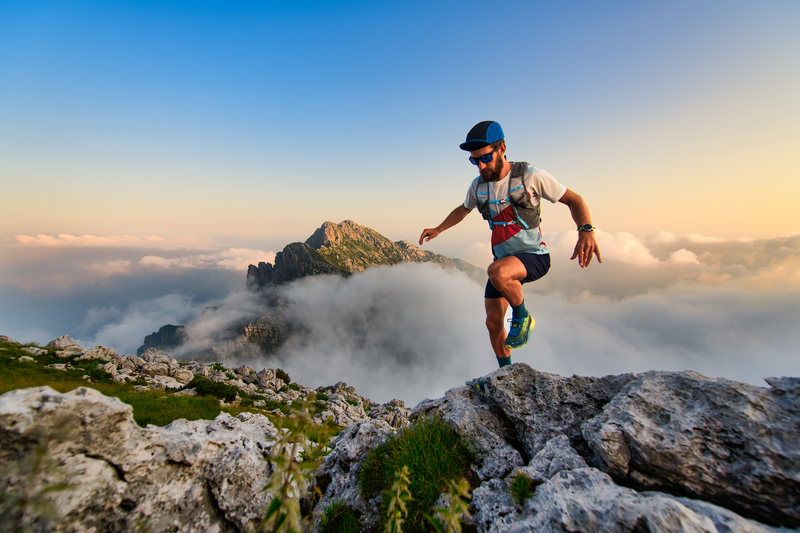
95% of researchers rate our articles as excellent or good
Learn more about the work of our research integrity team to safeguard the quality of each article we publish.
Find out more
ORIGINAL RESEARCH article
Front. Public Health , 01 May 2024
Sec. Health Economics
Volume 12 - 2024 | https://doi.org/10.3389/fpubh.2024.1331522
This article is part of the Research Topic Multilevel Medical Security Systems and Big Data in Healthcare: Trends and Developments View all 11 articles
Background: Measuring the development of Chinese centers for disease control and prevention only by analyzing human resources for health seems incomplete. Moreover, previous studies have focused more on the quantitative changes in healthcare resources and ignored its determinants. Therefore, this study aimed to analyze the allocation of healthcare resources in Chinese centers for disease control and prevention from the perspective of population and spatial distribution, and to further explore the characteristics and influencing factors of the spatial distribution of healthcare resources.
Methods: Disease control personnel density, disease control and prevention centers density, and health expenditures density were used to represent human, physical, and financial resources for health, respectively. First, health resources were analyzed descriptively. Then, spatial autocorrelation was used to analyze the spatial distribution characteristics of healthcare resources. Finally, we used spatial econometric modeling to explore the influencing factors of healthcare resources.
Results: The global Moran index for disease control and prevention centers density decreased from 1.3164 to 0.2662 (p < 0.01), while the global Moran index for disease control personnel density increased from 0.4782 to 0.5067 (p < 0.01), while the global Moran index for health expenditures density was statistically significant only in 2016 (p < 0.1). All three types of healthcare resources showed spatial aggregation. Population density and urbanization have a negative impact on the disease control and prevention centers density. There are direct and indirect effects of disease control personnel density and health expenditures density. Population density and urbanization had significant negative effects on local disease control personnel density. Urbanization has an indirect effect on health expenditures density.
Conclusion: There were obvious differences in the spatial distribution of healthcare resources in Chinese centers for disease control and prevention. Social, economic and policy factors can affect healthcare resources. The government should consider the rational allocation of healthcare resources at the macro level.
Adequate healthcare resources are the cornerstone of a functioning health system and a prerequisite for ensuring that effective health services are available to different groups (1, 2). Healthcare resources allocation refers to the manner in which healthcare resources flow between health care institutions (sectors) or regions, and can reflect the level of health services (2). Healthcare resources affect the residents’ access to health care services, and studies show a positive correlation between healthcare resources and access to health care (3, 4). To optimize the allocation of healthcare resources in rural areas and contribute to more convenient access to health services for local residents (4). Rational healthcare resources allocation helps people achieve more desirable health outcomes (5, 6). For example, it has been found that adequate healthcare resources are conducive to reducing child COVID-19 mortality and morbidity (7). Health professionals are able to provide quality health services to tuberculosis patients, improving their quality of life and health outcomes (8).
The uneven distribution of healthcare resources is a long-standing challenge in China. It is also prevalent in other countries (regions), such as the United States (9), Portugal (10), Japan (11), Kenya (12), and Southeast Asia (13). As the largest developing country with a vast land area and a large population, China faces the challenge of maximizing the provision of comprehensive health care services given the constraints on available healthcare resources (14). A number of studies had evaluated healthcare resources allocation in China, but focused more on the entire health care system (15), Traditional Chinese Medicine (14), maternal and child health care (16), and primary health care system (17). Little attention is paid to the disease prevention and control system.
Chinese Centers for Disease Control and Prevention (CCDC) are an important part of China’s public health system. It has a mission to create a healthy and safe living environment, maintain social stability and promote people’s health by preventing and controlling disease, injury and disability (18). CCDC began in the 1940s and has been developing for more than 70 years. Nowadays, a unique four-tier disease prevention and control system has been formed, which contains national, provincial, municipal and county-level disease prevention and control centers (19). In 2016, the Healthy China 2030 plan emphasized adherence to prevention as the mainstay and prevention and control of major diseases, highlighting the importance of the CCDC in promoting the process of Healthy China (20). In 2019, the COVID-19 outbreak gave the public a more direct understanding of CCDC’s critical role in public health incident response. After the epidemic, the government of China accelerated the development and construction of the disease prevention and control system, with particularly focusing on optimizing the allocation of healthcare resources in regional CCDC (21). In 2023, the State Council of China issued the Guiding Opinions on Promoting the High-Quality Development of the Disease Prevention and Control Business, which particularly emphasized the necessity of promoting the reform of the CCDC system and building a strong public health system (22).
Healthcare resources affect the capacity and capability of CCDC to provide preventive health services (23). Health workforce is the most dynamic and critical element of the CCDC system, determining the level and quality of health services and influencing citizens’ opportunities to access preventive care (24). Health material resources are the essential condition for the provision of preventive health services to citizens and the basic indicator of the level of public health service. Health financial resources represent the impact of healthcare resources allocation on the health of the population (25). In summary, healthcare resources are crucial to the development of CCDC. Adequate healthcare resources will not only be conducive to promoting the high-quality development of CDC, but will also facilitate the achievement of the strategic goal of Healthy China. Therefore, it is necessary to conduct a detailed study of CCDC healthcare resources.
Currently, scholars mostly use equity analysis methods to analyze healthcare resources, such as the Gini coefficient, the Theil index and the Health Resource Density Index(HRDI), etc. (26). Ao et al. used the Gini coefficient, the Theil index and HRDI to evaluate the equity of rural healthcare resources in China (27). Jian sun used the concentration index to analyze the equity in the distribution of health materials and health human resources (28). Liu et al. used the Gini coefficient combined with spatial autocorrelation to analyze the inequality of healthcare resources in Traditional Chinese Medicine hospitals (29). These methods analyzed equity in the regional allocation of healthcare resources only in terms of population and geographic area. Although spatial autocorrelation analysis was applied, the distribution of healthcare resources was not spatially analyzed in depth. In addition, most of the studies on CCDC only used descriptive analysis methods such as rates and composition ratios to make a simple quantitative analysis of human resources for health (30–32). Fewer studies have addressed financial and material resources for health and have not been analyzed using more specialized statistical methods.
Based on panel data from 2016 to 2021, this study aims to use spatial autocorrelation analysis to explore the characteristics of the distribution of healthcare resources in CCDC and use spatial econometric models to assess the factors influencing health resources in CCDC from four major aspects: social, economic, health, and policy. To provide a theoretical basis for the health department to carry out the next health system reform and to gain a more comprehensive understanding of the factors affecting the development of the CDC from multiple perspectives.
This study used panel data for 31 provinces in China from 2016 to 2021. Hong Kong, Macau and Taiwan provinces are not included because of inconsistent statistical standards for the data. All data are from the China Health and Family Planning Statistical Yearbook, China Health Statistical Yearbook and China Statistical Yearbook.
Based on the configuration of the population served and with reference to other studies, the number of disease control personnel per 10,000 population (disease control personnel density), the number of CCDC per 10,000 population (CCDC density), and per capita health expenditures (health expenditures density) were selected as indicators of healthcare resources in this study (33–36). The calculation method is as follows.
As health systems develop, health resources are influenced by a variety of factors, such as geographic differences (17) economic levels (37), and topographic conditions (38). Based on previous studies and data availability, we chose to explore four factors that may influence CCDC healthcare resources development in terms of social development, economic conditions, health factors, and policy factor. Table 1 shows the meaning and abbreviation of the variables.
Urbanization can reflect the level of public health services in a region (39). A higher level of urbanization means more developed transportation and easier access to health care services (40). The population density is used to measure the demand for healthcare resources in an area, which also benefits the government in measuring how to allocate health expenditures (41).
Per capita GDP is often used as an indicator of the level of economic development (41). The distribution of human resources for health is influenced by per capita GDP, with higher per capita GDP attracting more health professionals (42). In both urban and rural areas, per capita health care expenditures are correlated with the amount of healthcare resources available in the neighborhood (43).
Aging and infectious diseases can increase health expenditure, leading to financial burden on the country (44–46). The older adult have more and more urgent needs for healthcare services due to the deterioration of their natural physiological functions, such as reduced mobility and resistance (47). The outbreak of infectious diseases not only threatens the health of population, but also seriously affects the utilization of health care resources (42).
In China, health expenditures of the CCDC are composed of government financial input, income from healthcare services, and social funds. In fact, government finances make up a larger proportion. When the financial proportion is low, it affects the scope of health services provided by health care institutions (48).
In this study, we use the queen collinearity matrix (49), and regions with common points or common edges are adjacent to each other. Since there is no neighboring province in Hainan Province, it is set that Hainan Province and Guangdong Province are mutually neighboring according to previous studies (50, 51). The assignment rules are as follows:
The global Moran index and the local Moran index are usually used to analyze the spatial correlation of the studied indicators (52, 53). The global Moran index is used to characterize the spatial aggregation and distribution of overall health indicators, but it cannot clearly indicate the spatial aggregation area. Therefore, local spatial autocorrelation is used to measure the regions where spatial aggregation occurs (54). The formula is as follows:
where and denote HP_D or CCDC_D or HE_D in provinces i and j, respectively, is the average value, and denotes the spatial weight matrix between provinces i and j.
The standardized statistic z-value is used to determine whether the global Moran index and local Moran index pass the test. The formula is as follows:
where E[I] and var[I] are the mathematical expectation and variance, respectively.
The global Moran index has a range of values from-1 to 1. When the global Moran index I > 0, it indicates spatial positive correlation; when the global Moran index I < 0, it indicates spatial negative correlation; when I = 0, it indicates spatial random distribution. If the local Moran index >0, it means that the health indicators tend to cluster together (high values are adjacent to high values or low values are adjacent to low values), and if the local Moran index <0, it means that the health indicators do not tend to cluster together (high values are adjacent to low values or low values are adjacent to high values).
Traditional OLS regression models do not consider spatial factors. Therefore, this study uses spatial econometric models to explore the factors influencing healthcare resources. There are three common traditional spatial econometric models: spatial error model (SEM), spatial lag model (SLM), and spatial Durbin model (SDM).
The SLM model is often used to explore whether the value of a spatial unit is influenced by its neighboring spaces, while the SEM model is often used to analyze the case where the dependent variable is related to a set of variables and a spatial autocorrelation error term. However, the SDM model is used to analyze the case where the dependent variable is influenced by the independent variables of this spatial unit and the neighboring spatial units. The SEM, SLM and SDM models constructed in this study are formulated as follows:
SEM:
SLM:
SDM:
where denotes the dependent variable (CCDC_D, DCP_D, or HE_D) in province i in year t; W denotes the spatial weight matrix; is the spatial autoregressive coefficient; denotes the intercept of the regression; and denote the individual and time effects, respectively; denotes the random error term; is the spatial effect of the random error; denotes the effect of the explanatory variable in province i on the explained variable; denotes the effect of the explanatory variable in neighboring provinces on the explained variable in province i.
This study uses a logarithmic transformation to reduce heteroskedasticity, implying that the effect of the independent variable on the dependent variable is explained in percentage form (55). If the correlation coefficient is less than 0.85, there is no multicollinearity in the regression model. Table 2 shows the correlations among the variables, all of which are less than 0.85, which indicates that there is no problem of multicollinearity in this study.
To select the optimal spatial econometric model, we used the Lagrange multiplier test statistic (LM), robust Lagrange multiplier statistic (RLM), and likelihood ratio test (LR) to determine the appropriate model, and then performed the Hausman test to determine whether to choose a fixed-effects model or a random-effects model. Finally, the Goodness-of-Fit (R2) as the main basis for determining the model. All analyses were performed in STATA 16.0. p < 0.05 was considered statistically significant in this study.
From 2016 to 2021, DCP_D and HE_D showed an overall increasing trend, while CCDC_D showed a decreasing trend year by year (Figure 1). The descriptive statistical analysis of each dependent and independent variable in this study was shown in Table 3. The mean values of CCDC_D, DCP_D and HE_D for 2016–2021 were 1.58, 0.04 and 11.07, respectively (Table 3). It should be noted that CCDC_D and DCP_D in the western region were significantly more than those in the central and eastern regions, with Tibet having the highest CCDC_D and DCP_D. Beijing has the highest HE_D of all provinces. Compared to the policy’s disease control personnel density standards, only eight provinces qualified in 2021, and mostly located in the western region (Supplementary Table S1).
Besides HE_D and lnPD, other variables showed significant spatial autocorrelation (Tables 4, 5). The global Moran index of CCDC_D decreased from 0.1364 to 0.2662 in 6 years. However, the global Moran index of DCP_D increased from 0.4782 to 0.5067, which indicated a gradually increasing spatial autocorrelation. In addition, we mapped the spatial distribution of CCDC_D, DCP_D, and HE_D (Figures 2A–C). Overall, the three types of healthcare resources showed a trend of gradually decreasing from west to east, and the provinces with lower healthcare resources density were mainly in the eastern coastal areas.
Figure 2. (A) spatial distribution of DCP_D; (B) spatial distribution of CCDC_D; (C) spatial distribution of HE_D; (D) univariate local indicator of spatial association cluster map of DCP_D in 2021; (E) univariate local indicator of spatial association cluster map of CCDC_D in 2021; (F) univariate local indicator of spatial association cluster map of HE_D in 2021. The average value of DCP_D, CCDC_D and HE_D in (A–C) was divided into five levels based on natural breaks (Jenks). These figures are drawn based on the standard map from the National Center for Basic Geographic Information (https://ngcc.cn/ngcc).
The spatial agglomeration of healthcare resources density was presented as maps (Figures 2D–F). In general, high-high cluster was mainly distributed in the western and northeastern regions, including Xinjiang, Tibet, Qinghai and Heilongjiang. Eastern region (Shandong, Jiangsu and Zhejiang, etc.) and central region (Anhui, Jiangxi and Shanxi, etc.) dominated by low-low cluster.
Regarding the DCP_D, high-low cluster was mainly distributed in Henan and Fujian, while low-low cluster was mainly distributed in the central and eastern regions, and most provinces were located near the Yangtze River basin. In terms of the CCDC_D, only Sichuan showed low-high cluster, while high-high cluster was mainly distributed in the west and low-low cluster was concentrated in the east. In terms of HE_D, only a few provinces showed spatial aggregation, with Heilongjiang showing high-high cluster and the other four provinces showing low-low cluster.
The results of the statistical tests used to determine the best spatial econometric model were shown in Table 6. As an example of constructing a spatial econometric model of CCDC_D, LM (error) was significant, and the LR test judged that the SDM model would degenerate into SEM and SLM models, and the Hausman test indicated that the choice of random effects was more appropriate. In summary, the SEM model with random effects was chosen for the spatial effect analysis of the CCDC_D. Through LM test, LR test and Hausman test, we chosen the SDM model with fixed effects to analyze the spatial effects of DCP_D and HE_D.
We calculated the Goodness-of-Fit(R2) to determine individual effects, time effects, and two-way fixed effects. The bigger the R2 value, the better the model fit. As shown in Table 7, time effects were selected for both DCP_D and HE_D. Since there might be inter-individual differences among provinces over time, we chosen two-way fixed effects for further analysis of CCDC_D.
The spatial econometric models for three healthcare resources density were shown in Table 8. In the spatial error model of CCDC_D, population density and urbanization were negatively correlated with CCDC_D, with each 1% increase in population density decreasing CCDC_D by 0.03%. However, each 1% increase in infectious disease prevalence and aging increased the CCDC_D by 0.01 and 0.01%, respectively.
Since there are total, direct and indirect effects in the SDM model, the respective effects of DCP_D and HE_D are shown in Tables 9, 10. In term of DCP_D, population density, per capita GDP, per capita total health expenditure, and urbanization had different degrees of influence on local disease control personnel density. Urbanization and per capita total health expenditure increased by 1%, resulting in 2.38% decrease and 0.74% increase in the DCP_D in the province, respectively. In terms of spillover effects, aging, infectious disease prevalence, and per capita GDP increased by 1%, resulting in 1.05, 0.70, and 1.21% decrease in DCP_D in neighboring provinces, respectively. However, each 1% increase in urbanization was followed by a 1.50% increase in DCP_D in neighboring provinces (Table 9). Population density, urbanization and government financial share had negative effects on HE_D in the province, with each 1% increase decreasing HE_D in the province by 6.55, 24.18 and 14.46%, respectively. Urbanization only had significant spillover effect, with each 1% increase in local urbanization increasing the HE_D of neighboring provinces by 36.92% (Table 10).
This study aims to determine the characteristics of the spatial distribution of healthcare resources in Center for Disease Control and Prevention in China and to explore the influencing factors of health resources, which will help the health department to develop a reasonable resources allocation plan. The results showed that there was a significant spatial autocorrelation of health human resources and health physical resources, and the spatiality of health financial resources was not significant. Social, economic, policy, and health factors all had various degrees of impact on the healthcare resources of the Chinese center for disease control.
We found differences in CCDC density between provinces and the national average, in addition to marked regional differences in health expenditures density. By 2021, disease control personnel density was still below the basic requirements set by the government, with a national average disease control personnel density of 1.48 in 2021, while the government set a standard of 1.75 (56). In general, health professionals and recent medical graduates are reluctant to work at the CCDC. They prefer to work in hospitals or pharmaceutical companies with good development prospects and high salaries. This may have contributed to the shortage of health human resources in CCDC (23). In order to respond the crisis of future disease pandemics, more attention should be paid to developing the quantity and quality of disease control personnel.
This study analyzed the characteristics of the spatial distribution of disease control personnel density, CCDC density, and health expenditures density. The density of CCDC and disease control personnel showed a gradual decrease from west to east, with the highest in western regions such as Tibet, Xinjiang and Qinghai, and the lowest in eastern regions such as Shanghai, Guangdong and Jiangsu. The government of China stipulated that only one CDC should be set up in administrative districts at the county level and above (57). Western provinces such as Xinjiang and Tibet cover a wide area, and have more counties, so more CDC have been established. Moreover, the number of permanent residents in the western provinces is small, both of which make for a high CCDC density in the west. Previous studies had shown that the western region has an adequate health workforce (27, 52, 58), which was made a variety of national recruitment programs to attract talented people to work in the west (59).
However, in the developed regions of eastern China, disease control personnel density is low due to the huge local population base and the limited number of disease control personnel. The treatment of the CCDC may not match the local consumption level, leading to the flow of health talent to hospitals or companies with high salaries (60). Based on the distribution of health expenditures density, it is reasonable to infer that health expenditures are related to the severity of the epidemic in each province. In 2021, the COVID-19 epidemic is more serious in several provinces such as Tibet, Hainan and Liaoning. The government also spent significantly more on health in these provinces than in other provinces with more stable epidemics. Therefore, each province should make a strategic plan for the development of health human resources in the CCDC that is suitable for its own development, and appropriately adjust the size of CCDC personnel on the basis of the actual situation. Healthcare resources should be allocated scientifically to improve the accessibility of health services, taking into account various factors such as the number of inhabitants, geographic environment and public health events.
Spatially, the global Moran index of CCDC_D decreases, indicating that the number of CCDC gradually tends to be evenly distributed in space. Since China began a new round of health care reform in 2009, the public health system had accelerated the construction of CCDC to ensure that preventive health services are fully available (57). However, the global Moran index of disease control personnel density increased, which showed a strong spatial autocorrelation, which was similar to the results of other studies (61). Dong et al. found that the global Moran index of healthcare resources (e.g., doctors, nurses, technicians, etc.) increased to a certain extent from 2010 to 2016 (62). Cheng et al. found that the average density of health personnel increased from 1.60 in 2016 to 1.88 in 2019 (50). These studies implied a gradually expanding spatial aggregation of human resources for health. The high-high aggregation type of the density of CCDC and disease control personnel were mainly distributed in the western region, such as Xinjiang, Tibet and Qinghai, while the low-low aggregation type was distributed in the eastern region, such as Jiangxi, Zhejiang and Jiangsu. Policy changes, population mobility, and flexible employment of health workers may have contributed to this spatial aggregation. There is a necessity for the health sector to have a better understanding of the factors affecting the distribution of healthcare resources in order to be able to formulate an effective regional healthcare resources allocation policy.
In general, healthcare resources are closely linked to social development, economic conditions, policies, and so on. Urbanization, as one of the factors representing social development, has a significant impact on each of the three healthcare resources in this study. The impact of urbanization on health expenditure density is much greater than the impact on the density of CCDC and the density of disease control personnel. This may be due to that the higher the urbanization, the higher the income from health expenditures, which then has a greater impact on the density of health expenditures. In health, disease control personnel density and CCDC density are positively affected by the incidence of infectious diseases. Other scholars have also concluded that during epidemic periods, a large and well-trained health workforce is necessary to actively carry out epidemiological investigations, and so on (63).
In addition, aging has a positive effect on CCDC density. This may be due to the fact that, as the level of awareness increases and aging becomes more serious, people pay more attention to preventive health care, and local governments increase their investment in preventive health care services for the older adult population (64). At the same time, this means that the need for the CCDC is also increasing. Among the economic factors, GDP per capita and per capita health care expenditures have a positive impact on disease control personnel density, and per capita health care expenditures have twice the impact of GDP per capita. In terms of regional impacts, social development, health factors and economic factors have spillover effects on disease control personnel density in neighboring provinces. The GDP of the province is negatively correlated with the density of disease control personnel in the surrounding provinces. Each 1% increase in the province’s GDP leads to 1.21% decrease in disease control personnel density in the surrounding provinces.
Areas with higher economic levels are more likely to attract health personnel from neighboring areas who choose to work across districts (65). Particularly, as the economic level rises, it stimulates the demand for health services among the population (66), and it also increased the local demand for disease control personnel. Aging has a negative spillover effect on the density of disease control personnel in neighboring provinces. Studies have confirmed that the higher the aging, the greater the need for health workforce (67). This may be attributed to a greater need for more and more specialized health care services for the local older adult, which has led to a reduction in the number of disease control personnel in the neighboring provinces. Urbanization has a positive spillover effect on the density of disease control personnel in neighboring provinces, and we believe that urbanization has a spillover effect on health manpower, as confirmed by previous studies (68). Increasing urbanization is conducive to accelerating the mobility of human resources for health across regions. While focusing on the development of urbanization, the government should also pay attention to the impact of urbanization on healthcare resources of CCDC, and actively promote the high quality development of urbanization and CCDC.
In terms of spillover effects of health financing density, only urbanization development has a positive spillover effect on health expenditures density. Increasing urbanization in the province by 1% will cause the health expenditures density in the surrounding provinces to increase by 36.92%. It can be understood that the better developed the city, the higher the level of the health economy, causing the government to redirect investment to neighboring poorer provinces.
This study also has some limitations. Firstly, we calculated healthcare resources density based on population size only and conducted a study on spatial distribution and influencing factors. In the next study, healthcare resources density can be calculated based on land area. Secondly, other factors such as education levels and salary levels may have an impact on healthcare resources (69, 70). However, due to the availability of data, it was not possible to include the level of education and salary level in the study of influencing factors.
In conclusion, our study identified significant spatial variation in healthcare resources allocation of CDC in China. Moreover, the spatial aggregation of health human and health financial resources increased positively over the study period, which reflects the expansion of spatial variability. Social development, economic conditions, health factors and policy factors had impacts on the allocation of healthcare resources not only in the province but also in neighboring provinces.
Publicly available datasets were analyzed in this study. This data can be found at: https://www.stats.gov.cn/.
YiY: Writing – original draft, Writing – review & editing. JL: Writing – review & editing. XD: Writing – review & editing. YaY: Writing – review & editing. LZ: Writing – review & editing.
The author(s) declare that no financial support was received for the research, authorship, and/or publication of this article.
We would like to express our sincere gratitude to all the individuals who contributed to this study.
The authors declare that the research was conducted in the absence of any commercial or financial relationships that could be construed as a potential conflict of interest.
All claims expressed in this article are solely those of the authors and do not necessarily represent those of their affiliated organizations, or those of the publisher, the editors and the reviewers. Any product that may be evaluated in this article, or claim that may be made by its manufacturer, is not guaranteed or endorsed by the publisher.
The Supplementary material for this article can be found online at: https://www.frontiersin.org/articles/10.3389/fpubh.2024.1331522/full#supplementary-material
CCDC_D, Chinese Centers for Disease Control and Prevention density; DCP_D, disease control personnel density; HE_D, health expenditures density; PD, population density; UR, urbanization rate; PTHE, per capita total health expenditure; GDP, per capita GDP; IID, incidence of infectious diseases; EP, proportion of older adult population (aged over 65); GF, proportion of government finance.
1. Qiu, L, Yang, L, Li, H, and Wang, L. The impact of health resource enhancement and its spatiotemporal relationship with population health. Front Public Health. (2023) 10:10. doi: 10.3389/fpubh.2022.1043184
2. Wang, Z, He, H, Liu, X, Wei, H, Feng, Q, and Wei, B. Health resource allocation in Western China from 2014 to 2018. Arch Public Health. (2023) 81:30. doi: 10.1186/s13690-023-01046-x
3. Hou, J, and Ke, Y. Addressing the shortage of health professionals in rural China: issues and progress comment on have health human resources become more equal between rural and urban areas after the new reform? Int J Health Policy Manag. (2015) 4:327–8. doi: 10.15171/ijhpm.2015.57
4. Chen, Y, Yin, Z, and Xie, Q. Suggestions to ameliorate the inequity in urban/rural allocation of healthcare resources in China. Int J Equity Health. (2014) 13:34. doi: 10.1186/1475-9276-13-34
5. Farahani, M, Subramanian, SV, and Canning, D. The effect of changes in health sector resources on infant mortality in the short-run and the long-run: a longitudinal econometric analysis. Soc Sci Med. (2009) 68:1918–25. doi: 10.1016/j.socscimed.2009.03.023
6. Meyer, SP. Comparing spatial accessibility to conventional medicine and complementary and alternative medicine in Ontario. Canada Health Place. (2012) 18:305–14. doi: 10.1016/j.healthplace.2011.10.005
7. Marwali, EM, Kekalih, A, Yuliarto, S, Wati, DK, Rayhan, M, Valerie, IC, et al. Paediatric COVID-19 mortality: a database analysis of the impact of health resource disparity. BMJ Paediatr Open. (2022) 6:e001657. doi: 10.1136/bmjpo-2022-001657
8. Lisboa, M, Fronteira, I, Mason, PH, and Martins, MRO. National TB program shortages as potential factor for poor-quality TB care cascade: healthcare workers’ perspective from Beira, Mozambique. PLoS One. (2020) 15:e0228927. doi: 10.1371/journal.pone.0228927
9. Bartsch, SM, Ferguson, MC, McKinnell, JA, O'Shea, KJ, Wedlock, PT, Siegmund, SS, et al. The potential health care costs and resource use associated with COVID-19 in the United States. Health Aff. (2020) 39:927–35. doi: 10.1377/hlthaff.2020.00426
10. Pinho, M, and Araújo, A. How to fairly allocate scarce medical resources? Controversial preferences of healthcare professionals with different personal characteristics. Health Economics, Policy and Law, pp. 1–18. (2021).
11. Kodama, S, Uwatoko, F, and Koriyama, C. Relationship between changes in the public health nurses’ workforce and the empirical Bayes estimates of standardized mortality ratio: a longitudinal ecological study of municipalities in Japan. BMC Health Serv Res. (2023) 23:266. doi: 10.1186/s12913-023-09273-2
12. Noor, AM, Ngugi, AK, Agoi, F, Mahoney, MR, Lakhani, A, Mang’ong’o, D, et al. Utilization of health services in a resource-limited rural area in Kenya: prevalence and associated household-level factors. PLoS One. (2017) 12:728. doi: 10.1371/journal.pone.0172728
13. Kanchanachitra, C, Lindelow, M, Johnston, T, Hanvoravongchai, P, Lorenzo, FM, Huong, NL, et al. Human resources for health in Southeast Asia: shortages, distributional challenges, and international trade in health services. Lancet. (2011) 377:769–81. doi: 10.1016/S0140-6736(10)62035-1
14. Kaur, G, Prinja, S, Lakshmi, PVM, Downey, L, Sharma, D, and Teerawattananon, Y. Criteria used for priority-setting for public health resource allocation in low-and middle-income countries: a systematic review. Int J Technol Assess Health Care. (2019) 35:474–83. doi: 10.1017/S0266462319000473
15. Liu, W, Liu, Y, Twum, P, and Li, S. National equity of health resource allocation in China: data from 2009 to 2013. Int J Equity Health. (2016) 15:68. doi: 10.1186/s12939-016-0357-1
16. Wang, X, Luo, H, Qin, X, Feng, J, Gao, H, and Feng, Q. Evaluation of performance and impacts of maternal and child health hospital services using data envelopment analysis in Guangxi Zhuang autonomous region, China: a comparison study among poverty and non-poverty county level hospitals. Int J Equity Health. (2016) 15:131. doi: 10.1186/s12939-016-0420-y
17. Wang, X, Yang, H, Duan, Z, and Pan, J. Spatial accessibility of primary health care in China: a case study in Sichuan Province. Soc Sci Med. (2018) 209:14–24. doi: 10.1016/j.socscimed.2018.05.023
18. Tong, MX, Hansen, A, Hanson-Easey, S, Xiang, J, Cameron, S, Liu, Q, et al. Public health professionals’ perceptions of the capacity of China’s CDCs to address emerging and re-emerging infectious diseases. J Public Health. (2021) 43:209–16. doi: 10.1093/pubmed/fdz070
19. Li, C, Sun, M, Shen, JJ, Cochran, CR, Li, X, and Hao, M. Evaluation on the efficiencies of county-level Centers for Disease Control and Prevention in China: results from a national survey. Trop Med Int Health. (2016) 21:1106–14. doi: 10.1111/tmi.12753
20. China SCotCPo. Outline of the "healthy China 2030" plan 2016. (2016). Available at: https://www.gov.cn/zhengce/2016-10/25/content_5124174.htm.
21. Luo Li, WY, and Tiantian, Z. Thoughts on the construction of disease prevention and control system in the new era. Chin Health Resources. (2020) 23:7–13. doi: 10.13688/j.cnki.chr.2020.19226
22. Wei, X. Measures to shore up disease control and prevention capacities: CHINADAILY. (2024). Available at: https://www.chinadaily.com.cn/a/202401/03/WS65949e1ca3105f21a507a372.html.
23. Li, YQ, Chen, H, and Guo, HY. Examining inequality in the public health workforce distribution in the Centers for Disease Control and Prevention (CDCs) system in China, 2008-2017. Biomed Environ Sci. (2020) 33:374–83. doi: 10.3967/bes2020.051
24. Chen Cheng, ZN, and Ting, C. Spatial analysis of the staffing level of Centers for Disease Control and Prevention in China. Chin J Health Policy. (2021) 14:58–65. doi: 10.3969/j.issn.1674-2982.2021.06.009
25. Li Liping, ZX, Zhao Yulan, L, and Zuxun, Z. Degree of coordination between primary care resource allocation and economic development in eastern, central and Western China. Chin. Gen Pract. (2021) 24:2777–84. doi: 10.12114/j.issn.1007-9572.2021.00.234
26. Love-Koh, J, Griffin, S, Kataika, E, Revill, P, Sibandze, S, and Walker, S. Methods to promote equity in health resource allocation in low-and middle-income countries: an overview. Glob Health. (2020) 16:6. doi: 10.1186/s12992-019-0537-z
27. Ao, Y, Feng, Q, Zhou, Z, Chen, Y, and Wang, T. Resource allocation equity in the China’s rural three-tier healthcare system. Int J Environ Res Public Health. (2022) 19:589. doi: 10.3390/ijerph19116589
28. Sun, J. Equality in the distribution of health material and human resources in Guangxi: evidence from southern China. BMC Res Notes. (2017) 10:2760. doi: 10.1186/s13104-017-2760-0
29. Lu, L, and Zeng, J. Inequalities in the geographic distribution of hospital beds and doctors in traditional Chinese medicine from 2004 to 2014. Int J Equity Health. (2018) 17:165. doi: 10.1186/s12939-018-0882-1
30. Yan, K, Chun-Li, C, Jia-Ni, WU, Yi-Feng, Z, Shi-Zhu, LI, and Xiao-Nong, Z. General status and research progress of human resource in the institutions of disease control and prevention in China. Chin. J. Public Health Manag. (2017) 33:334–8. doi: 10.19568/j.cnki.23-1318.2017.03.014
31. Yao Min, MH, Lidong, G, Fuqiang, L, Qun, Z, and Juan, Z. Analysis of the status of health human resources allocation in Hunan Province's disease control institutions. Pract Prev Med. (2020) 27:11. doi: 10.3969/j.issn.1006-3110.2020.09.033
32. Qiongwenqian, W, Lieyu, H, Yang, L, and Yan, G. Current situation and suggestions on the construction of talent team in the institutions of disease control and prevention in China. Chinese journal of public health. Management. (2021) 37:165–8. doi: 10.19568/j.cnki.23-1318.2021.02.0007
33. Shan Ying, LG, and Shixue, L. A temporal and spatial analysis of the staffing allocation level of China's centers for disease and prevention from 2009 to 2019. Chinese. Health Econ. (2022) 41:46–50. doi: 10.7664/j.issn.1003-0743.2022.3.zgwsjj202203011
34. Chai, K-C, Zhang, Y-B, and Chang, K-C. Regional disparity of medical resources and its effect on mortality rates in China. Front Public Health. (2020) 8:8. doi: 10.3389/fpubh.2020.00008
35. Panopoulou, E, and Pantelidis, T. Convergence in per capita health expenditures and health outcomes in the OECD countries. Appl Econ. (2012) 44:3909–20. doi: 10.1080/00036846.2011.583222
36. Railaite, R, and Čiutienė, R. The impact of public health expenditure on health component of human capital. Eng Econ. (2020) 31:371–9. doi: 10.5755/j01.ee.31.3.25158
37. Bhattacharjee, A, Maiti, T, and Petrie, D. General equilibrium effects of spatial structure: health outcomes and health behaviours in Scotland. Reg Sci Urban Econ. (2014) 49:286–97. doi: 10.1016/j.regsciurbeco.2014.10.003
38. Wu, X, Mao, R, and Guo, X. Equilibrium of tiered healthcare resources during the COVID-19 pandemic in China: a case study of Taiyuan, Shanxi Province. Int J Environ Res Public Health. (2022) 19:35. doi: 10.3390/ijerph19127035
39. Haixiang, L. Research on the health risk of public governance in the process of urbanization - based on spatial vision. J Shanghai Univ Int Bus Econ. (2020) 27:102–11. doi: 10.16060/j.cnki.issn2095-8072.2020.03.009
40. Zou, Y, Jia, L, Chen, S, Deng, X, Chen, Z, He, Y, et al. Spatial accessibility of emergency medical services in Chongqing, Southwest China. Front Public Health. (2023) 10:10. doi: 10.3389/fpubh.2022.959314
41. Liu, T, Li, J, Chen, J, and Yang, S. Regional differences and influencing factors of allocation efficiency of rural public health resources in China. Healthcare. (2020) 8:270. doi: 10.3390/healthcare8030270
42. Carvalho Rde, S, Diniz, AS, Lacerda, FM, and Mello, PA. Gross domestic product (GDP) per capita and geographical distribution of ophthalmologists in Brazil. Arq Bras Oftalmol. (2012) 75:407–11. doi: 10.1590/S0004-27492012000600007
43. Song, X, Wei, Y, Deng, W, Zhang, S, Zhou, P, Liu, Y, et al. Spatio-temporal distribution, spillover effects and influences of China’s two levels of public healthcare resources. Int J Environ Res Public Health. (2019) 16:582. doi: 10.3390/ijerph16040582
44. Tang, B, and Li, Z. A country-level empirical study on the fiscal effect of elderly population health: the mediating role of healthcare resources. Healthcare. (2021) 10:30. doi: 10.3390/healthcare10010030
45. Pammolli, F, Riccaboni, M, and Magazzini, L. The sustainability of European health care systems: beyond income and aging. Eur J Health Econ. (2011) 13:623–34. doi: 10.1007/s10198-011-0337-8
46. Bloom, DE, and Cadarette, D. Infectious disease threats in the twenty-first century: strengthening the global response. Front Immunol. (2019) 10:549. doi: 10.3389/fimmu.2019.00549
47. Guida, C, and Carpentieri, G. Quality of life in the urban environment and primary health services for the elderly during the Covid-19 pandemic: an application to the city of Milan (Italy). Cities. (2021) 110:103038. doi: 10.1016/j.cities.2020.103038
48. Li, Z, Hung, P, He, R, and Zhang, L. Association between direct government subsidies and service scope of primary care facilities: a cross-sectional study in China. Int J Equity Health. (2020) 19:135. doi: 10.1186/s12939-020-01248-7
49. Smiley, MJ, Diez Roux, AV, Brines, SJ, Brown, DG, Evenson, KR, and Rodriguez, DA. A spatial analysis of health-related resources in three diverse metropolitan areas. Health Place. (2010) 16:885–92. doi: 10.1016/j.healthplace.2010.04.014
50. Chen, C, Chen, T, Zhao, N, and Dong, S. Regional maldistribution of human resources of rehabilitation institutions in China mainland based on spatial analysis. Front Public Health. (2022) 10:1028235. doi: 10.3389/fpubh.2022.1028235
51. Chen, B, and Jin, F. Spatial distribution, regional differences, and dynamic evolution of the medical and health services supply in China. Front Public Health. (2022) 10:10. doi: 10.3389/fpubh.2022.1020402
52. Zhu, B, Fu, Y, Liu, J, He, R, Zhang, N, and Mao, Y. Detecting the priority areas for health workforce allocation with LISA functions: an empirical analysis for China. BMC Health Serv Res. (2018) 18:957. doi: 10.1186/s12913-018-3737-y
53. Chen, J, Lin, Z, Li, L-a, Li, J, Wang, Y, Pan, Y, et al. Ten years of China’s new healthcare reform: a longitudinal study on changes in health resources. BMC Public Health. (2021) 21:2272. doi: 10.1186/s12889-021-12248-9
54. Anselin, L. Local indicators of spatial association-LISA. Geogr Anal. (2010) 27:93–115. doi: 10.1111/j.1538-4632.1995.tb00338.x
55. Zhu, B, Hsieh, C-W, and Mao, Y. Addressing the licensed doctor maldistribution in China: a demand-and-supply perspective. Int J Environ Res Public Health. (2019) 16:1753. doi: 10.3390/ijerph16101753
56. Institute SIoCMR. Suggestions on accelerating the allocation of public health resources (2020). Available at: https://www.ndrc.gov.cn/xxgk/jd/wsdwhfz/202012/t20201218_1253062.html.
57. China GOotSCo. National health service system planning outline. (2015). Available at: https://www.gov.cn/zhengce/content/2015-03/30/content_9560.htm.
58. Zhu, B, Hsieh, C-W, and Zhang, Y. Incorporating spatial statistics into examining equity in health workforce distribution: an empirical analysis in the Chinese context. Int J Environ Res Public Health. (2018) 15:1309. doi: 10.3390/ijerph15071309
59. CPC Central Committee SC. Guiding opinions on promoting the development of the western region to form a new pattern in the new era 2020. Available at: http://www.xinhuanet.com/politics/zywj/2020-05/17/c_1125996720.htm.
60. Liu, S, Gu, Y, Yang, Y, Schroeder, E, and Chen, Y. Tackling brain drain at Chinese CDCs: understanding job preferences of public health doctoral students using a discrete choice experiment survey. Hum Resour Health. (2022) 20:46. doi: 10.1186/s12960-022-00743-y
61. Bai, Q, Ke, X, Huang, L, Liu, L, Xue, D, and Bian, Y. Finding flaws in the spatial distribution of health workforce and its influential factors: an empirical analysis based on Chinese provincial panel data, 2010–2019. Front Public Health. (2022) 10:10. doi: 10.3389/fpubh.2022.953695
62. Dong, E, Sun, X, Xu, T, Zhang, S, Wang, T, Zhang, L, et al. Measuring the inequalities in healthcare resource in facility and workforce: a longitudinal study in China. Front Public Health. (2023) 11:11. doi: 10.3389/fpubh.2023.1074417
63. Kilmarx, PH, Long, T, and Reid, MJA. A National Public Health Workforce to control COVID-19 and address health disparities in the United States. Open Forum Infect Dis. (2021) 8:304. doi: 10.1093/ofid/ofab304
64. Cristea, M, Noja, GG, Stefea, P, and Sala, AL. The impact of population aging and public health support on EU labor markets. Int J Environ Res Public Health. (2020) 17:439. doi: 10.3390/ijerph17041439
65. Yang, Y, Zhang, L, Zhang, X, Yang, M, and Zou, W. Efficiency measurement and spatial spillover effect of provincial health systems in China: based on the two-stage network DEA model. Front Public Health. (2022) 10:952975. doi: 10.3389/fpubh.2022.952975
66. Jakovljevic, M, Timofeyev, Y, Ranabhat, CL, Fernandes, PO, Teixeira, JP, Rancic, N, et al. Real GDP growth rates and healthcare spending – comparison between the G7 and the EM7 countries. Glob Health. (2020) 16:64. doi: 10.1186/s12992-020-00590-3
67. Sellers, K, Leider, JP, Gould, E, Castrucci, BC, Beck, A, Bogaert, K, et al. The state of the US governmental public health workforce, 2014–2017. Am J Public Health. (2019) 109:674–80. doi: 10.2105/AJPH.2019.305011
68. Wang, L, and Chen, Y. Determinants of China’s health expenditure growth: based on Baumol’s cost disease theory. Int J Equity Health. (2021) 20:213. doi: 10.1186/s12939-021-01550-y
69. Ravangard, R, Hatam, N, Teimourizad, A, and Jafari, A. Factors affecting the technical efficiency of health systems: a case study of economic cooperation organization (ECO) countries (2004–10). Int J Health Policy Manag. (2014) 3:63–9. doi: 10.15171/ijhpm.2014.60
Keywords: healthcare resources, spatial autocorrelation, spatial econometric model, influential factors, China
Citation: Yu Y, Lu J, Dou X, Yi Y and Zhou L (2024) Spatial distribution and influencing factors of CDC health resources in China: a study based on panel data from 2016–2021. Front. Public Health. 12:1331522. doi: 10.3389/fpubh.2024.1331522
Received: 01 November 2023; Accepted: 19 April 2024;
Published: 01 May 2024.
Edited by:
Fei Fan, Wuhan University, ChinaReviewed by:
Serap Taşkaya, Osmaniye Korkut Ata University, TürkiyeCopyright © 2024 Yu, Lu, Dou, Yi and Zhou. This is an open-access article distributed under the terms of the Creative Commons Attribution License (CC BY). The use, distribution or reproduction in other forums is permitted, provided the original author(s) and the copyright owner(s) are credited and that the original publication in this journal is cited, in accordance with accepted academic practice. No use, distribution or reproduction is permitted which does not comply with these terms.
*Correspondence: Ling Zhou, emhvdWxpbmcwNjA5QDE2My5jb20=
Disclaimer: All claims expressed in this article are solely those of the authors and do not necessarily represent those of their affiliated organizations, or those of the publisher, the editors and the reviewers. Any product that may be evaluated in this article or claim that may be made by its manufacturer is not guaranteed or endorsed by the publisher.
Research integrity at Frontiers
Learn more about the work of our research integrity team to safeguard the quality of each article we publish.