- 1Eye Institute and Department of Ophthalmology, Eye & ENT Hospital, Fudan University, Shanghai, China
- 2NHC Key Laboratory of Myopia (Fudan University), Key Laboratory of Myopia, Chinese Academy of Medical Sciences, Shanghai, China
- 3Shanghai Research Center of Ophthalmology and Optometry, Shanghai, China
- 4Shanghai Engineering Research Center of Laser and Autostereoscopic 3D for Vision Care, Shanghai, China
- 5Department of Nursing, Eye & ENT Hospital, Fudan University, Shanghai, China
- 6Center for Optometry and Visual Science, Guangxi Academy of Medical Sciences, Nanning, China
Background: Myopia poses a global health concern and is influenced by both genetic and environmental factors. The incidence of myopia tends to increase during infectious outbreaks, such as the COVID-19 pandemic. This study examined the screen-time behaviors among Chinese children and adolescents and investigated the efficacy of artificial intelligence (AI)-based alerts in modifying screen-time practices.
Methods: A cross-sectional analysis was performed using data from 6,716 children and adolescents with AI-enhanced tablets that monitored and recorded their behavior and environmental light during screen time.
Results: The median daily screen time of all participants was 58.82 min. Among all age groups, elementary-school students had the longest median daily screen time, which was 87.25 min and exceeded 4 h per week. Children younger than 2 years engaged with tablets for a median of 41.84 min per day. Learning accounted for 54.88% of participants’ screen time, and 51.03% (3,390/6,643) of the participants used tablets for 1 h at an average distance <50 cm. The distance and posture alarms were triggered 807,355 and 509,199 times, respectively. In the study, 70.65% of the participants used the tablet under an illuminance of <300 lux during the day and 61.11% under an illuminance of <100 lux at night. The ambient light of 85.19% of the participants exceeded 4,000 K color temperature during night. Most incorrect viewing habits (65.49% in viewing distance; 86.48% in viewing posture) were rectified swiftly following AI notifications (all p < 0.05).
Conclusion: Young children are increasingly using digital screens, with school-age children and adolescents showing longer screen time than preschoolers. The study highlighted inadequate lighting conditions during screen use. AI alerts proved effective in prompting users to correct their screen-related behavior promptly.
1 Introduction
In recent decades, the global electronics industry has developed rapidly, accelerating the advent of the digital information age. Various digital products, including desktop computers, laptops, digital televisions, and smartphones, affect nearly all aspects of people’s lives. For instance, traditional forms of work, learning, social communication, and entertainment are being replaced by telecommuting, remote courses, cyber communication, and online entertainment, respectively. The use of digital devices is efficient and convenient; however, it prolongs near work and screen time, leading to video display terminal syndrome (or computer vision syndrome) (1–3).
The COVID-19 pandemic led to widespread school closures and in-house quarantines, which popularized remote education, telecommuting, and online entertainment. Unfortunately, this has also led to an increase in myopia worldwide (4–6). Studies have shown that school-aged children experienced a myopic refractive shift during the pandemic compared to the period before (7). Furthermore, myopia progression has accelerated in terms of spherical equivalent refractive (−0.83D) and axial length (0.36 mm) (8). Various environmental factors, such as increased digital screen time, near work, and limited outdoor and dim-light exposure, are considered significant risks of myopia onset and progression in children and adolescents, except for genetic factors (9, 10). Moreover, using digital screens at a short distance, with poor posture, under poor illumination, or improper color temperature increases the incidence of myopia (11, 12), strabismus (13), anisometropia (2), postural scoliosis (14), and abnormalities in musculoskeletal development among youth (15). However, children and adolescents often use electronic devices in an unsupervised environment, and thus, little is known about their screen-time behaviors, such as daily usage schedules, duration, frequency, viewing content, viewing posture, and viewing distance, and the ambient light environments, such as illuminance and color temperature.
This study employed commercially available artificial intelligence (AI)-enhanced tablets to map screen-time behaviors and luminous environments in Chinese children and adolescents during the COVID-19 pandemic. Furthermore, this study investigated the efficacy of AI-based visual and audible alerts in screen-time behavior correction among children and adolescents.
2 Methods
2.1 Participants and ethical approval
The study was conducted following the principles of the Declaration of Helsinki. Informed consent was obtained from all participants and their legal guardians, who agreed to disclose the identifying information in an online open-access publication. The study protocol was approved by the Ethics Committee of the Fudan University Eye and ENT Hospital Review Board (No. 2022113).
In the cross-sectional study, participants were users of commercially available AI-enhanced tablets (manufactured by BOE Yiyun Technology Co., Ltd., Changsha, Hunan Province, China; Model A127CS 6 + 128G) between October 9, 2022 and December 31, 2022. Participants aged over 18 years were excluded. Anonymous electronic survey questionnaires were sent to the participants and their legal guardians to verify the valid use of the tablets and collect information on the age and gender of the recruited children and adolescents. The tablets continuously recorded behavioral characteristics, including screen-time duration, frequency, content, viewing distance, body posture, head position, eye position, blinking frequency, and parameters of luminous environments (illumination and color temperature) during their use from October 9, 2022, to December 31, 2022. Data was collected from 6,716 children and adolescents (3,526 boys and 3,190 girls). Following the elimination of participants with missing data, data from 6,643 children and adolescents (3,485 boys and 3,158 girls, mean age 6.1 ± 3.0 years, 0–18 years old) were collected from 25 provinces and 4 municipalities across mainland China. No participants were infected during this period, except for those in Shanghai (n = 123) who were infected within their households. This was attributed to the liberalization of epidemic control measures in December 2022. Participants were divided into five groups according to age: participants aged from 0 to 2 years (before preschool) were enrolled in Group BPS, 3 to 5 years (preschool) in Group PS, 6 to 11 years (elementary school) in Group ES, 12 to 14 years (junior high school) in Group JHS, and 15 to 18 years (senior high school) in Group SHS.
2.2 Equipment
The tablets were 279 × 215.4 × 7.2 mm in size equipped with a capacitive touch screen (12.7 in) for display and interaction, main front digital camera (16 megapixels, G127, DMEGC) for photography and AI action recognition, secondary front digital camera (5 megapixels, G127, DMEGC) for posture monitoring, rear digital camera (13 megapixels, CL3A872 V2, C&T), flash, range sensor (ranging from 1 to 250 cm, TMF 8805, ams), and ambient light sensor (TCS3707, ams, Figure 1A). For privacy protection, only the outlines of the body, head and eyes were extracted and analyzed.
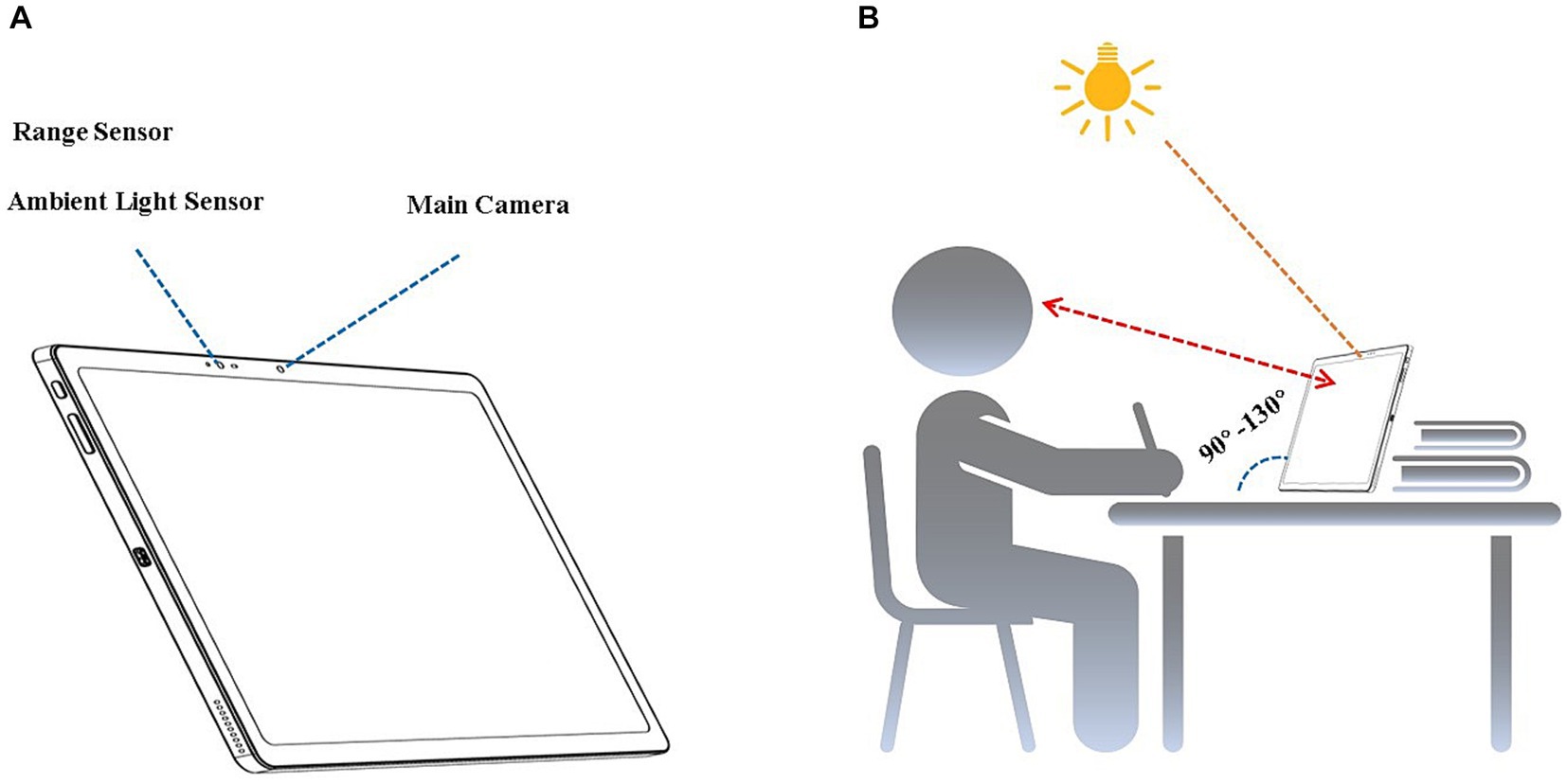
Figure 1. Equipment and measurements. (A) Artificial intelligence-enhanced tablet computer. (B) Viewing distance, posture, ambient illumination, and color temperature monitoring.
2.3 Screen-time duration and period
After each user logged into their account, the tablet usage time was accumulated when the camera obtained a stable image of their facial outline. The average daily and weekly tablet usage times were calculated using Eqs 1, 2:
Sixty-eight applications (apps) with the most usage during the study period were classified into social networking, search engines, entertainment, and education apps. The duration of use (accumulated usage time) and time intervals (morning, 6:00–11:00; noon, 11:00–13:00; afternoon, 13:00–16:00; evening, 16:00–18:00; night, 18:00–24:00; and before dawn, 0:00–6:00) were recorded for each category.
2.4 Ambient illumination and color temperature
The ambient light sensor measured the ambient illumination and color temperature during screen time. The ambient illuminance values obtained during three-time intervals (daytime, 8:00 to 20:00; nighttime, 20:00 to 8:00; and the entire day) were, respectively, divided into four illumination intervals (rows): (0 lux, 100 lux), [100 lux, 300 lux), [300 lux, 1,000 lux), [1,000 lux, 4,000 lux]. In each time interval, the exposure time ratio of each illuminance interval was calculated as.
The color temperature values (kelvin, K) obtained during the three-time intervals were divided into four intervals: (0 K, 4,000 K), [4,000 K, 6,000 K), [6,000 K, 8,000 K), and [8,000 K, +∞ K). In each time interval, the exposure time ratio of each color temperature interval was calculated as.
2.5 Viewing distance
The viewing distance was measured using a range sensor combined with the tablet’s main front camera. After the main camera captured the facial outline, the range sensor obtained the distance between the screen and face plane every 3 s. The viewing-distance data were considered valid only when the angle between the screen and desktop was 90° to 130° (Figure 1B). The average viewing distance was calculated and analyzed. When the viewing distance was detected to be less than 30 cm for 12 s, and an audio alert was played for 10 s to remind users to maintain a sufficient distance during screen time. If the user corrected the viewing distance to beyond 30 cm in 10 s, the alert was stopped and recorded as effective. If the user ignored the alert, a pop-up notification appeared on the screen, interrupting the user’s interaction with the tablet until the user adjusted the viewing distance to beyond 35 cm. The audio and pop-up notifications were recorded, and the effective alert ratio was calculated.
2.6 Blink frequency
Eye blinks, lasting between 50 to 500 ms (16), represent brief eyelid closures. Blink frequency, measured as the number blinks per minute, was assessed using the primary front camera. This camera continuously observed users’ pupil and eyelid movements, with AI models detecting blink occurrences. Blink actions that persisted for over 1 min were documented.
2.7 Identification of viewing posture and alerting
Head posture refers to the position and orientation of the three-dimensional space of the head. Head postures are described by the egocentric rotation angles of pitch, roll, and yaw (17). Using a low-resolution thermal map regression model, semi-supervised learning with a self-training algorithm was employed. To increase the robustness of the model, generative adversarial networks (GANs) were used to generate facial data for different ages, skin colors, and angles. A total of 300,000 images (100,000 labeled and 200,000 unlabeled) were included in the training set and 10,000 images in the testing set (Figure 2).
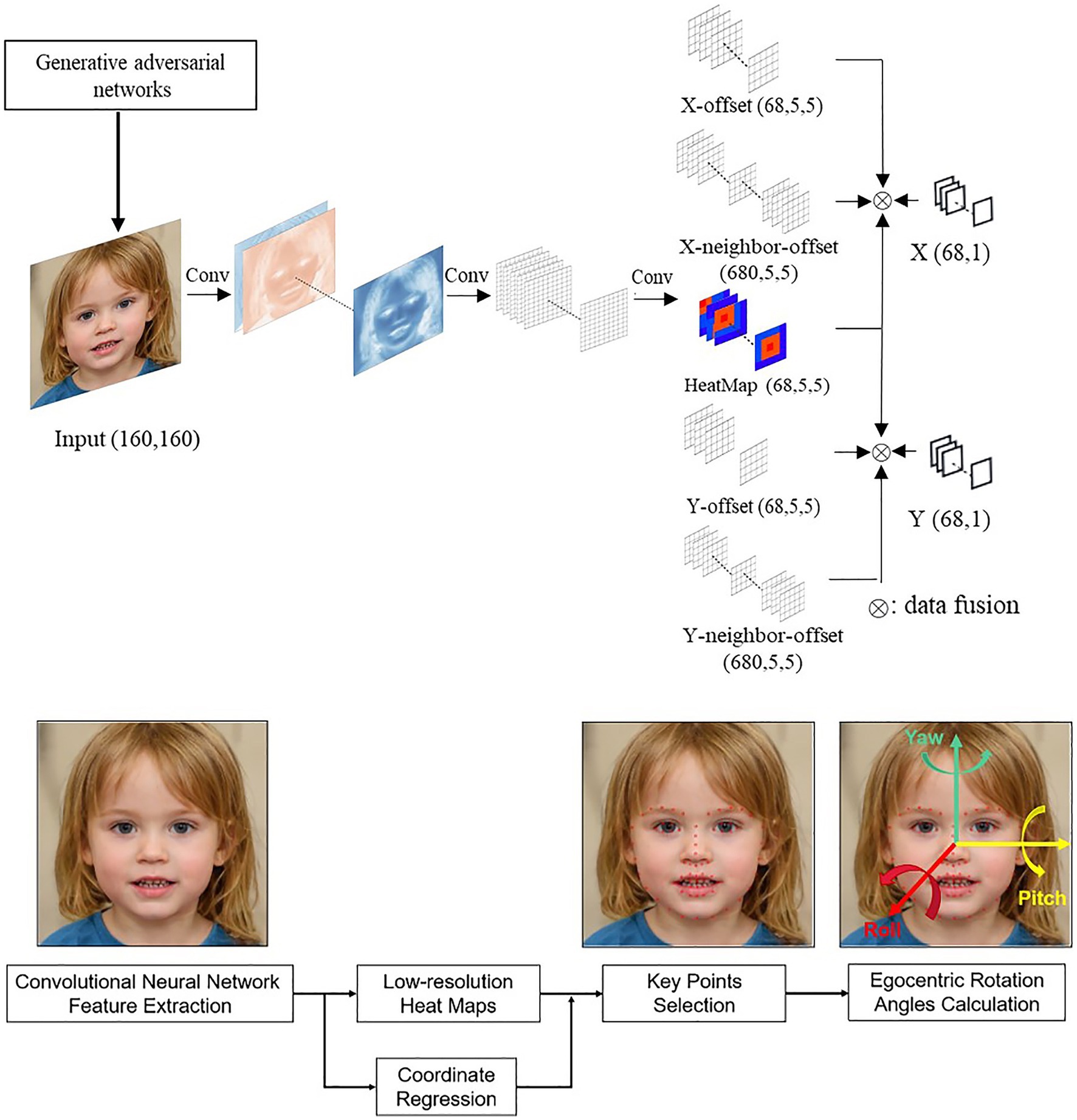
Figure 2. Artificial intelligence model: Identify and Alert poor postures and improper viewing distance. The top flowchart is the generative adversarial network to coordinate the offset of the X and Y dimensions. The bottom flowchart is the convolutional neural network to compute egocentric rotation angles.
In this study, the head was considered to be tilted when the roll angle exceeded ±30° for more than 12 s. When a tilted head was detected, an audio alert reminded the user to correct their head posture within the next 10 s. If the user ignored the alert, a pop-up notification appeared on the screen to interrupt the tablet use until the user corrected their head pose. The total number of audio alerts and pop-up notifications were recorded, and the effective alert ratio was calculated.
2.8 Statistical analysis
Statistical analyses were performed using SPSS version 20.0. Data were presented as mean ± standard deviation. Mixed-effects models were used to analyze differences in usage time and viewing distance, with age brackets as the intra-group factors, as well as the blink frequency, changed over time. R × C chi-square tests were also employed to compare the difference in the effective alert ratios of viewing distance and head posture among age brackets.
3 Results
3.1 Demographics
Among the 6,643 Chinese children and adolescents recruited for this study, Groups BPS, PS, ES, JHS, and SHS included 607, 2,370, 3,370, 204, and 92 participants, respectively. The Gross Domestic Product (GDP) values corresponding to the geographical areas where these participants were located, as well as the average age and gender of participants from each area, are listed in Table 1.
3.2 Usage time
The median daily usage time and sample sizes of each group are listed in Table 2. A significant difference in daily usage time was observed among the five groups (F = 255.72, p < 0.001). Bonferroni post hoc tests showed that the daily usage time of Group ES was significantly longer than that of the other groups (all post hoc p < 0.05); the daily usage times of Groups JHS and SHS were the second longest (post hoc p = 0.354) and longer than those of Groups BPS and PS (all post hoc p < 0.001). The daily usage times of Groups BPS and PS were similar (post hoc p = 1.000).
The weekly usage time of all the groups is listed in Table 3. The median weekly usage time of Groups ES (562.03 min), JHS (566.73 min), and SHS (425.35 min) were significantly longer than those of Groups BPS (all post hoc p ≤ 0.001) and PS (all post hoc p < 0.001). However, no significant differences were detected in the weekly usage time between Groups BPS and PS (post hoc p = 1.000) and Groups ES, JHS, and SHS (all post hoc p > 0.05).
3.3 Luminance and ambient lighting color temperature
Figure 3 lists the findings of the tablet computer’s ambient light sensor, indicating that during the day, luminance levels fell below 100 lux for 36.16%, between 100–300 lux at 34.49%, 300–1,000 lux at 21.78% and above 1,000 lux at 7.57% of the screen time. During the night, these levels fell below 100 lux at 61.11%, between 100–300 lux at 25.02%, 300–1,000 lux at 9.74% and above 1,000 lux at 4.13% of the screen time. Figure 4 details the distribution of ambient lighting color temperature during screen time. In daytime conditions, the color temperature was below 4,000 K at 8.22%, between 4,000- 6000 K at 63.89%, 6,000-8000 K at 24.87% and above 8,000 K at 3.02% of the screen time. During nighttime usage, it was below 4,000 K at 14.91%, between 4,000-6000 K at 49.14%, 6,000-8000 K at 31.01% and above 8,000 K at 4.94% of the screen time.
3.4 Viewing content
The top 48 most used applications (apps) were filtered from 5,424 apps and classified into 4 categories: social networking, search engines, entertainment, and education. Their session durations were recorded (Figure 5). Educational applications accounted for 54.88% (3,646/6,643) of the sessions over the entire day (ranked first in all categories), including the most significant proportion of all session durations except for the before-dawn period, in which entertainment applications ranked first (48.33%; 478/988).
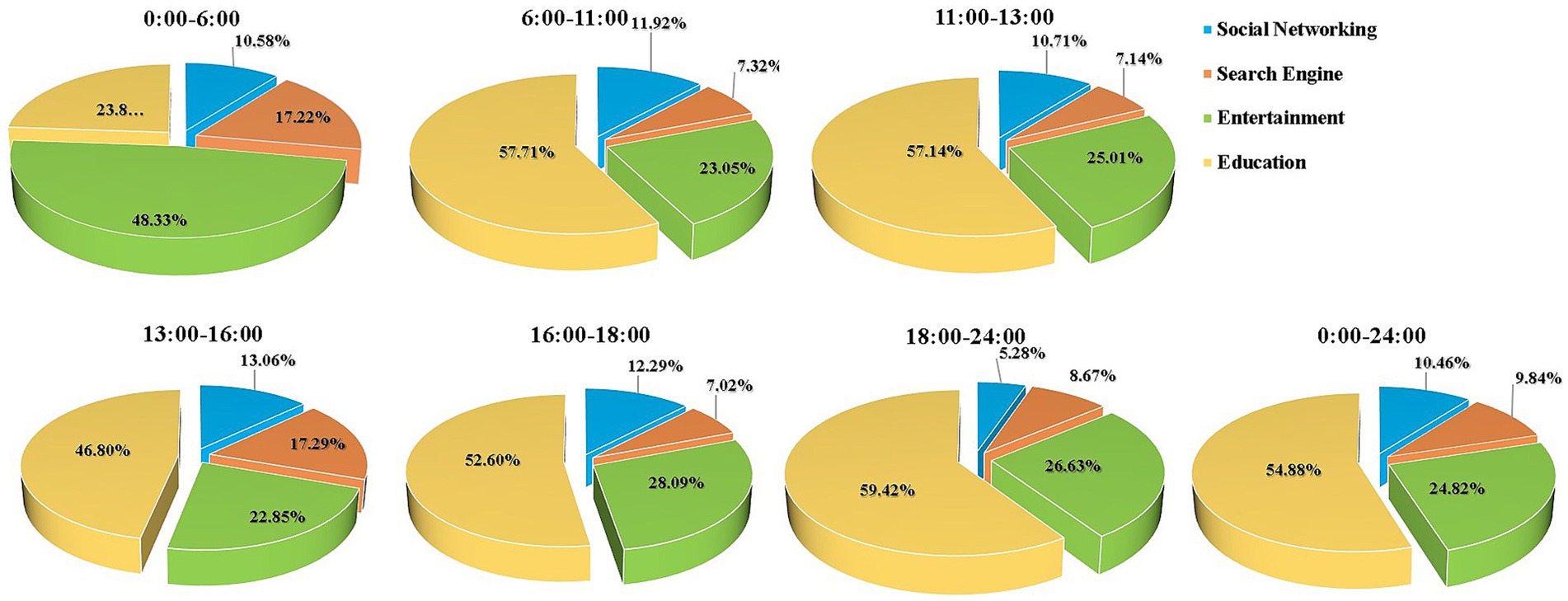
Figure 5. View content at different times of the day (social networking, search engine, entertainment, and education).
3.5 Blinking rate
Table 4 presents the blinking rates observed across six-time slices for each age group.
These rates exhibited minor fluctuations over time for each age group (p > 0.05). However, significant variations were noted among age groups during the following time slices: 0–10 min (F = 38.502, p < 0.001), 10–20 min (F = 54.388, p < 0.001), 20–30 min (F = 39.553, p < 0.001), 30–40 min (F = 29.567, p < 0.001), 40–50 min (F = 35.385, p < 0.001), and 50–60 min (F = 25.138, p < 0.001). Bonferroni post hoc comparisons revealed that the blinking rates of Groups ES and JHS were significantly higher than those of Groups BPS and PS in all six-time slices (all post hoc p < 0.01). However, no significant difference in blinking rates were observed between Groups BPS and PS (all post hoc p > 0.05) or among Groups ES, JHS, and SHS (all post hoc p > 0.05) in all-time slices.
3.6 Efficacy of AI models in viewing distance and posture alert
Tables 5, 6 show that 6,378 and 6,403 participants used viewing distance and posture monitoring, respectively. Of the subjects, 83.76% (5,564/6,643) used the tablets with an average viewing distance of less than 50 cm, 60.71% (4,033/6,643) used the tablets continuously for 1 h, and 51.03% (3,390/6,643) used the tablets continuously for 1 h with a viewing distance of less than 50 cm. In total, 807,355 and 509,199 improper viewing distance and posture alerts were triggered, respectively. The ES group had the highest per-capita alerts (479.58) of viewing distance among all groups. The effects of viewing-distance correction among the groups differed significantly (χ2 = 1,174.165, p < 0.001). The efficacy of viewing-distance correction in the JHS group (324,269/479,567; 69.67%) was the highest of all groups (all post hoc p < 0.05), whereas that in Group BPS (25,442/41,409; 61.44%) was the lowest (all post hoc p < 0.05). The per-capita posture alerts were the highest in Group BPS (90.58). The efficacy of the AI alerts in posture correction differed among the groups (χ2 = 2,519.789, p < 0.001). The effectiveness of the posture correction in Groups ES (235,254/267,951; 87.80%) and SHS (5,238/5,984, 87.62%) were significantly higher than those in the other groups (all post hoc p < 0.05). The difference in efficacy between Groups ES and SHS was non-significant (post hoc p > 0.05).
4 Discussion
4.1 Emergence of electronic screen usage in younger children
During the COVID-19 pandemic, most educational institutions substituted online courses for in-person classes. Students studied at home using electronic devices. As most outdoor activities were restricted, leisure time was filled with online entertainment, and the duration of near work dramatically increased. This study found that 0–2-year-old children used tablets for more than 40 min per day on median, violating the recommendation of the American Academy of Pediatrics to avoid digital media use (except video chatting) in children younger than 18 to 24 months (18).
Moreover, excessive use of digital screens in early childhood may delay the development of cognition, language, and emotions (2). The median screen time for elementary-school students was more than 4 h (315.86 min) per week, and the median daily screen time approached 1.5 h (87.25 min), which is the longest among all age groups. This finding differs from the results of Wang et al.’s investigation (19). Saxena et al. (20) reported that children and adolescents who use electronic screens for more than 4 h per week are at more than eight times higher risk of contracting myopia than those who do not use electronic screens. A systematic review and meta-analysis indicated that the prolonged use of electronic screens could significantly increase the axial eye length and risk of myopia (21). Furthermore, excessive electronic screen time may replace outdoor activity time, reduce natural light exposure, increase the risk of obesity (22), and decrease sleep quality (23), increasing the risk of myopia occurrence and progression.
4.2 Prolonged use of electronic screens at close range by children and adolescents
Regarding the rhythm and viewing distance of tablet usage, our findings demonstrate that the vast majority of participants (83.76%; 5,564/6,643) had an average distance of less than 50 cm, and more than half of the participants (51.03%; 3,390/6,643) used the tablets continuously for 1 h at such viewing distances. The distance alarm was triggered 807,355 times. A study using apps to record the smartphone usage time of teenagers showed that in the group with fewer outdoor activities, the myopic diopter was lower for those who rested every 20 min after watching the screen (24). Additionally, prolonged use of electronic display devices at close distances can cause asthenopia. The incidence of dry eye syndrome in children has reached 50–60%, and its symptoms include dry eyes, foreign body sensation, tears, blurred vision, headache, esotropia, and abnormal vision. A study of 52 children in grades 3–4 revealed that the level of asthenopia was lowest when the screen was approximately 50 cm from the eyes (3). Reid et al. (25) proposed that children and adolescents follow the 20–20-20 principle while using digital products: rest and look at an object 20 feet away for 20 s after using the devices for 20 min. According to a recent study, it is recommended to rest and look further for more than 5 min to relieve asthenopia (26).
4.3 Increased blink frequency in school-aged children compared to preschoolers during screen use
Decreased blinking rates, increased tear evaporation, and aggravation of dry eye symptoms are significant contributors to asthenopia when individuals focus on screens. An investigation involving children in grades 3 and 4 revealed that blinking rates increased after an average of 15 min of tablet usage and were positively correlated with the duration of screen time (3). This study also identified notable differences in blinking rates among various age groups. Specifically, elementary, middle, and high school students, who spent more time on screens, exhibited significantly higher blinking rates than preschool and infant groups with shorter screen exposure. These findings align with those of Feng et al. (3), suggesting that the intensity of daily near work was greater for elementary, middle, and high school students. However, during the initial hour of screen time, the blinking rate in each age group fluctuated only slightly, with no statistically significant differences. This contrasts the results reported by Feng et al. (3). One possible explanation for this disparity is that our study’s baseline blinking rate, averaging 14 times per minute, was notably higher than the 8–10 times per minute reported by Feng et al. (3). Second, the blinking rate of each participant was measured in their homes, where they may have engaged in other near work activities, such as traditional reading and homework, prior to electronic screen use. Finally, variations in temperature, humidity, and illuminance conditions at home compared to laboratory settings may introduce confounding factors affecting blinking rates.
4.4 Children and adolescents use of electronic screens in low-illuminance environments
This study discovered that over 50% of the time was spent on learning, except during the pre-dawn period. This finding is consistent with those of Ma et al. (27) and Kohmarn et al. (28). In the present study, more than 70% of the children and adolescents used tablets with illuminance below 300 lux during the day, more than 85% of them used tablets with illuminance below 300 lux and more than 60% of them used tablets with illuminance below 100 lux at night. In addition, traditional paper-based work was often involved. These findings indicate insufficient home illumination, which is consistent with the results of cloud clip (29) monitoring of daily white light exposure (253 ± 36 lux) and light at eye level at night (139.6 ± 82.7 lux) (30). According to the dark-focus theory, the refractive state of the human eye will drift toward myopia in a dim-light environment, which necessitates engaging in proximate tasks such as reading and writing (31, 32). Furthermore, low illumination can easily cause a decrease in contrast on paper media, which reduces accommodation accuracy, causes blurred vision, aggravates asthenopia, and even induces myopia (33). In addition, an electronic screen is a self-luminous medium, and a low-ambient light and luminous display screen will lead to uneven space illumination and glare.
4.5 Children and adolescents use of electronic screens under ambient light with high color temperature at night
When monitoring the light environment with electronic screens, we found that for more than 85% of the participants, the ambient light exceeded 4,000 K color temperature at night, and it exceeded 6,000 K color temperature for more than 35% of the participants. Several studies (34, 35) have suggested that high-color temperature lighting at night disrupts the melatonin secretion cycle, influences sleep in children and adolescents, and adversely affects physical and psychological development. Sleep deprivation and poor sleep quality are risk factors for myopia occurrence and progression. In addition, the content displayed by the tablet computer inevitably contains high color temperature information, which can affect the hormone secretion rhythm in a specific period. We found that 1,612 participants used the tablets in the early morning (midnight to 6:00), whereas during lunch (11:00–13:00) and dinner (16:00–18:00), more than 6,000 of the total 6,643 participants were still recorded using electronic screens; however, the cumulative time was significantly lower than that in the morning, afternoon, and evening. The American Children’s Association recommends that children and adolescents avoid using electronic devices before and after meals and 1 h before sleep (18). The vast majority (more than 90%) of the participants in this study violated this recommendation. The content on electronic screens in the early morning was primarily focused on entertainment. This period is likely to be without parental supervision. Therefore, it is necessary to assist parents in managing the light environment, duration, and content on children’s and adolescents’ electronic screens.
4.6 Effective use of AI in correcting poor screen-use behaviors among children and adolescents
In this study, most children and adolescents received correction alerts for viewing distance (84.81%;5,409/6,378) and viewing posture (84.97%;5,441/6,403), respectively, while using tablets. This indicated that this demographic generally exhibits incorrect viewing behavior when using electronic screens. Notably, elementary school students received the highest number of alerts for viewing distance (479.58 times per person). This trend could be attributed to the substantial increase in learning tasks during this developmental stage, coupled with a potential lack of cognitive maturity, self-discipline, and healthy near work habits. Previous research (19) has found that the growth rate of myopia in children in lower-grade elementary schools during the COVID-19 period was the highest. This indicates that children aged 6–8 years may be especially responsive to environmental factors, heightened use of eyes in close proximity indoors, and decreased outdoor activities, all of which affect the onset and progression of myopia. This observation is also supported by Vander Veen et al. (36). In our study, most behaviors (65.49% for viewing distance and 86.48% for viewing posture) were corrected within 10 s after the AI alerts, with the highest correction rate in the middle school group (69.67%; 10,871/15,604) and the lowest correction rate in the before preschool group (61.44%; 164,947/254,870); this indicated that the application of AI can help monitor and correct unhealthy behaviors on electronic screen usage in children and adolescents; and older children and adolescents, such as middle school students, are able to respond more promptly and proactively under reminders to correcting bad viewing behaviors when using electronic screens, which might be correlated with their more complete cognitive and autonomous behavior control and correction abilities than younger preschool children. Therefore, it is recommended that parents provide more intensive supervision and guidance to younger children when they use electronic screens.
4.7 Practical implications
The findings of this study underscore the potential of AI technologies in assisting parents to monitor, warn, correct poor behaviors of near work and develop good viewing habits in children using electronic screens. These technologies have the capacity to facilitate the development of healthy viewing habits among children, thus helping to anticipate and mitigate the risk factors associated with children’s eye health. This may pave the way for novel approaches to myopia prevention and control, enabling parents to intervene proactively. As a result, there is a transition from clinical settings to the home environment in the prevention and management of myopia. Furthermore, this study lays the groundwork for the establishment of standards and guidelines on eye health care for children who use electronic screens. These guidelines encompass aspects such as viewing distance, view time, indoor ambient lighting illuminance, and color temperature in both home or classroom, for children and adolescents using electronic screens. Such standards contribute to the normalization of intelligent electronic screens for children and adolescents, fostering advancements in related industries.
4.8 Strengths and limitations
This study used the novel method of AI identification and monitoring to objectively measure the data of incorrect viewing behaviors and ambient light parameters during screen time among children and adolescents. And the inclusion of participants from over 20 provinces and municipalities ensured a diverse and geographically widespread representation of samples. However, the sample sizes extracted from different regions differed considerably, and the deviation between the sampling results and the population may be significant. Moreover, data were collected during the COVID-19 pandemic. The time, duration, and rhythm of children’s and adolescents’ screen time, like more online courses and longer continuous electronic screen usage because of more time at home, etc., differed from those during the normal period. After the relaxation of epidemic restrictions, 1.83% of the randomly selected sample were found to be infected. This may have led to a slight reduction in screen time for this group. Last, this study only monitored and investigated the screen-time behavior of children and adolescents during the use of BOE devices and did not monitor the participants’ use of other electronic devices, such as mobile phones, TVs, desktop computers, and tablets of other brands.
5 Conclusion
In summary, children of younger ages have started using electronic screens. Screen time for school-age children and adolescents with a closer viewing distance and higher incidence of poor viewing posture was longer than that for preschool children. During screen time, illumination was insufficient, paired with a high color temperature at night. AI can effectively monitor the time, content, and light environment during screen time and remind children and adolescents to correct their poor posture and behavior. Future research endeavors should consider adopting more rigorous sampling techniques, extended monitoring and observation periods, and the inclusion of supplementary online reporting for comprehensive data collection. This could involve gathering additional data on aspects such as time spent on other electronic devices, among other relevant factors.
Data availability statement
The original contributions presented in the study are included in the article/supplementary material, further inquiries can be directed to the corresponding authors.
Ethics statement
The studies involving humans were approved by the Ethics Committee of the Fudan University Eye and ENT Hospital Review Board. The studies were conducted in accordance with the local legislation and institutional requirements. Written informed consent for participation in this study was provided by the participants' legal guardians/next of kin.
Author contributions
JW: Data curation, Formal analysis, Funding acquisition, Writing – original draft, Project administration. YS: Conceptualization, Funding acquisition, Writing – original draft. JZ: Formal analysis, Writing – original draft. XW: Conceptualization, Writing – review & editing. ZC: Funding acquisition, Writing – review & editing. TH: Formal analysis, Writing – original draft. YH: Formal analysis, Writing – original draft. YW: Data curation, Writing – original draft. WZ: Formal analysis, Funding acquisition, Writing – review & editing. WW: Formal analysis, Writing – review & editing. XZ: Funding acquisition, Supervision, Writing – review & editing. YX: Conceptualization, Supervision, Writing – review & editing.
Funding
The author(s) declare financial support was received for the research, authorship, and/or publication of this article. This study was funded by: National Natural Science Foundation of China (Grant No. 82101183; 82171095; 82360214); Research Project of Shanghai Municipal Health Commission (Grant No. 202340243); Nursing Research Fund of Fudan University (Grant No. FNF202234); Key Discipline Program of Sixth Round of the Three-year Public Health Action Plan (Year 2023–2025) of Shanghai, China: GWVI-11.1–32; Construction of a 3D digital intelligent prevention and control platform for the whole life cycle of highly myopic patients in the Yangtze River Delta (Grant No. 21002411600); Project of Shanghai Xuhui District Science and Technology (Grant No. XHLHGG202104). The funding organization had no role in the design or conduct of this study.
Acknowledgments
We would like to thanks Yuchen Wu, Bo Wen, and Yongting Hong from BOE Yiyun Technology CO., Ltd., for providing the tablets and technical supports in this research, and the language-editing service provided by Editage.
Conflict of interest
The authors declare that the research was conducted in the absence of any commercial or financial relationships that could be construed as a potential conflict of interest.
Publisher’s note
All claims expressed in this article are solely those of the authors and do not necessarily represent those of their affiliated organizations, or those of the publisher, the editors and the reviewers. Any product that may be evaluated in this article, or claim that may be made by its manufacturer, is not guaranteed or endorsed by the publisher.
References
1. Alabdulkader, B . Effect of digital device use during COVID-19 on digital eye strain. Clin Exp Optom. (2021) 104:698–704. doi: 10.1080/08164622.2021.1878843
2. Kaur, K , Gurnani, B , Nayak, S , Deori, N , Kaur, S , Jethani, J, et al. Digital eye strain- a comprehensive review. Ophthalmol Ther. (2022) 11:1655–80. doi: 10.1007/s40123-022-00540-9
3. Feng, D , Lu, C , Cai, Q , and Lu, J . A study on the design of vision protection products based on children's visual fatigue under online learning scenarios. Healthcare (Basel). (2022) 10:621. doi: 10.3390/healthcare10040621
4. Wang, G , Zhang, Y , Zhao, J , Zhang, J , and Jiang, F . Mitigate the effects of home confinement on children during the COVID-19 outbreak. Lancet. (2020) 395:945–7. doi: 10.1016/S0140-6736(20)30547-X
5. Chang, P , Zhang, B , Lin, L , Chen, R , Chen, S , Zhao, Y, et al. Comparison of myopic progression before, during, and after COVID-19 lockdown. Ophthalmology. (2021) 128:1655–7. doi: 10.1016/j.ophtha.2021.03.029
6. Wang, J , Li, Y , Musch, DC , Wei, N , Qi, X , Ding, G, et al. Progression of myopia in school-aged children after COVID-19 home confinement. JAMA Ophthalmol. (2021) 139:293–300. doi: 10.1001/jamaophthalmol.2020.6239
7. Sanz Diez, P , Ohlendorf, A , Barraza-Bernal, MJ , Kratzer, T , and Wahl, S . Evaluating the impact of COVID-19 pandemic-related home confinement on the refractive error of school-aged children in Germany: a cross-sectional study based on data from 414 eye care professional centres. BMJ Open. (2023) 13:e071833. doi: 10.1136/bmjopen-2023-071833
8. Laan, D , Tan, ETC , Huis in het Veld, PI , Jellema, HM , and Jenniskens, K . Myopia progression in children during home confinement in the COVID-19 pandemic: a systematic review and meta-analysis. J Optom. (2024) 17:100493. doi: 10.1016/j.optom.2023.100493
9. Morgan, IG , Wu, PC , Ostrin, LA , Tideman, JWL , Yam, JC , Lan, W, et al. IMI risk factors for myopia. Invest Ophthalmol Vis Sci. (2021) 62:3. doi: 10.1167/iovs.62.5.3
10. Ku, PW , Steptoe, A , Lai, YJ , Hu, HY , Chu, D , Yen, YF, et al. The associations between near visual activity and incident myopia in children- a Nationwide 4-year follow-up study. Ophthalmology. (2019) 126:214–20. doi: 10.1016/j.ophtha.2018.05.010
11. Yang, GY , Huang, LH , Schmid, KL , Li, CG , Chen, JY , He, GH, et al. Associations between screen exposure in early life and myopia amongst Chinese preschoolers. Int J Environ Res Public Health. (2020) 17:1056–72. doi: 10.3390/ijerph17031056
12. Hinterlong, JE , Holton, VL , Chiang, CC , Tsai, CY , and Liou, YM . Association of multimedia teaching with myopia: a national study of school children. J Adv Nurs. (2019) 75:3643–53. doi: 10.1111/jan.14206
13. Németh, J , Tapasztó, B , Aclimandos, WA , Kestelyn, P , Jonas, JB , De Faber, JHN, et al. Update and guidance on management of myopia. European Society of Ophthalmology in cooperation with international myopia institute. Eur J Ophthalmol. (2021) 31:853–83. doi: 10.1177/1120672121998960
14. Nault, ML , Allard, P , Hinse, S , Le Blanc, R , Caron, O , Labelle, H, et al. Relations between standing stability and body posture parameters in adolescent idiopathic scoliosis. Spine (Phila Pa 1976). (2002) 27:1911–7. doi: 10.1097/00007632-200209010-00018
15. David, D , Giannini, C , Chiarelli, F , and Mohn, A . Text neck syndrome in children and adolescents. Int J Env Res Public Health. (2021) 18:1565. doi: 10.3390/ijerph18041565
16. Wang, Y , Toor, SS , Gautam, R , and Henson, DB . Blink frequency and duration during Perimetry and their relationship to test–retest threshold variability. Invest Ophthalmol Vis Sci. (2011) 52:4546–50. doi: 10.1167/iovs.10-6553
17. Murphy-Chutorian, E , and Trivedi, MM . Head pose estimation in computer vision: a survey. IEEE Trans Pattern Anal Mach Intell. (2009) 31:607–26. doi: 10.1109/TPAMI.2008.106
18. Council on Communications and Media . Media and young minds. Pediatrics. (2016) 138:e20162591. doi: 10.1542/peds.2016-2591
19. Wang, J , Han, Y , Musch, DC , Li, Y , Wei, N , Qi, X, et al. Evaluation and follow-up of myopia prevalence among school-aged children subsequent to the COVID-19 home confinement in Feicheng, China. JAMA Ophthalmol. (2023) 141:333–40. doi: 10.1001/jamaophthalmol.2022.6506
20. Saxena, R , Vashist, P , Tandon, R , Pandey, RM , Bhardawaj, A , Menon, V, et al. Prevalence of myopia and its risk factors in urban school children in Delhi: the North India myopia study (NIM study). PLoS One. (2015) 10:e117349. doi: 10.1371/journal.pone.0117349
21. Foreman, J , Salim, AT , Praveen, A , Fonseka, D , Ting, DSW , Guang He, M, et al. Association between digital smart device use and myopia: a systematic review and meta-analysis. Lancet Digit Health. (2021) 3:e806–18. doi: 10.1016/S2589-7500(21)00135-7
22. Mireku, MO , Barker, MM , Mutz, J , Dumontheil, I , Thomas, MSC , Röösli, M, et al. Night-time screen-based media device use and adolescents' sleep and health-related quality of life. Environ Int. (2019) 124:66–78. doi: 10.1016/j.envint.2018.11.069
23. Robinson, TN , Banda, JA , Hale, L , Lu, AS , Fleming-Milici, F , Calvert, SL, et al. Screen media exposure and obesity in children and adolescents. Pediatrics. (2017) 140:S97–S101. doi: 10.1542/peds.2016-1758K
24. Enthoven, CA , Polling, JR , Verzijden, T , Tideman, JWL , Al-Jaffar, N , Jansen, PW, et al. Smartphone use associated with refractive error in teenagers: the myopia app study. Ophthalmology. (2021) 128:1681–8. doi: 10.1016/j.ophtha.2021.06.016
25. Reid Chassiakos, YL , Radesky, J , Christakis, D , Moreno, MA , and Cross, CCOUNCIL ON COMMUNICATIONS AND MEDIA. Children and adolescents and digital media. Pediatrics. (2016) 138:e20162593. doi: 10.1542/peds.2016-2593
26. Pucker, AD , and Gawne, TJ . Fighting myopia with intermittent Nearwork breaks: 20 seconds every 20 minutes might not be enough time. Optom Vis Sci. (2023) 100:31–2. doi: 10.1097/OPX.0000000000001965
27. Ma, M , Xiong, S , Zhao, S , Zheng, Z , Sun, T , and Li, C . COVID-19 home quarantine accelerated the progression of myopia in children aged 7 to 12 years in China. Invest Ophthalmol Vis Sci. (2021) 62:37. doi: 10.1167/iovs.62.10.37
28. Kohmarn, T , Srisurattanamethakul, N , Watcharapalakorn, A , Poyomtip, T , and Poolsanam, C . Outbreak of COVID-19-related myopia progression in adults: a preliminary study. Clin Optom. (2022) 14:125–31. doi: 10.2147/OPTO.S374155
29. Bhandari, KR , Shukla, D , Mirhajianmoghadam, H , and Ostrin, LA . Objective measures of near viewing and light exposure in school children during COVID-19. Optom Vis Sci. (2022) 99:241–52. doi: 10.1097/OPX.0000000000001871
30. Higuchi, S , Lee, SI , Kozaki, T , Harada, T , and Tanaka, I . Late circadian phase in adults and children is correlated with use of high color temperature light at home at night. Chronobiol Int. (2016) 33:448–52. doi: 10.3109/07420528.2016.1152978
31. Leibowitz, HW , and Owens, DA . Night myopia and the intermediate dark focus of accommodation. J Opt Soc Am. (1975) 65:1121–8. doi: 10.1364/josa.65.001121
32. Ebenholtz, SM , and Zander, PA . Accommodative hysteresis: influence on closed loop measures of far point and near point. Invest Ophthalmol Vis Sci. (1987) 28:1246–9.
33. Muralidharan, AR , Lança, C , Biswas, S , Barathi, VA , Wan Yu Shermaine, L , Seang-Mei, S, et al. Light and myopia: from epidemiological studies to neurobiological mechanisms. Ther. Adv Ophthalmol. (2021) 13:25158414211059246. doi: 10.1177/25158414211059246
34. Osterhaus, W , Hemphälä, H , and Nylén, P . Lighting at computer workstations. Work. (2015) 52:315–28. doi: 10.3233/WOR-152163
35. Heo, JY , Kim, K , Fava, M , Mischoulon, D , Papakostas, GI , Kim, MJ, et al. Effects of smartphone use with and without blue light at night in healthy adults: a randomized, double-blind, cross-over, placebo- controlled comparison. J Psychiatr Res. (2017) 87:61–70. doi: 10.1016/j.jpsychires.2016.12.010
Keywords: artificial intelligence, display terminal, light environment, myopia, screen time, viewing distance
Citation: Wang J, Shen Y, Zhao J, Wang X, Chen Z, Han T, Huang Y, Wang Y, Zhao W, Wen W, Zhou X and Xu Y (2024) Algorithmic and sensor-based research on Chinese children’s and adolescents’ screen use behavior and light environment. Front. Public Health. 12:1352759. doi: 10.3389/fpubh.2024.1352759
Edited by:
Peter Kokol, University of Maribor, SloveniaReviewed by:
Xuejiao Qin, The Second Hospital of Shandong University, ChinaBojan Žlahtič, University of Maribor, Slovenia
Copyright © 2024 Wang, Shen, Zhao, Wang, Chen, Han, Huang, Wang, Zhao, Wen, Zhou and Xu. This is an open-access article distributed under the terms of the Creative Commons Attribution License (CC BY). The use, distribution or reproduction in other forums is permitted, provided the original author(s) and the copyright owner(s) are credited and that the original publication in this journal is cited, in accordance with accepted academic practice. No use, distribution or reproduction is permitted which does not comply with these terms.
*Correspondence: Xingtao Zhou, doctzhouxingtao@163.com; Ye Xu, janetyeye@hotmail.com
†These authors have contributed equally to this work and share first authorship