- 1Department of Acute Infectious Diseases Control and Prevention, Nanjing Municipal Center for Disease Control and Prevention, Nanjing, China
- 2Wujin District Center for Disease Control and Prevention, Changzhou, China
- 3Jiangsu Field Epidemiology Training Program, Jiangsu Provincial Centre for Disease Control and Prevention, Nanjing, China
Background: SARS-CoV-2 strains have been of great concern due to their high infectivity and antibody evasion.
Methods: In this study, data were collected on indigenous aggregated outbreaks in Nanjing from January 2020 to December 2022, caused by five strains including the original strain, the Delta variant, and the Omicron variant (BA.2, BA.5.2, and BF.7). The basic epidemiological characteristics of infected individuals were described and then parametric analysis of transmission dynamics was performed, including the calculation of incubation period, serial interval (SI), the basic reproductive number (R0), and the household secondary attack rate (HSAR). Finally, we compared the trends of transmission dynamic parameters of different strains.
Results: The incubation period for the original strain, the Delta variant, Omicron BA.2, Omicron BA.5.2, and Omicron BF.7 were 6 d (95% CI: 3.5–7.5 d), 5 d (95% CI: 4.0–6.0 d), 3 d (95% CI: 3.0–4.0 d), 3 d (95% CI: 3.0–3.0 d), and 2 d (95% CI: 2.0–3.0 d), respectively; Also, the SI of the five strains were 5.69 d, 4.79 d, 2.7 d, 2.12 d, and 2.43 d, respectively. Notably, the incubation period and SI of the five had both a progressive shortening trend (p < 0.001); Moreover, R0 of the five were 2.39 (95% CI: 1.30–4.29), 3.73 (95% CI: 2.66–5.15), 5.28 (95% CI: 3.52–8.10), 5.54 (95% CI: 2.69–11.17), 7.39 (95% CI: 2.97–18.76), with an increasing trend gradually (p < 0.01); HSAR of the five were 25.5% (95% CI: 20.1–31.7%), 27.4% (95% CI: 22.0–33.4%), 42.9% (95% CI: 34.3–51.8%), 53.1% (95% CI: 45.0–60.9%), 41.4% (95% CI, 25.5–59.3%), also with an increasing trend (p < 0.001).
Conclusion: Compared to the original strain, the incubation period and SI decreased while R0 and HSAR increased, suggesting that transmission in the population was faster and the scope of the population was wider. Overall, it’s crucial to keep implementing comprehensive measures like monitoring and alert systems, herd immunization plans, and outbreak control.
1 Introduction
Severe Acute Respiratory Syndrome Coronavirus 2 (SARS-CoV-2), first identified in December 2019, is constantly changing throughout the global pandemic of coronavirus disease 2019 (COVID-19) (1). SARS-CoV-2 has evolved from the original strain of the first epidemic to the Beta, Delta, and now the predominantly endemic Omicron strains, which are of great concern due to the increasing transmission and immune escape ability (2).
Several localized outbreaks in clusters occurred in Nanjing between 2020 and 2022, with different pathogenic strains, including the original strain, the Delta variant (B.1.617.2) and the Omicron variant (BA.2, BA.5.2, and BF.7) (3). Therefore, it is of great public health significance to optimize the prevention and control strategy, the combination of government control, and personal protection measures according to the transmission characteristics of the epidemic in Nanjing.
Transmission dynamics parameters can reflect the speed, scale, and prevention and control effects of pathogen transmission in the population, and are often used to study the transmission trends and transmission risks of COVID-19 epidemics around the world during epidemics (4–6).
In this study, we collected case information through epidemiological surveys to understand the epidemiological characteristics of the outbreaks and analyzed the transmission dynamics parameters of different strains. The aim of this study is to provide a scientific basis for the development of prevention and control strategies in the context of continuous virus mutation.
2 Methods
2.1 Data collection
We retrospectively collected data on all confirmed cases of six localized outbreaks caused by five strains of original strain, Delta variant, and Omicron variant (BA.2, BA.5.2, and BF.7) during the period of the “Dynamic Zeroing” strategy in Nanjing, Jiangsu Province, from January 2020 to December 2022, including case information, age, sex, clinical typing, vaccination status, laboratory test results, initial screening positive cycle threshold (Ct) value, onset date, initial exposure date, last exposure date, and initial screening positive date. The data in this study were from epidemiological investigations, which were updated and managed in the National Infectious Diseases Information Reporting Management System and stored in Nanjing Center for Disease Control and Prevention.
2.2 Case definition
Cases were defined according to the “Protocol for Prevention and Control of COVID-19” released by the National Health Commission of the People’s Republic of China (7), and SARS-CoV-2-infected cases were those with positive nucleic acid results using reverse transcription-polymerase chain reaction (RT-PCR) targeting ORF1ab and N genes of SARS-CoV-2. Samples were collected including nasal and oropharyngeal swabs, sputum, and other respiratory tract specimens.
2.3 Diagnostic criteria
The diagnostic criteria to determine COVID-19 severity in this study were based on the diagnosis and treatment protocol for COVID-19 issued by the National Health Commission (8, 9). Clinical classification is as follows: asymptomatic infections, moderate cases, severe cases and critical case. Confirmed cases are classified according to the severity of infection into moderate cases, severe cases and critical cases. Asymptomatic infections are characterized as individuals who test positive for the novel coronavirus through pathogen examination but do not display associated clinical symptoms or signs.
2.4 Calculation of transmission dynamics parameters
According to the case information, 36 (original strain), 72 (delta), 76 (omicron BA.2), 124 (omicron BA.5.2), and 103 (omicron BF.7) “transmission pairs” were established for the five strains of infector-infected, respectively.
The incubation period refers to the time interval between the pathogen’s invasion of an individual and the onset of symptoms (10). The incubation period was calculated using infected individuals with a single exposure in this study.
Serial interval (SI), the time between symptoms in a case and their infector (11), was calculated by R software using the fitted pairs of transmitters with a clear intergenerational transmission relationship. Of these, the original strain, Omicron BA.2 and Omicron BF.7 followed a gamma distribution, the Delta strain followed a Weibull distribution and the Omicron BA.5.2 strain followed a lognormal distribution.
Basic reproductive number (R0) measures the average number of secondary cases in a susceptible population infected by a typical primary case during the infection period (12). Referring to previous literature, this study applied an exponential growth (EG) model to calculate R0 by using the incidence curves and serial interval of infected individuals before the outbreaks were detected (3).
In addition, household secondary attack rates (HSAR), which is the percentage of secondary cases that are following primary cases among their household contacts. In this study, we collected the number of household secondary infected persons and the number of household contacts to calculate the household secondary attack rates.
2.5 Statistical analysis
Data were recorded using Excel software. Data were analyzed using R 4.1.3 software to calculate parameters including incubation period, SI, R0, and HSAR. Simple linear regression (continuous variable) and chi-squared test of trend (categorical variable) were utilized to compare the trends of transmission dynamic parameters of different strains with the time order of variation. The statistical significance level was α = 0.05.
3 Results
3.1 Characteristics of the epidemic of COVID-19
As shown in Figure 1, the six aggregated outbreaks in Nanjing reported 126 infected cases (original strain), 235 cases (Delta), 105 cases (Omicron BA.2), 44 cases (Omicron BA.5.2), 184 cases (Omicron BA.5.2) and 105 cases (Omicron BF.7). The first to last case onset dates are January 12–February 17, 2020; July 13–August 12, 2021; March 8–March 26, 2022; October 12–October 21, 2022; October 24–November 5, 2022; and November 28, 2022 (policy adjustment).
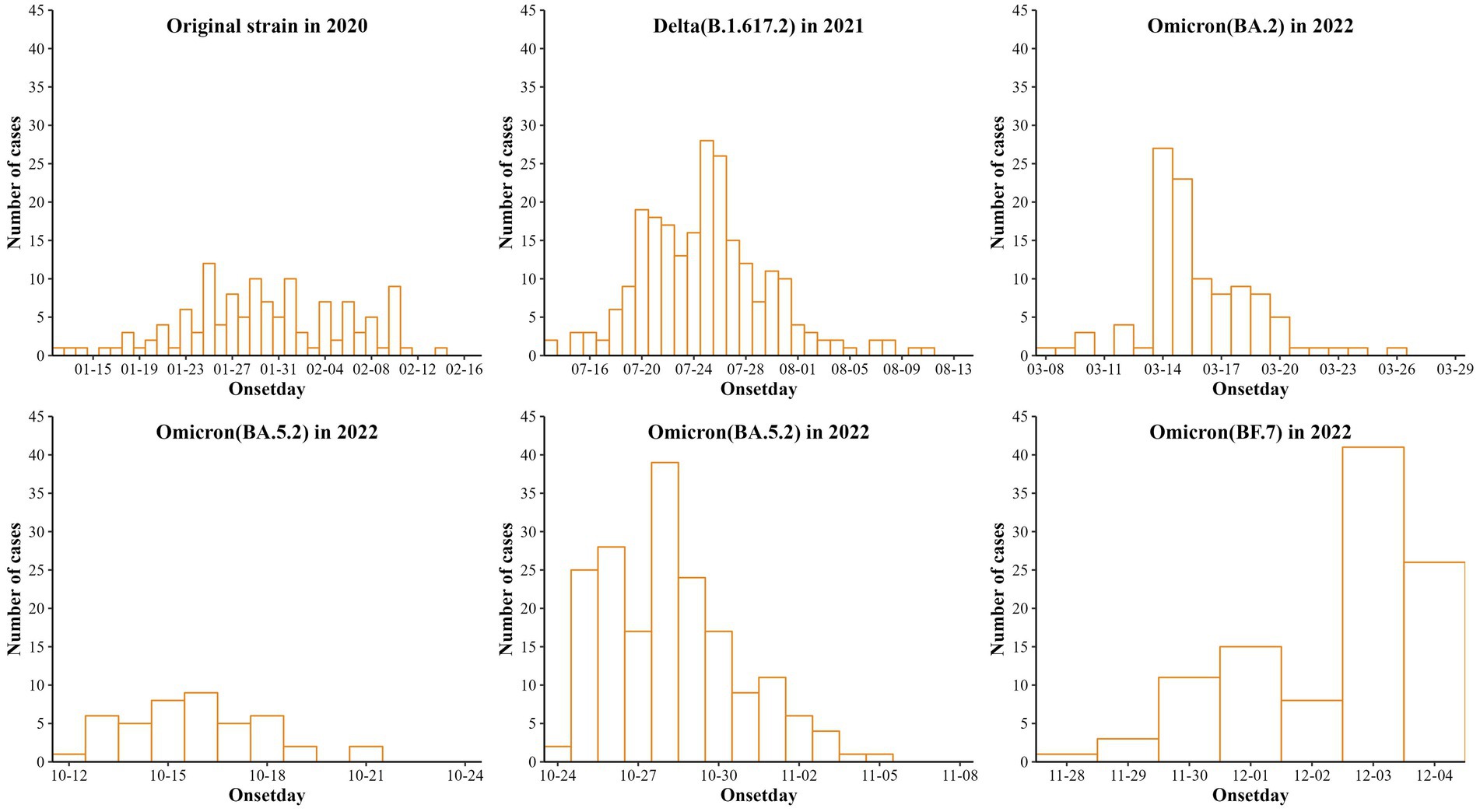
Figure 1. Reporting of six cases of aggregated outbreaks of COVID-19 caused by 5 strains in Nanjing.
Details of reported infections by strains are shown in Table 1. It also shows that the number and percentage of each sex were 65 (51.6), 94 (40.0), 44 (41.9), 32 (72.7), 127 (69.0), 50 (47.6) for males and 61 (48.4), 141 (60.0), 61 (58.1), 12 (27.3), 57 (31.0), 55 (52.4) for females. Ages [Median (IQR)] were 44 (30–58), 44 (34–53), 37 (28–56), 33 (20–46), 46 (32–57), and 60 (48–66). The number and percentage of unvaccinated persons were 126 (100.0), 55 (23.4), 11 (doi: 10.5), 3 (6.8), 8 (4.4), 14 (13.3). First positive nucleic acid Ct values were 30.2 (25.0–33.3), 24.5 (19.8–29.2), 28.9 (21.9–33.4), 32.4 (28.3–35.1), 31.1 (26.2–34.4), and 29.8 (25.3–33.2) for the N gene [Median (IQR)] and 29.8 (24.8–32.6), 25.7 (21.4–30.7), 29.1 (23.5–34.5), 33.2 (29.8–36.1), 32.1 (27.6–35.7), 30.4 (26.4–33.7) for Orf1ab gene [Median (IQR)].
3.2 Transmission dynamics parameter fitting for incubation period and serial interval (SI)
Also, the incubation periods [Median (Min-Max)] of the five strains were 6 d (2-12 d), 5 d (2-10 d), 3 d (2-6 d), 3 d (1-6 d), and 2 d (1-7 d), with 95% CI of 3.5–7.5 d, 4.0–6.0 d, 3.0–4.0 d, 3.0–3.0 d, and 2.0–3.0 d. These reflected a gradual shortening trend (t = −11.88, p < 0.001) (Figure 2). In addition, the SI [Mean (SD)] were 5.69 d (2.91 d), 4.79 d (3.47 d), 2.70 (1.29 d), 2.12 d (1.28 d), and 2.43 d (0.93 d), respectively, with a gradual shortening trend (t = −doi: 10.63, p < 0.001) (Figure 3).
3.3 Calculation of basic reproductive number (R0) and household secondary attack rates (HSAR)
In this study, the EG model was applied, using the incidence curves and SI of infected individuals before the outbreak was detected (before intervention) to calculate R0. As shown in Figure 4, the R0 of the five strains were 2.39 (95% CI: 1.30–4.29), 3.73 (95% CI: 2.66–5.15), 5.28 (95% CI: 3.52–8.10), 5.54 (95% CI: 2.69–11.17), and 7.39 (95% CI: 2.97–18.76), with a gradually increasing trend (t = 9.44, p < 0.01).
In addition, the results showed that the HSAR of the five strains were 25.5% (95% CI: 20.1–31.7%), 27.4% (95% CI: 22.0–33.4%), 42.9% (95% CI: 34.3–51.8%), 53.1% (95% CI: 45.0–60.9%), and 41.4% (95% CI: 25.5–59.3%), which were in an increasing trend (χ2trend = 32.28, p < 0.001), as detailed in Table 2.
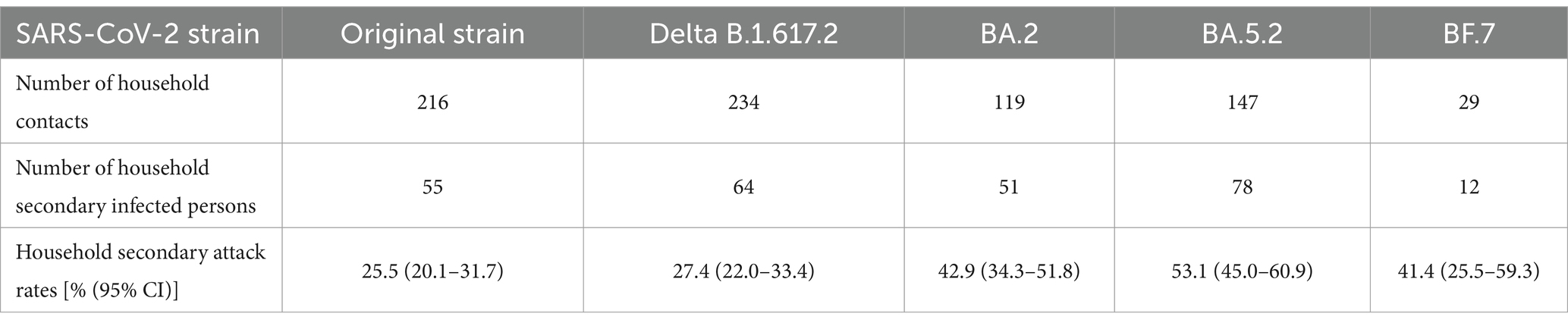
Table 2. Number of household contacts, number of household secondary infected persons, and household secondary attack rates for the five SARS-CoV-2strains.
4 Conclusion
SARS-CoV-2 have mutated significantly since their first discovery. Notably, Omicron variant strain is the most highly mutated variant and has become the most predominant epidemic strain worldwide (13, 14). Therefore, in this study, we comparatively analyzed the epidemiological characteristics and transmission dynamics parameters of local aggregated outbreaks caused by five SARS-CoV-2 variant strains in Nanjing, aiming to provide a reference for recognizing the transmission capacity of SARS-CoV-2 strains (especially the Omicron variant).
The original strain is the most common strain in 2021 in Nanjing, the most common strain is the Delta strain in 2022, and it is dominated by the different variants of Omicron in 2023. Omicron BA.5.2 and BF.7 are the main reasons for the surge in cases after mid-November 2022. In this study, the median incubation periods of five SARS-CoV-2 strains in Nanjing, i.e., the original strain, Delta, Omicron BA.2, Omicron BA.5.2, and Omicron BF.7, were found to be 6, 5, 3, 3, and 2 days. There were some differences in the incubation periods between these five types of variant infection, and the incubation period of variant BF.7 infection was much shorter than others, which is consistent with recent research found in Beijing or Shenzhen (15–17). In short, participants with Omicron had a shorter incubation period than those with the historical strains, which has been recognized in many studies (18, 19), suggesting the need for rapid response and strict disease control in the event of an epidemic, especially for Omicron.
Normally, the faster the infected person is identified and isolated, the shorter the SI is and the lower the chance of virus transmission (20). The results showed that the median SI of the five strains that caused the six aggregated outbreaks in Nanjing were 5.69, 4.79, 2.70, 2.12, and 2.43 days, respectively, which showed a trend of gradual shortening, and all of them were lower than that of the initial outbreak in Wuhan (7.5 days) (21). In conclusion, the mutation of the SARS-CoV-2 strains, the incubation period showed a trend of gradual shortening, and the speed of transmission became faster.
R0 is used as an important indicator of the transmission ability of SARS-CoV-2 (22), and the results of EG model fitting in this study showed that the R0 of the five strains were 2.39, 3.73, 5.28, 5.54, and 7.39, respectively. The results suggest that the evolved strains (Delta, Omicron BA.2, Omicron BA.5.2, Omicron BF.7) were more infectious than the original strain in Nanjing, resulting in rapid transmission of COVID-19 among susceptible populations. Accumulating studies have shown that R0 tends to decline with the development of the COVID-19 epidemic and the implementation of interventions (23, 24). This suggests that in the later stage of prevention and control, the gradually increasing R0 of the virulent strains should be taken into full consideration and appropriate interventions should be selected to control the spread of the epidemic.
In addition, family aggregation is important in epidemic prevention, control and disposal (25). The number of household contacts and the number of household secondary infected persons were collected in order to calculate the HSAR, and the HSAR of the five strains were 25.5, 27.4, 42.9, 53.1, and 41.4%, respectively, which showed an increasing trend. The estimated HSAR of the five SARS-CoV-2 variant strains in Nanjing exceed by far in Wuhan (the HASR of 15.6% between Dec 2019 and April 2020), Guangzhou (17.7% between Jan and Feb, 2020), and Beijing (23% from Feb to Mar, 2020) found by previous studies (26–28), but was similar to that in Zhejiang province (31.6% from Jan to Feb, 2020) (29). Also, HSAR estimates in mainland China have tended to be higher than those for other locations like doi: 10.5% in the USA (between Jan and Feb, 2020) and 4.6% in Taiwan (from Jan to Apr, 2020) (30, 31). The HSAR across different regions is probably due to differences in control measures, surveillance practices, and crowdedness in households. Overall, there is no doubt that persons living together in a family with longer contact time, frequent contact opportunities and closer contacts had the highest incidence rate in family aggregation of outbreaks. Therefore, with the gradual increase of HSAR, it suggests that monitoring and control should be implemented as early as possible for family members living together in order to effectively contain the spread of the epidemic.
This study has some limitations. The study only analyzed data in Nanjing until November 2022 due to the termination of large-scale nucleic acid testing. The results may have some degree of bias. Our data better represent the incidence of Nanjing, but more surveillance data are needed for the future spread and pathogenic impact.
In summary, from the original strain, Delta, to Omicron, the incubation period and SI were progressively shortened while R0 and HSAR were progressively increased, suggesting a faster spread and reaching a wider range of people in a population. Under the “Dynamic Zeroing” strategy, the rapid spread of the epidemic was effectively interrupted. The mutation of the virus has increased the pressure to prevent and control the epidemic, and comprehensive strategies such as surveillance and early warning, mass immunization, and outbreak control should continue to be implemented.
Data availability statement
The raw data supporting the conclusions of this article will be made available by the authors, without undue reservation.
Ethics statement
The epidemiological investigation was undertaken according to the Protocol for Prevention and Control of COVID-19. Individual-identifying information was not retained in analytic data sets. The studies were conducted in accordance with the local legislation and institutional requirements. Written informed consent for participation was not required from the participants or the participants’ legal guardians/next of kin in accordance with the national legislation and institutional requirements.
Author contributions
TM: Conceptualization, Formal analysis, Supervision, Writing – original draft, Writing – review & editing. CC: Formal analysis, Writing – original draft, Writing – review & editing. JW: Data curation, Formal analysis, Investigation, Writing – review & editing. HW: Data curation, Formal analysis, Investigation, Writing – review & editing. YZha: Data curation, Investigation, Writing – review & editing. YZhu: Data curation, Investigation, Writing – review & editing. ZY: Data curation, Investigation, Writing – review & editing. SD: Conceptualization, Project administration, Supervision, Writing – review & editing. JD: Conceptualization, Project administration, Supervision, Writing – review & editing.
Funding
The author(s) declare financial support was received for the research, authorship, and/or publication of this article. The Project of Nanjing Health Science and Technology Development (YKK22190) and Nanjing Key Medical Subject (Infectious Disease Prevention and Control).
Acknowledgments
We thank Nanjing Municipal Center for Disease Control and Prevention and Jiangsu Provincial Center for Disease Control and Prevention for assisting. We are very grateful to Nanjing Municipal Health Commission for public the data and all medical staff members and field workers who are working on the front line of caring for patients and collecting the data.
Conflict of interest
The authors declare that the research was conducted in the absence of any commercial or financial relationships that could be construed as a potential conflict of interest.
Publisher’s note
All claims expressed in this article are solely those of the authors and do not necessarily represent those of their affiliated organizations, or those of the publisher, the editors and the reviewers. Any product that may be evaluated in this article, or claim that may be made by its manufacturer, is not guaranteed or endorsed by the publisher.
References
1. Marjenberg, Z, Leng, S, Tascini, C, Garg, M, Misso, K, El Guerche Seblain, C, et al. Risk of long COVID main symptoms after SARS-CoV-2 infection: a systematic review and meta-analysis. Sci Rep. (2023) 13:15332. doi: 10.1038/s41598-023-42321-9
2. Klink, GV, Safina, KR, Garushyants, SK, Moldovan, M, Nabieva, E, Komissarov, AB, et al. Spread of endemic SARS-CoV-2 lineages in Russia before April 2021. PLoS One. (2022) 17:e0270717. doi: 10.1371/journal.pone.0270717
3. Wang, J, Ma, T, Ding, S, Xu, K, Zhang, M, Zhang, Z, et al. Dynamic characteristics of a COVID-19 outbreak in Nanjing, Jiangsu province. Front Public Health. (2022) 10:933075. doi: 10.3389/fpubh.2022.933075
4. Kioutsioukis, I, and Stilianakis, NI. On the transmission dynamics of SARS-CoV-2 in a temperate climate. Int J Environ Res Public Health. (2021) 18:18041660. doi: 10.3390/ijerph18041660
5. Chen, J, Chen, S, Duan, G, Zhang, T, Zhao, H, Wu, Z, et al. Epidemiological characteristics and dynamic transmissions of COVID-19 pandemics in Chinese mainland: a trajectory clustering perspective analysis. Epidemics. (2023) 45:100719. doi: 10.1016/j.epidem.2023.100719
6. He, M, Tang, B, Xiao, Y, and Tang, S. Transmission dynamics informed neural network with application to COVID-19 infections. Comput Biol Med. (2023) 165:107431. doi: 10.1016/j.compbiomed.2023.107431
7. National Health Commission of the People’s Republic of China. Protocol for prevention and control of COVID-19. (2022) Available at: http://www.nhc.gov.cn/jkj/s3577/202003/4856d5b0458141fa9f376853224d41d7.shtml.
8. National Health Commission of the People’s Republic of China. Diagnosis and treatment protocol for novel coronavirus infection. (2023) Available at: http://www.nhc.gov.cn/ylyjs/pqt/202301/32de5b2ff9bf4eaa88e75bdf7223a65a.shtml.
9. National Health Commission of the People’s Republic of China. Prevention and control protocol for novel coronavirus infection. (2023) Available at: http://www.nhc.gov.cn/xcs/zhengcwj/202301/bdc1ff75feb94934ae1dade176d30936.shtml.
10. Xu, X, Wu, Y, Kummer, AG, Zhao, Y, Hu, Z, Wang, Y, et al. Assessing changes in incubation period, serial interval, and generation time of SARS-CoV-2 variants of concern: a systematic review and meta-analysis. BMC Med. (2023) 21:374. doi: 10.1186/s12916-023-03070-8
11. Nash, RK, Bhatt, S, Cori, A, and Nouvellet, P. Estimating the epidemic reproduction number from temporally aggregated incidence data: a statistical modelling approach and software tool. PLoS Comput Biol. (2023) 19:e1011439. doi: 10.1371/journal.pcbi.1011439
12. Liu, Y, and Rocklöv, J. The reproductive number of the Delta variant of SARS-CoV-2 is far higher compared to the ancestral SARS-CoV-2 virus. J Travel Med. (2021) 28:124. doi: 10.1093/jtm/taab124
13. Torres, C, Nabaes Jodar, M, Acuna, D, Montano, RMZ, Culasso, ACA, Amadio, AF, et al. Omicron waves in Argentina: dynamics of SARS-CoV-2 lineages BA.1, BA.2 and the emerging BA.2.12.1 and BA.4/BA.5. Viruses. (2023) 15:15020312. doi: 10.3390/v15020312
14. Feng, S, Fan, Z, Zhou, K, Ma, S, Liang, M, Zhang, H, et al. Subunit vaccine raised against the SARS-CoV-2 spike of Delta and omicron variants. J Med Virol. (2023) 95:e29160. doi: 10.1002/jmv.29160
15. Cheng, YP, Kong, DF, Zhang, J, Lyu, ZQ, Chen, ZG, Xiong, HW, et al. Epidemiological characteristics of a 2019-nCoV outbreak caused by omicron variant BF.7 in Shenzhen. Zhonghua Liu Xing Bing Xue Za Zhi. (2023) 44:379–85. doi: 10.3760/cma.j.cn112338-20221031-00926
16. Gao, X, Wang, F, Liu, H, Chai, J, Tian, G, Yao, L, et al. BF.7: a new omicron subvariant characterized by rapid transmission. Clin Microbiol Infect. (2023) 30:137–41. doi: 10.1016/j.cmi.2023.09.018
17. Xu, X, Cai, YX, Liu, YH, Shen, Y, Pan, Y, Yao, H, et al. Comparison of incubation periods of infections of omicron variants BA.2 and BF.7 in Beijing. Zhonghua Liu Xing Bing Xue Za Zhi. (2023) 44:1397–401. doi: 10.3760/cma.j.cn112338-20230316-00153
18. Galmiche, S, Cortier, T, Charmet, T, Schaeffer, L, Chény, O, von Platen, C, et al. SARS-CoV-2 incubation period across variants of concern, individual factors, and circumstances of infection in France: a case series analysis from the ComCor study. Lancet Microbe. (2023) 4:e409–17. doi: 10.1016/s2666-5247(23)00005-8
19. Guo, Z, Zhao, S, Mok, CKP, So, RTY, Yam, CHK, Chow, TY, et al. Comparing the incubation period, serial interval, and infectiousness profile between SARS-CoV-2 omicron and Delta variants. J Med Virol. (2023) 95:e28648. doi: 10.1002/jmv.28648
20. Kim, T, Lee, H, Kim, S, Kim, C, Son, H, and Lee, S. Improved time-varying reproduction numbers using the generation interval for COVID-19. Front Public Health. (2023) 11:1185854. doi: 10.3389/fpubh.2023.1185854
21. Li, Q, Guan, X, Wu, P, Wang, X, Zhou, L, Tong, Y, et al. Early transmission dynamics in Wuhan, China, of novel coronavirus-infected pneumonia. N Engl J Med. (2020) 382:1199–207. doi: 10.1056/NEJMoa2001316
22. Nishiura, H, Linton, NM, and Akhmetzhanov, AR. Serial interval of novel coronavirus (COVID-19) infections. Int J Infect Dis. (2020) 93:284–6. doi: 10.1016/j.ijid.2020.02.060
23. Luo, L, and Lv, J. Mathematical modelling of virus spreading in COVID-19. Viruses. (2023) 15:15091788. doi: 10.3390/v15091788
24. Rihan, FA, Kandasamy, U, Alsakaji, HJ, and Sottocornola, N. Dynamics of a fractional-order delayed model of COVID-19 with vaccination efficacy. Vaccines. (2023) 11:11040758. doi: 10.3390/vaccines11040758
25. Chaw, L, Koh, WC, Jamaludin, SA, Naing, L, Alikhan, MF, and Wong, J. Analysis of SARS-CoV-2 transmission in different settings, Brunei. Emerg Infect Dis. (2020) 26:2598–606. doi: 10.3201/eid2611.202263
26. Jing, QL, Liu, MJ, Zhang, ZB, Fang, LQ, Yuan, J, Zhang, AR, et al. Household secondary attack rate of COVID-19 and associated determinants in Guangzhou, China: a retrospective cohort study. Lancet Infect Dis. (2020) 20:1141–50. doi: 10.1016/s1473-3099(20)30471-0
27. Li, F, Li, YY, Liu, MJ, Fang, LQ, Dean, NE, Wong, GWK, et al. Household transmission of SARS-CoV-2 and risk factors for susceptibility and infectivity in Wuhan: a retrospective observational study. Lancet Infect Dis. (2021) 21:617–28. doi: 10.1016/s1473-3099(20)30981-6
28. Wang, Y, Tian, H, Zhang, L, Zhang, M, Guo, D, Wu, W, et al. Reduction of secondary transmission of SARS-CoV-2 in households by face mask use, disinfection and social distancing: a cohort study in Beijing, China. BMJ Glob Health. (2020) 5:e002794. doi: 10.1136/bmjgh-2020-002794
29. Sun, WW, Ling, F, Pan, JR, Cai, J, Miao, ZP, Liu, SL, et al. Epidemiological characteristics of COVID-19 family clustering in Zhejiang Province. Zhonghua Yu Fang Yi Xue Za Zhi. (2020) 54:625–9. doi: 10.3760/cma.j.cn112150-20200227-00199
30. Burke, RM, Midgley, CM, Dratch, A, Fenstersheib, M, Haupt, T, Holshue, M, et al. Active monitoring of persons exposed to patients with confirmed COVID-19 - United States, January-February 2020. MMWR Morb Mortal Wkly Rep. (2020) 69:245–6. doi: 10.15585/mmwr.mm6909e1
Keywords: COVID-19, SARS-CoV-2, transmission dynamics, parametric analysis, indigenous aggregated outbreaks
Citation: Ma T, Chen C, Wang J, Wang H, Zhao Y, Zhu Y, Yan Z, Ding S and Ding J (2024) Parametric analysis of the transmission dynamics during indigenous aggregated outbreaks caused by five SARS-CoV-2 strains in Nanjing, China. Front. Public Health. 12:1358577. doi: 10.3389/fpubh.2024.1358577
Edited by:
Amira Adel Taha Abdel Aleem AL-Hosary, Assiut University, EgyptReviewed by:
Mahmoud Mohamed Shehata, University of Giessen, GermanyAmal Sayed, Assiut University, Egypt
Copyright © 2024 Ma, Chen, Wang, Wang, Zhao, Zhu, Yan, Ding and Ding. This is an open-access article distributed under the terms of the Creative Commons Attribution License (CC BY). The use, distribution or reproduction in other forums is permitted, provided the original author(s) and the copyright owner(s) are credited and that the original publication in this journal is cited, in accordance with accepted academic practice. No use, distribution or reproduction is permitted which does not comply with these terms.
*Correspondence: Songning Ding, c29uZ25pbmcxMTI3QDE2My5jb20=; Jie Ding, eXUyYW4yMDAyQDE2My5jb20=
†These authors share first authorship