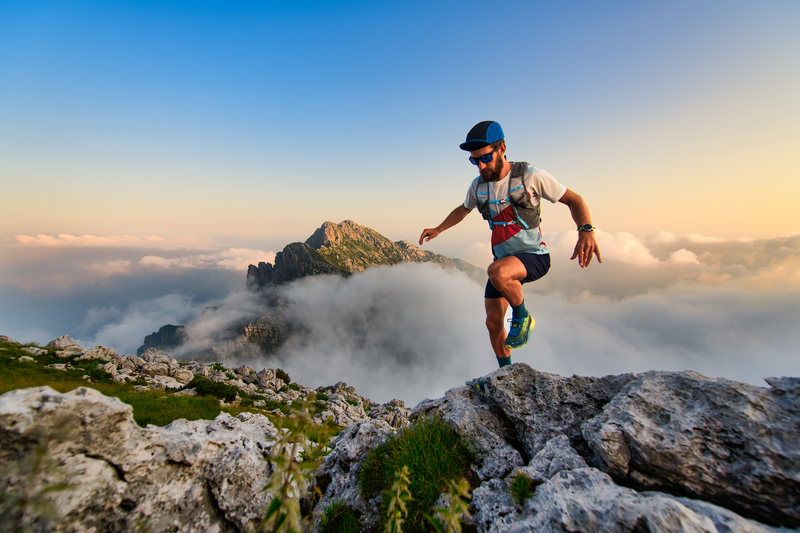
94% of researchers rate our articles as excellent or good
Learn more about the work of our research integrity team to safeguard the quality of each article we publish.
Find out more
ORIGINAL RESEARCH article
Front. Public Health , 17 May 2024
Sec. Health Economics
Volume 12 - 2024 | https://doi.org/10.3389/fpubh.2024.1305458
This article is part of the Research Topic Macroeconomic Impact of Disease Dynamics: A Temporal-Spatial Analysis View all 6 articles
Background: Healthcare service utilization is unequal among different subpopulations in low-income countries. For healthcare access and utilization of healthcare services with partial or full support, households are recommended to be enrolled in a community-based health insurance system (CBHIS). However, many households in low-income countries incur catastrophic health expenditure. This study aimed to assess the spatial distribution and factors associated with households' enrollment level in CBHIS in Ethiopia.
Methods: A cross-sectional study design with two-stage sampling techniques was used. The 2019 Ethiopian Mini Demographic and Health Survey (EMDHS) data were used. STATA 15 software and Microsoft Office Excel were used for data management. ArcMap 10.7 and SaTScan 9.5 software were used for geographically weighted regression analysis and mapping the results. A multilevel fixed-effect regression was used to assess the association of variables. A variable with a p < 0.05 was considered significant with a 95% confidence interval.
Results: Nearly three out of 10 (28.6%) households were enrolled in a CBHIS. The spatial distribution of households' enrollment in the health insurance system was not random, and households in the Amhara and Tigray regions had good enrollment in community-based health insurance. A total of 126 significant clusters were detected, and households in the primary clusters were more likely to be enrolled in CBHIS. Primary education (AOR: 1.21, 95% CI: 1.05, 1.31), age of the head of the household >35 years (AOR: 2.47, 95% CI: 2.04, 3.02), poor wealth status (AOR: 0.31, 95% CI: 0.21, 1.31), media exposure (AOR: 1.35, 95% CI: 1.02, 2.27), and residing in Afar (AOR: 0.01, 95% CI: 0.003, 0.03), Gambela (AOR: 0.03, 95% CI: 0.01, 0.08), Harari (AOR: 0.06, 95% CI: 0.02, 0.18), and Dire Dawa (AOR: 0.02, 95% CI: 0.01, 0.06) regions were significant factors for households' enrollment in CBHIS. The secondary education status of household heads, poor wealth status, and media exposure had stationary significant positive and negative effects on the enrollment of households in CBHIS across the geographical areas of the country.
Conclusion: The majority of households did not enroll in the CBHIS. Effective CBHIS frameworks and packages are required to improve the households' enrollment level. Financial support and subsidizing the premiums are also critical to enhancing households' enrollment in CBHIS.
Many countries face significant challenges in financing healthcare (1, 2). Health services are unavailable and unaffordable for households of poor wealth status (3, 4), leading to unequal access to healthcare across different subpopulations (5). The demands of medical care expenditure and utilization are different between inpatients and outpatients (6). Poor quality, financial constraints, absence of pre-payment financial arrangements (7, 8), and direct user-fee charges (9) were major factors for the low utilization of healthcare services. Globally, 50% of the world's population cannot access needed health services, while 100 million people are pushed into extreme poverty each year because of health expenses (10). Every year, 150 million people suffer from financial catastrophes (11). Thus, the access to and utilization of healthcare services among households is low (12, 13).
In low- and middle-income countries, over 40% of total health expenditure is done through out-of-pocket payments (OPP), which has resulted in a scarcity of funds to be spent on health (14). It has been reported that 5.5%, 15%, and 2.6% of households in Mongolia (15), Burkina Faso (16), and Uganda (17) suffer from catastrophic health expenditure, respectively. Up to 90% of healthcare expenditure catastrophes occurred in low-income countries (18, 19), and OPP accounts for 37% of the total spending on healthcare (20).
Social health insurance (SHI) is a scheme that offers an opportunity for healthcare finance by raising and pooling funds (21) to provide affordable, cost-effective, and equitable healthcare services (22–24). Community-based health insurance schemes (CBHIS) are one of the mechanisms for raising funds for access to healthcare services (25). A CBHIS is a type of insurance in which households or household members register and pay a premium to the health insurance system. The head of the households or one of the members is registered in the proposed CBHIS regardless of the amount paid for partial or full health insurance coverage (41). Thus, the medical and surgical expenses would be covered by the health insurance system, which allows the members of the household to access health services without any financial hardship (10).
CBHIS has been advocated by the World Health Organization (WHO) to achieve universal healthcare coverage and ensure access to healthcare services (26). CBHIS is a primary agenda for health reform in many countries for universal health coverage (27, 28), and it guarantees individuals' access to healthcare services (29, 30). Moreover, CBHIS is designed for the agricultural and other informal sectors to enhance productivity and provide food security (31). The enrollment process in health insurance schemes is voluntary, and it is a complementary or alternative source of healthcare finance (32). Sometimes, people may be forced to pay CBHIS premium based on the principle of social solidarity (23).
Despite health insurance being focused on whole populations, it is used more by older adult people due to low incomes and the absence of social security (6). Hence, CBHIS has not reached the level of universal coverage (5). Moreover, health service utilization is different among people in different health insurance schemes (33) and CBHIS is ineffective in many countries (34). For instance, 45% of people are not covered by CBHIIS in China (35).
In Ethiopia, according to the 2016 Ethiopian Demographic and Health Survey (EDHS), health insurance coverage is shallow (10); more than 94% of households are not covered by health insurance (36). Primary studies in Ethiopia show that only 12.8% of the households are enrolled in CBHIS in Sidama (37) and 33.30% of the households in south Omo (38), while 77.9% of the population complied with CBHIS requirements in southeast Ethiopia (39). Educational status of the household, monthly income, sex (25), media exposure, age, occupation, wealth status, size of family members (10), knowledge and attitudes toward CBHIS, and trust in CBHIS management (37) are factors for the enrollment of households in CBHIS.
A literature search shows that studies on the assessment of household enrollment in CBHIS are scarce. The enrollment of households in the cold areas (low) in CBHIS has not been identified in Ethiopia. If geographical weighted regression analysis is employed for insufficient empirical evidence, policymakers and stakeholders cannot decide who gets benefits from the system and who is left behind. Hence, the policymakers require potential evidence as a source for future enhancement of CBHIS coverage and to take proper action. Identifying geographical variations of households' enrollment in CBHIS is very important to prioritize and design a framework and install packages in the CBHIS programs in the specific target location. Therefore, this study aimed to explore the spatial distribution and factors associated with households' enrollment level in CBHIS.
A community-based cross-sectional study design was conducted across the nine regions of Ethiopia. Ethiopia is located in the Horn of Africa and bordered by Eritrea to the north, Djibouti and Somalia to the east, Sudan and South Sudan to the west, and Kenya to the south. Ethiopia comprises nine regional states with two administrative cities (10).
The 2019 Ethiopian Mini Demographic and Health Survey (EMDHS) dataset was used from the Measure Demographic and Health Survey (DHS) program website (https://dhsprogram.com). The survey was conducted by the Ethiopian Public Health Institute (EPHI) in collaboration with the Central Statistical Agency (CSA). According to the EMDHS report, the survey was conducted from 21 March to 28 June 2019. Shapefiles were downloaded from https://africaopendata.org.
A two-stage stratified cluster sampling was used. Each region was stratified into urban and rural areas. In the selected enumeration areas, a household listing operation was done, and the results were used as a sampling frame for household selection in the second stage. Finally, a fixed number of households per cluster were selected. Samples from enumeration areas were selected independently in each stratum through implicit stratification and equal proportional allocation. The details about the methodology are found from the 2019 EMDHS reports (40).
Sociodemographic characteristics of households, such as wealth status, and household-related variables such as age, sex, educational status, and household media exposures were considered individual-level variables, whereas the place of residence and region were used as community-level variables.
Households' enrollment level in the CBHIS is dependent variable.
Households' enrollment level in CBHIS : The households were considered as enrolled in the CBHIS if they had registered for partial or full health service cost waiver under the proposed CBHIS, and these households were coded as “1”. Otherwise, households that had not enrolled in CBHIS were coded as “0” (41).
Media exposure: If the households had either radio or television or both, then we considered that they are exposed to media, and if they did not have either of them, then we considered that they are unexposed to media (42).
The STATA version 15 software and Microsoft Office Excel were used for data management. ArcMap version 10.7 software was used for spatial autocorrelation detection and interpolations of households' enrollment levels in CBHIS in Ethiopia.
Global spatial autocorrelation: The global spatial autocorrelation (Global Moran's I) statistic measure was used to assess whether the households' enrollment level in CBHIS was dispersed, clustered, or randomly distributed in Ethiopia (43). Moran's I values close to −1, close to +1, and zero (0), respectively, indicate a dispersed, clustered pattern and random distribution (44, 45) of households' enrollment level in CBHIS. The Z-scores and p-values were used to determine the hot and cold areas.
Spatial interpolation: Unsampled areas were predicted by the spatial interpolation of the households' enrollment level in CBHIS based on sampled EAs. For the prediction of unsampled EAs, we used the radial basis function interpolation technique.
Spatial scan statistics: SaTScan version 9.5 software was used for local cluster detection (46). We employed purely spatial Bernoulli-based model scan statistics to determine the geographical locations of significant clusters with high rates of household enrollment level in CBHIS (47). Those households that were enrolled in CBHIS were treated as cases, and those that had not enrolled were taken as controls to fit the Bernoulli model for the scanning window that moves across the study area. The default size of < 50% of the population was used as an upper limit, allowing both small and large clusters to be detected and ignoring clusters that contained more than the maximum limit because of the circular shape of the window. The log-likelihood ratio (LLR) was used to determine whether the number of observed cases within the potential cluster was significantly higher than expected. The circle with the maximum LLR was defined as the most likely cluster, and it was then compared with the overall distribution of maximum values. The significant clusters were assigned p-values and ranked based on their LLR value based on the 9999 Monte Carlo replications (48).
Both the ordinary least squares (OLS) model and the geographical weighted regression (GWR) model were considered for model fitness comparison. The extracted predictor variables were fitted into the two models. Adjusted R2 and Akaike's information criterion (AIC) were used to compare and determine the best-fit model for local parameter estimation. Variance inflation factors (VIF) were used for multicollinearity checking by the same dependent and explanatory variables in the OLS model. Variables with VIF values of > 0.7S were considered redundant.
A GWR analysis model was used to determine the aggregate effects of each explanatory variable for households' enrollment in the CBHIS. A GWR model was used for the estimation of the local parameter to reflect changes that occur over space in the spatial association between a dependent variable and explanatory variables, as well as for relaxing the geographical independence of explanatory variables (44, 49). Therefore, the following GWR model linear assumption of mathematical equations (model structure) was written. Wi = β (Xi, Yi)+ZHα(Xi, Yi)Zi+i, where Wi is the response variable, (Xi, Yi) are the geographical coordinates of point i, β is the intercept at the (Xi, Yi) coordinate, α e coefficient of the covariate Z at the (Xi, Yi) coordinate, and ϵi is the random error term. Finally, the explanatory variables with a p < 0.05 were considered as stationary significant factors for households' enrollment level in CBHIS.
Since the nature of the EMDHS dataset was hierarchical, the records within the cluster might be correlated, which disturbs the assumption of independence. A biased statistical report might be generated by fitting a model with correlated data. Therefore, multilevel mixed-effect logistic regression analysis was assumed to be have been used to generate and report good results. To assess the correlation between the clusters, four models have been set: model A (a null model that assesses the households' enrollment level in CBHIS within the cluster); model B (contains individual-level variables); model C (contains community-level variables); and model D (the aggregate model of models B and C). The intraclass correlation coefficient (ICC) was calculated to check the correlation within the cluster. If the ICC value is >0.25, the data are fitted for a multilevel fixed-effect logistic regression model (50, 51). An LLR was used for model comparison, and the model with the highest value was taken as the best-fit model to solve the correlation within the cluster (52). In the multilevel fixed-effect logistic regression analysis, a p < 0.05 and 95% CIs were used to assess the strength of the association between independent and outcome variables.
A total of 40,659 weighted sampled households were used in this study. Four out of 10 (40.5%) and one-fifth (20.1%) of the households were from the Oromia region and South Nation Nationality and People of the Region (SNNPR), respectively. The majority (72.9%) of the households were from rural areas of the country. Of the sample, 46.9% of household heads were not educated, and only four out of 10 (42.1%) of the household heads had completed primary education. Four out of 10 (40.3%) households were in the rich wealth index status. The majority (76.2%) of household heads were more than 34 years of age, and 82.3% of household heads were men. More than seven out of 10 (70%) of households had no television (TV) or radio (Table 1).
As stated in Figure 1, 28.6% (95% CI: 28.16–29.04%) of the households in Ethiopia had good levels of enrollment in CBHIS. The spatial distribution of households' enrollment level in CBHIS was not random in Ethiopia (Global Moran's I = 0.256697, p < 0.00001). The spatial autocorrelation report indicated that the spatial distribution of households' enrollment in CBHIS was significantly clustered across the regions of Ethiopia (Figure 2).
A good level of households' enrollment in CBHIS was observed in the regional states of Ethiopia such as Amhara, Tigray, SNNPR, and Oromia, whereas households' enrollment levels in CBHIS in the Benishangul Gumuz, Harari, Afar, and Dire Dawa regions were low (Figures 3, 4).
The radial basis function interpolation method was employed to predict the households' enrollment level in CBHIS. The interpolation result indicated that the risk of households' enrollment in CBHIS was less likely to occur in the Amhara, Tigray, SNNPR, and Oromia regions, whereas high risk of households' enrollment in CBHIS was more likely observed in Benishangul Gumuz, Gambela, Somalia, Afar, Harari, and Dire Dawa regions (Figure 5).
A total of 126 significant clusters for households' enrollment levels in CBHIS were detected. Of these, 65, 15, and 19 clusters were primary, secondary, and tertiary clusters, respectively. The primary clusters were located at 12.322718 N, 37.959425 E within a 265.25 km radius in the north and northwest parts of Ethiopia. The secondary clusters were located at 11.072967 N, 38.884163 E within a 135.21 km radius in the southern part of the Amhara regional state of the country. Households in the primary, secondary, and tertiary clusters were 3.58, 4.24, and 2.80 times more likely to have good levels of enrollment in CBHIS than households outside the clusters (Table 2, Figure 6).
Table 2. Significant spatial scan statistics clusters for households' enrollment levels in CBHIS, 2019 EMDHS dataset.
As stated above, ICC and LLR were used for multilevel fixed-effect analysis. A comparison revealed that 68.7% of the ICC values in Model D confirmed that there was a significant correlation among respondents within the cluster on households' enrollment level in CBHIS. Additionally, model D was the best-fit model as its LLR score was higher (−12941.701) than other models shown in Table 3. Therefore, a multilevel fixed-effect logistic regression analysis model was employed to determine the correlations within the cluster among respondents based on their enrollment in CBHIS. In the multilevel fixed-effect logistic regression analysis, educational status, age of the household head, wealth status, media exposure, and regions were statistically associated with households' enrollment in CBHIS.
Table 3. Multilevel fixed-effect logistic regression analysis of factors associated with households' enrollment in CBHIS in Ethiopia.
Household heads older than 35 years were 2.5 (AOR: 2.47, 95% CI: 1.04, 3.02) times more likely to be enrolled in CBHIS than household heads younger than 35 years. Households that had media exposure were 1.4 (AOR: 1.35, 95% CI: 1.02, 2.27) times more likely to be enrolled in CBHIS than their counterparts. Household heads with secondary education were 1.3 (AOR: 1.25, 95% CI: 1.89, 2.44) times more likely to enroll in CBHIS than household heads with no education. Households under the poor wealth index were 69% (AOR: 0.31, 95% CI: 0.21, 0.81) less likely to enroll in CBHIS than rich households. Households in Afar, Gambela, Harari, and Dire Dawa regions were 99% (AOR: 0.01, 95% CI: 0.003, 0.03), 97% (AOR: 0.03, 95% CI: 0.01, 0.08), 94% (AOR: 0.06, 95% CI: 0.02, 0.18), and 98% (AOR: 0.02, 95% CI: 0.01, 0.06), respectively, less likely to enroll in CBHIS (Table 3).
As shown in Table 4, the GWR model was the best-fit model as compared with the OLS model, with a low AIC (3053.37) as compared with the AIC value of 3162.63 in the OLS model. Additionally, the GWR model was best explained by the predictor variables with an adjusted R2 value of 66.72% as compared with the adjusted R2 value of 37.44% in the OLS model. The variables that had multicollinearity (redundancy) in the GWR model were removed from the GWR model (Supplementary material).
In the geographically weighted regression model, explanatory variables such as secondary education status, poor wealth status, and household media exposure were statistically associated with households' enrollment in CBHIS. The significant explanatory variables have a positive and negative effect on the households' enrollment level in CBHIS across the region of Ethiopia. In addition, the strength of the association between heterogeneity predictors and outcome of interest varies geographically.
As shown in Figure 7, the secondary education status of household heads exhibited different levels of statistical significance in different parts of the country for the enrollment of households in CBHIS. The coefficients of secondary education vary geographically between −0.347 and 0.193 significantly. Keeping all the other predictors constant, the enrollment level of households in CBHIS significantly increased from 34.7% (−0.347) to 80.4% (0.196), with an increase in the number of household heads with secondary education.
Figure 7. Geographically weighted regression model of households' enrollment level in CBHIS with secondary education status.
As shown in Figure 8, the poor wealth status of the households exhibited different levels statistical significance in different parts of the country for the enrollment of households in CBHIS. The coefficients of poor wealth status in households vary geographically between 0.056 and 0.452. Keeping all the predictors constant, the households' enrollment level in CBHIS significantly decreased from 45.2% (0.452) to 5.6% (0.056) as their wealth status decreased by one unit.
Figure 8. Geographically weighted regression model of households' enrollment level in CBHIS with poor wealth status.
As shown in Figure 9, the households' media exposure had different statistical significance effects in different parts of the country for the households' enrollment in CBHIS. The coefficients of households' media exposure vary geographically and significantly between 0.033 and −0.354. This finding indicated that the media exposure had significant negative and positive effects on households' enrollment level in CBIHS. Keeping all the predictors as constant, as households become more exposed to media, the risk of enrollment level of households in CBHIS decreased from 3.3% (0.033) to 35.4% (−0.354). Except for the vertical intercept of Tigray, Amhara, Oromia, and SNNPR regions, media exposure was not a geographically significant factor in other parts of the country.
Figure 9. Geographically weighted regression model of households' enrollment level in CBHIS with households' media exposure.
In this study, 28.6% of households had a good level of enrollment in CBHIS in Ethiopia. The finding was in line with studies conducted in Nigeria (15.5%) (53), Kenya, where 93% of women have no access to any type of health insurance (54), and Ethiopia (12.8%) (37). However, the evidence indicated lower level of enrollment than the studies conducted in Ethiopia (45%) (55) and Uganda (44%) (56). This difference might be due to household members' compliance with the CBHIS requirements (39), household members' unwillingness to renew their membership (57), and households' inadequate perception of CBHIS (58). In addition, poor government financial support, the high dropout rate from CBHIS (59), lack of clear legislative and regulatory frameworks (54), and an unrealistic enrollment requirement (60) might be possible reasons for the low household enrollment level in CBHIS.
The spatial distribution of households' enrollment level in CBHIS was not random, and the jeopardy of households' enrollment in CBHIS was less likely to occur in Amhara, Tigray, SNNPR, and Oromia regions, whereas high jeopardy of households' enrollment level in CBHIS was more likely observed in Benishangul Gumuz, Gambela, Somalia, Afar, Harari, and Dire Dawa regions. Households in the primary, secondary, and tertiary clusters were more likely to be enrolled in CBHIS than households outside of these clusters. This evidence was strongly supported by a similar spatial study done in Ethiopia (10). This disparity might be due to the difference in access to tertiary-level care and premium payment methods across regions (61).
In the multilevel fixed-effect logistic regression analysis, secondary education status, age of the household head ≥ 35 years, poor wealth status, media exposure, and regions were significantly associated with households' enrollment level in CBHIS. Additionally, geographical heterogeneity factors were assessed according to the GWR analysis report. Thus, predictors such as secondary education status, poor wealth status, and media exposure had significant positive and negative effects on households' enrollment in CBHIS across various geographical areas. The strength of the association between the predictors and outcome of interest was locally independent and varied geographically across the regions of Ethiopia.
The secondary education status contributed to 30% of households' enrollment level in CBHIS. Additionally, the secondary education level status of the household head had the impact of decreasing the jeopardy of households' enrollment level in CBHIS by 45.7%. Additionally, the enrollment level of households in CBHIS significantly increased from 34.7% to 80.4% as the household head's secondary education status increased. This finding indicated that the secondary education of household heads had both negative and positive impacts on the enrollment level of households in CBHIS across the regions of Ethiopia. Moreover, secondary educational status of the household heads was not a geographically significant factor in most parts of Somali, Gambela, SNNPR, Tigray, and southwest parts of Oromia regions. The evidence was in line with studies conducted in Ethiopia (37, 62), Senegal (63), and Asia (64). This evidence might be due to the fact that educated people understand the principles and benefits of CBHIS easily (65) and have more knowledge about the advantages of health insurance. In addition, educated households might be more concerned about their health and insure themselves against unexpected out-of-pocket payments (10).
Having poor wealth status reduces the households' level of enrollment in CBHIS by 69%. Furthermore, the households' enrollment level in CBHIS significantly decreased from 45.2% to 5.6% as their wealth status decreased by one unit. This finding indicates that the poor wealth status of households had different levels of statistical significance in different parts of the country for the enrollment of households in CBHIS. In most parts of the Somali region, poor wealth status was not geographically significantly associated with household enrollment in CBHIS. This finding was in line with studies conducted in Ethiopia (10, 62), Burkina Faso (66), and Ghana (67). This similarity might be because the payment of premium may not be affordable for poor households (68), and high premiums might deter poor households from renewing their membership (69). Moreover, financial constraints and lack of money might be major constraints for households' enrollment in CBHIS (14) in addition to the absence of subsidies in place to cover the premiums (70).
Households exposed to media were 1.4 times more likely to be enrolled in CBHIS. Furthermore, as households become more exposed to media, the risk of enrollment level of households in CBHIS decreased from 3.3% (0.033) to 35.4% (−0.354). This indicated that media exposure had significant negative and positive effects on the enrollment level of households in CBIHS across various geographical areas. The household's media exposure had a significant geographical impact on households' enrollment levels in CBHIS and varied geographically. As households had more media exposure, the households' enrollment level in CBHIS increased by 9.6%. This finding was supported by studies done in Ethiopia (10, 71), Nigeria (72), Uganda (56), and Kenya (54). Since household media exposure has a direct implication for households' access to information about CBHIS, those households that access information about CBHIS directly correlate with good awareness of CBHIS enrollment (57). This correlation might be due to the media creating awareness among communities regarding the principles and implementation of CBHIS (73). Households with good awareness of CBHIS might make informed choices and engage themselves in different knowledge enhancement activities through reading materials (10); individuals with better information may ask for details of the services and get a better understanding of the advantages of CBHIS that drive them to be enrolled (62). Moreover, the health insurance advertisements by health extension workers during their health facility visits also contribute to information about CBHIS (73).
Household heads aged older than 35 years were 2.5 times more likely to be enrolled in CBHIS than household heads who are between 15 and 24 years of age. This evidence was supported by various studies (39, 74, 75). This difference in enrollment might be because older people might be less compliant with CBHIS requirements (39), and they might be less likely to pay membership premiums for shared health insurance than younger people (53).
Households in Afar, Gambela, Harari, and Dire Dawa regions were, respectively, 99%, 97%, 94%, and 98% less likely to be enrolled in CBHIS. This finding was supported by a study that stated that geographical location is a factor in inequality in access to CBHIS (76). Household members in these regions might be less willing to enroll in the CBHIS (77), and health insurance workers and the government in those regions might be less perceived as consistent with insurance activity. Additionally, household members in these regions might have access to other subsidy packages (61).
Households' enrollment level in the CBHIS is inadequate. Households in Benishangul Gumuz, Gambela, Somalia, Afar, Harari, and Dire Dawa had a high jeopardy of enrollment in CBHIS. Educational status, age of the household heads, wealth status, media exposure, and regions were statistically significant factors for households' enrollment in CBHIS. The secondary education status of household heads, poor wealth status, and media exposure had positive and negative geographical effects on the households' enrollment in CBHIS.
Priority attention needs to be paid to the enrollment of households in cold areas in CBHIS. Enhancing the educational status of household members, providing financial support, and premium subsidies would enhance households' enrollment levels in CBHIS. It is recommended that health policymakers and implementers propose good CBHIS implementation frameworks and packages. Quantitative and qualitative research is needed considering contextual, technological, behavioral, and structural factors of households' enrollment level in CBHIS.
A cross-sectional study may have a temporal relationship between the outcome and independent variables. The data for EMDHS were gathered based on the respondents' recall ability, which might be subjected to recall bias. Moreover, important variables that can significantly determine the households' enrollment level in CBHIS may not be included as limited variables are found in the 2019 EMDHS data sets. Despite these limitations, the data for the study were collected across the country, and the results can be nationally representative. Furthermore, multilevel analysis was employed, which is more appropriate for cluster data to solve data dependency. The geographically weighted regression analysis was performed. The findings and various data analysis techniques used in this study could be used as inputs in future research.
The original contributions presented in the study are included in the article/Supplementary material, further inquiries can be directed to the corresponding author.
Ethical approval was not required for the studies involving humans because this study was based on secondary data source, which is publicly accessible from The Measure Demographic and Health Survey website. Thus, direct ethical approval from the ethical review board was not necessary. The studies were conducted in accordance with the local legislation and institutional requirements. Written informed consent for participation was not required from the participants or the participants' legal guardians/next of kin in accordance with the national legislation and institutional requirements because this study was based on secondary data source, which is publicly accessible from The Measure Demographic and Health Survey website. Thus, direct consent from the study participants was not necessary.
AWD: Conceptualization, Data curation, Formal analysis, Investigation, Methodology, Validation, Visualization, Writing – original draft, Writing – review & editing.
The author(s) declare that no financial support was received for the research, authorship, and/or publication of this article.
I would like to express my deepest appreciation and gratitude to the Measure DHS program for permission to data use for this study.
The author declares that the research was conducted in the absence of any commercial or financial relationships that could be construed as a potential conflict of interest.
All claims expressed in this article are solely those of the authors and do not necessarily represent those of their affiliated organizations, or those of the publisher, the editors and the reviewers. Any product that may be evaluated in this article, or claim that may be made by its manufacturer, is not guaranteed or endorsed by the publisher.
The Supplementary Material for this article can be found online at: https://www.frontiersin.org/articles/10.3389/fpubh.2024.1305458/full#supplementary-material
CBHIS, Community-Based Health Insurance Scheme; CI, Confidence Interval; CSA, Central Statistical Agency; DHS, Demographic and Health Survey; EAs, Enumeration Areas; EDHS, Ethiopian Demographic and Health Survey; EMDHS, Ethiopia Mini Demography and Health Survey; EPHC, Ethiopian Population and Housing Census; EPHI, Ethiopian Public Health Institute; GTP, Growth and Transformation Plan; GWR, Geographically Weighted Regression; RR, Relative Risk; LLR, Log-likelihood Ratio; OLS, Ordinary Least Square; OPP, Out of Pocket Payment; SDG, Sustainable Development Goals; SHI, Social Health Insurance; SNNPR, South Nations and Nationality and Peoples of the region; STATA, Statistical software for data science; WHO, World Health Organization.
1. Devadasan N, Criel B, Van Damme W, Lefevre P, Manoharan S, Van der Stuyft P, et al. Community health insurance schemes and patient satisfaction-evidence from India. Indian J Med Res. (2011) 133:40–9.
2. Vialle-Valentin CE, Ross-Degnan D, Ntaganira J, Wagner AK. Medicines coverage and community-based health insurance in low-income countries. Health Res Policy Syst. (2008) 6:1–11. doi: 10.1186/1478-4505-6-11
3. Boubacar A. Healthcare financing in low and middle-income countries and achieving universal health coverage. Resolusi: J Sosial Politik. (2021) 4:86–94. doi: 10.32699/resolusi.v4i2.2195
4. Demsash AW, Chereka AA, Walle AD, Kassie SY, Bekele F, Bekana T, et al. Machine learning algorithms' application to predict childhood vaccination among children aged 12–23 months in Ethiopia: evidence 2016 Ethiopian demographic and health survey dataset. PLoS ONE. (2023) 18:e0288867. doi: 10.1371/journal.pone.0288867
5. Barber SL, Yao L. Health Insurance Systems in China: A Briefing Note. Geneva: World health report (2010). 37.
6. Wang Z, Li X, Chen M. Catastrophic health expenditures and its inequality in elderly households with chronic disease patients in China. Int J Equity Health. (2015) 14:1–11. doi: 10.1186/s12939-015-0134-6
7. Garg CC, Karan AK. Reducing out-of-pocket expenditures to reduce poverty: a disaggregated analysis at rural-urban and state level in India. Health Policy Plan. (2009) 24:116–28. doi: 10.1093/heapol/czn046
8. Wagstaff A, Doorslaer EV. Catastrophe and impoverishment in paying for health care: with applications to Vietnam 1993–1998. Health Econ. (2003) 12:921–33. doi: 10.1002/hec.776
9. Demissie B, Gutema Negeri K. Effect of community-based health insurance on utilization of outpatient health care services in Southern Ethiopia: a comparative cross-sectional study. Risk Manag Healthc Policy. (2020) 13:141–53. doi: 10.2147/RMHP.S215836
10. Kebede SA, Liyew AM, Tesema GA, Agegnehu CD, Teshale AB, Alem AZ, et al. Spatial distribution and associated factors of health insurance coverage in Ethiopia: further analysis of Ethiopia demographic and health survey, 2016. Arch Pub Health. (2020) 78:1–10. doi: 10.1186/s13690-020-00407-0
11. Minyihun A, Gebregziabher MG, Gelaw YA. Willingness to pay for community-based health insurance and associated factors among rural households of Bugna District, Northeast Ethiopia. BMC Res Notes. (2019) 12:1–7. doi: 10.1186/s13104-019-4091-9
12. Smit N, Mpedi LG. Social protection for developing countries: Can social insurance be more relevant for those working in the informal economy?. Law. Democr Dev. (2010) 14:1–33. doi: 10.4314/ldd.v14i1.4
13. Demsash AW, Walle AD. Women's health service access and associated factors in Ethiopia: application of geographical information system and multilevel analysis. BMJ Health Care Inf. (2023) 30:720. doi: 10.1136/bmjhci-2022-100720
14. Adebayo EF, Ataguba JE, Uthman OA, Okwundu CI, Lamont KT, Wiysonge CS, et al. Factors that affect the uptake of community-based health insurance in low-income and middle-income countries: a systematic protocol. BMJ Open. (2014) 4:e004167. doi: 10.1136/bmjopen-2013-004167
15. Dorjdagva J, Batbaatar E, Svensson M, Dorjsuren B, Kauhanen J. Catastrophic health expenditure and impoverishment in Mongolia. Int J Equity Health. (2016) 15:1–9. doi: 10.1186/s12939-016-0395-8
16. Su TT, Kouyaté B, Flessa S. Catastrophic household expenditure for health care in a low-income society: a study from Nouna District, Burkina Faso. Bull World Health Organ. (2006) 84:21–7. doi: 10.2471/BLT.05.023739
17. Xu K, Evans DB, Kadama P, Nabyonga J, Ogwal PO, Nabukhonzo P, et al. Understanding the impact of eliminating user fees: utilization and catastrophic health expenditures in Uganda. Soc Sci Med. (2006) 62:866–76. doi: 10.1016/j.socscimed.2005.07.004
18. Xu K, Evans DB, Carrin G, Aguilar-Rivera AM, Musgrove P, Evans T, et al. Protecting households from catastrophic health spending. Health Aff. (2007) 26:972–83. doi: 10.1377/hlthaff.26.4.972
19. Ikegami N. Universal Health Coverage for Inclusive and Sustainable Development: Lessons from Japan. London: World Bank Publications. (2014).
20. Esmael A, Tsegaye G, Wubie M, Abera H, Endris M. Treatment outcomes of tuberculosis patients in Debre Markos referral hospital, north West Ethiopia (June 2008-august 2013): a five year retrospective study. Int J Pharm Sci Res. (2014) 5:1500. doi: 10.13040/IJPSR.0975-8232.5(4).1500-05
21. World Health Organization. Strategy on Health Care Financing For countries of the Western Pacific and South-East Asia Regions (2006-2010). Geneva: WHO (2005).
23. McIntyre D, Kutzin J. Health Financing Country Diagnostic: A Foundation for National Strategy Development. Geneva: WHO (2016).
24. Doetinchem O, Carrin G, Evans D. Thinking of Introducing Social Health Insurance? Ten Questions. Geneva: World Health Organization (2010).
25. Mekonne A, Seifu B, Hailu C, Atomsa A. Willingness to pay for social health insurance and associated factors among health care providers in Addis Ababa, Ethiopia. BioMed Res Int. (2020) 2020:7. doi: 10.1155/2020/8412957
26. Evans DB, Etienne C. Health systems financing and the path to universal coverage. Bull World Health Organ. (2010) 88:402–3. doi: 10.2471/BLT.10.078741
27. Etienne C, Asamoa-Baah A, Evans DB. Health Systems Financing: The Path to Universal Coverage. Geneva: World Health Organization (2010).
28. Evans DB, Hsu J, Boerma T. Universal Health Coverage and Universal Access. London: SciELO Public Health (2013), 546–546A.
29. Saksena P, Hsu J, Evans DB. Financial risk protection and universal health coverage: evidence and measurement challenges. PLoS Med. (2014) 11:e1001701. doi: 10.1371/journal.pmed.1001701
30. Kutzin J. Health financing for universal coverage and health system performance: concepts and implications for policy. Bull World Health Organ. (2013) 91:602–11. doi: 10.2471/BLT.12.113985
31. Demsash AW, Emanu MD, Walle AD. Exploring spatial patterns, and identifying factors associated with insufficient cash or food received from a productive safety net program among eligible households in Ethiopia: a spatial and multilevel analysis as an input for international food aid programmers. BMC Public Health. (2023) 23:1141. doi: 10.1186/s12889-023-16001-2
32. Awosika L. Health insurance and managed care in Nigeria. Annal Ibadan Postgr Med. (2005) 3:40–51. doi: 10.4314/aipm.v3i2.39066
33. Tang S, Meng Q, Chen L, Bekedam H, Evans T, Whitehead M, et al. Tackling the challenges to health equity in China. Lancet. (2008) 372:1493–501. doi: 10.1016/S0140-6736(08)61364-1
35. Wang Z, Li X, Chen M, Si L. Social health insurance, healthcare utilization, and costs in middle-aged and elderly community-dwelling adults in China. Int J Equity Health. (2018) 17:1–13. doi: 10.1186/s12939-018-0733-0
36. ICF and CSA. Ethiopia Demographic and Health Survey 2016. Addis Ababa, Ethiopia. Rockville, MD: CSA and ICF (2016).
37. Nageso D, Tefera K, Gutema K. Enrollment in community based health insurance program and the associated factors among households in Boricha district, Sidama Zone, Southern Ethiopia; a cross-sectional study. PLoS ONE. (2020) 15:e0234028. doi: 10.1371/journal.pone.0234028
38. Abdilwohab MG, Abebo ZH, Godana W, Ajema D, Yihune M, Hassen H, et al. Factors affecting enrollment status of households for community based health insurance in a resource-limited peripheral area in Southern Ethiopia. Mixed Method PloS ONE. (2021) 16:e0245952. doi: 10.1371/journal.pone.0245952
39. Workneh SG, Biks GA, Woreta SA. Community-based health insurance and communities' scheme requirement compliance in Thehuldere district, northeast Ethiopia: cross-sectional community-based study. ClinicoEconomics Outcomes Res. (2017) 9:353–9. doi: 10.2147/CEOR.S136508
40. Ethiopian Public Health Institute (EPHI) [Ethiopia] and ICF. Rockville, MD: USA: EPHI and ICF. Ethiopia Mini Demographic and Health Survey 2019: Final Report. (2021) Available online at: https://dhsprogram.com/publications/publication-FR363-DHS-Final-Reports.cfm
41. Yitayew MY, Adem MH, Tibebu NS. Willingness to enroll for community-based health insurance and associated factors in Simada District, north-west, Ethiopia, 2020: a community-based cross-sectional study. Risk Manag Healthc Policy. (2020) 13:3031–8. doi: 10.2147/RMHP.S280685
42. Demsash AW, Chereka AA, Kassie SY, Donacho DO, Ngusie HS, Tegegne MD, et al. Spatial distribution of vitamin A rich foods intake and associated factors among children aged 6–23 months in Ethiopia: spatial and multilevel analysis of 2019 Ethiopian mini demographic and health survey. BMC Nutr. (2022) 8:77. doi: 10.1186/s40795-022-00573-0
43. Anselin L, Getis A. Spatial statistical analysis and geographic information systems. Ann Reg Sci. (1992) 26:19–33. doi: 10.1007/BF01581478
44. O'Sullivan D. Geographically weighted regression: the analysis of spatially varying relationships. Geogr Anal. (2003) 35:272–5. doi: 10.1353/geo.2003.0008
45. Chaikaew N, Tripathi NK, Souris M. Exploring spatial patterns and hotspots of diarrhea in Chiang Mai, Thailand. Int J Health Geogr. (2009) 8:1–10. doi: 10.1186/1476-072X-8-36
46. Kulldorff M. Information Management Services, Inc SaTScan™ Version 4, 0 Software for the Spatial and Space-Time Scan Statistics. Rockville, MD: Information Management Services (2004).
47. Kulldorff M. A spatial scan statistic. Commun Stat Theor Methods. (1997) 26:1481–96. doi: 10.1080/03610929708831995
48. Alemu K, Worku A, Berhane Y, Kumie A. Spatiotemporal clusters of malaria cases at village level, northwest Ethiopia. Malar J. (2014) 13:1–8. doi: 10.1186/1475-2875-13-223
49. Fotheringham AS, Brunsdon C, Charlton M. Geographically Weighted Regression: The Analysis of Spatially Varying Relationships. New York, NY: John Wiley and Sons (2003).
50. Gezie LD. Predictors of CD4 count over time among HIV patients initiated ART in Felege Hiwot Referral Hospital, northwest Ethiopia: multilevel analysis. BMC Res Notes. (2016) 9:1–9. doi: 10.1186/s13104-016-2182-4
51. Demsash AW, Tegegne MD, Wubante SM, Walle AD, Donacho DO, Senishaw AF, et al. Spatial and multilevel analysis of sanitation service access and related factors among households in Ethiopia: using 2019 Ethiopian national dataset. PLOS Global Public Health. (2023) 3:e0001752. doi: 10.1371/journal.pgph.0001752
52. Tiruneh SA, Ayele BA, Yitbarek GY, Asnakew DT, Engidaw MT, Gebremariam AD, et al. Spatial distribution of iron rich foods consumption and its associated factors among children aged 6–23 months in Ethiopia: spatial and multilevel analysis of 2016 Ethiopian demographic and health survey. Nutr J. (2020) 19:1–13. doi: 10.1186/s12937-020-00635-8
53. Onwujekwe O, Onoka C, Uzochukwu B, Okoli C, Obikeze E, Eze S, et al. Is community-based health insurance an equitable strategy for paying for healthcare? Experiences from southeast Nigeria. Health Policy. (2009) 92:96–102. doi: 10.1016/j.healthpol.2009.02.007
54. Kimani JK, Ettarh R, Warren C, Bellows B. Determinants of health insurance ownership among women in Kenya: evidence from the 2008–09 Kenya demographic and health survey. Int J Equity Health. (2014) 13:1–8. doi: 10.1186/1475-9276-13-27
55. Mebratie AD. Enrollment in Ethiopia's community-based health insurance scheme. World Dev. (2015) 74:58–76. doi: 10.1016/j.worlddev.2015.04.011
56. Nshakira-Rukundo E, Mussa EC, Nshakira N, Gerber N, Von Braun J. Determinants of enrolment and renewing of community-based health insurance in households with under-5 children in rural south-western Uganda. Int J Health Policy Manage. (2019) 8:593. doi: 10.15171/ijhpm.2019.49
57. Kwon S. Community-Based Health Insurance in Ethiopia: Enrollment, memebrship renewal, and effects on health service utilization (2018).
58. Chanie MG, Ewunetie GE. Determinants of enrollment in community based health insurance among Households in Tach-Armachiho Woreda, North Gondar, Ethiopia, 2019. PLoS One. (2020) 15:e0236027. doi: 10.1371/journal.pone.0236027
59. Umeh CA, Feeley FG. Inequitable access to health care by the poor in community-based health insurance programs: a review of studies from low-and middle-income countries. Global Health: Sci Prac. (2017) 5:299–314. doi: 10.9745/GHSP-D-16-00286
60. Odeyemi IA. Community-based health insurance programmes and the national health insurance scheme of Nigeria: challenges to uptake and integration. Int J Equity Health. (2014) 13:1–13. doi: 10.1186/1475-9276-13-20
61. Yilma Z, Mebratie A, Sparrow R, Dekker M, Alemu G, Bedi AS, et al. Impact of Ethiopia's community based health insurance on household economic welfare. World Bank Econ Rev. (2015) 29:S164–73. doi: 10.1093/wber/lhv009
62. Fite MB, Roba KT, Merga BT, Tefera BN, Beha GA, Gurmessa TT, et al. Factors associated with enrollment for community-based health insurance scheme in Western Ethiopia: Case-control study. PLoS ONE. (2021) 16:e0252303. doi: 10.1371/journal.pone.0252303
63. Jütting J. Health insurance for the poor?: determinants of participation in community-based health insurance schemes in rural Senegal (2003). doi: 10.1787/18151949
64. Alkenbrack S, Jacobs B, Lindelow M. Achieving universal health coverage through voluntary insurance. BMC Health Serv Res. (2013) 13:1–14. doi: 10.1186/1472-6963-13-521
65. Negash B, Dessie Y, Gobena T. Community based health insurance utilization and associated factors among informal workers in Gida Ayana District, Oromia Region, West Ethiopia. East Afr J Health Biomed Sci. (2019) 3:13–22.
66. Parmar D, De Allegri M, Savadogo G, Sauerborn, R. Do community-based health insurance schemes fulfil the promise of equity? A study from Burkina Faso. Health Policy Plan. (2014) 29:76–84. doi: 10.1093/heapol/czs136
67. Jehu-Appiah C, Aryeetey G, Spaan E, Hoop D, Agyepong TI, Baltussen R. Equity aspects of the National Health Insurance Scheme in Ghana: Who is enrolling, who is not and why?. Soc Sci Med. (2011) 72:157–65. doi: 10.1016/j.socscimed.2010.10.025
68. Dong H, Allegri D, Gnawali M, Souares DA, Sauerborn R. Drop-out analysis of community-based health insurance membership at Nouna, Burkina Faso. Health Policy. (2009) 92:174–9. doi: 10.1016/j.healthpol.2009.03.013
69. Allegri D, Kouyaté M, Becher B, Gbangou H, Pokhrel A, Sanon S, et al. Understanding enrolment in community health insurance in sub-Saharan Africa: a population-based case-control study in rural Burkina Faso. Bullet World Health Org. (2006) 84:852–8. doi: 10.2471/BLT.06.031336
70. Annear PL, Bigdeli M, Jacobs B. A functional model for monitoring equity and effectiveness in purchasing health insurance premiums for the poor: evidence from Cambodia and the Lao PDR. Health Policy. (2011) 102:295–303. doi: 10.1016/j.healthpol.2011.03.005
71. Worku GT. Determinants of Enrollment Decision in the Community Based Health Insurance, North West Ethiopia: A Case Control Study. In: 31st EPHA Annual Conference (2020).
72. Ogben C, Ilesanmi O. Community based health insurance scheme: preferences of rural dwellers of the federal capital territory Abuja, Nigeria. J Pub Health Afr. (2018) 9:540. doi: 10.4081/jphia.2018.540
73. Ebrahim K, Yonas F, Kaso M. Willingness of community to enroll in community based health insurance and associated factors at household Level in Siraro District, West Arsi Zone, Ethiopia. J Pub Health Epidemiol. (2019) 11:137–44. doi: 10.5897/JPHE2018.1094
74. Fadlallah R, El-Jardali F, Hemadi N, Morsi RZ, Abou Samra CA, Ahmad A, et al. Barriers and facilitators to implementation, uptake and sustainability of community-based health insurance schemes in low-and middle-income countries: a systematic review. Int J Equity Health. (2018) 17:1–18. doi: 10.1186/s12939-018-0721-4
75. Dror DM, Hossain SS, Majumdar A, Pérez Koehlmoos TL, John D, Panda PK, et al. What factors affect voluntary uptake of community-based health insurance schemes in low-and middle-income countries? A systematic review and meta-analysis. PLoS ONE. (2016) 11:e0160479. doi: 10.1371/journal.pone.0160479
76. Carrin G, Waelkens MP, Criel B. Community-based health insurance in developing countries: a study of its contribution to the performance of health financing systems. Trop Med Int Health. (2005) 10:799–811. doi: 10.1111/j.1365-3156.2005.01455.x
Keywords: health insurance, households, enrollment, spatial analysis, geographical analysis
Citation: Demsash AW (2024) Spatial distribution and geographical heterogeneity factors associated with households' enrollment level in community-based health insurance. Front. Public Health 12:1305458. doi: 10.3389/fpubh.2024.1305458
Received: 01 October 2023; Accepted: 25 April 2024;
Published: 17 May 2024.
Edited by:
John Yfantopoulos, National and Kapodistrian University of Athens, GreeceReviewed by:
Mengqian Zhang, Lanzhou University, ChinaCopyright © 2024 Demsash. This is an open-access article distributed under the terms of the Creative Commons Attribution License (CC BY). The use, distribution or reproduction in other forums is permitted, provided the original author(s) and the copyright owner(s) are credited and that the original publication in this journal is cited, in accordance with accepted academic practice. No use, distribution or reproduction is permitted which does not comply with these terms.
*Correspondence: Addisalem Workie Demsash, YWRkaXNhbGVtd29ya2llNTk5QGdtYWlsLmNvbQ==
Disclaimer: All claims expressed in this article are solely those of the authors and do not necessarily represent those of their affiliated organizations, or those of the publisher, the editors and the reviewers. Any product that may be evaluated in this article or claim that may be made by its manufacturer is not guaranteed or endorsed by the publisher.
Research integrity at Frontiers
Learn more about the work of our research integrity team to safeguard the quality of each article we publish.