- 1Department of Rehabilitation Medicine, China Emergency General Hospital, Beijing, China
- 2Unit of Psychiatry, Department of Public Health and Medicinal Administration, Institute of Translational Medicine, Faculty of Health Sciences, University of Macau, Macau, Macao SAR, China
- 3Centre for Cognitive and Brain Sciences, University of Macau, Macau, Macao SAR, China
- 4Unit of Medical Psychology and Behavior Medicine, School of Public Health, Guangxi Medical University, Nanning, China
- 5Beijing Key Laboratory of Mental Disorders, National Clinical Research Center for Mental Disorders and National Center for Mental Disorders, Beijing Anding Hospital, Capital Medical University, Beijing, China
- 6School of Public Health, Southeast University, Nanjing, China
- 7School of Nursing, Hong Kong Polytechnic University, Kowloon, Hong Kong SAR, China
- 8Department of Psychiatry, TheMelbourne Clinic and St Vincent’s Hospital, University of Melbourne, Richmond, Victoria, VIC, Australia
Background: Research on the mental health and quality of life (hereafter QOL) among fire service recruits after the end of the COVID-19 restrictions is lacking. This study explored the network structure of depression, anxiety and insomnia, and their interconnections with QOL among fire service recruits in the post-COVID-19 era.
Methods: This cross-sectional study used a consecutive sampling of fire service recruits across China. We measured the severity of depression, anxiety and insomnia symptoms, and overall QOL using the nine-item Patient Health Questionnaire (PHQ-9), seven-item Generalized Anxiety Disorder scale (GAD-7), Insomnia Severity Index (ISI) questionnaire, and World Health Organization Quality of Life-brief version (WHOQOL-BREF), respectively. We estimated the most central symptoms using the centrality index of expected influence (EI), and the symptoms connecting depression, anxiety and insomnia symptoms using bridge EI.
Results: In total, 1,560 fire service recruits participated in the study. The prevalence of depression (PHQ-9 ≥ 5) was 15.2% (95% CI: 13.5–17.1%), while the prevalence of anxiety (GAD-7 ≥ 5) was 11.2% (95% CI: 9.6–12.8%). GAD4 (“Trouble relaxing”) had the highest EI in the whole network model, followed by ISI5 (“Interference with daytime functioning”) and GAD6 (“Irritability”). In contrast, PHQ4 (“Fatigue”) had the highest bridge EI values in the network, followed by GAD4 (“Trouble relaxing”) and ISI5 (“Interference with daytime functioning”). Additionally, ISI4 “Sleep dissatisfaction” (average edge weight = −1.335), which was the central symptom with the highest intensity value, had the strongest negative correlation with QOL.
Conclusion: Depression and anxiety were important mental health issues to address among fire service recruits in the post-COVID-19 era in China. Targeting central and bridge symptoms identified in network analysis could help address depression and anxiety among fire service recruits in the post-COVID-19 era.
1 Introduction
Fire service recruits are a unique population affected by mental health issues. According to the regulations and policies in China, it is mandatory for fire service recruits to receive strict training for several months, pass stringent physical and professional assessment, and meet the full accreditation requirements before they can qualified and work as firefighters (1). As such, facing the pressure of meeting the high standards of assessments could increase the risk of mental health problems such as depression, anxiety and sleep problems among the fire service recruits.
The Coronavirus Disease 2019 (COVID-19) has resulted in a massive burden on the medical systems, as well as unprecedented health challenges for China and the global community (2). During the COVID-19 pandemic, the imposition of strict confinement measures and social isolation have also led to heightened psychological stress, significantly elevating the risk of mental health problems, such as depression, anxiety and insomnia (3, 4), in many subpopulations including fire service recruits. Apart from enduring extensive physical training and strict isolation measures during the COVID-19 epidemic, fire service recruits also experience prolonged detachment from the outside world, and separation from their relatives and friends. Consequently, the lack of social and familial support further increases the risk of mental health problems like anxiety, depression, and insomnia. Previous studies found that individuals who experienced strict isolation during the COVID-19 pandemic had a higher likelihood of adverse psychological outcomes, including depression, anxiety and insomnia, compared to those who had not experienced such isolation (5, 6). Moreover, studies conducted across various populations also found detrimental impact of the COVID-19 pandemic and associated lockdown measures on both physical and mental well-being of isolated individuals, which affected their quality of life (QOL) (7–9). However, there is a dearth of research data concerning the mental health of fire service recruits and its relationship to QOL in the post-COVID-19 era, thereby prompting further investigation into this population.
In recent years, network analysis has been increasingly employed in the field of mental health to better understand the interconnectedness between different psychiatric symptoms from the perspective of network (10, 11). The network graph visualizes the structure of the correlation matrix, which reflects the magnitude of correlation between symptoms, composing of core components referred to as “nodes” and “edges” (12, 13). Furthermore, by identifying highly connected central nodes, network analysis can provide insights into the risks, prevention, and interventions associated with specific syndromes (14). Further, network analysis can help identify psychiatric comorbidities that are important for prevention and treatment, as well as symptoms that serve as “bridge symptoms” for inter-disease transmission risk (15).
Network analysis has been widely applied across various psychiatric conditions such as anxiety, depression, insomnia, and QOL during the COVID-19 pandemic (16–20). For instance, a network analysis study on anxiety-depression-insomnia in Macao residents showed that “Sleep maintenance,” “Trouble relaxing” and “Interference with daytime functioning” were the most central (influential) symptoms during the COVID-19 pandemic (21). In the late stage of the COVID-19 pandemic, a network analysis study of anxiety-depression in Chinese nurses showed that “restlessness,” and “trouble relaxing” were the most central symptoms (22). The variation in the results between studies may be due to the differences in demographic features, methodology (e.g., sampling method and study design), workplace demands and environments, and the pandemic stage (23, 24).
There has been little research on fire service recruits, although some studies have examined the mental health status of firefighters in China. For instance, a study on mental health status of Chinese firefighters reported that cognitive reappraisal could negatively predict anxiety and depression symptoms (25). Another study found that mental health problems among firefighters in China were not uncommon and deserve more attention (26). Although fire service recruits and fire service recruits face stricter quarantine measures than the general population during and after the COVID-19 pandemic, and they also endure physically demanding training that can result in substantial physical fatigue and psychological stress (27, 28), there is insufficient research using network analysis to investigate psychiatric symptoms among fire service recruits in the post-COVID-19 era.
To address this gap, we employed network analysis to examine the interrelationships between depression, anxiety, and insomnia symptoms among Chinese fire service recruits in the post-COVID-19 era, with a focus on identifying central symptoms and bridge symptoms. Additionally, the study aimed to identify symptoms associated with depression, anxiety, and insomnia that impact on the QOL of Chinese fire service recruits, thus providing a theoretical foundation for targeted preventive interventions to address such symptoms and enhance their QOL.
2 Methods
2.1 Participants and study procedure
A consecutive sampling method was used in this multicentre, cross-sectional study that was conducted between February 13 and February 16, 2023. Due to the COVID-19 pandemic, face-to-face assessment could not be adopted; instead, an online survey was conducted. Following previous studies (29, 30), the online assessment was conducted on WeChat based on the “Questionnaire Star.” As all fire service recruits in the training centers had to report their health status regularly using WeChat during the study period, all participants were WeChat users.
All fire service recruits who received training across the four fire training centers located in Beijing, Sichuan, Guangxi and Guizhou during the study period were consecutively invited to participate on a voluntary and anonymous basis. A quick response code (QR code) was linked to the study invitation and questionnaire. Inclusion criteria were as follows: (1) fire service recruits aged 18 years or older; (2) were able to read Chinese and understand the purpose and content of the assessment. Based on relevant regulations in China, fire service recruits who were receiving training were not qualified to participate in firefighting mission tasks. Those with significant physical diseases and/psychiatric disorders (including COVID-19 complications and sequelae) were unable to receive regular training, therefore, could not be involved in this study. The study was approved by the ethics committee of the Emergency General Hospital and all participants signed the electronic written informed consent.
2.2 Measures
Following previous research (31, 32), the severity of depressive symptoms was measured using the validated Chinese version of the nine-item Patient Health Questionnaire (PHQ-9), which has good reliability, validity and has been widely used as a measure of depressive symptoms in various populations (33–35). The PHQ-9 consists of 9 items, with each scoring from 0 to 3 (from not at all to nearly every day) and the total score ranging from 0 to 27. A higher total score indicates more severe depressive symptoms, with a total score of 5, 10, 15, and 20, represent mild, moderate, moderate-to-severe and severe depressive symptoms, respectively (36).
Following previous research (37, 38), the severity of anxiety symptoms was measured using the validated Chinese version of the seven-item Generalized Anxiety Disorder scale (GAD-7) scale, which has satisfactory psychometric properties and has been widely used in various populations (39, 40). The GAD comprises seven items, each scoring from 0 (“not at all”) to 3 (“nearly every day”) (39, 41). The GAD-7 total score ranges from 0 to 21, with a higher total score indicating the more severe anxiety symptoms. The total scores of 5, 10, and 15 represent mild, moderate, and severe anxiety symptoms, respectively (42).
The severity of insomnia symptoms was measured using part of the Insomnia Severity Index (ISI) questionnaire (i.e., the items 1, 2, 3, 4, 5, and 6) (43–45), with each item ranging from 0 (none) to 4 (very severe). According to previous studies (46, 47), the PHQ-9, GAD-7 and ISI scores in this study were positively skewed. Therefore, we binarized each item: for all items, with a value of 0 was considered “absence” and recorded as 0; for PHQ-9 and GAD-7 items, each item with a value of 1 or 2 or 3 was considered “presence” and recorded as 1; and for ISI items, each item with a value of 1 or 2 or 3 or 4 was considered “presence” and recorded as 1 (22, 46, 47). Following previous research (48), overall QOL was measured using the first item of the World Health Organization Quality of Life-brief version (WHOQOL-BREF): “How would you rate your quality of life?,” with each item scored from 1 (extremely dissatisfied) to 5 (extremely satisfied) (49). In this network analysis, responses 1, 2 and 3 were recoded as 0, while responses 4 and 5 were recoded as 1 (50). Cronbach’s alpha values for the PHQ-9, GAD-7, ISI and the two-item WHOQOL were 0.858, 0.945, 0.896 and 0.846, respectively in the present study sample.
2.3 Data analysis
2.3.1 Network estimation
The network analysis of depression, anxiety and insomnia model was performed using R software (51). This study applied the Ising model, which has been widely used in anxiety and/or depressive symptom networks conducted in different populations, to estimate the anxiety-depression-insomnia network (22, 47, 52). In this study, the score distributions of PHQ-9, GAD-7 and ISI items were all skewed. Therefore, following previous research (53–55), the Ising model was used to estimate this network. In the Ising model, all PHQ-9, GAD-7, and ISI items were divided into two categories, with “0” and “1” representing the absence and presence of depression, anxiety, or insomnia symptoms, respectively. Scores of “0” for all items were considered to indicate the absence of depression, anxiety, or insomnia symptoms, while scores of “1,” “2,” and “3” were considered to reflect the presence of depression, anxiety, or insomnia symptoms (56). The Ising model has also been widely used in the construction of network models for psychological binary data (53). In the Ising network, each item of the PHQ-9, GAD-7 and ISI is viewed as a node, while the edge connecting two nodes represents the association between two symptoms. The R packages “qgraph” (version 1.9.4) (12) and “bootnet” (version 1.5) (57) were used to visualize the network analysis of depression, anxiety, and insomnia, where a green edge represents a positive correlation, a red edge represents a negative correlation, and a thicker edge represents a stronger correlation between nodes. The goldbricker function in R package networktools (58) was used to examine potential item redundancy (i.e., less than 25% of the significant different correlations). Following previous research (59–61), the item PHQ3 (“Sleep”) in the PHQ-9 was considered redundant given that another specific measure on insomnia was used, hence, PHQ3 was excluded from the network analysis.
2.3.2 Centrality and stability
We estimated the centrality index of expected influence (EI) of the nodes in the network through R package “qgraph” (version 1.9.4) (12), where EI refers to the sum of the weights of the edges shared by the node symptoms and other nodes. The EI centrality was calculated by summarizing the sum of positive and negative edges connected to a specific node, which can identify the most influential nodes (i.e., symptoms) within the network model (62). A higher EI indicates a more influential the symptom in the network (62). The R package “networktools” (version 1.5.0) (58) was used to calculate the Bridge Expected Influence (BEI) index to identify bridge symptoms connecting different communities of depression, anxiety and insomnia, namely central symptoms connecting other different symptom communities (15). A higher BEI value indicates a stronger bridging effect of nodes between different communities.
In addition, the predictability index of each node was estimated using the “mgm” (version 1.2.13) (63) network model in the R package. The predictability value quantifies the extent to which a given node is likely to be explained by other neighboring nodes in the model.
The bootstrap method in R package “bootnet” (version 1.5) (57) was used to evaluate the accuracy and stability of the network model. In addition, the correlation stability coefficient (CS-coefficient) using the case-dropping bootstrap approach was used to test the centrality and stability of EI and BEI (57); values greater than 0.25 represent good stability.
2.3.3 Network comparison
Previous studies (64–66) found that education levels were associated with severity of depression and anxiety; therefore, their potential influence on the network model was examined using R package “NetworkComparisonTest” (version 2.2.1) (67). Furthermore, the overall network structure, global strength, and differences at each edge between subsamples were compared between different education levels.
3 Results
Of the 1,564 fire service recruits who were invited to participate in this study, 1,560 met the study entry criteria and completed all the assessments. These included 479 (30.7%) recruits from Beijing, 489 (31.3%) from Sichuan, 275 (17.6%) from Guangxi and 317 (20.3%) from Guizhou, giving a participation rate of 99.7%. Of the four fire service recruits who declined to participate in this study due to lack of interest, two came from Guangxi, and one came from Beijing and Sichuan each. All participants were males. The mean age of the participants was 22.6 [standard deviation (SD): 1.8] years; namely 22.3 (SD: 1.9) years in Beijing, 22.5 (SD: 1.8) years in Sichuan, 22.7 (SD: 1.7) years in Guangxi, and 23.0 (SD: 1.8) years in Guizhou; (p < 0.001). A total of 874 (56.03%) had college educational and above level (44.1% in Beijing; 55.8% in Sichuan; 69.1% in Guangxi; 63.1% in Guizhou; p < 0.001). The prevalence of depression (PHQ-9 ≥ 5) was 15.2% (95% CI: 13.5–17.1%) (11.1% in Beijing; 20.0% in Sichuan; 13.5% in Guangxi; 15.5% in Guizhou; p = 0.001), while the prevalence of anxiety (GAD-7 ≥ 5) was 11.2% (95% CI: 9.6–12.8%) (6.7% in Beijing; 12.9% in Sichuan; 9.1% in Guangxi; 17.0% in Guizhou; p < 0.001). The overall QOL score was 3.8 (SD: 0.8) in the whole sample, while the corresponding figure was 4.0 (SD: 0.8) in Beijing; 3.7 (SD: 0.8) in Sichuan; 3.8 (SD: 0.8) in Guangxi; 3.9 (SD: 0.7) in Guizhou (p < 0.001).
The correlation matrix of PHQ-9, GAD-7 and ISI items are shown in Supplementary Table S1. GAD1 (“Nervousness”) – GAD2 (“Uncontrollable worry”) was the strongest positive edge, followed by GAD6 (“Irritability”) – GAD7 (“Feeling afraid”) and ISI5 (“Interference with daytime functioning”) – ISI6 (“Noticeability of sleep problems by others”) (Figure 1).
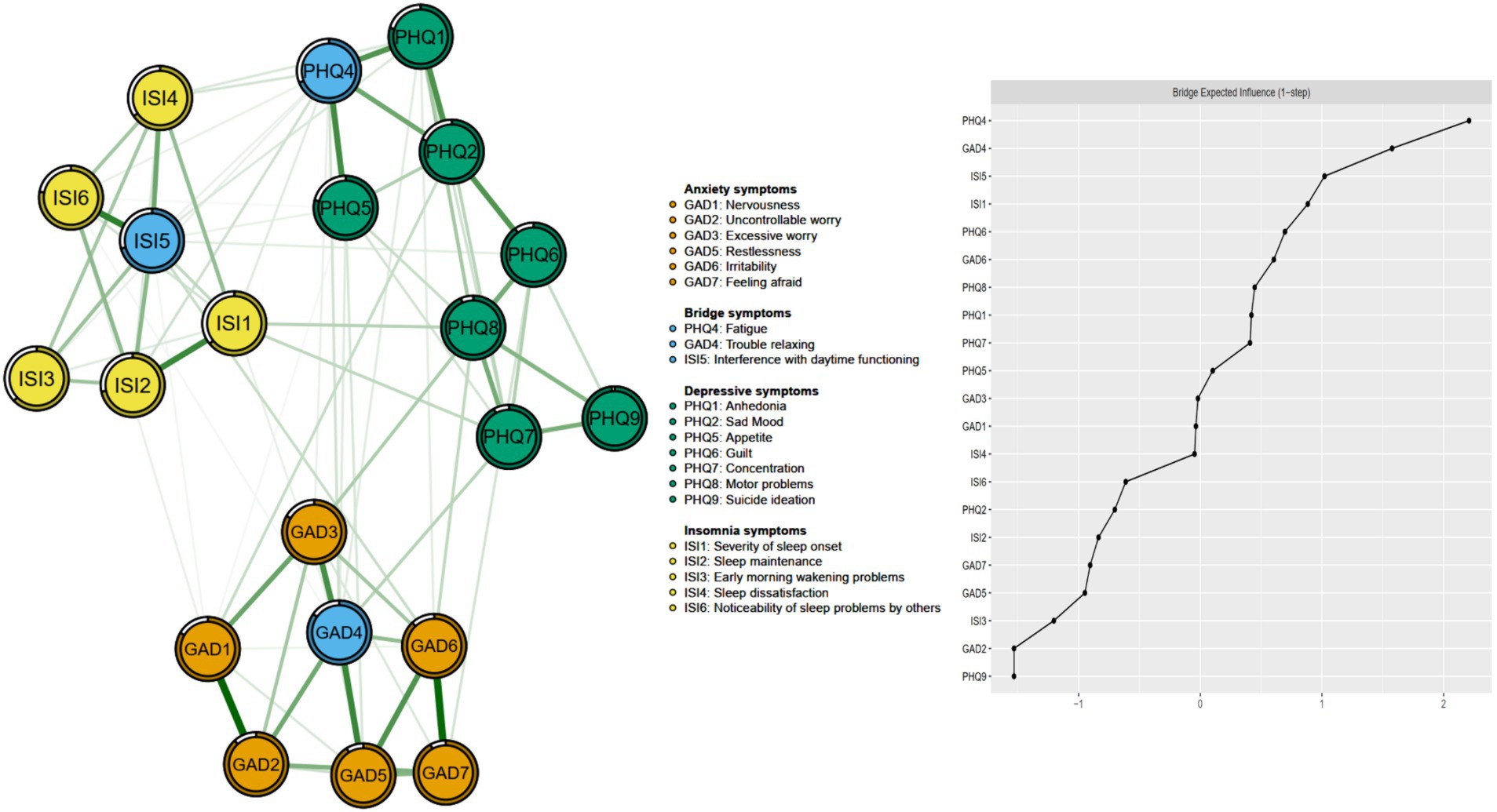
Figure 1. Network structure of insomnia, anxiety, and depressive symptoms showing bridge symptoms in fire service recruits during the post-COVID-19 era.
3.1 Network structure
The item redundancy analysis did not reveal any redundant ISI or PHQ-9 items. Therefore, all items of depression, anxiety and insomnia except for PHQ3 (“Sleep”) were included for analysis.
The left panel of Figure 1 shows the network structure of depression, anxiety, and insomnia symptoms. The average predictability of nodes was 0.813, ranging from 0.627 to 0.984, indicating that on average 81.3% variance of each node could be explained by its neighboring nodes in the model (Figure 1 and Table 1). Table 1 and Figure 2 shows that GAD4 “Trouble relaxing” (EI: 8.512) had the highest EI in the whole network structure, followed by ISI5 “Interference with daytime functioning” (EI: 8.135) and GAD6 “Irritability” (EI: 7.981), indicating that these were the most influential symptoms in the overall network model. In contrast, PHQ4 “Fatigue” (EI: 2.577) had the highest bridge EI values, followed by GAD4 “Trouble relaxing” (EI: 2.140) and ISI5 “Interference with daytime functioning” (EI: 1.758) in this network (Figure 1).
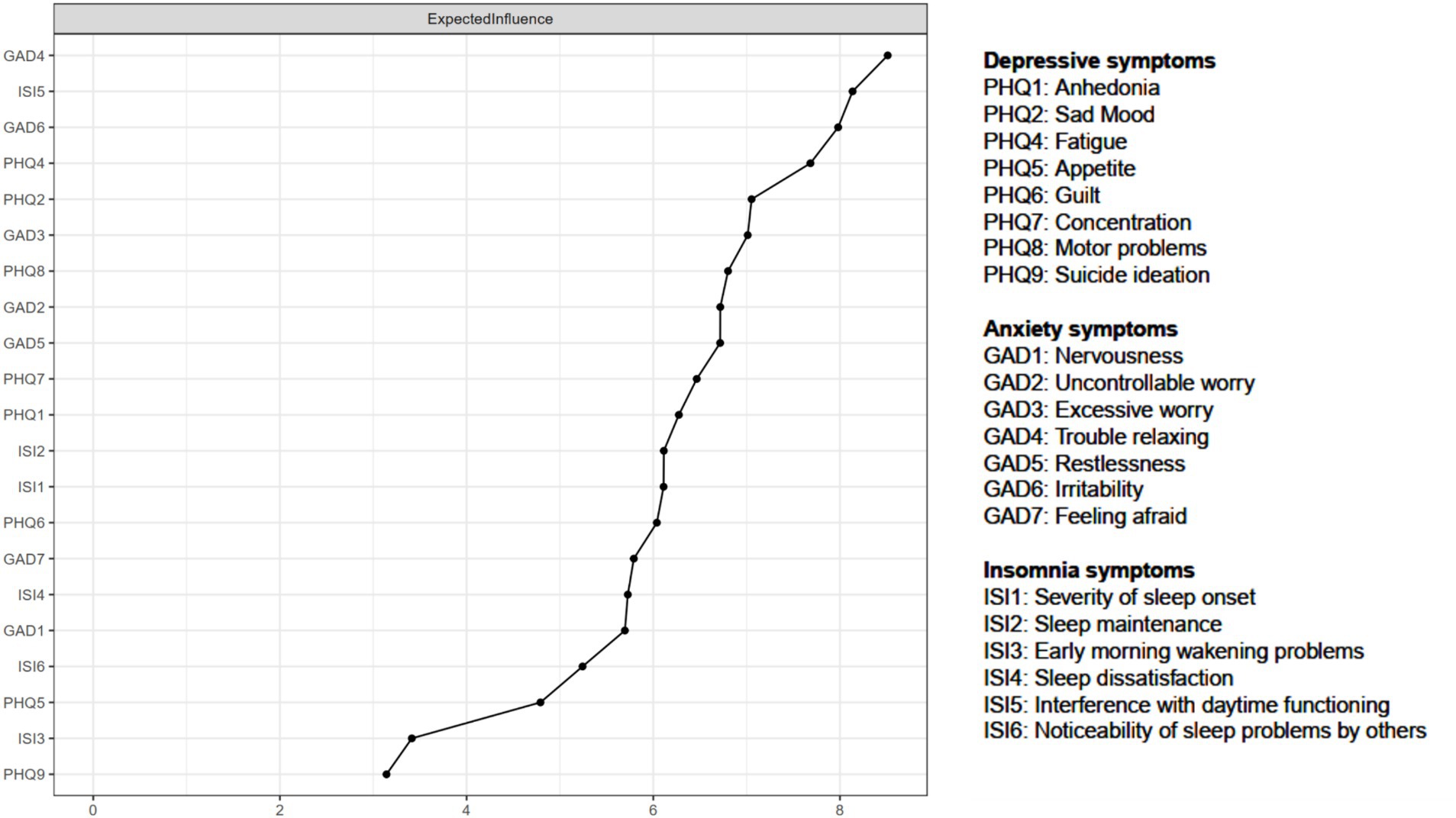
Figure 2. Expected influence of the network structure of depressive, anxiety and insomnia symptoms among fire service recruits during the post-COVID-19 era.
3.2 Network stability
In terms of network stability, as shown in Figure 3, the CS-coefficient of strength was 0.533, indicating excellent stability as the network structure would not significantly change even if 53.3% of the samples were removed. In addition, the CS-coefficient of the bridge strength obtained by the case dropping bootstrap method was 0.244 (Figure 3). The edge-weight bootstrap difference test showed that most comparisons between edge weights were statistically significant (Supplementary Figure S1). Moreover, the bootstrapped stability test for EI showed that these central symptom nodes were statistically stronger than other nodes, indicating that the primary results were reliable (Supplementary Figure S2). Therefore, the network model demonstrated acceptable reliability and stability.
3.3 Flow network of quality of life
Figure 4 shows the flow network model of PHQ-9, GAD-7 and ISI symptoms and QOL. ISI4 “Sleep dissatisfaction” (average edge weight = −1.335), as the central symptom with the highest intensity value, had the strongest negative correlation with QOL, followed by PHQ1 “Anhedonia” (average edge weight = −0.741) and ISI6 “Noticeability of sleep problems by others” (average edge weight = −0.589).
3.4 Network comparison test by educational level (high school and lower vs. above high school)
Network models by educational level are shown in Supplementary Figure S3. In the comparison of different models, no significant difference was found in the network global strengths (high school and lower: 58.357; above high school: 57.694; S = 0.662, p = 0.97; Supplementary Figure S3). There was also no significant difference in the network structure-distribution of edge weights (M = 1.640, p = 0.59).
4 Discussion
This study was the first network analysis to investigate the interrelationships between depression, anxiety and insomnia symptoms among Chinese fire service recruits in the post-COVID-19 era. The prevalence of depression and anxiety in this sample was 15.2% (95% CI: 13.5–17.1%) and 11.2% (95% CI: 9.6–12.8%), respectively, which are lower than the corresponding prevalence of depression (45.3%) and anxiety (48.1%) among medical trainees in New York (68), and that of depression (22.8%; 95% CI: 20.22–25.32) and anxiety (20.0%; 95% CI: 17.55–22.41) among the general population in Ireland during the pandemic (69). However, our findings are higher than the prevalence of depression (8.4%; PHQ-9 ≥ 10) and anxiety (4.8%; GAD-7 ≥ 10) among the general population aged 35–69 in Russian in the pre-COVID-19 period (70). The possible reasons for the discrepancy included differences in the study samples, sampling methods, assessment tools on depression and anxiety, and stages of the pandemic. For instance, a study found that compared with the figures at the peak of the COVID-19 pandemic, the prevalence of depression and anxiety among participants increased after the COVID-19 peak (71). Further, the long-term effects of COVID-19 might also perpetuate or exacerbate mental health problems (72). Although daily physical training could reduce the risk and severity of depression and anxiety (73, 74), other factors could offset the benefits. These include long-term heavy physical training, strict isolation measures, fear of infectious transmission, personal uncertainty about future career development, and lack of social and family support, all of which could increase risk of depression, anxiety and sleep disturbances among fire service recruits during and immediately after the COVID-19 pandemic, (75, 76).
We found that differences in prevalence of depression and anxiety across the four provinces in China, with Beijing having the lowest prevalence and Sichuan having the highest prevalence. Possible reasons included the differences in the stages of the COVID-19 pandemic wave, prevention and control policies, and economic conditions between provinces (77, 78). Compared with most of other areas in China, Beijing, as the capital of China, had stricter public health policies, better economic conditions, and better social support (79, 80), all of which were associated with lower risk of depression and anxiety than that of other provinces (77).
In this network analysis, GAD4 “Trouble relaxing” was a central and bridge symptom, and therefore one of the most influential symptom in maintaining the whole network model, which is consistent with previous findings reported in Macau residents, Filipino domestic helpers in Macau, and Chinese female nursing students (21, 81, 82). Interventions that target this symptom might be effective in reducing the severity of related symptoms in the anxiety-depression-sleep network (14). A study of psychopathological symptom network in the COVID-19 outbreak and post-peak periods found that “inability to relax” symptoms played an important role with high centrality during the COVID-19 outbreak, but significantly reduced after the COVID-19 peak (71). For the Chinese fire service recruits in the post-COVID-19 era, the long-term closed training coupled with the strict lockdown measures likely resulted in marked exhaustion and a lack of ability to rest or relax (83). In addition, such quarantine reduced communication with the outside world as well as entertainment activities that served as coping mechanisms for long-term state of tension (84), thus increasing the risk for anxiety symptoms and other mental health problems among fire service recruits.
GAD6 “Irritability,” which refers to a low threshold for anger due to frustration (85, 86), is a diagnostic criterion for generalized anxiety disorder (87). “Irritability” was a central symptom in this study, which is consistent with previous findings among adolescents (17). According to the relevant regulations and policies of China’s National comprehensive fire protection, fire service recruits need to undergo arduous physical training and professional assessments in order to meet the required standards to qualify as firefighters (1). The fear of failing such strict assessments and meeting the high standards could result in irritability and anxiety among the fire service recruits.
Another central and bridge symptom identified in this study was ISI5 (“Interference with daytime functioning”), which might exacerbate symptoms of depression, anxiety, and insomnia (19, 21). This is consistent with another network analysis study conducted among clinicians in China, in which “Interference with daytime functioning” was the most influential symptom in the network model of anxiety, insomnia, and depressive symptoms (59). Previous studies found that physical discomfort and fatigue are significantly associated with daytime functioning caused by sleep disturbances (88, 89). The rigorous and continuous high-intensity physical training could lead to extreme physical fatigue, susceptibility to sports injuries, and increased risk of insomnia, all of which could interfere with their normal daytime function. Therefore, exploring ways to address inability to relax, irritability, and daytime functioning might reduce the risk of their mental health problems in fire service recruits during the post-pandemic era.
Fatigue is a common health issue across many populations; for instance, the prevalence of fatigue was found to affect up to 83.5% of nursing students (90). In this study, PHQ4 (“Fatigue”) was both the central and bridge symptom in the depression-anxiety-insomnia network, which supports previous network analysis findings among clinicians working in public hospitals (59). In a previous network analysis study of depressive and anxiety symptoms among female nursing students, “fatigue” was also the most central symptom (82). Similar results were also reported in another network study involving residents in Guangdong province of China (91). Fatigue and insomnia symptoms often co-occur in patients with depression, and those with fatigue usually have a higher risk of depression (92). Moreover, excessive activities and insomnia could increase the risk of fatigue (90). After ceasing the dynamic zero-COVID policy in China, fire service recruits remained prone to experience physical fatigue and depression (93), which might be partly due to ongoing rigorous physical training, strict isolation and a lack social support and recreational activities. Therefore, the occurrence and development of mental health problems, including physical fatigue and negative emotions persisted (94).
In network theory, bridge symptoms are considered transdiagnostic, which means that interventions targeting bridge symptoms, such as “fatigue,” could be effective for all the three communities in this study, i.e., depression, anxiety and insomnia (59, 95). Previous research found that individuals who reported fatigue had a higher risk of depression, and interventions targeting fatigue could relieve depressive and anxiety symptoms (91). Therefore, improving fatigue might influence the severity and dynamics of the communities of depression, anxiety and insomnia network. For example, cognitive behavioral therapy, practical rehabilitation treatment, supportive listening counseling interventions and self-guided online interventions appeared to be effective and accessible measures for fatigue in primary care (96, 97). Moreover, traditional Chinese medicine (TCM) treatment such as acupuncture and moxibustion could also improve fatigue symptoms (98, 99), through regulating immune dysfunction and abnormal activities of the hypothalamic–pituitary–adrenal (HPA) axis (100). These interventions for fatigue might be effective in reducing the severity and interaction of depression, anxiety and insomnia symptoms in patients (101).
The QOL is a multidimensional and significant health outcome indicator of an individual’s overall well-being, encompassing both physical and mental health (102). Prior studies demonstrated the negative relationship between QOL and various mental health problems such as insomnia, depressive (specifically anhedonia) and anxiety symptoms and suicidal ideation across different populations (82, 103–107). As expected, ISI4 “Sleep dissatisfaction” had the strongest negative correlation with QOL among fire service recruits in this study, which is consistent with previous findings among Macau residents (21). Hence, reducing the occurrence of insomnia symptoms, such as “Sleep dissatisfaction,” might play an important role in improving QOL. Insomnia could lead to a variety of physical and mental health problems, including cardiovascular disease, depression, anxiety, and suicide (108–110), which could affect daytime function, normal work and life activities, and QOL (111). During the COVID-19 pandemic, the rigorous physical training in an isolated environment (112), could have affected sleep satisfaction and lowered QOL among new fire service recruits in China.
PHQ1 (“Anhedonia”) was another symptom that was negatively associated with QOL, indicating the influential role of anhedonia on QOL among fire service recruits. For fire service recruits, the fear of COVID-19 infection, isolation from their families/friends due to the nature of their work (113), and the uncertainty of the future (114), might have aggravated the concerns about their future career development and life (115, 116). These factors could increase the risk of depressive symptoms including anhedonia, and thereby reducing their QOL (117–119).
The strengths of this study consisted of the inclusion of an under-studied sample, a large sample size and the use of novel and sophisticated statistical analyses. However, several limitations should be acknowledged. First, due to the cross-sectional nature of the study, it was not possible to assess dynamic changes of individual symptoms or establish causal relationships between the symptoms. Second, due to the restrictive measures during the COVID-19 pandemic, this study relied solely on online self-report to assess various symptoms, which might have resulted in recall and reporting bias. Third, for logistical reasons, diagnostic instruments on psychiatric disorders were not used. Therefore, the diagnoses of anxiety and depressive disorders among fire service recruits could not be examined.
In conclusion, depression and anxiety were key mental health problems to address among fire service recruits in the post-COVID-19 era. ISI4 “Sleep dissatisfaction” was the most central symptom, with the strongest negative correlation with QOL, followed by PHQ1 “Anhedonia” and ISI6 “Noticeability of sleep problems by others.” In addition, the central and bridge symptoms (e.g., GAD4, ISI5, GAD6, and PHQ4) in this network analysis warrant attention when addressing depression and anxiety and developing future targeted interventions in this population.
Data availability statement
The datasets presented in this article are not readily available because the the Ethics Committee of the Emergency General Hospital that approved the study prohibits the authors from making publicly available the research dataset of clinical studies. Requests to access the datasets should be directed to eHl1dGx5QGdtYWlsLmNvbQ==.
Ethics statement
The study was approved by the ethics committee of the Emergency General Hospital and all participants signed the electronic written informed consent.
Author contributions
JL: Data curation, Investigation, Writing – review & editing. ZG: Conceptualization, Data curation, Formal analysis, Investigation, Methodology, Project administration, Software, Writing – original draft, Writing – review & editing. PC: Data curation, Formal analysis, Writing – review & editing, Methodology. HC: Writing – review & editing, Data curation, Formal analysis. YF: Data curation, Writing – review & editing. T-IH: Data curation, Writing – review & editing. S-YR: Data curation, Writing – review & editing. ZS: Data curation, Writing – review & editing. TC: Data curation, Writing – review & editing. CN: Writing – review & editing. GW: Data curation, Writing – review & editing, Funding acquisition. Y-TX: Data curation, Writing – review & editing, Formal analysis, Investigation, Methodology, Project administration, Resources, Software, Writing – original draft.
Funding
The author(s) declare that financial support was received for the research, authorship, and/or publication of this article. This study was supported by the Medical Research and Development Fund of Emergency General Hospital (Number: KY202214), the National Science and Technology Major Project for investigational new drug (2018ZX09201-014), the Beijing Hospitals Authority Clinical Medicine Development of special funding support (XMLX202128), and the University of Macau (MYRG2019-00066-FHS and MYRG2022-00187-FHS).
Acknowledgments
The authors are grateful to all participants and clinicians involved in this study.
Conflict of interest
The authors declare that the research was conducted in the absence of any commercial or financial relationships that could be construed as a potential conflict of interest.
Publisher’s note
All claims expressed in this article are solely those of the authors and do not necessarily represent those of their affiliated organizations, or those of the publisher, the editors and the reviewers. Any product that may be evaluated in this article, or claim that may be made by its manufacturer, is not guaranteed or endorsed by the publisher.
Supplementary material
The Supplementary material for this article can be found online at: https://www.frontiersin.org/articles/10.3389/fpubh.2024.1348870/full#supplementary-material
References
1. Ma, HR. Study on the mechanism of combat-oriented training for newly recruited firefighters. China Acad J Electron Publ House. (2020) 24:52–3. doi: 10.16859/j.cnki.cn12-9204/tu.2020.24.031
2. Miller, IF, Becker, AD, Grenfell, BT, and Metcalf, CJE. Disease and healthcare burden of COVID-19 in the United States. Nat Med. (2020) 26:1212–7. doi: 10.1038/s41591-020-0952-y
3. Butler, RJ, Contreras, M, Burton, LC, Plisky, PJ, Goode, A, and Kiesel, K. Modifiable risk factors predict injuries in firefighters during training academies. Work. (2013) 46:11–7. doi: 10.3233/WOR-121545
4. Miranda, AR, Scotta, AV, Cortez, MV, and Soria, EA. Triggering of postpartum depression and insomnia with cognitive impairment in Argentinian women during the pandemic COVID-19 social isolation in relation to reproductive and health factors. Midwifery. (2021) 102:11. doi: 10.1016/j.midw.2021.103072
5. Chen, WC, Chen, SJ, and Zhong, BL. Sense of alienation and its associations with depressive symptoms and poor sleep quality in older adults who experienced the lockdown in Wuhan, China, during the COVID-19 pandemic. J Geriatr Psychiatry Neurol. (2022) 35:215–22. doi: 10.1177/08919887221078564
6. Gu, Y, Zhu, Y, Xu, F, Xi, J, and Xu, G. Factors associated with mental health outcomes among patients with COVID-19 treated in the Fangcang shelter hospital in China. Asia Pac Psychiatry. (2021) 13. doi: 10.1111/appy.12443
7. Epifanio, MS, Andrei, F, Mancini, G, Agostini, F, Piombo, MA, Spicuzza, V, et al. The impact of COVID-19 pandemic and lockdown measures on quality of life among Italian general population. J Clin Med. (2021) 10:289. doi: 10.3390/jcm10020289
8. Kilincel, S, Kilincel, O, Muratdagi, G, Aydin, A, and Usta, MB. Factors affecting the anxiety levels of adolescents in home-quarantine duringCOVID-19 pandemic in Turkey. Asia Pac Psychiatry. (2021) 13:e12406. doi: 10.1111/appy.12406
9. Ferreira, LN, Pereira, LN, da Fé, BM, and Ilchuk, K. Quality of life under the COVID-19 quarantine. Qual Life Res. (2021) 30:1389–405. doi: 10.1007/s11136-020-02724-x
10. Montazeri, F, de Bildt, A, Dekker, V, and Anderson, GM. Network analysis of behaviors in the depression and autism realms: inter-relationships and clinical implications. J Autism Dev Disord. (2020) 50:1580–95. doi: 10.1007/s10803-019-03914-4
11. McNally, RJ, Robinaugh, DJ, Wu, GWY, Wang, L, Deserno, MK, and Borsboom, D. Mental disorders as causal systems: a network approach to posttraumatic stress disorder. Clin Psychol Sci. (2015) 3:836–49. doi: 10.1177/2167702614553230
12. Epskamp, S, Cramer, AOJ, Waldorp, LJ, Schmittmann, VD, and Borsboom, D. Qgraph: network visualizations of relationships in psychometric data. J Stat Softw. (2012) 48:1–18. doi: 10.18637/jss.v048.i04
13. Gauld, C, and Micoulaud-Franchi, JA. Network analysis by systemic text excavation of the concept of personalized psychiatry and precision. Enceph-Rev Psychiatr Clin Biol Ther. (2021) 47:341–7. doi: 10.1016/j.encep.2020.08.008
14. Cramer, AOJ, Waldorp, LJ, Van Der Maas, HLJ, and Borsboom, D. Complex realities require complex theories: refining and extending the network approach to mental disorders. Behav Brain Sci. (2010) 33:178–93. doi: 10.1017/S0140525X10000920
15. Jones, PJ, Ma, RF, and McNally, RJ. Bridge centrality: a network approach to understanding comorbidity. Multivariate Behav Res. (2021) 56:353–67. doi: 10.1080/00273171.2019.1614898
16. Qi, JJ, Sun, R, and Zhou, X. Network analysis of comorbid posttraumatic stress disorder and depression in adolescents across COVID-19 epidemic and typhoon Lekima. J Affect Disord. (2021) 295:594–603. doi: 10.1016/j.jad.2021.08.080
17. Cai, H, Chow, IHI, Lei, SM, Lok, GKI, Su, ZH, Cheung, T, et al. Inter-relationships of depressive and anxiety symptoms with suicidality among adolescents: a network perspective. J Affect Disord. (2023) 324:480–8. doi: 10.1016/j.jad.2022.12.093
18. Li, W, Zhao, YJ, Zhang, SF, Yang, BX, Cheung, T, Jackson, T, et al. Mapping post-traumatic stress disorder symptoms and quality of life among residents of Wuhan, China after the COVID-19 outbreak: a network perspective. J Affect Disord. (2022) 318:80–7. doi: 10.1016/j.jad.2022.08.074
19. Chen, P, Zhang, L, Sha, S, Lam, MI, Lok, KI, Chow, IHI, et al. Prevalence of insomnia and its association with quality of life among Macau residents shortly after the summer 2022 COVID-19 outbreak: a network analysis perspective. Front Psych. (2023) 14:11. doi: 10.3389/fpsyt.2023.1113122
20. Liu, D, Epskamp, S, Isvoranu, AM, Chen, CX, Liu, WJ, and Hong, XY. Network analysis of physical and psychiatric symptoms of hospital discharged patients infected with COVID-19. J Affect Disord. (2021) 294:707–13. doi: 10.1016/j.jad.2021.07.043
21. Bai, W, Zhao, YJ, Cai, H, Sha, S, Zhang, QE, Lei, SM, et al. Network analysis of depression, anxiety, insomnia and quality of life among Macau residents during the COVID-19 pandemic. J Affect Disord. (2022) 311:181–8. doi: 10.1016/j.jad.2022.05.061
22. Peng, P, Chen, QN, Liang, MN, Liu, YH, Chen, SB, Wang, YF, et al. A network analysis of anxiety and depression symptoms among Chinese nurses in the late stage of the COVID-19 pandemic. Front Public Health. (2022) 10:10. doi: 10.3389/fpubh.2022.996386
23. Galletta, M, Piras, I, Finco, G, Meloni, F, D'Aloja, E, Contu, P, et al. Worries, preparedness, and perceived impact of Covid-19 pandemic on Nurses' mental health. Front public health. (2021) 9:566700. doi: 10.3389/fpubh.2021.566700
24. Firew, T, Sano, ED, Lee, JW, Flores, S, Lang, K, Salman, K, et al. Protecting the front line: a cross-sectional survey analysis of the occupational factors contributing to healthcare workers' infection and psychological distress during the COVID-19 pandemic in the USA. BMJ Open. (2020) 10:e042752. doi: 10.1136/bmjopen-2020-042752
25. Tao, Y, Liu, X, Hou, W, Niu, H, Wang, S, Ma, Z, et al. The mediating role of emotion regulation strategies in the relationship between big five personality traits and anxiety and depression among Chinese firefighters. Front Public Health. (2022) 10:901686. doi: 10.3389/fpubh.2022.901686
26. Chen, X, Zhang, L, Peng, Z, and Chen, S. Factors influencing the mental health of firefighters in Shantou City, China. Psychol Res Behav Manag. (2020) 13:529–36. doi: 10.2147/prbm.S249650
27. Roberts, MA, O'Dea, J, Boyce, A, and Mannix, ET. Fitness levels of firefighter recruits before and after a supervised exercise training program. J Strength Cond Res. (2002) 16:271–7.
28. Davis, J, and Gallagher, S. Physiological demand on firefighters crawling during a search exercise. Int J Ind Ergon. (2014) 44:821–6. doi: 10.1016/j.ergon.2014.10.001
29. Xu, ZJ, Yu, XY, Zhang, DX, Zheng, XX, Zhang, ZH, Lee, RCM, et al. Does it matter who you live with during COVID-19 lockdown? Association of living arrangements with psychosocial health, life satisfaction, and quality of life: a pilot study. Int J Environ Res Public Health. (2022) 19:1827. doi: 10.3390/ijerph19031827
30. Xie, M, Tang, YG, Zhu, L, Dai, MH, Wu, YL, Huang, YQ, et al. Childhood trauma and mental health status in general population: a series mediation examination of psychological distress in COVID-19 pandemic and global sleep quality. Front Psych. (2021) 12:9. doi: 10.3389/fpsyt.2021.782913
31. Cai, H, Bai, W, Sha, S, Zhang, L, Chow, IHI, Lei, SM, et al. Identification of central symptoms in internet addictions and depression among adolescents in Macau: a network analysis. J Affect Disord. (2022) 2022:415–23. doi: 10.1016/j.jad.2022.01.068
32. Cai, H, Zhao, YJ, He, F, Li, SY, Li, ZL, Zhang, WY, et al. Internet addiction and residual depressive symptoms among clinically stable adolescents with major psychiatric disorders during the COVID-19 pandemic: a network analysis perspective. Transl Psychiatry. (2023) 13:186. doi: 10.1038/s41398-023-02468-5
33. Zhou, JY, Liu, L, Xue, P, Yang, XR, and Tang, XD. Mental health response to the COVID-19 outbreak in China. Am J Psychiatry. (2020) 177:574–5. doi: 10.1176/appi.ajp.2020.20030304
34. Chen, M, Sheng, L, and Qu, S. Diagnostic test of screening depressive disorder in general hospital with the patient health questionnaire (in Chinese). Chin Mental Health. (2015) 29:241–5.
35. Xuyong, HW. The reliability and validity of patient health questionnaire depression module (PHQ-9) in Chinese elderly. Shanghai Arch Psychiatry. (2007) 5:257–259+276.
36. Kroenke, K, Spitzer, RL, and Williams, JBW. The PHQ-9 - validity of a brief depression severity measure. J Gen Intern Med. (2001) 16:606–13. doi: 10.1046/j.1525-1497.2001.016009606.x
37. Cai, H, Xi, HT, An, FR, Wang, ZW, Han, L, Liu, S, et al. The association between internet addiction and anxiety in nursing students: a network analysis. Front Psych. (2021) 12:10. doi: 10.3389/fpsyt.2021.723355
38. Löwe, B, Decker, O, Müller, S, Brähler, E, Schellberg, D, Herzog, W, et al. Validation and standardization of the generalized anxiety disorder screener (GAD-7) in the general population. Med Care. (2008) 46:266–74. doi: 10.1097/MLR.0b013e318160d093
39. He, XY, Li, CB, Qian, J, Cui, HS, and Wu, WY. Reliability and validity of a generalized anxiety scale in general hospital outpatients (in Chinese). Shanghai Arch Psychiatry. (2010) 22:200–3.
40. Qing-Zhi, Z, Yan-Ling, HE, Han, L, Ju-Ming, M, Jian-Xin, C, and Hai-Nan, XU. Reliability and validity of Chinese version of the generalized anxiety disorder 7-item (GAD-7) scale in screening anxiety disorders in outpatients from traditional Chinese internal department. Chin Ment Health J. (2013) 3:163–8.
41. Spitzer, RL, Kroenke, K, Williams, JBW, and Lowe, B. A brief measure for assessing generalized anxiety disorder – the GAD-7. Arch Intern Med. (2006) 166:1092–7. doi: 10.1001/archinte.166.10.1092
42. Kroenke, K, Spitzer, RL, Williams, JBW, and Lowe, B. The patient health questionnaire somatic, anxiety, and depressive symptom scales: a systematic review. Gen Hosp Psych. (2010) 32:345–59. doi: 10.1016/j.genhosppsych.2010.03.006
43. Yu, DSF. Insomnia severity index: psychometric properties with Chinese community-dwelling older people. J Adv Nurs. (2010) 66:2350–9. doi: 10.1111/j.1365-2648.2010.05394.x
44. Bai, C, Daihong, J, Chen, L, Liang, L, and Wang, C. Reliability and validity of insomnia severity index in clinical insomnia patients. Chin J Prac Nurs. (2018) 34:2182–6.
45. Bastien, CH, Vallieres, A, and Morin, CM. Validation of the insomnia severity index as an outcome measure for insomnia research. Sleep Med. (2001) 2:297–307. doi: 10.1016/S1389-9457(00)00065-4
46. Cheung, T, Jin, Y, Lam, S, Su, ZH, Hall, BJ, and Xiang, YT. Network analysis of depressive symptoms in Hong Kong residents during the COVID-19 pandemic. Transl Psychiatry. (2021) 11:460. doi: 10.1038/s41398-021-01543-z
47. Kim, D, Kwon, HJ, Ha, M, Lim, MH, and Kim, KM. Network analysis for the symptom of depression with Children's depression inventory in a large sample of school-aged children. J Affect Disord. (2021) 281:256–63. doi: 10.1016/j.jad.2020.12.002
48. Zhao, YJ, Zhang, C, Guo, T, Sha, S, Su, ZH, Cheung, T, et al. Associations between post-traumatic stress symptoms and quality of life among psychiatric healthcare personnel in China during the COVID-19 pandemic: a network approach. Front Psych. (2023) 14:975443. doi: 10.3389/fpsyt.2023.975443
49. Harper, A, Power, M, and Grp, W. Development of the World Health Organization WHOQOL-BREF quality of life assessment. Psychol Med. (1998) 28:551–8. doi: 10.1017/s0033291798006667
50. Martinez-Quintana, E, Estupiñán-León, H, Rojas-Brito, AB, Déniz-Déniz, L, Barreto-Martín, A, and Rodríguez-González, F. Quality of life in congenital heart disease patients according to their anatomical and physiological classification. Congenit Heart Dis. (2023) 18:197–206. doi: 10.32604/CHD.2021.013308
51. R Core Team. (2020). R: A Language and Environment for Statistical Computing. Available at: https://www.R-project.org/ (accessed April 18, 2023).
52. Zavlis, O, Butter, S, Bennett, K, Hartman, TK, Hyland, P, Mason, L, et al. How does the COVID-19 pandemic impact on population mental health? A network analysis of COVID influences on depression, anxiety and traumatic stress in the UK population. Psychol Med. (2022) 52:3825–33. doi: 10.1017/S0033291721000635
53. Van Borkulo, CD, Borsboom, D, Epskamp, S, Blanken, TF, Boschloo, L, Schoevers, RA, et al. A new method for constructing networks from binary data. Sci Rep. (2014) 4:5918. doi: 10.1038/srep05918
54. Chen, M-Y, Chen, P, An, F-R, Sha, S, Feng, Y, Su, Z, et al. Depression, anxiety and suicidality among Chinese mental health professionals immediately after China's dynamic zero-COVID policy: a network perspective. J Affect Disord. (2024) 352:153–62. doi: 10.1016/j.jad.2024.01.270
55. Jin, Y, Sun, H-L, Lam, SC, Su, Z, Hall, BJ, Cheung, T, et al. Depressive symptoms and gender differences in older adults in Hong Kong during the COVID-19 pandemic: a network analysis approach. Int J Biol Sci. (2022) 18:3934–41. doi: 10.7150/ijbs.69460
56. Zhao, N, Li, W, Zhang, S-F, Yang, BX, Sha, S, Cheung, T, et al. Network analysis of depressive symptoms among residents of Wuhan in the later stage of the COVID-19 pandemic. Front Psych. (2021) 12:735973. doi: 10.3389/fpsyt.2021.735973
57. Epskamp, S, Borsboom, D, and Fried, EI. Estimating psychological networks and their accuracy: a tutorial paper. Behav Res Methods. (2018) 50:195–212. doi: 10.3758/s13428-017-0862-1
58. Jones, P. Networktools: tools for identifying important nodes in Networks_. R package version 1.5.0. (2022).
59. Cai, H, Zhao, YJ, Xing, XM, Tian, TF, Qian, W, Liang, SX, et al. Network analysis of comorbid anxiety and insomnia among clinicians with depressive symptoms during the late stage of the COVID-19 pandemic: a cross-sectional study. Nat Sci Sleep. (2022) 14:1351–62. doi: 10.2147/nss.S367974
60. Marchetti, I. Hopelessness: a network analysis. Cogn Ther Res. (2019) 43:611–9. doi: 10.1007/s10608-018-9981-y
61. Zhao, N, Zhao, YJ, An, F, Zhang, Q, Sha, S, Su, Z, et al. Network analysis of comorbid insomnia and depressive symptoms among psychiatric practitioners during the COVID-19 pandemic. J Clin Sleep Med. (2023) 19:1271–9. doi: 10.5664/jcsm.10586
62. Robinaugh, DJ, Millner, AJ, and McNally, RJ. Identifying highly influential nodes in the complicated grief network. J Abnorm Psychol. (2016) 125:747–57. doi: 10.1037/abn0000181
63. Haslbeck, JMB, and Waldorp, LJ. Mgm: estimating time-varying mixed graphical models in high-dimensional data. J Stat Softw. (2020) 93:1–46. doi: 10.18637/jss.v093.i08
64. Le, HT, Lai, AJX, Sun, JQ, Hoang, MT, Vu, LG, Pham, HQ, et al. Anxiety and depression among people under the Nationwide partial lockdown in Vietnam. Front Public Health. (2020) 8:8. doi: 10.3389/Fpubh.2020.589359
65. Lei, L, Huang, XM, Zhang, S, Yang, JR, Yang, L, and Xu, M. Comparison of prevalence and associated factors of anxiety and depression among people affected by versus people unaffected by quarantine during the COVID-9 epidemic in southwestern China. Med Sci Monitor. (2020) 26:12. doi: 10.12659/msm.924609
66. Sutton, DA, Moldofsky, H, and Badley, EM. Insomnia and health problems in Canadians. Sleep. (2001) 24:665–70. doi: 10.1093/sleep/24.6.665
67. Van Borkulo, CD, Van Bork, R, Boschloo, L, Kossakowski, JJ, Tio, P, Schoevers, RA, et al. Comparing network structures on three aspects: a permutation test. Psychol Methods. (2022) 28:14. doi: 10.1037/met0000476
68. Gupta, P, Anupama, BK, and Ramakrishna, K. Prevalence of depression and anxiety among medical students and house staff during the COVID-19 health-care crisis. Acad Psych. (2021) 45:575–80. doi: 10.1007/s40596-021-01454-7
69. Hyland, P, Shevlin, M, McBride, O, Murphy, J, Karatzias, T, Bentall, RP, et al. Anxiety and depression in the Republic of Ireland during the COVID-19 pandemic. Acta Psychiatr Scand. (2020) 142:249–56. doi: 10.1111/acps.13219
70. Cook, S, Kudryavtsev, AV, Bobrova, N, Saburova, L, Denisova, D, Malyutina, S, et al. Prevalence of symptoms, ever having received a diagnosis and treatment of depression and anxiety, and associations with health service use amongst the general population in two Russian cities. BMC Psychiatry. (2020) 20:537. doi: 10.1186/s12888-020-02938-w
71. Wang, YY, Hu, ZS, Feng, Y, Wilson, A, and Chen, RS. Changes in network centrality of psychopathology symptoms between the COVID-19 outbreak and after peak. Mol Psychiatry. (2020) 25:3140–9. doi: 10.1038/s41380-020-00881-6
72. Zurcher, SJ, Banzer, C, Adamus, C, Lehmann, AI, Richter, D, and Kerksieck, P. Post-viral mental health sequelae in infected persons associated with COVID-19 and previous epidemics and pandemics: systematic review and meta-analysis of prevalence estimates. J Infect Public Health. (2022) 15:599–608. doi: 10.1016/j.jiph.2022.04.005
73. Smits, JAJ, Berry, AC, Rosenfield, D, Powers, MB, Behar, E, and Otto, MW. Reducing anxiety sensitivity with exercise. Depress Anxiety. (2008) 25:689–99. doi: 10.1002/da.20411
74. Paolucci, EM, Loukov, D, Bowdish, DME, and Heisz, JJ. Exercise reduces depression and inflammation but intensity matters. Biol Psychol. (2018) 133:79–84. doi: 10.1016/j.biopsycho.2018.01.015
75. Baumann, MR, Gohm, CL, and Bonner, BL. Phased training for high-reliability occupations: live-fire exercises for civilian firefighters. Hum Factors. (2011) 53:548–57. doi: 10.1177/0018720811418224
76. Escobar-Cordoba, F, Ramirez-Ortiz, J, and Fontecha-Hernandez, J. Effects of social isolation on sleep during the COVID-19 pandemic. Sleep Sci. (2021) 14:86–93. doi: 10.5935/1984-0063.20200097
77. Liu, WN, Yu, FY, Geldsetzer, P, Yang, JT, Wang, ZR, Golden, T, et al. Prevalence of depression in China during the early stage of the COVID-19 pandemic: a cross-sectional study in an online survey sample. BMJ Open. (2022) 12:56667. doi: 10.1136/bmjopen-2021-056667
78. Li, YW, Yang, YS, Luo, GR, Huang, JZ, and Wu, T. The economic recovery from traffic restriction policies during the COVID-19 through the perspective of regional differences and sustainable development: based on human mobility data in China. Sustain For. (2022) 14:6453. doi: 10.3390/su14116453
79. Meng, YL, Wang, X, Dong, P, Yang, YJ, Wang, K, Yan, XL, et al. Comparative analysis of prevention and control measures toward COVID-19 epidemic between Shanghai and Beijing. Front Public Health. (2023) 11:8. doi: 10.3389/fpubh.2023.1121846
80. Mao, AY, Yang, YJ, and Qiu, WQ. The running track of government responsibility during the pandemic, characteristic analysis of policy documents relevant to the COVID-19 pandemic released by Beijing municipal government in 2020. Front Public Health. (2021) 9:10. doi: 10.3389/fpubh.2021.713879
81. Garabiles, MR, Lao, CK, Xiong, YX, and Hall, BJ. Exploring comorbidity between anxiety and depression among migrant Filipino domestic workers: a network approach. J Affect Disord. (2019) 250:85–93. doi: 10.1016/j.jad.2019.02.062
82. Ren, L, Wang, YF, Wu, L, Wei, ZH, Cui, LB, Wei, XY, et al. Network structure of depression and anxiety symptoms in Chinese female nursing students. BMC Psychiatry. (2021) 21:279. doi: 10.1186/s12888-021-03276-1
83. Leung, K, Lau, EHY, Wong, CKH, Leung, GM, and Wu, JT. Estimating the transmission dynamics of SARS-CoV-2 omicron BF.7 in Beijing after adjustment of the zero-COVID policy in November-December 2022. Nat Med. (2023) 29:579–82. doi: 10.1038/s41591-023-02212-y
84. Brooks, SK, Webster, RK, Smith, LE, Woodland, L, Wessely, S, Greenberg, N, et al. The psychological impact of quarantine and how to reduce it: rapid review of the evidence. Lancet. (2020) 395:912–20. doi: 10.1016/S0140-6736(20)30460-8
85. Brotman, MA, Kircanski, K, and Leibenluft, E. Irritability in children and adolescents In: T Widiger and TD Cannon, editors. Annual review of clinical psychology, vol. 13. Palo Alto: Annual Reviews (2017). 317–41.
86. Leibenluft, E, and Stoddard, J. The developmental psychopathology of irritability. Dev Psychopathol. (2013) 25:1473–87. doi: 10.1017/s0954579413000722
87. Association. AP. Diagnostic and statistical manual of mental disorders. 5th ed. Washington DC: American Psychiatric Association (2013).
88. Ohayon, MM, and Roth, T. What are the contributing factors for insomnia in the general population? J Psychosom Res. (2001) 51:745–55. doi: 10.1016/s0022-3999(01)00285-9
89. Morin, CM, LeBlanc, M, Daley, M, Gregoire, JP, and Mérette, C. Epidemiology of insomnia:: prevalence, self-help treatments, consultations, and determinants of help-seeking behaviors. Sleep Med. (2006) 7:123–30. doi: 10.1016/j.sleep.2005.08.008
90. Amaducci, CD, Mota, D, and Pimenta, CAD. Fatigue among nursing undergraduate students. Rev Esc Enferm USP. (2010) 44:1052–8. doi: 10.1590/S0080-62342010000400028
91. Wang, SB, Xu, WQ, Gao, LJ, Tan, WY, Zheng, HR, Hou, CL, et al. Bridge connection between depression and anxiety symptoms and lifestyles in Chinese residents from a network perspective. Front Psych. (2023) 14:10. doi: 10.3389/fpsyt.2023.1104841
92. Carney, CE, Moss, TG, Lachowski, AM, and Atwood, ME. Understanding mental and physical fatigue complaints in those with depression and insomnia. Behav Sleep Med. (2014) 12:272–89. doi: 10.1080/15402002.2013.801345
93. Liu, J, Si, TL, Chen, P, Wang, Y-Y, Su, Z, Cheung, T, et al. Prevalence of COVID-19 fear and its association with quality of life among fire service recruits after ceasing the dynamic zero-COVID policy in China. Front Public Health. (2023) 11:1257943. doi: 10.3389/fpubh.2023.1257943
94. Tanaka, M, Tajima, S, Mizuno, K, Ishii, A, Konishi, Y, Miike, T, et al. Frontier studies on fatigue, autonomic nerve dysfunction, and sleep-rhythm disorder. J Physiol Sci. (2015) 65:483–98. doi: 10.1007/s12576-015-0399-y
95. Kaiser, T, Herzog, P, Voderholzer, U, and Brakemeier, EL. Unraveling the comorbidity of depression and anxiety in a large inpatient sample: network analysis to examine bridge symptoms. Depress Anxiety. (2021) 38:307–17. doi: 10.1002/da.23136
96. Pöttgen, J, Moss-Morris, R, Wendebourg, JM, Feddersen, L, Lau, S, Köpke, S, et al. Randomised controlled trial of a self-guided online fatigue intervention in multiple sclerosis. J Neurol Neurosurg Psychiatry. (2018) 89:970–6. doi: 10.1136/jnnp-2017-317463
97. Wearden, AJ, Riste, L, Dowrick, C, Chew-Graham, C, Bentall, RP, Morriss, RK, et al. Fatigue intervention by nurses evaluation – the FINE trial. a randomised controlled trial of nurse led self-help treatment for patients in primary care with chronic fatigue syndrome: study protocol. ISRCTN74156610. BMC Med. (2006) 4:12. doi: 10.1186/1741-7015-4-9
98. Wang, TW, Xu, C, Pan, KL, and Xiong, HY. Acupuncture and moxibustion for chronic fatigue syndrome in traditional Chinese medicine: a systematic review and meta-analysis. BMC Complement Altern Med. (2017) 17:11. doi: 10.1186/s12906-017-1647-x
99. Wang, YY, Li, XX, Liu, JP, Luo, H, Ma, LX, and Alraek, T. Traditional Chinese medicine for chronic fatigue syndrome: a systematic review of randomized clinical trials. Complement Ther Med. (2014) 22:826–33. doi: 10.1016/j.ctim.2014.06.004
100. Chen, R, Moriya, J, Yamakawa, J, Takahashi, T, and Kanda, T. Traditional Chinese medicine for chronic fatigue syndrome. Evid Complement Altern Med. (2010) 7:3–10. doi: 10.1093/ecam/nen017
101. Baldwin, DS, and Papakostas, GI. Symptoms of fatigue and sleepiness in major depressive disorder. J Clin Psychiatry. (2006) 67:9–15.
102. McAuley, E, and Morris, KS. Advances in physical activity and mental health: quality of life. Am J Lifestyle Med. (2007) 1:389–96. doi: 10.1177/1559827607303243
103. Barthel, AL, Pinaire, MA, Curtiss, JE, Baker, AW, Brown, ML, Hoeppner, SS, et al. Anhedonia is central for the association between quality of life, metacognition, sleep, and affective symptoms in generalized anxiety disorder: a complex network analysis. J Affect Disord. (2020) 277:1013–21. doi: 10.1016/j.jad.2020.08.077
104. Bonati, M, Campi, R, and Segre, G. Psychological impact of the quarantine during the COVID-19 pandemic on the general European adult population: a systematic review of the evidence. Epidemiol Psychiatr Sci. (2022) 31:19. doi: 10.1017/s2045796022000051
105. Pang, JCY, Chan, ELS, Lau, HMC, Reeves, KKL, Chung, THY, Hui, HWL, et al. The impacts of physical activity on psychological and behavioral problems, and changes in physical activity, sleep and quality of life during the COVID-19 pandemic in preschoolers, children, and adolescents: a systematic review and meta-analysis. Front Pediatr. (2023) 11:13. doi: 10.3389/fped.2023.1015943
106. Pappa, S, Chen, JY, Barnett, J, Chang, AA, Dong, RK, Xu, W, et al. A systematic review and meta-analysis of the mental health symptoms during the Covid-19 pandemic in Southeast Asia. Psychiatry Clin Neurosci. (2022) 76:41–50. doi: 10.1111/pcn.13306
107. Liu, JY, Kwan, C, Deng, J, and Hu, YX. The mental health impact of the COVID-19 pandemic on older adults in China: a systematic review. Int J Environ Res Public Health. (2022) 19:14362. doi: 10.3390/ijerph192114362
108. Supartini, A, Honda, T, Basri, NA, Haeuchi, Y, Chen, S, Ichimiya, A, et al. The impact of sleep timing, sleep duration, and sleep quality on depressive symptoms and suicidal ideation amongst Japanese freshmen: the EQUSITE study. Sleep Disorder. (2016) 2016:8737654–10. doi: 10.1155/2016/8737654
109. Feliciano, EMC, Quante, M, Rifas-Shiman, SL, Redline, S, Oken, E, and Taveras, EM. Objective sleep characteristics and Cardiometabolic health in young adolescents. Pediatrics. (2018) 142:e20174085. doi: 10.1542/peds.2017-4085
110. Scott, AJ, Webb, TL, Martyn-St James, M, Rowse, G, and Weich, S. Improving sleep quality leads to better mental health: a meta-analysis of randomised controlled trials. Sleep Med Rev. (2021) 60:19. doi: 10.1016/j.smrv.2021.101556
111. Yang, JP, Lin, RJ, Sun, K, and Gao, LL. Incidence and correlates of insomnia and its impact on health-related quality of life among Chinese pregnant women: a cross-sectional study. J Reprod Infant Psychol. (2023) 41:391–402. doi: 10.1080/02646838.2021.2020228
112. Ministry of Emergency Management of the People's Republic of China. National Comprehensive Fire Rescue Team 2022 Annual Examination Recruitment Cadre Interview Announcement. (2022). Available at: https://www.mem.gov.cn/gk/zfxxgkpt/fdzdgknr/202201/t20220120_407031.shtml (accessed October 12, 2023).
113. Luceño-Moreno, L, Talavera-Velasco, B, García-Albuerne, Y, and Martín-García, J. Symptoms of posttraumatic stress, anxiety, depression, levels of resilience and burnout in Spanish health personnel during the COVID-19 pandemic. Int J Environ Res Public Health. (2020) 17:5514. doi: 10.3390/ijerph17155514
114. Koffman, J, Gross, J, Etkind, SN, and Selman, L. Uncertainty and COVID-19: how are we to respond? J R Soc Med. (2020) 113:211–6. doi: 10.1177/0141076820930665
115. Blix, I, Birkeland, MS, and Thoresen, S. Worry and mental health in the Covid-19 pandemic: vulnerability factors in the general Norwegian population. BMC Public Health. (2021) 21:928. doi: 10.1186/s12889-021-10927-1
116. Wu, D, Yang, TZ, Hall, DL, Jiao, GH, Huang, LX, and Jiao, C. COVID-19 uncertainty and sleep: the roles of perceived stress and intolerance of uncertainty during the early stage of the COVID-19 outbreak. BMC Psychiatry. (2021) 21:306. doi: 10.1186/s12888-021-03310-2
117. Mohammadkhanizadeh, A, and Nikbakht, F. Investigating the potential mechanisms of depression induced-by COVID-19 infection in patients. J Clin Neurosci. (2021) 91:283–7. doi: 10.1016/j.jocn.2021.07.023
118. Liu, TY, Peng, MM, Au, WSH, Wong, FHC, Kwok, WW, Yin, JY, et al. Depression risk among community-dwelling older people is associated with perceived COVID-19 infection risk: effects of news report latency and focusing on number of infected cases. Aging Ment Health. (2023) 27:475–82. doi: 10.1080/13607863.2022.2045562
Keywords: depression, anxiety, quality of life, network analysis, insomnia
Citation: Liu J, Gui Z, Chen P, Cai H, Feng Y, Ho T-I, Rao S-Y, Su Z, Cheung T, Ng CH, Wang G and Xiang Y-T (2024) A network analysis of the interrelationships between depression, anxiety, insomnia and quality of life among fire service recruits. Front. Public Health. 12:1348870. doi: 10.3389/fpubh.2024.1348870
Edited by:
S. M. Yasir Arafat, Biomedical Research Foundation, BangladeshReviewed by:
Yanqiang Tao, Beijing Normal University, ChinaHongguang Chen, Peking University Sixth Hospital, China
Copyright © 2024 Liu, Gui, Chen, Cai, Feng, Ho, Rao, Su, Cheung, Ng, Wang and Xiang. This is an open-access article distributed under the terms of the Creative Commons Attribution License (CC BY). The use, distribution or reproduction in other forums is permitted, provided the original author(s) and the copyright owner(s) are credited and that the original publication in this journal is cited, in accordance with accepted academic practice. No use, distribution or reproduction is permitted which does not comply with these terms.
*Correspondence: Yu-Tao Xiang, eHl1dGx5QGdtYWlsLmNvbQ==; Gang Wang, Z2FuZ3dhbmdkb2NAY2NtdS5lZHUuY24=; Chee H. Ng, Y25nQHVuaW1lbGIuZWR1LmF1
†These authors have contributed equally to this work