- 1Department of Health Systems and Management, School of Public Health, College of Medicine and Health Sciences, Wollo University, Dessie, Ethiopia
- 2Department of Health Systems and Policy, Institute of Public Health, College of Medicine and Health Sciences, University of Gondar, Gondar, Ethiopia
- 3Department of Epidemiology and Biostatistics, School of Public Health, College of Medicine and Health Sciences, Wollo University, Dessie, Ethiopia
- 4Department of Nutrition, Institute of Public Health, College of Medicine and Health Science, University of Gondar, Gondar, Ethiopia
- 5Department of Environmental and Occupational Health and Safety, Institute of Public Health, College of Medicine and Health Science, University of Gondar, Gondar, Ethiopia
- 6Department of Epidemiology and Biostatistics, Institute of Public Health, College of Medicine and Health Science, University of Gondar, Gondar, Ethiopia
- 7Department of Human Physiology, School of Medicine, College of Medicine and Health Science, University of Gondar, Gondar, Ethiopia
Background: In sub-Saharan Africa, achieving universal health coverage (UHC) and protecting populations from health-related financial hardship remain challenging goals. Subsequently, community-based health insurance (CBHI) has gained interest in low and middle-income countries, such as Ethiopia. However, the rural–urban disparity in CBHI enrollment has not been properly investigated using multivariate decomposition analysis. Therefore, this study aimed to assess the rural–urban disparity of CBHI enrollment in Ethiopia using the Ethiopian Mini Demographic Health Survey 2019 (EMDHS 2019).
Methods: This study used the latest EMDHS 2019 dataset. STATA version 17.0 software was used for analyses. The chi-square test was used to assess the association between CBHI enrollment and the explanatory variables. The rural–urban disparity of CBHI enrollment was assessed using the logit-based multivariate decomposition analysis. A p-value of <0.05 with a 95% confidence interval was used to determine the statistical significance.
Results: The study found that there was a significant disparity in CBHI enrollment between urban and rural households (p < 0.001). Approximately 36.98% of CBHI enrollment disparities were attributed to the compositional (endowment) differences of household characteristics between urban and rural households, and 63.02% of the disparities were due to the effect of these characteristics (coefficients). The study identified that the age and education of the household head, family size, number of under-five children, administrative regions, and wealth status were significant contributing factors for the disparities due to compositional differences between urban and rural households. The region was the significant factor that contributed to the rural–urban disparity of CBHI enrollment due to the effect of household characteristics.
Conclusion: There were significant urban–rural disparities in CBHI enrollment in Ethiopia. Factors such as age and education of the household head, family size, number of under-five children, region of the household, and wealth status of the household contributed to the disparities attributed to the endowment, and region of the household was the contributing factor for the disparities due to the effect of household characteristics. Therefore, the concerned body should design strategies to enhance equitable CBHI enrollment in urban and rural households.
Background
Ensuring everyone has access to essential health services is a key commitment of the 2030 Agenda for Sustainable Development, which affirms health as a basic human right. The most effective way to achieve the motto of “leave no one behind” is by providing universal health coverage (UHC). It is aimed to ensure that people can access high-quality healthcare without facing financial risks due to healthcare expenditures (1–4).
Although the UHC movement has been gaining support worldwide (1), in 2021, nearly half of the global population—4.5 billion people—did not have access to essential healthcare services. In 2019, nearly 2 billion people experienced financial hardship because of their healthcare expenditures, with 344 million of them being extremely poor (1). Achieving UHC for most countries in sub-Saharan Africa has been a challenging agenda so far. This has led to more interest in community-based health insurance (CBHI), a health insurance scheme that has been established for workers of non-formal sectors in many countries such as Ethiopia (2, 3, 5–7).
Ethiopia is among the lowest spenders on health systems in Africa. In 2016, only 1.4% of its gross domestic product (GDP) was allocated to health (8). The country also fell short of the 15% target set by the Abuja Declaration, allocating only 11.1% of the total government budget to health in 2014 (9–11). The latest National Health Account report revealed that Ethiopia spends only 4.7% of its GDP on health, which is lower than the target recommended by the World Health Organization. Additionally, people had to pay 30% of the total healthcare costs directly from their own pockets, which is the highest rate in the world and in Africa (12). Moreover, accessing essential healthcare remains a significant challenge for achieving UHC in Ethiopia, especially for underprivileged people living in rural areas (2, 3, 6, 7, 13, 14).
CBHI is a type of volunteer health insurance that aims to provide financial protection and access to quality healthcare for the poor, vulnerable populations, and informal sector workers. Ethiopia launched CBHI in 2011 with a vision of reaching 80% of districts and 80% of its population by 2020 (14–20). However, despite the government’s efforts to expand CBHI to all districts and studies conducted on different aspects of CBHI including spatial analysis (7), to the best of authors knowledge, evidence is lacking on the assessment of rural–urban CBHI enrollment disparity and the factors that contributed to the disparities using the logit-based multivariate decomposition analysis (14, 18–20). Therefore, this study aimed to assess the disparities in CBHI enrollment between rural and urban households and identify the factors contributing to these disparities due to endowments (E) and coefficients (C) by conducting a multivariate decomposition analysis of the EMDHS 2019 data. This study used a multivariate decomposition analysis model because it provides distinct advantages over spatial analysis, particularly in its ability to identify and quantify the contribution of individual variables on the outcome variable disparity between groups and urban and rural dimensions in this study. While the spatial analysis is adept at identifying patterns and clusters within geographical data, the multivariate decomposition analysis provides a deeper understanding of the underlying factors determining these patterns. This method allows for a more nuanced analysis of trends and can inform targeted interventions by pinpointing the exact factors that contribute to changes in outcomes over time or between two distinct groups, making it a powerful tool for policy-making and strategic planning in various fields of research (21, 22). Therefore, this study aimed to answer the following two main research questions;
1. Was there an urban–rural disparity in the CBHI enrollment rate in Ethiopia in 2019?
2. If a disparity existed, what factors were contributing to the disparity?
Methods
Data sources, study setting, and populations
Ethiopia is a landlocked country in the Horn of Africa (3°–14° N and 330–48° E), with nine regional states (Tigray, Afar, Amhara, Oromia, Somalia, Benishangul-Gumuz, Southern Nations, Nationalities, and Peoples’ Region (SNNPR), Gambella, and Harari) and two city administrations (Addis Ababa and Dire Dawa city administrations) in 2019 (23). The source of data for this study was the recent EMDHS 2019 dataset. The survey provides nationally representative data on a wide range of demographic and health indicators (24, 25). The survey used a two-stage cluster sampling technique stratified in nature, wherein enumeration areas (EAs) were the primary units and households were the secondary units (26). The dataset has been made freely available on the Internet for academics and researchers. The source population for this study was all households in Ethiopia. We excluded households who had missing information for CBHI enrollment and/or any of the explanatory variables of interest. After all, the final sample size comprised 8,663 households, of which 2,645 households were from urban areas and 6,018 households were from rural areas in this study.
Study variables and measurements
Outcome variable
In this study, the outcome variable was CBHI enrollment. It was categorized as either “Yes” (labeled as 1) if enrolled for CBHI or “No” (labeled as 0) if the household did not enroll for CBHI.
Stratifying variable
Place of residency was the key independent variable used to stratify households by their CBHI enrollment status and was categorized as urban (labeled as “0”) or rural (coded as “1”).
Explanatory variables
Explanatory variables, known to be associated with CBHI enrollment from previous literature studies, were extracted from EMDHS 2019. These variables are the educational level of the household head (ordinal categorical variables with categories such as “no education”, “primary education”, “secondary education”, and “higher education”), wealth index (ordinal categorical variables with “poor,” “middle,” and “rich” category), age of household head, sex of household head, family size, and region of the household. The explanatory variables were categorized after consulting related previous literature (3, 4, 7, 15). All explanatory variables with their respective categories are presented in Table 1.
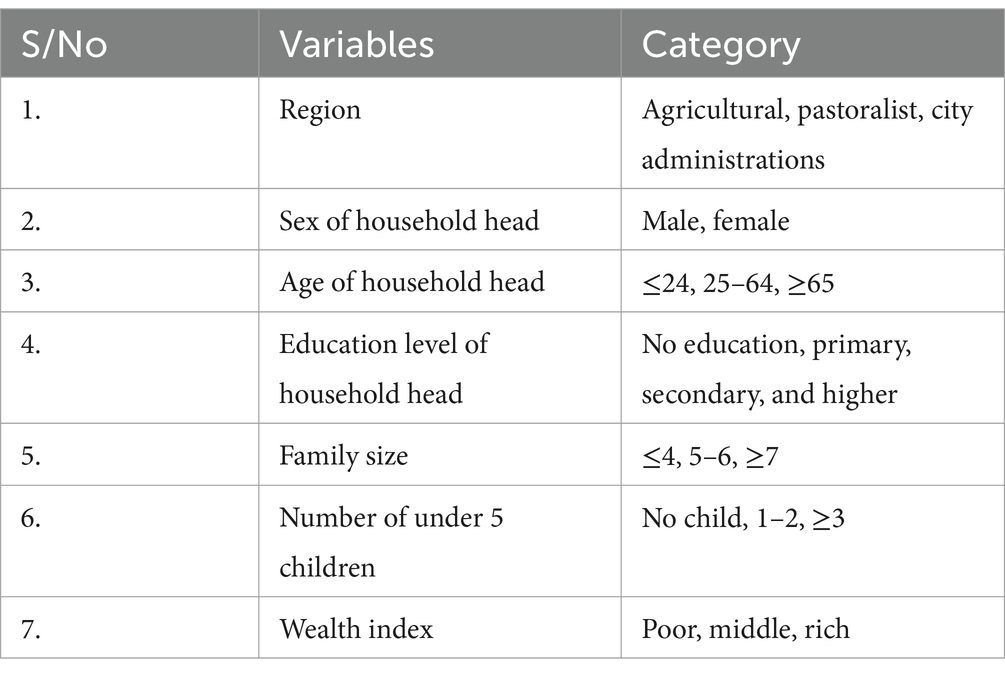
Table 1. List of extracted explanatory variables for urban–rural disparity in CBHI enrollment with their respective categories from the EMDHS 2019.
Statistical analysis
Descriptive statistics and chi-square test
STATA version 17.0 statistical software was used for data analysis. The STATA survey setting (svyset) command was used to control the complex clustering effect. The sociodemographic characteristics of the household heads and other variables were analyzed using descriptive statistics and were summarized as weighted frequencies and percentages. Pearson’s chi-square test was used to assess the association between CBHI enrollment and the explanatory variables. The explanatory variables having significant association (p-value <0.05) with CBHI enrollment during the chi-square test were included in a multivariate decomposition analysis.
Multivariate decomposition analysis
To estimate the observed disparities of the CBHI enrollment between rural and urban households, we used the logit-based multivariate decomposition analysis method (27). Multivariate decomposition is commonly used in social research to quantify the contributions to group differences in average predictions from multivariate models. It helps to divide the components of a group difference into those attributable to compositional differences (such as variations in characteristics or endowments) and those attributable to differences in the effects of characteristics (such as variations in returns, coefficients, or behavioral responses) (28).
Multivariate decomposition is useful to analyze disparities in health variables, such as race, sex, and other groupings. Although the method was originally designed for use in decomposing labor market outcomes between different groups, it has become useful in stratifying the outcome of health variables by various types of groups. This technique has also been applied in evaluating a given health variable across residencies, both urban and rural. The current study used the multivariate decomposition technique to decompose the difference in the CBHI enrollment for rural and urban areas of Ethiopia using the user-written mvdcmp STATA command (27, 29).
This method is appropriate because the difference in the observed CBHI enrollment rate between urban and rural households can be due to the difference in the distribution of the characteristics (compositional differences) and the effects of these characteristics (coefficient) or the interaction between the two components.
Result
Descriptive analysis and chi-square test
Table 2 shows the descriptive analysis of study participants and the association between explanatory variables with the outcome (i.e., CBHI enrollment) and equity-stratifying (i.e., place of residence) variables using the Pearson chi-square test. The overall weighted rate of CBHI enrollment in Ethiopia was 28.09% [95%CI, 26.68–29.58%] in 2019. Of the participants, approximately 68.04% were rural inhabitants residents without CBHI enrollment, and 80.60% were urban inhabitants without CBHI enrollment. Moreover, 77.93% of the households had male heads, with 47.38% having no education and 7% having higher education. Regarding the wealth status of the households, more than one-third (36.16%) and approximately half (44.50%) of the households were poor and rich, respectively (Table 2).
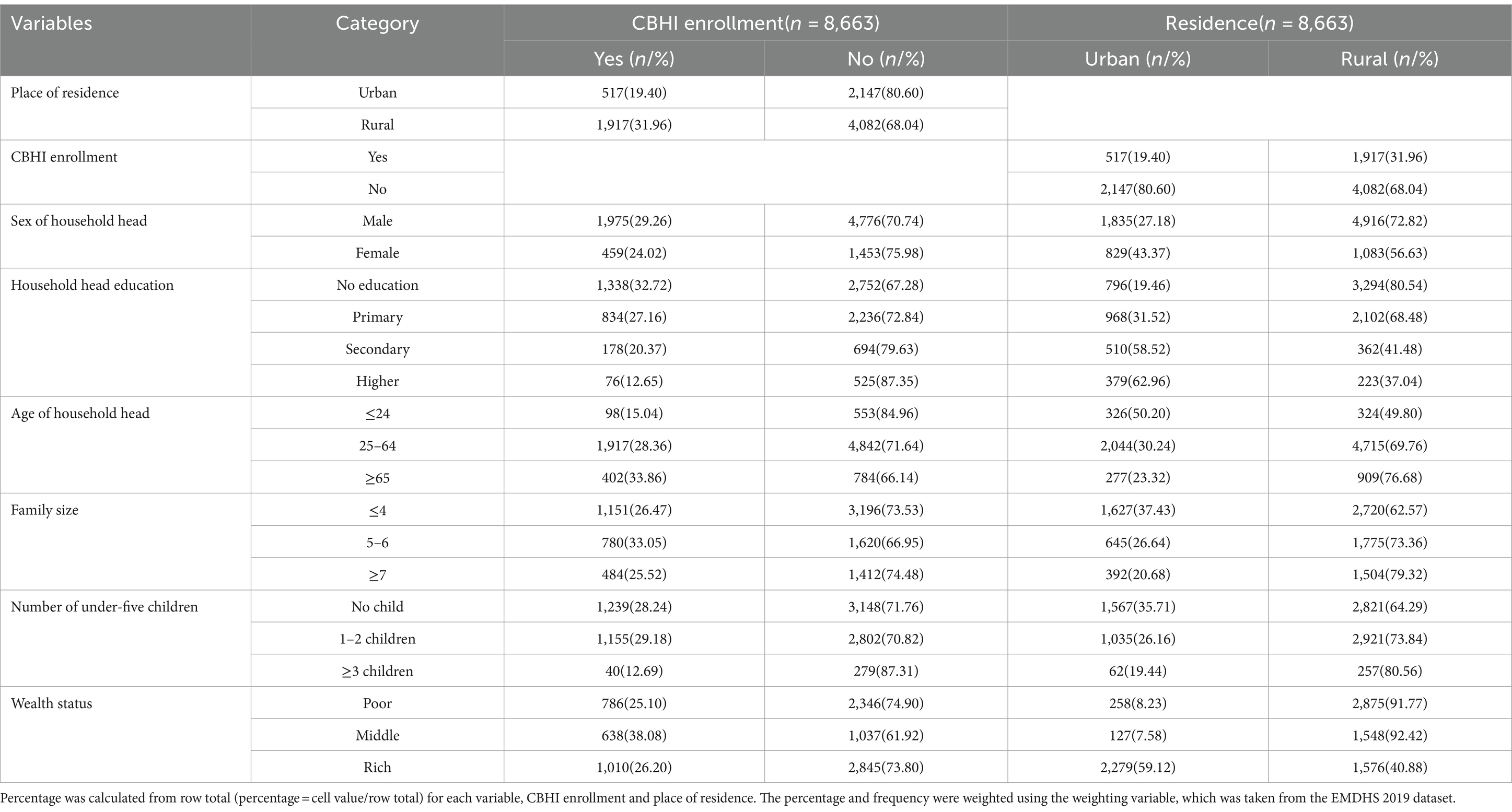
Table 2. Descriptive analysis of explanatory variables for CBHI enrollment disparity between urban and rural households using the EMDHS 2019.
Figure 1 shows the CBHI enrollment rate across places of residence, such as urban and rural households. Nearly 32% of the households in the rural area were enrolled for CBHI, whereas 19.40% of the households in the urban area were enrolled for CBHI in 2019 in Ethiopia, indicating that rural households were more likely to be enrolled for CBHI in 2019.
Multivariate decomposition analysis
Table 3 shows the overall decomposition analysis of the urban–rural CBHI enrollment rate disparity. This disparity was classified into three components, namely, a gap due to the difference in the composition of characteristics (i.e., explained/endowment), a gap due to the difference in the effect of the characteristics (i.e., unexplained/coefficient), and a gap due to the interaction between the two components, endowment (i.e., explained part and coefficients, unexplained part).
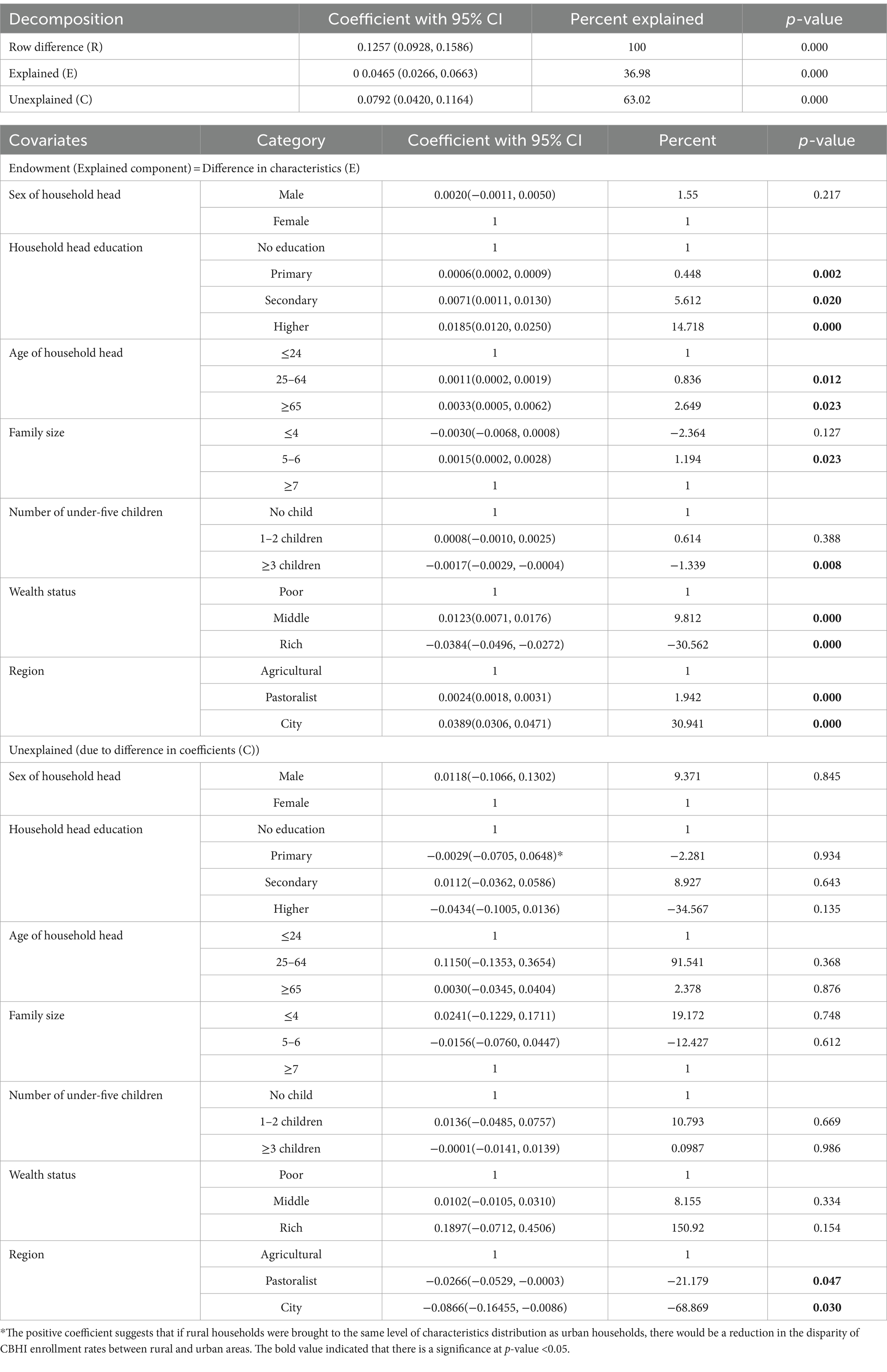
Table 3. Detailed decomposition of CBHI enrollment by place of residence among households in Ethiopia using EMDHS 2019.
The overall decomposition result showed that there is a significant disparity in the CBHI enrollment rate between urban and rural households (0.126, p < 0.001). The study found that 36.98% (p < 0.001) of the CBHI enrollment rate disparities were explained by the differences in the distribution of characteristics (endowments (E)) of households between urban and rural households, whereas we found that the differences in the effects (coefficients) of characteristics account for 63.02% (p < 0.001) of the observed rural–urban differences in the CBHI enrollment rate.
Table 3 also presents the detailed decomposition of the contribution of individual characteristics to the CBHI enrollment rate disparity between urban and rural households due to endowment and coefficients. The majority of the gap in CBHI enrollment was explained by the difference in wealth status between urban and rural households. The middle wealth status (9.81%) contributed to the narrowing of the gap, meaning if the composition of middle wealth status of the households was equalized between urban and rural households, the gap in CBHI enrollment rate would be narrowed by 9.81%, and rich wealth status (−30.56%) contributed to the widening of this gap.
Similarly, factors such as the distribution of age of household heads of 25–64 years (0.84%) and ≥ 65 years (2.65%), educational status of household heads with primary education (0.45%) and higher education (14.72%), family size of household 5–6 members (1.20%), and the household region category being city administration (30.94%) and pastoralist (1.94%) contributed to the narrowing of the gap in CBHI enrollment across rural and urban areas. This indicates that equalizing the composition of these characteristics between urban and rural households would be expected to reduce the gap in CBHI enrollment rates by 0.84% for household heads aged 25–64 years, 2.65% for household heads aged ≥65 years, 14.72% for household heads with higher education, and 31% for households in different regions (Table 3). Conversely, households with heads having secondary education (−5.62%) and households with 3 or more under-five children (−1.34%) contribute to widening the gap in CBHI enrollment rates across rural and urban households in Ethiopia (Table 3).
Discussion
This study aimed to assess the rural–urban disparity of CBHI enrollment rate in Ethiopia using data from the EMDHS 2019 and the logit-based decomposition analysis. The finding of this study revealed that there was a significant disparity in CBHI enrollment between urban and rural households. We found that 36.98% of the observed rural–urban CBHI enrollment differentials were attributed to differences in household characteristics, and 63.02% of the observed differences were attributed to differences in the effect of household characteristics between rural and urban households. The previous study conducted on CBHI enrollment using spatial analysis supported this finding, revealing that there were geographical variations, such as urban and rural variations, in CBHI enrollment in Ethiopia (7). This implied that there is a need for targeted interventions to address the significant disparity in CBHI enrollment between urban and rural households. Policymakers, health planners, and healthcare managers should focus on equalizing CBHI enrollment among rural and urban households by ensuring the accessibility of the CBHI scheme in both urban and rural areas (4, 24, 30).
This study also found that the secondary and higher education levels of the household heads were the significant factors contributing to the reduction in urban–rural disparity of CBHI enrollment approximately by 6 and 15%, respectively. This finding implies that higher education among household heads plays a crucial role in narrowing the gap between urban and rural areas in terms of CBHI enrollment. When household heads have higher education levels, it positively impacts CBHI enrollment rates, potentially leading to more equitable access to healthcare services. Thus, policymakers and health planners should prioritize efforts to improve education levels, particularly among household heads in rural areas. Investing in education and expanding educational opportunities can help empower individuals to make informed decisions about healthcare, including enrolling in the CBHI scheme (7, 31, 32).
The number of under-five children was also found to be a significant contributing factor in the widening of the urban–rural disparity of CBHI enrollment rate by 1.34%. The finding implies that there is a need to have focused policies considering the number of under-five children in CBHI enrollment-related strategies and activities. Policymakers should prioritize family planning and reproductive health programs to empower individuals and families to make informed decisions. Additionally, strengthening maternal and child healthcare services in rural areas can help alleviate the burden on families and increase CBHI enrollment rates. Targeted interventions and support for families with multiple children, alongside addressing broader socioeconomic challenges, are essential to narrowing the gap and ensuring equitable access to healthcare services for all (31, 32).
Similarly, the study stated that the rich wealth status of the households significantly contributed to the widening of the disparity in CBHI enrollment by 30.56% between urban and rural households. This finding is supported by the study conducted on multidimensional determinants of CBHI in Ethiopia, which revealed that socioeconomic status was the determinant of CBHI enrollment (33). The finding implies the need for targeted interventions, improved financial accessibility, efforts to reduce income inequality, and strengthened social protection programs. Health decision-makers should prioritize strategies that address the specific needs of disadvantaged households, such as implementing subsidy programs and premium waivers, promoting inclusive economic growth, and providing targeted support to vulnerable populations. By addressing wealth disparities and enhancing access to healthcare services, policymakers can work toward narrowing the wealth-related disparity and ensuring equitable CBHI enrollment for households across both urban and rural areas (34–36).
Policy and practical implications
The results of the study on the rural–urban disparity in CBHI enrollment in Ethiopia have important practical and policy implications. Policymakers and health managers should focus on addressing the disparities in enrolling for CBHI programs and strive to achieve equal enrollment rates across urban and rural households. Moreover, the study highlights the role of administrative regions in contributing to the rural–urban disparity in CBHI enrollment. This can help policymakers identify regions with lower enrollment rates and allocate resources equitably. It is important to address regional disparities and ensure that CBHI programs are accessible and effective across all regions of the country.
Limitations of the study
The limitation of this study is its reliance on data from the EMDHS 2019, which may not reflect the current status of the urban–rural disparity in CBHI enrollment in Ethiopia as of 2024.
Conclusion
The study found that there was a significant disparity in CBHI enrollment rate between urban and rural households in Ethiopia in 2019. Factors such as the age of household heads, educational level of household heads, family size, number of under-five children, region of the household, and wealth status of the household contributed significantly to the observed disparities due to the differentials in endowments of the households, and region of the households was the significant contributing factor for the disparities attributed to the effect of characteristics (coefficients).
Data availability statement
The original contributions presented in the study are included in the article/supplementary material, and further inquiries can be directed to the corresponding author.
Ethics statement
Ethical approval was not required for our study as we used the demographic and health survey, which identifies all data before making it public, and the DHS datasets we used are openly accessible. We obtained an authorization letter from CSA to download the DHS dataset at https://dhsprogram.com/. The dataset and all methods and data of demographic and health survey were performed based on DHS research guidelines.
Author contributions
YT: Writing – review & editing, Writing – original draft, Visualization, Supervision, Software, Methodology, Investigation, Formal analysis, Data curation, Conceptualization. HA: Writing – review & editing, Methodology, Conceptualization. DG: Writing – review & editing, Methodology. AH: Writing – review & editing, Methodology. MJ: Writing – review & editing, Supervision, Methodology. KA: Writing – review & editing, Writing – original draft, Supervision. MT: Writing – review & editing, Supervision. KD: Writing – review & editing, Methodology. LA: Writing – review & editing, Methodology. AE: Data curation, Software, Writing – original draft, Writing – review & editing. WN: Writing – review & editing, Data curation. AW: Writing – review & editing, Data curation. LY: Writing – review & editing, Data curation. MG: Writing – review & editing, Data curation. NW: Writing – review & editing, Writing – original draft, Conceptualization. AB: Conceptualization, Writing – review & editing, Writing – original draft.
Funding
The author(s) declare that no financial support was received for the research, authorship, and/or publication of this article.
Acknowledgments
The authors are honored to appreciate the Demographic and Health Survey (DHS) program for providing the EDHS dataset with an authorization letter.
Conflict of interest
The authors declare that the research was conducted in the absence of any commercial or financial relationships that could be construed as a potential conflict of interest.
Publisher’s note
All claims expressed in this article are solely those of the authors and do not necessarily represent those of their affiliated organizations, or those of the publisher, the editors and the reviewers. Any product that may be evaluated in this article, or claim that may be made by its manufacturer, is not guaranteed or endorsed by the publisher.
Abbreviations
CBHI, Community-Based Health Insurance; LMICs, Low and Middle-Income Countries; DHS, Demographic and Health Survey; EMDHS 2019, Ethiopian Mini Demographic Health Survey 2019; UHC, Universal Health Coverage
References
1. World Health Organization. Tracking universal health coverage: 2023 global monitoring report. Geneva: World Health Organization (2023).
2. Abebe, Y, and Belayneh, F. Determinants of willingness to pay for community-based health insurance scheme among households in rural community of southern Ethiopia. BMC Health Serv Res. (2023) 23:1365. doi: 10.1186/s12913-023-10406-w
3. Atnafu, A, and Gebremedhin, T. Community-based health insurance enrollment and child health service utilization in Northwest Ethiopia: a cross-sectional case comparison study. ClinicoEconomics and Outcomes Research, (2020). 12:435–44. doi: 10.2147/CEOR.S262225,
4. Akafu, W, Daba, T, Tesfaye, E, Teshome, F, and Akafu, T. Determinants of trust in healthcare facilities among community-based health insurance members in the manna district of Ethiopia. PLoS One. (2023) 23:171. doi: 10.1186/s12889-023-15124-w
5. Abdilahi, AO, Farah, AE, and Dagnaw, FT. Community-based health insurance, healthcare service utilization and associated factors in South Gondar zone northwest, Ethiopia, 2021: a comparative cross-sectional study. Health Econ Rev. (2022) 17:e0270758. doi: 10.1371/journal.pone.0270758
6. Mulat, AK, Mao, W, Bharali, I, Balkew, RB, and Yamey, G. Scaling up community-based health insurance in Ethiopia: a qualitative study of the benefits and challenges. BMC Health Serv Res. (2022) 22:473. doi: 10.1186/s12913-022-07889-4
7. Terefe, B, Alemu, TG, Techane, MA, Wubneh, CA, Assimamaw, NT, Belay, GM, et al. Spatial distribution and associated factors of community based health insurance coverage in Ethiopia: further analysis of Ethiopian Demography and Health Survey, 2019. Glob Health Action. (2022) 22:1523. doi: 10.1186/s12889-022-13950-y
8. González, R, and Marino, J. Analysis of out-of-pocket health expenditure using the WHO Global Health expenditure database (GHED): a systematic review. Gac Med Caracas. (2020) 128:474–9. doi: 10.47307/GMC.2020.128.4.4
9. FMOH. Health sector transformation plan (HSTP) II 2015/16–2019/20. Addis Ababa: Federal Democratic Republic of Ethiopia Ministry of Health (2015).
10. Badacho, AS, Tushune, K, Ejigu, Y, and Berheto, TM. Household satisfaction with a community-based health insurance scheme in Ethiopia. BMC Res Notes. (2016) 9:424. doi: 10.1186/s13104-016-2226-9
11. Begashaw Bekele, B, Tesema Berkesa, S, Tefera, E, and Kumalo, A. Self-medication practice in Limmu Genet, Jimma zone, Southwest Ethiopia: does community based health insurance scheme have an influence? J Pharm (Cairo). (2018) 2018:1–8. doi: 10.1155/2018/1749137
12. FMOH. Ethiopian health accounts household health service utilization and expenditure survey 2019/2020 [internet]. Addis Ababa: Federal Democratic Republic of Ethiopia Ministry of Health (2022).
13. Atafu, A, and Kwon, S. Adverse selection and supply-side factors in the enrollment in community-based health insurance in Northwest Ethiopia: a mixed methodology. Risk Manag Healthc Policy. (2018) 33:902–14. doi: 10.1002/hpm.2546
14. Babore, GO, Ashine, TM, Heliso, AZ, and Habebo, TT. Client satisfaction and associated factors towards the health service provided to members of a community-based health insurance scheme in southern Ethiopia. Front Health Serv. (2023) 3:1237895. doi: 10.3389/frhs.2023.1237895
15. Alemu, G, Bedi, AS, and Moyehodie, YA. The effects of individual and community-level factors on community-based health insurance enrollment of households in Ethiopia. Int J Environ Res Public Health. (2022) 17:e0275896. doi: 10.1371/journal.pone.0275896
16. Anbesu, EW. Willingness to pay for community-based health insurance and associated factors in Ethiopia: a systematic review and meta-analysis. BMJ Open. (2022) 10:20503121221135876. doi: 10.1177/20503121221135876
17. Asfaw, A, Etana, B, Mulatu, B, and Babure, ZK. Household heads satisfaction and associated factors on community-based health insurance scheme in Gudeya Bila District, Oromia, Ethiopia. J Public Health Res. (2023) 12:227990362311811. doi: 10.1177/22799036231181181
18. Asfaw, DM, Shifaw, SM, Belete, AA, and Aychiluhm, SB. The impact of community-based health insurance on Household's welfare in Chilga District, Amhara regional state, Ethiopia. Front Public Health. (2022) 10:868274. doi: 10.3389/fpubh.2022.868274
19. Ayana, ID. Investigation of moral Hazard deportments in community-based health Insurance in Guto Gida District, Western Ethiopia: a qualitative study. Clinicoecon Outcomes Res. (2020) 12:733–46. doi: 10.2147/CEOR.S269561
20. Deksisa, A, Abdo, M, Mohamed, E, Tolesa, D, Garoma, S, Zewdie, A, et al. Willingness of community based health insurance uptake and associated factors among urban residents of Oromia regional state, Oromia, Ethiopia, a cross-sectional study. BMC Health Serv Res. (2020) 20:864. doi: 10.1186/s12913-020-05583-x
21. Belay, DG, and Aragaw, FM. Trend, multivariate decomposition and spatial variations of unintended pregnancy among reproductive-age women in Ethiopia: evidence from demographic and health surveys. Trop Med Health. (2022) 50:47. doi: 10.1186/s41182-022-00440-5
22. Teshale, AB, Worku, MG, and Tesema, GA. Spatial distribution and determinants of the change in pre-lacteal feeding practice over time in Ethiopia: a spatial and multivariate decomposition analysis. PLoS One. (2021) 16:e0244574. doi: 10.1371/journal.pone.0244574
23. Factbook CW. Ethiopian Demographics Profile. Index Mundi; (2021). Available at: https://www.indexmundi.com/ethiopia/demographics_profile.html
24. Habte, A, Tamene, A, Ejajo, T, Dessu, S, Endale, F, Gizachew, A, et al. Towards universal health coverage: the level and determinants of enrollment in the community-based health insurance (CBHI) scheme in Ethiopia: a systematic review and meta-analysis. PLoS One. (2022) 17:e0272959. doi: 10.1371/journal.pone.0272959
25. Hussien, M, Azage, M, and Bayou, NB. A mixed methods study of community-based health insurance enrollment trends and underlying challenges in two districts of Northeast Ethiopia: a proxy for its sustainability Plos one (2022) 17:e0266583. doi: 10.1371/journal.pone.0266583,
27. Powers, DA, Yoshioka, H, and Yun, M-S. Mvdcmp: multivariate decomposition for nonlinear response models. Stata J. (2011) 11:556–76. doi: 10.1177/1536867X1201100404
28. Asresie, MB, Dagnew, GW, and Bekele, YA. Changes in immunization coverage and contributing factors among children aged 12-23 months from 2000 to 2019, Ethiopia: multivariate decomposition analysis. PLoS One. (2023) 18:e0291499. doi: 10.1371/journal.pone.0291499
29. Tesema, GA, Tessema, ZT, and Tamirat, KS. Decomposition and Spatio-temporal analysis of health care access challenges among reproductive age women in Ethiopia, 2005–2016. BMC Health Serv Res. (2020) 20:760. doi: 10.1186/s12913-020-05639-y
30. Workneh, SG, Biks, GA, and Woreta, SA. Community-based health insurance and communities' scheme requirement compliance in Thehuldere district, Northeast Ethiopia: cross-sectional community-based study. Clinicoecon Outcomes Res. (2017) 9:353–9. doi: 10.2147/CEOR.S136508
31. Fite, MB, Roba, KT, Merga, BT, Tefera, BN, Beha, GA, and Gurmessa, TT. Factors associated with enrollment for community-based health insurance scheme in Western Ethiopia: case-control study. PLoS One. (2021) 16:e0252303. doi: 10.1371/journal.pone.0252303
32. Mirach, TH, Demissie, GD, and Biks, GA. Determinants of community-based health insurance implementation in west Gojjam zone, Northwest Ethiopia: a community based cross sectional study design. BMC Health Serv Res. (2019) 19:1–8. doi: 10.1186/s12913-019-4363-z
33. Atnafu, DD, and Alemu, YA. Multidimensional determinants of willingness to pay for community-based health insurance in Ethiopia and its implication towards universal health coverage: a narrative synthesis. Prev Med Rep. (2023) 36:102474. doi: 10.1016/j.pmedr.2023.102474
34. Tahir, A. Pooled coverage of community based health insurance scheme enrolment in Ethiopia, systematic review and meta-analysis, 2016-2020. J Environ Public Health. (2022) 12:38. doi: 10.1186/s13561-022-00386-8
35. Tariku, MK, Asresie, MB, Shibesh, BF, Kado, A, and Merga, BT. Willingness to pay for community-based health insurance scheme and associated factors among rural communities in Gemmachis District, Eastern Ethiopia. Glob Health Res Policy. (2020) 12:609–18. doi: 10.2147/CEOR.S266497
Keywords: rural–urban, disparity, community-based health insurance, multivariate decomposition analysis, EMDHS 2019
Citation: Tsega Y, Alemu HT, Geberu DM, Hagos A, Jejaw M, Abera KM, Tiruneh MG, Demissie KA, Asmare L, Endawkie A, Negash WD, Workie AM, Yohannes L, Getnet M, Worku N and Belay AY (2024) Rural–urban disparity in community-based health insurance enrollment in Ethiopia: a multivariate decomposition analysis using Ethiopian Mini Demographic Health Survey 2019. Front. Public Health. 12:1361793. doi: 10.3389/fpubh.2024.1361793
Edited by:
Adam Atherly, Virginia Commonwealth University, United StatesReviewed by:
Afeez Kolawole Shittu, Federal College of Education (Special), NigeriaWondesen Teshome Bekele, Dire Dawa University, Ethiopia
Copyright © 2024 Tsega, Alemu, Geberu, Hagos, Jejaw, Abera, Tiruneh, Demissie, Asmare, Endawkie, Negash, Workie, Yohannes, Getnet, Worku and Belay. This is an open-access article distributed under the terms of the Creative Commons Attribution License (CC BY). The use, distribution or reproduction in other forums is permitted, provided the original author(s) and the copyright owner(s) are credited and that the original publication in this journal is cited, in accordance with accepted academic practice. No use, distribution or reproduction is permitted which does not comply with these terms.
*Correspondence: Yawkal Tsega, eWF3a2FsdHNlZ2FAZ21haWwuY29t