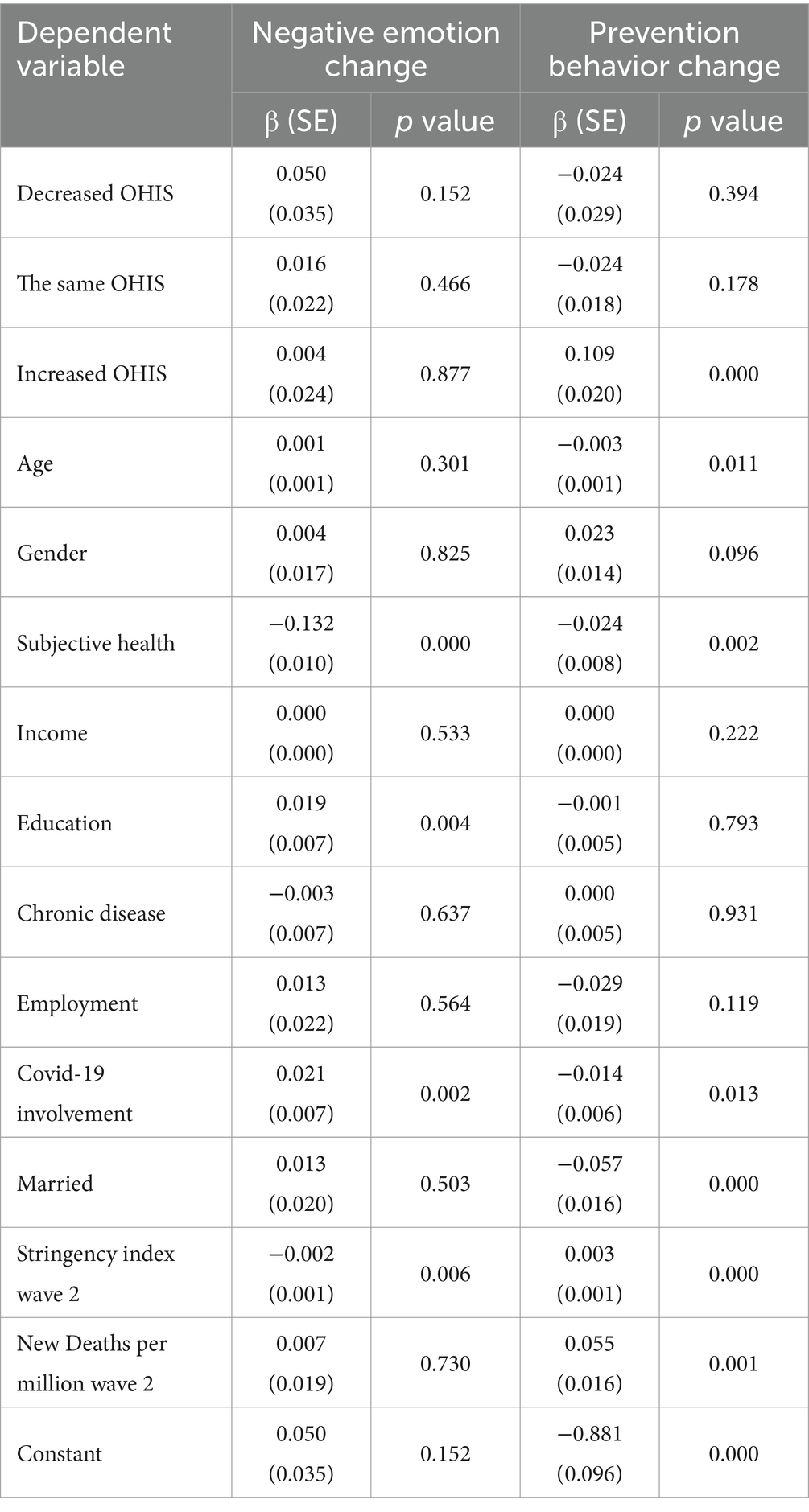
Table 3. Ordinary least squares estimate for the impact of OHIS on prevention behavior change and negative emotion change (n = 19,489).
4.4 The moderating effect of OHIS between negative emotions and prevention behaviors
In Figure 5, Models A-D are for different changes in OHIS behavior frequency (Model A: not at all (N = 4,440), Model B: less often (N = 1,589), Model C: approximately the same (N = 9,050), Model D: more often (N = 5,815)). There was no measurement invariance between the groups. The cross-lagged effect of negative emotions in Wave 1 on prevention behaviors in Wave 2 was significant in Models 1, 3 and 4 (negative emotions had a positive effect on prevention behaviors; β = 0.031, p = 0.045; β = 0.035, p < 0.001; β = 0.038, p = 0.003, respectively) but was not significant in Model 2 (β = 0.026, p = 0.306).
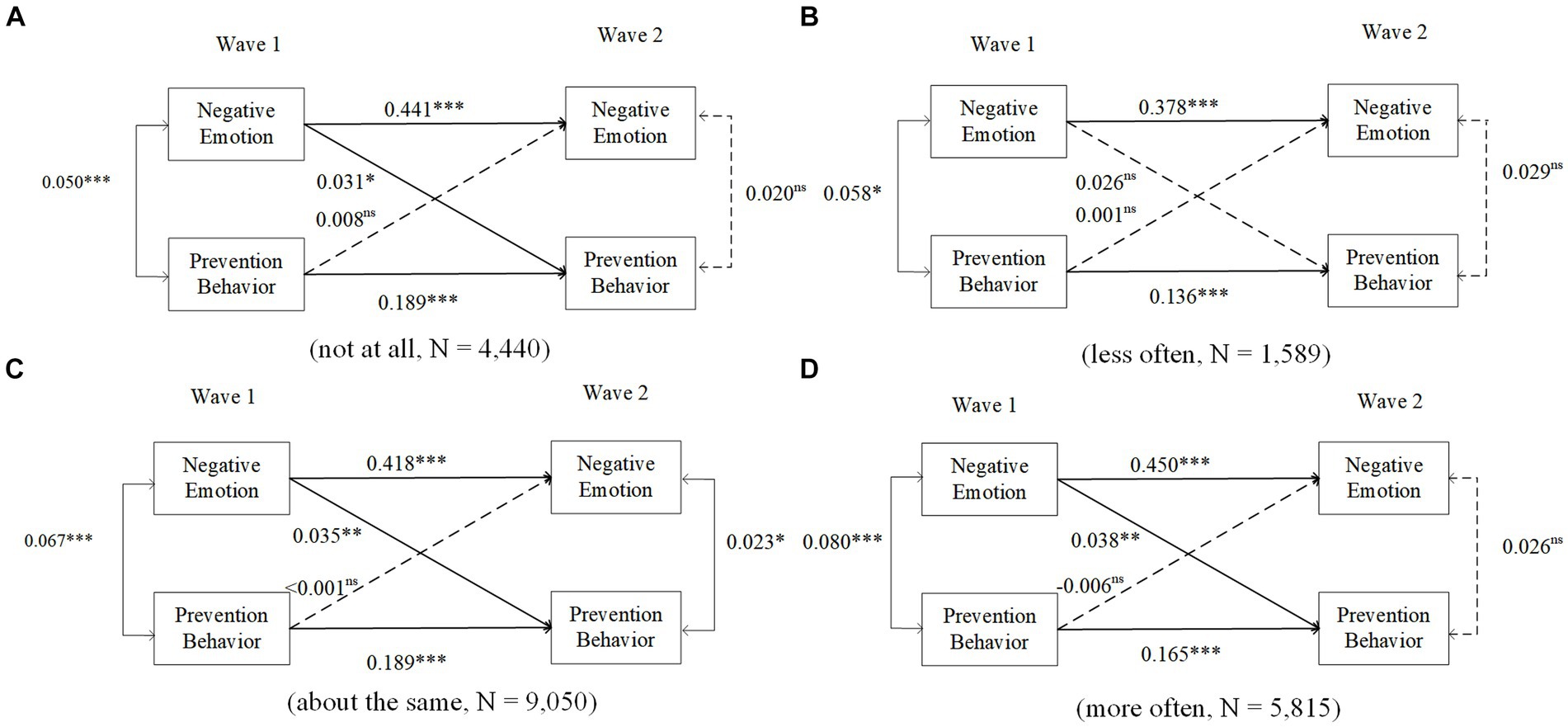
Figure 5. The moderating effect of OHIS between negative emotions and prevention behaviors. Covariates are not shown for clarity. ns: nonsignificant, *p < 0.05, **p < 0.01, ***p < 0.001. Models A-D are for different changes in OHIS behavior frequency (Model A: not at all, Model B: less often, Model C: approximately the same, Model D: more often).
In terms of the coefficients in the models, all the data are reported in Supplementary material A2. The results are similar to the results in Supplementary material A1. To increase the validity of the results, this study also examined the moderating effect of OHIS on the relationship between increased negative emotions and prevention behaviors, as shown in Table 4. The results among the different groups are similar to the results mentioned above.
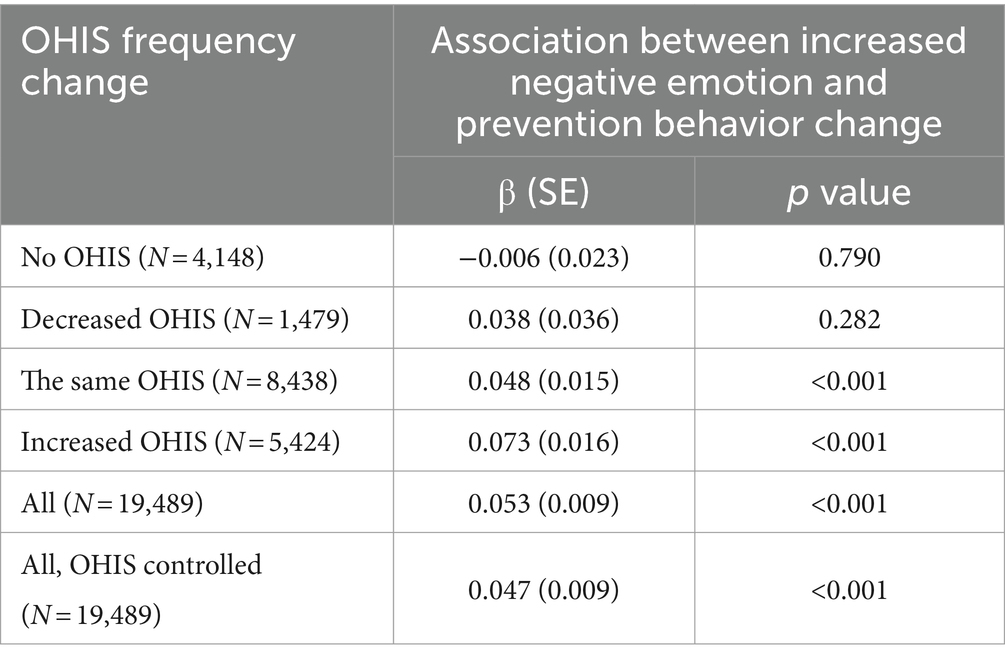
Table 4. Grouped regression estimate for the moderating effect of OHIS on the relationship between increased negative emotions and prevention behaviors (n = 19,489).
Therefore, for the third research question, we can conclude that a change in OHIS frequency can moderate the association between negative emotions and preventive behaviors in older adults.
5 Discussion
5.1 Key findings
This study investigated the reciprocal relationship between negative emotions and prevention behaviors in older adults, as well as the direct and moderating effects of older adults’ OHIS behavior on negative emotions and prevention behaviors, based on SHARE data.
The results indicate that negative emotions can positively predict the prevention behaviors of older adults. This finding is in line with previous studies showing that anxiety is associated with the prevention behaviors of older adults (7, 48). However, a previous study also showed that fear did not predict prevention behavior 6 months later in US women (60), possibly because older adults have more life experiences and tend to become more cautious and inclined to have better compliance with preventive measures after experiencing negative psychological emotions (61). Moreover, the cross-lagged effect between prevention behavior in wave 1 and negative emotions in wave 2 was not significant. The results again indicate that adhering to prevention behavior is not a sufficient solution to older adults’ mental health issues, and further coping strategies are needed. In terms of the magnitude of the relationship, increased OHIS, subjective health, employed, gender, and stringency index have higher coefficients. The results suggest that the impact of negative emotions might be relatively moderate compared to that of these other influential factors. In addition, it reflects the multidimensional nature of factors that influence health behaviors, including emotional, socioeconomic, contextual, and informational factors. This study also revealed that the discrepancies in previous literature are possibly caused by differences in certain behaviors studied. Many previous studies treated prevention behaviors as an aggregate variable (30, 34, 35), or as a single behavior (31, 32), but in this study we found that negative emotions can have a greater influence on socialized prevention behavior than on personalized behavior. This finding is in line with the study by Dixon et al. (29), which revealed that anxiousness is associated with physical distancing but not hand washing or face covering.
The OLS results show that compared with no OHIS, only increased OHIS has a significant positive impact on prevention behavior change. A possible explanation is that older adults with increased OHIS can obtain information and methods related to prevention, and in combination with their concern for their own health, they will be more proactive in prevention. In contrast, although the information obtained by OHIS related to the pandemic may enable older adults to understand the pandemic and reduce panic, reports on its serious consequences may also increase anxiety. However, the study revealed that subjective health had the strongest correlation with negative emotion change, showing a negative association. This finding is consistent with a previous study (62). This study also revealed no significant effect of OHIS on older adults’ negative emotions, which is consistent with the findings of a previous study suggesting that OHIS in older adults may stem from their anxiety as they strive to find information about themselves or social relationships (63). Therefore, OHIS can significantly enhance prevention behaviors but has no significant effect on alleviating negative emotions, which are more strongly associated with physical health. The discrepancy among previous studies regarding the influence of OHIS on prevention behaviors was possibly caused by the measurement of OHIS [e.g., the existence of OHIS (38), access to health information (39), and excessive OHIS behavior (40)]. Few studies have investigated the changes in OHIS usage frequency over time (64). This result is consistent with a previous study showing that the likelihood of changing health behavior is positively correlated with the frequency of OHIS behavior (65).
The results of the multigroup CLPM revealed that the long-term effect of negative emotions on prevention behavior varies among older adults with different changes in OHIS frequency. With the exception of older adults with reduced OHIS, negative emotions have a significant positive influence on their prevention behaviors, with the highest among those with increased OHIS, followed by those with unchanged frequency, and the lowest among those without OHIS. This suggests that efforts to promote OHIS among older adults could be particularly important for individuals with negative emotions, as OHIS may help to promote the impact of mental health issues on prevention behaviors. In addition, the decreased OHIS among older adults may indicate the existence of information avoidance, which weakens the impact of mental health issues. Health information avoidance is a barrier to older adults’ pandemic prevention (66). The determinants of information avoidance about the COVID-19 pandemic include less negative affective risk responses, more pronounced descriptive and injunctive avoidance norms, and perceived information overload (67), so it is important to provide interventions on both information accessibility and health literacy to older adults who have less OHIS. Therefore, older adults who engage in OHIS behavior have greater access to accurate and up-to-date information about the pandemic, which can help them better understand the risks and benefits of prevention behaviors and therefore support prevention behaviors. In general, OHIS plays a moderating role in the relationship between older adults’ negative emotions and prevention behaviors, which promotes the positive impact of negative emotions on prevention behaviors.
6 Implications
6.1 Theoretical implications
Overall, our study has several theoretical implications. First, it contributes to the growing body of literature on the relationships among OHIS, mental health, and behavior, particularly among older adults. This study examines the reciprocal longitudinal relationship between negative emotions and prevention behaviors and proves that OHIS and negative emotions are both important antecedents of protection behaviors. This study also highlights the unique contribution of OHIS in moderating the relationship between negative emotions and prevention behaviors. The results reduce the doubts and confusion caused by the previous literature. Second, the study also provides a reference for other well-known predictors in the model, such as subjective health. Our results revealed that subjective health has a significant positive correlation with negative emotion and prevention behavior. For future research, the significant role of subjective well-being in our model implies the importance of considering both emotional representation and illness representation according to CSM when studying pandemic-related health behaviors and designing interventions.
6.2 Practical implications
The findings of this research also have several implications for healthcare professionals and policymakers who work with older adults.
First, to promote effective prevention behavior, it is crucial to consider the emotional status of older adults, and a comprehensive and personalized approach is needed to promote older adults’ mental health. According to our findings, healthcare professionals should pay special attention to older adults who report lower subjective health, as they are more likely to exhibit decreased prevention behavior and negative emotional changes.
Second, the direct and moderating effects of OHIS on prevention behavior suggest that providing older adults with access to credible health information can be an effective strategy for promoting prevention behavior, but according to previous literature, OHIS may also have negative effects, so it is crucial to prevent information avoidance and take care of those suffering from unhealthy levels of health anxiety. Thus, healthcare professionals should consider providing older adults with health information and resources that are tailored to their specific needs and preferences and continuing to combat misinformation and conflicting health information online. Policymakers should also invest in digital inclusion initiatives to bridge the digital divide among older adults, including providing affordable technology devices, improving internet connectivity, and offering digital literacy training to enhance older adults’ digital skills and confidence.
Third, in light of the study’s finding that negative emotions have a bidirectional significant positive relationship with socialized protection behaviors. Thus, healthcare campaigns can emphasize the collective aspect of protective measures. Community organizations can play a pivotal role in organizing health education programs, peer support networks, and social activities for older adults. These interventions can foster social connections, alleviate feelings of isolation, and promote engagement in preventive behaviors.
Finally, the findings from this study highlight the importance of considering the long-term effects of OHIS during public health crises. Healthcare providers and organizations should prioritize promoting OHIS behavior throughout the pandemic. Regular updates, reminders, and practical tips can help maintain older adults’ motivation to engage in protection behaviors and stay informed. In addition, policymakers and healthcare authorities should incorporate OHIS promotion as a crucial component of public health preparedness for future crises and develop proactive communication strategies to empower older adults with accurate and timely health information.
6.3 Limitations and future research
The study also has several limitations that have implications for future research. First, the data we used are based on self-reports and may therefore reflect a subjective perspective. Future research can consider the inclusion of secondary data such as user logs. Second, a single item rather than psychometrically sound instruments was used to measure each negative emotion and prevention behavior due to the secondary analysis of SHARE data. Thus, the specific impact paths and mechanisms have not been explored in depth, and more research can be conducted in the future by combining qualitative and quantitative analysis methods. In addition, exploring the different effects of actively and passively obtaining health information on older adults’ prevention behavior is worthwhile.
7 Conclusion
Based on SHARE data, this study investigated the reciprocal relationship between negative emotions and prevention behaviors in older adults, as well as the direct and moderating effects of older adults’ OHIS during the pandemic. The findings suggest that negative emotions can positively predict prevention behaviors. In addition, OHIS can have a direct effect on changes in prevention behaviors and can have a moderating effect on the relationship between negative emotions and prevention behaviors. This study enhances the understanding of older adults’ OHIS on negative emotions and pandemic prevention behaviors and demonstrates the value of fostering OHIS in resolving long-term public health crises and preventing infectious diseases.
Data availability statement
The data analyzed in this study is subject to the following licenses/restrictions: The data that support the findings of this study are available at the SHARE Research Data Center to the entire research community free of charge (www.share-project.org). Restrictions apply to the availability of these data, which were used under license for the current study, and so are not publicly available. Requests to access these datasets should be directed to http://www.share-project.org/data-access/user-registration.html?L=.
Author contributions
TL: Writing – original draft, Writing – review & editing, Conceptualization, Data curation, Formal analysis, Validation. XS: Writing – review & editing, Conceptualization, Methodology, Funding acquisition. QZ: Writing – review & editing, Funding acquisition, Supervision.
Funding
The author(s) declare that financial support was received for the research, authorship, and/or publication of this article. This work was jointly supported by grants from the Key Projects of the National Social Science Foundation of China (22&ZD327) and the National Natural Science Foundation of China (72204210 and 72174083). This paper uses data from SHARE 8 and 9 (DOIs: 10.6103/SHARE.w8ca.800, 10.6103/SHARE.w9ca800) and, see Börsch-Supan et al. (41) for methodological details. The SHARE data collection has been funded by the European Commission, DG RTD through FP5 (QLK6-CT- 2001-00360), FP6 (SHARE-I3: RII-CT-2006-062193, COMPARE: CIT5-CT-2005-028857, SHARELIFE: CIT4-CT-2006-028812), FP7 (SHARE- PREP: GA N°211909, SHARE-LEAP: GA N°227822, SHARE M4: GA N°261982, DASISH: GA N°283646) and Horizon 2020 (SHARE-DEV3: GA N°676536, SHARE-COHESION: GA N°870628, SERISS: GA N°654221, SSHOC: GA N°823782, SHARE-COVID19: GA N°101015924) and by DG Employment, Social Affairs & Inclusion through VS 2015/ 0195, VS 2016/0135, VS 2018/0285, VS 2019/0332, and VS 2020/ 0313. Additional funding from the German Ministry of Education and Research, the Max Planck Society for the Advancement of Science, the U. S. National Institute on Aging (U01_AG09740-13S2, P01_AG005842, P01_AG08291, P30_AG12815, R21_AG025169, Y1-AG-4553-01, IAG_BSR06-11, OGHA_04–064, HHSN271201300071C, RAG052527A) and from various national funding sources is gratefully acknowledged (see www.share-project.org).
Conflict of interest
The authors declare that the research was conducted in the absence of any commercial or financial relationships that could be construed as a potential conflict of interest.
Publisher’s note
All claims expressed in this article are solely those of the authors and do not necessarily represent those of their affiliated organizations, or those of the publisher, the editors and the reviewers. Any product that may be evaluated in this article, or claim that may be made by its manufacturer, is not guaranteed or endorsed by the publisher.
Supplementary material
The Supplementary material for this article can be found online at: https://www.frontiersin.org/articles/10.3389/fpubh.2024.1377017/full#supplementary-material
A1 Parameter estimates in the CLPM of negative emotions and prevention behaviors.
A2 Parameters of the four CLPMs with Different Changes in the OHIS Behavior Frequency.
References
1. Yanez, ND, Weiss, NS, Romand, JA, and Treggiari, MM. COVID-19 mortality risk for older men and women. BMC Public Health. (2020) 20:1742. doi: 10.1186/s12889-020-09826-8
2. Liu, K, Chen, Y, Lin, R, and Han, K. Clinical features of COVID-19 in elderly patients: a comparison with young and middle-aged patients. J Infect. (2020) 80:e14–8. doi: 10.1016/j.jinf.2020.03.005
3. Wu, B . Social isolation and loneliness among older adults in the context of COVID-19: a global challenge. Glob Health Res Policy. (2020) 5:27. doi: 10.1186/s41256-020-00154-3
4. Banerjee, D . The impact of Covid-19 pandemic on elderly mental health. Int J Geriatr Psychiatry. (2020) 35:1466–7. doi: 10.1002/gps.5320
5. Atzendorf, J, and Gruber, S. Depression and loneliness of older adults in Europe and Israel after the first wave of COVID-19. Aging Ment Health. (2021) 19:849–61. doi: 10.1007/s10433-021-00640-8
6. Pearman, A, Hughes, ML, Smith, EL, and Neupert, SD. Age differences in risk and resilience factors in COVID-19-related stress. J Gerontol B Psychol Sci Soc Sci. (2021) 76:e38–44. doi: 10.1093/geronb/gbaa120
7. Xiang, H, Luo, J, Zhou, J, and Zhou, Z. Older adults' prevention and communication to beat anxiety: the diminishing utility of proactive coping actions. Univ Access Inf Soc. (2022) 22:1–20. doi: 10.1007/s10209-022-00915-6
8. Choi, EY, Farina, MP, Wu, Q, and Ailshire, J. COVID-19 social distancing measures and loneliness among older adults. J Gerontol B Psychol Sci Soc Sci. (2022) 77:e167–78. doi: 10.1093/geronb/gbab009
9. Peng, S, Luo, X, Liang, S, Deng, F, Liu, Y, Zeng, H, et al. Psychological and emotional responses during different stages of the COVID-19 pandemic based on a survey of a mental health hotline. Int J Ment Health Promot. (2022) 11:711–24. doi: 10.3389/fpsyg.2020.566212
10. Pourrazavi, S, Kouzekanani, K, Jafarabad, MA, Bazargan-Hejazi, S, Hashemiparast, M, and Allahverdipour, H. Correlates of older Adults' E-health information-seeking behaviors. Gerontology. (2022) 68:935–42. doi: 10.1159/000521251
11. Medlock, S, Eslami, S, Askari, M, Arts, DL, Sent, D, De Rooij, SE, et al. Health information-seeking behavior of seniors who use the internet: a survey. J Med Internet Res. (2015) 17:e10. doi: 10.2196/jmir.3749
12. Zhang, C, Liao, WF, Ma, Y, and Liang, C. Research on older people’s health information search behavior based on risk perception in social networks—a case study in China during COVID-19. Front Public Health. (2022) 10:946742. doi: 10.3389/fpubh.2022.946742
13. Lund, B, and Ma, J. Exploring information seeking of rural older adults during the COVID-19 pandemic. Aslib J Inf Manag. (2022) 74:54–77. doi: 10.1108/AJIM-04-2021-0118
14. Kim, HK, Ahn, J, Atkinson, L, and Kahlor, LA. Effects of COVID-19 misinformation on information seeking, avoidance, and processing: a multicountry comparative study. Sci Commun. (2020) 42:586–615. doi: 10.1177/1075547020959670
15. Zhou, J, Xiang, H, and Xie, B. Better safe than sorry: a study on older adults' credibility judgments and spreading of health misinformation. Univ Access Inf Soc. (2022) 22:1–10. doi: 10.1007/s10209-022-00899-3
16. Soroya, SH, Farooq, A, Mahmood, K, Isoaho, J, and Zara, SE. From information seeking to information avoidance: understanding the health information behavior during a global health crisis. Inf Process Manag. (2021) 58:102440. doi: 10.1016/j.ipm.2020.102440
17. Song, S, Yao, X, and Wen, N. What motivates Chinese consumers to avoid information about the COVID-19 pandemic?: the perspective of the stimulus-organism-response model. Inf Process Manag. (2021) 58:102407. doi: 10.1016/j.ipm.2020.102407
18. Pluye, P, El Sherif, R, Granikov, V, Hong, QN, Vedel, I, Galvao, MCB, et al. Health outcomes of online consumer health information: a systematic mixed studies review with framework synthesis. J Assoc Inf Sci Technol. (2019) 70:643–59. doi: 10.1002/asi.24178
19. Liu, PL . COVID-19 information seeking on digital media and preventive behavior: the mediation role of worry. Cyberpsychol Behav Soc Netw. (2020) 23:677–82. doi: 10.1089/cyber.2020.0250
20. Kim, K, Yang, J, Jeon, YJ, Lee, YJ, Lee, Y, Kim, HC, et al. The effects of information-seeking behaviours on prevention behaviours during the COVID-19 pandemic: the mediating effects of anxiety and fear in Korea. Epidemiol Health. (2021) 43:e2021085. doi: 10.4178/epih.e2021085
21. Frijda, NH, Kuipers, P, and ter Schure, E. Relations among emotion, appraisal, and emotional action readiness. J Pers Soc Psychol. (1989) 57:212–28. doi: 10.1037/0022-3514.57.2.212
22. Mo, PKH, Fong, VWI, Song, B, Di, J, Wang, Q, and Wang, L. Association of Perceived Threat, negative emotions, and self-efficacy with mental health and personal protective behavior among Chinese pregnant women during the COVID-19 pandemic: cross-sectional survey study. J Med Internet Res. (2021) 23:e24053. doi: 10.2196/24053
23. Rogers, RW . A protection motivation theory of fear appeals and attitude change. J Psychol. (1975) 91:93–114. doi: 10.1080/00223980.1975.9915803
24. Janz, NK, and Becker, MH. The health belief model: a decade later. Health Educ Q. (1984) 11:1–47. doi: 10.1177/109019818401100101
25. Leventhal, H, Meyer, D, and Nerenz, D. The common sense representation of illness danger. Contrib Med Psychol. (1980) 2:7–30.
26. Myrick, JG . The role of emotions and social cognitive variables in online health information seeking processes and effects. Comput Human Behav. (2017) 68:422–33. doi: 10.1016/j.chb.2016.11.071
27. Leventhal, H, Benyamini, Y, Brownlee, S, Diefenbach, M, Leventhal, EA, Patrick-Miller, L, et al. Illness representations: theoretical foundations In: KJ Petrie and JA Weinman, editors. Perceptions of health and illness: Current research and applications. New York: Harwood Academic Publishers (1997). 19–45.
28. Hagger, MS, Koch, S, Chatzisarantis, NL, and Orbell, S. The common sense model of self-regulation: Meta-analysis and test of a process model. Psychol Bull. (2017) 143:1117–54. doi: 10.1037/bul0000118
29. Dixon, D, Den Daas, C, Hubbard, G, and Johnston, M. Using behavioural theory to understand adherence to behaviours that reduce transmission of COVID-19; evidence from the CHARIS representative national study. Br J Health Psychol. (2022) 27:116–35. doi: 10.1111/bjhp.12533
30. Ning, L, Niu, J, Bi, X, Yang, C, Liu, Z, Wu, Q, et al. The impacts of knowledge, risk perception, emotion and information on citizens’ protective behaviors during the outbreak of COVID-19: a cross-sectional study in China. BMC Public Health. (2020) 20:1751. doi: 10.1186/s12889-020-09892-y
31. Vollmann, M, and Salewski, C. To get vaccinated, or not to get vaccinated, that is the question: illness representations about COVID-19 and perceptions about COVID-19 vaccination as predictors of COVID-19 vaccination willingness among young adults in the Netherlands. Vaccine. (2021) 9:941. doi: 10.3390/vaccines9090941
32. Luo, YF, Shen, HY, Yang, SC, and Chen, LC. The relationships among anxiety, subjective well-being, media consumption, and safety-seeking behaviors during the COVID-19 epidemic. Int J Environ Res Public Health. (2021) 18:13189. doi: 10.3390/ijerph182413189
33. Oh, YS, and Song, NK. Investigating relationships between health-related problems and online health information seeking. CIN. (2021) 35:29–35. doi: 10.1097/CIN.0000000000000234
34. Ezati Rad, R, Mohseni, S, Kamalzadeh Takhti, H, Hassani Azad, M, Shahabi, N, Aghamolaei, T, et al. Application of the protection motivation theory for predicting COVID-19 preventive behaviors in Hormozgan, Iran: a cross-sectional study. BMC Public Health. (2021) 21:466. doi: 10.1186/s12889-021-10500-w
35. Alegria, KE, Fleszar-Pavlović, SE, Ngo, DD, Beam, A, Halliday, DM, Hinojosa, BM, et al. The role of risk perceptions and affective consequences in COVID-19 protective behaviors. Int J Behav Med. (2021) 28:801–7. doi: 10.1007/s12529-021-09970-4
36. Jacobs, W, Amuta, AO, and Jeon, KC. Health information seeking in the digital age: an analysis of health information seeking behavior among US adults. Cogent Soc Sci. (2020) 3:e15906. doi: 10.1080/23311886.2017.1302785
37. Anagaw, TF, and Guadie, HA. Coronavirus disease 2019 information-seeking behavior globally: a systematic review. SAGE Open Med. (2023) 11:205031212311535. doi: 10.1177/20503121231153510
38. Yang, Q, and Cao, W. Health disparities in online COVID-19 information seeking and protective behavior: a two-wave longitudinal study. Health Commun. (2022) 37:1534–43. doi: 10.1080/10410236.2022.2056980
39. Yodmai, K, Pechrapa, K, Kittipichai, W, Charupoonpol, P, and Suksatan, W. Factors associated with good COVID-19 preventive behaviors among older adults in urban communities in Thailand. J Prim Care Community Health. (2021) 12:21501327211036251. doi: 10.1177/21501327211036251
40. Vismara, M, Vitella, D, Biolcati, R, Ambrosini, F, Pirola, V, Dell’Osso, B, et al. The impact of COVID-19 pandemic on searching for health-related information and Cyberchondria on the general population in Italy. Front Psych. (2021) 12:754870. doi: 10.3389/fpsyt.2021.754870
41. Börsch-Supan, A, Brandt, M, Hunkler, C, Kneip, T, Korbmacher, J, Malter, F, et al. Data resource profile: the survey of health, ageing and retirement in Europe (SHARE). Int J Epidemiol. (2013) 42:992–1001. doi: 10.1093/ije/dyt088
42. SHARE-ERIC . Survey of Health, Ageing and Retirement in Europe (SHARE) Wave 8. Release version: 9.0.0. SHARE-ERIC. Data set. (2024). doi: 10.6103/SHARE.w8.900
43. Bergmann, M, and Börsch-Supan, A eds. SHARE wave 8 methodology: Collecting cross-National Survey Data in times of COVID-19. MEA, Max Planck Institute for Social Law and Social Policy: Munich (2021).
44. Börsch-Supan, A. Survey of health, ageing and retirement in Europe (SHARE) wave 9. COVID-19 survey 2. Release version: 8.0.0. SHARE-ERIC. Data set. (2022).
45. Scherpenzeel, A, Axt, K, Bergmann, M, Douhou, S, Oepen, A, Sand, G, et al. Collecting survey data among the 50+ population during the COVID-19 outbreak: the survey of health, ageing and retirement in Europe (SHARE). Survey Res Methods. (2020) 14:217–21. doi: 10.18148/srm/2020.v14i2.7738
46. Hale, T, Angrist, N, Goldszmidt, R, Kira, B, Petherick, A, Phillips, T, et al. A global panel database of pandemic policies (Oxford COVID-19 government response tracker). Nat Human Behav. (2021) 5:529–38. doi: 10.1038/s41562-021-01079-8
47. Zhao, YC, Zhao, M, and Song, S. Online health information seeking behaviors among older adults: systematic scoping review. J Med Internet Res. (2022) 24:e34790. doi: 10.2196/34790
48. Sand, G, and Bristle, J. The relationship of threat perceptions and optimistic attitude with protective behavior in the COVID-19 crisis. SSRN Electron J. (2021). doi: 10.2139/ssrn.3832914
49. Wester, CT, Bovil, T, Scheel-Hincke, LL, Ahrenfeldt, LJ, Möller, S, and Andersen-Ranberg, K. Longitudinal changes in mental health following the COVID-19 lockdown: results from the survey of health, ageing, and retirement in Europe. Ann Epidemiol. (2022) 74:21–30. doi: 10.1016/j.annepidem.2022.05.010
50. Peña-Longobardo, LM, Oliva-Moreno, J, and Rodríguez-Sánchez, B. The effects of severe acute respiratory syndrome coronavirus 2 on the reported mental health symptoms of nonprofessional carers: an analysis across Europe. Value Health. (2022) 25:736–43. doi: 10.1016/j.jval.2021.10.011
51. Cohn-Schwartz, E, Hoffman, Y, and Shrira, A. The effect of PTSD and depression symptoms on mental distress among older adults during COVID-19. J Psychiatr Res. (2022) 151:633–7. doi: 10.1016/j.jpsychires.2022.05.022
52. Selig, J, and Little, T. Autoregressive and cross-lagged panel analysis for longitudinal data In: B Laursen, TD Little, and NA Card, editors. Handbook of developmental research methods. New York, NY: The Guilford Press (2011). 265–78.
53. Hwang, J, Toma, CL, Chen, J, Shah, DV, Gustafson, D, and Mares, ML. Effects of web-based social connectedness on older adults’ depressive symptoms: a two-wave cross-lagged panel study. J Med Internet Res. (2021) 23:e21275. doi: 10.2196/21275
54. Lindwall, M, Larsman, P, and Hagger, MS. The reciprocal relationship between physical activity and depression in older European adults: a prospective cross-lagged panel design using SHARE data. Health Psychol. (2011) 30:453–62. doi: 10.1037/a0023268
55. Ulrich, SM, Lux, U, Liel, C, and Walper, S. Long-term effects of targeted prevention programmes for families with young children: a population-based study on parenting stress and children's negative emotionality. Child Care Health Dev. (2022) 48:763–71. doi: 10.1111/cch.12985
57. Harper, CA, Satchell, LP, Fido, D, and Latzman, RD. Functional fear predicts public health compliance in the COVID-19 pandemic. Int J Ment Health Addic. (2021) 19:1875–88. doi: 10.1007/s11469-020-00281-5
58. Yastrebov, G, and Maskileyson, D. The effect of COVID-19 confinement and economic support measures on the mental health of older population in Europe and Israel. Soc Sci Med. (2022) 314:115445. doi: 10.1016/j.socscimed.2022.115445
59. Petherick, A, Goldszmidt, R, Andrade, EB, Furst, R, Hale, T, Pott, A, et al. A worldwide assessment of changes in adherence to COVID-19 protective behaviours and hypothesized pandemic fatigue. Nat Hum Behav. (2021) 5:1145–60. doi: 10.1038/s41562-021-01181-x
60. Anderson, KM, and Stockman, JK. Fear of COVID-19 and prevention behavior: cross-lagged panel analysis. JMIR Form Res. (2022) 6:e35730. doi: 10.2196/35730
61. Neubauer, AB, Smyth, JM, and Sliwinski, MJ. Age differences in proactive coping with minor hassles in daily life. J Gerontol B Psychol Sci Soc Sci. (2019) 74:7–16. doi: 10.1093/geronb/gby061
62. Karademas, EC, Tsalikou, C, and Tallarou, MC. The impact of emotion regulation and illness-focused coping strategies on the relation of illness-related negative emotions to subjective health. J Health Psychol. (2011) 16:510–9. doi: 10.1177/1359105310392093
63. He, R, and Li, Y. Media exposure, cancer beliefs, and cancer-related information-seeking or avoidance behavior patterns in China. Int J Environ Res Public Health. (2021) 18:3130. doi: 10.3390/ijerph18063130
64. Xiong, Z, Zhang, L, Li, Z, Xu, W, Zhang, Y, and Ye, T. Frequency of online health information seeking and types of information sought among the general Chinese population: cross-sectional study. J Med Internet Res. (2021) 23:e30855. doi: 10.2196/30855
65. Ayers, SL, and Kronenfeld, JJ. Chronic illness and health-seeking information on the internet. Health. (2007) 11:327–47. doi: 10.1177/1363459307077547
66. Link, E . Information avoidance during health crises: predictors of avoiding information about the COVID-19 pandemic among German news consumers. Inf Process Manag. (2021) 58:102714. doi: 10.1016/j.ipm.2021.102714
Keywords: online health information seeking, older adults, SHARE, COVID-19, negative emotion, longitudinal study
Citation: Liu T, Song X and Zhu Q (2024) Exploring the relationship between older adults’ online health information seeking, negative emotions and prevention behaviors in the pandemic context: a two-wave longitudinal study. Front. Public Health. 12:1377017. doi: 10.3389/fpubh.2024.1377017
Edited by:
Steven Hoffman, Brigham Young University, United StatesReviewed by:
Jiangjie Sun, Hefei University of Technology, ChinaJeff Bolles, University of North Carolina at Pembroke, United States
Mariela Gonzalez Tovar, Pontificia Universidad Católica de Chile, Chile
Copyright © 2024 Liu, Song and Zhu. This is an open-access article distributed under the terms of the Creative Commons Attribution License (CC BY). The use, distribution or reproduction in other forums is permitted, provided the original author(s) and the copyright owner(s) are credited and that the original publication in this journal is cited, in accordance with accepted academic practice. No use, distribution or reproduction is permitted which does not comply with these terms.
*Correspondence: Qinghua Zhu, qhzhu@nju.edu.cn