- 1Unit of Psychiatry, Department of Public Health and Medicinal Administration, Institute of Translational Medicine, Faculty of Health Sciences, University of Macau, Macao, Macao SAR, China
- 2Kiang Wu Nursing College of Macau, Macao, Macao SAR, China
- 3Centre for Cognitive and Brain Sciences, University of Macau, Taipa, Macao SAR, China
- 4Beijing Key Laboratory of Mental Disorders, National Clinical Research Center for Mental Disorders & National Center for Mental Disorders, Beijing Anding Hospital, Capital Medical University, Beijing, China
- 5School of Public Health, Southeast University, Nanjing, China
- 6School of Nursing, Hong Kong Polytechnic University, Kowloon, Hong Kong SAR, China
- 7Section of Psychiatry, University of Notre Dame Australia, Fremantle, WA, Australia
- 8Division of Psychiatry, School of Medicine, University of Western Australia, Perth, WA, Australia
- 9Department of Psychiatry, The Melbourne Clinic and St Vincent's Hospital, University of Melbourne, Richmond, VIC, Australia
Background: China recorded a massive COVID-19 pandemic wave after ending its Dynamic Zero-COVID Policy on January 8, 2023. As a result, mental health professionals (MHPs) experienced negative mental health consequences, including an increased level of fear related to COVID-19. This study aimed to explore the prevalence and correlates of COVID-19 fear among MHPs following the end of the Policy, and its association with quality of life (QoL) from a network analysis perspective.
Methods: A cross-sectional national study was conducted across China. The correlates of COVID-19 fear were examined using both univariate and multivariate analyses. An analysis of covariance (ANCOVA) was conducted to determine the relationship between fear of COVID-19 and QoL. Central symptoms were identified using network analysis through the “Expected Influence” of the network model while specific symptoms directly correlated with QoL were identified through the “flow function.”
Results: A total of 10,647 Chinese MHPs were included. The overall prevalence of COVID-19 fear (FCV-19S total score ≥ 16) was 60.8% (95% CI = 59.9–61.8%). The binary logistic regression analysis found that MHPs with fear of COVID-19 were more likely to be married (OR = 1.198; p < 0.001) and having COVID-19 infection (OR = 1.235; p = 0.005) and quarantine experience (OR = 1.189; p < 0.001). Having better economic status (good vs. poor: OR = 0.479; p < 0.001; fair vs. poor: OR = 0.646; p < 0.001) and health status (good vs. poor: OR = 0.410; p < 0.001; fair vs. poor: OR = 0.617; p < 0.001) were significantly associated with a lower risk of COVID-19 fear. The ANCOVA showed that MHPs with fear of COVID-19 had lower QoL [F = 228.0, p < 0.001]. “Palpitation when thinking about COVID-19” was the most central symptom in the COVID-19 fear network model, while “Uncomfortable thinking about COVID-19” had the strongest negative association with QoL (average edge weight = −0.048).
Conclusion: This study found a high prevalence of COVID-19 fear among Chinese MHPs following the end of China’s Dynamic Zero-COVID Policy. Developing effective prevention and intervention measures that target the central symptoms as well as symptoms correlated with QoL in our network structure would be important to address COVID-19 fear and improve QoL.
1. Introduction
The COVID-19 pandemic has been a major global public health challenge (1) which poses a substantial physical health threat and also results in significant mental health burden (2, 3). A unique characteristic of the pandemic (4) has been the widespread fear of COVID-19 in the community (5), which can be triggered by the lack of knowledge regarding the novel illness (6) and its potential life-threatening risk (7).
Notably, fear of COVID-19 has been more profound among healthcare professionals (HPs) compared to the general population (8), and ranked as the top mental health challenge among HPs (9). Healthcare professionals have reported moderate to high levels of COVID-19 fear during the pandemic (10–12), while still needing to provide healthcare to infected patients during this critical period, thus causing an increased vulnerability to negative psychological impacts (3) and work-related burden (13). In recent meta-analyses, fear of COVID-19 was associated with a broad range of mental health problems such as stress, anxiety, insomnia, and depression among both the general population and HPs (7, 14). Additionally, fear of COVID-19 was linked to a lower quality of life (QoL) among the general populations (15, 16) and higher levels of job stress (10, 17, 18), burnout (13, 17), and turnover intention (10, 19, 20).
A recent meta-analysis (12) reported that the highest level of COVID-19 fear was found in Asia compared to other continents. Further, the level of COVID-19 fear was significantly associated with the highest increase of infection in United States (21). As such, the massive and sudden COVID-19 surge that occurred after the end of China’s Dynamic Zero-COVID policy in China resulted in widespread fear of COVID-19. Since August 2021, China had effectively adopted the Dynamic Zero-COVID policy through strict quarantine and management measures to control COVID-19 transmission (22). As omicron variants were found to have less pathogenicity than original strains (23), the Chinese government formally ended the Dynamic Zero-COVID policy on January 8, 2023, and discontinued all centralized quarantine, contact tracing, and mass testing of nucleic acids (24). However, Omicron variants, due to its enhanced and faster transmission ability (25), rapidly resulted in a large-scale infection wave across China (26). Hence, a massive surge in infections occurred immediately within a short period, estimated to be between 167 and 279 million cases (27).
The mental health impact of the COVID-19 pandemic affected more people than the physical health impact (3). As the COVID-19 pandemic evolved, the demand for mental health services increased dramatically (28), and Mental Health Professionals (MPHs) played vital roles in the provision of mental health services (3). Apart from the excessive work burden, MPHs had to adapt to unfamiliar environments caused by changes in the delivery and settings of mental health services, as well as dealing with the fear of COVID-19 (29, 30). In response to the increasing mental health burden, MPHs and academic societies in China published various guidelines concerning the delivery of mental health services (i.e., outreach and hotline services) (5, 31). Although substantial measures to mitigate the psychiatric effects of the pandemic have been implemented, the psychological well-being of MHPs in hospitals and community centers has received little attention (30) compared to frontline HPs during pandemic (32). Moreover, to date, there is a lack of research on the impact of COVID-19 fear on QoL, and the relationship between COVID-19 fear and QoL among MHPs. Previous research only explored the impact of COVID-19 fear on health-related QoL among the general population (15, 16), work-related QoL among MPHs in Greece (13) and in nurses in Spanish (33). QoL refers to the perceived physical, material, social, and mental well-being over time by individuals (34, 35). Measuring QoL is critical for both assessing the population needs and informing policy decisions (36, 37). Thus, it is imperative to investigate the relevant correlates of COVID-19 fear among MHPs and its links with QoL after the end of China’s Dynamic Zero-COVID policy.
Network analysis (NA) provides a novel way to examine psychiatric problems conceptualized as causal interactions between symptom systems (38). In the network, the nodes represent the symptoms associated with a specific syndrome, and the edges represent the correlations between the symptoms (39), while the central node represents the most influential symptom in a network (40, 41) which can be prioritized for specific intervention based on the interconnection to other symptoms (42). NA has been widely utilized for various psychiatric disorders among multiple population subgroups during the COVID-19 pandemic (43–45). Previous network analyses focused only on the fear of COVID-19 symptoms network model among the general population in Iran, Bangladesh, and Norway (46), while a recent network analysis study examined the interrelationship between anxiety, depression, and QoL among HPs in China during the pandemic (47). However, to date, no studies have focused on the symptoms of COVID-19 fear or their links with QoL among MHPs in China following the end of China’s Dynamic Zero-COVID policy.
To address these gaps, this study (1) investigated the prevalence and correlates of COVID-19 fear among MHPs in China immediately after the end of China’s Dynamic Zero-COVID policy, (2) identified the most central symptoms of COVID-19 fear in the network model, and (3) analyzed the relationship between symptoms of COVID-19 fear and QoL.
2. Methods
2.1. Study design and participants
A cross-sectional, national survey was conducted by the panel members of the Psychiatry Branch of the Chinese Nursing Association and the Chinese Society of Psychiatry between January 22 and February 10, 2023 immediately after the end of the Dynamic Zero-COVID policy in China. To decrease the possibility of infection during the COVID-19 pandemic, as recommended in previous studies (44, 48, 49), a snowball convenience sampling method with the WeChat-based Questionnaire Star was adopted for this study. WeChat is one of the most popular communication methods and is widely used in clinical practice and continuing education in China (50, 51). During the COVID-19 pandemic, all health professionals in China were required to report their health status daily using WeChat. Hence, thus it could be assumed that all MHPs were WeChat users (48, 52). Questionnaire Star program is a commonly used research tool in China’s epidemiological survey (53). To be eligible, participants were: (1) adults aged 18 years or above; (2) MHPs (e.g., psychiatrists, nurses or technicians) who worked in psychiatric hospitals or in psychiatric departments of general hospitals in China during the COVID-19 pandemic; and (3) able to understand Chinese and provide written informed consent. The Ethics Committee of the Beijing Anding Hospital in China approved the study protocol and all participants provided electronic informed consent.
2.2. Measures
The sociodemographic data were collected, including age, sex, marital status, educational level, clinical work experience (years), living status, perceived economic and health status, previous COVID-19 infection, and experience of quarantine during the COVID-19 pandemic.
The fear of COVID-19 infection was assessed using the validated Chinese version of the Fear of COVID-19 Scale (FCV-19S) (4, 54) that has good reliability and validity among Chinese populations (55). The FCV-19S consisted of seven items, covering two dimensions: physical response (three items) of fear and thoughts of fear (four items) (4, 54). Each item was rated on a five-point Likert scale from 1 (“strongly disagree”) to 5 (“strongly agree”). The total score ranged from 7 to 35, with a higher score indicating greater fear of COVID-19 (4). A total score of FCV-19S of ≥16 was considered as “having COVID-19 fear,” which could significantly reflect the psychological impact of COVID-19 fear (55).
The assessment of the global Quality of Life (QoL) was based on the sum of scores for the first two items of the Chinese version of the World Health Organization Quality of Life-Brief Version (WHOQOL-BREF) (56–58). The Chinese version of the WHOQOL-BREFF has been validated in Chinese populations with good sensitivity and specificity (58, 59) with a higher total score indicating better QoL (56, 58).
2.3. Statistical analysis
2.3.1. Univariate and multivariate analyses
Statistical analyses were conducted using SPSS version 22.0 for both univariate and multivariate analysis (SPSS Inc., Chicago, Illinois, United States). One-sample Kolmogorov–Smirnov tests were used to assess the distribution normality of continuous variables. The sociodemographic and clinical characteristics of participants with and without fear of COVID-19 infection were compared using independent sample t-tests or Mann–Whitney U tests for continuous variables and Chi-square tests for categorical variables. To determine the independent correlates of COVID-19 fear, a binary logistic regression analysis was conducted, using fear of COVID-19 infection as the dependent variable and variables with significant differences in univariate analyses as independent variables by applying an “Enter” method. The threshold for significant statistical differences was set at p < 0.05 (two-tailed).
2.3.2. Network estimation
Network structure analysis was conducted using R software (version 4.2.2) (60). The fear of COVID-19 infection network structure was analyzed using a Graphical Gaussian Model (GGM) with graphic least absolute shrinkage and selection operator (LASSO) and an Extended Bayesian Information Criterion (EBIC) model (61), which could provide enhanced prediction accuracy, interpretability, and optimality of the network model (62). The network estimation was evaluated using the “estimateNetwork” function in the R package “bootnet” with “EBICglasso” as the default method (63). The visualization of the network was conducted using the R package “qgraph” (61) and optimized with the visual representation by “ggplot2” (61, 64). In a network model, each node represents an individual symptom of COVID-19 fear, while each edge represents the association between two symptoms. Thick edges indicate stronger correlations, while green edges indicate positive correlations and red edges indicate negative correlations (63). Expected Influence (EI) in the network model was used to determine central symptoms based on its reliability as an indicator of centrality (65); nodes with a greater EI were considered to be more important and influential (66). The value of predictability was indicated as the linkage between its neighboring nodes (66), which was calculated using the “mgm” package (67). Additionally, the ‘flow’ function in the R package “qgraph” was used to identify specific symptoms of COVID-19 fear that were directly associated with QoL (63).
To determine the stability and accuracy of the network model, the “bootnet” function in R package (Version 1.4.3) (61) was used with 1,000 permutations of the case dropping bootstrap procedure for each node. The stability of the network was assessed using a correlation stability coefficient (CS-coefficient). In the presence of a correlation greater than 0.7, a maximum proportion of cases could be dropped, indicating a 95% probability that the original centrality indices would be correlated with the centrality of subset networks (61). According to previous studies (43, 61, 66), a CS-coefficient value exceeding 0.25 was considered stable in the network model, while a value exceeding 0.5 was considered preferable. An edge accuracy estimate was derived using bootstrapped 95% confidence intervals (CIs), where a narrower CI would suggest a more trustworthy network (61). A non-parametric bootstrapped difference test was conducted to evaluate differences between edge pairs. The difference between two nodes or edges was significant if zero was excluded based on the 95% CI (61).
3. Results
3.1. Participant characteristics
A total of 11,524 MHPs were invited to participate in this study, of whom 10,647 met the study entry criteria and completed the assessment, with a participation rate of 98.0%. The demographic and clinical characteristics of the participants are shown in Table 1. In the study, the mean age of participants was 34.85 (SD = 8.395) years and 18.0% were males (n = 1,920). Most participants had at least a college degree (n = 10,809; 94.8%), were married (n = 7,722; 72.5%) and lived with others (n = 9,454; 88.8%).
3.2. Prevalence and correlates of having COVID-19 fear
The mean total score of FCV-19S was 17.36 ± 6.147 (95% CI = 17.25–17.48%) and the overall prevalence of COVID-19 fear (FCV-19S total score ≥ 16) was 60.8% (n = 6,477; CI = 59.9–61.8%). A summary of the differences between subgroups with and without fear of COVID-19 is provided in Table 1. Participants with fear of COVID-19 were more likely to be male (p = 0.039), married (p = 0.011), living with others (p = 0.005), and have college and above education level (p < 0.001), poorer perceived economic status (p < 0.001), poorer perceived health status (p < 0.001), COVID-19 infection (p = 0.001), at least 1-week quarantine experience during the COVID-19 pandemic (p < 0.001), and a lower mean QoL score (p < 0.001). After controlling for covariates (i.e., gender, education, marital status, living status, economic and health status, COVID-19 infection, and quarantine experience), the analysis of covariance (ANCOVA) showed that MPHs with COVID fear still had lower QoL score [F =228.0, p < 0.001].
Table 2 shows the results of the binary logistic regression analysis of the participants with fear of COVID-19 infection. Participants who were married (OR = 1.198; p < 0.001), had COVID-19 infection since 2019 (OR = 1.235; p = 0.005), had at least 1-week quarantine experience during the COVID-19 pandemic (OR = 1.189; p < 0.001) were significantly associated with a higher risk of COVID-19 fear. Additionally, participants with better economic status (e.g., good vs. poor: OR = 0.479; p < 0.001; fair vs. poor: OR = 0.646; p < 0.001) and health status (e.g., good vs. poor: OR = 0.410; p < 0.001; fair vs. poor: OR = 0.617; p < 0.001) were significantly associated with a lower risk of fear of COVID-19 infection.
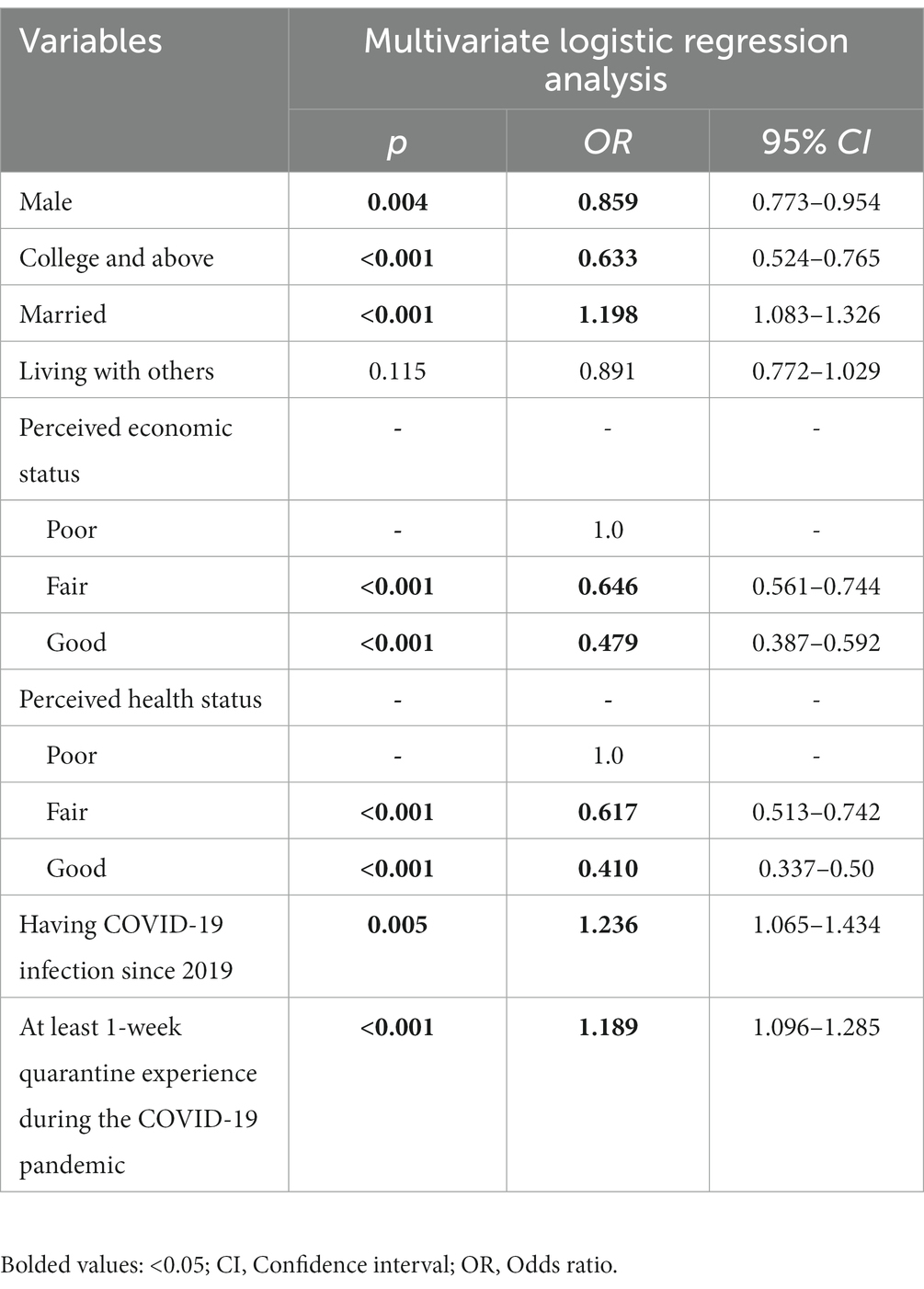
Table 2. Independent correlates of COVID-19 fear among Chinese mental health professionals (N = 10,647).
3.3. Network structure of symptoms of COVID-19 fear
A network structure of fear of COVID-19 symptoms as measured by the FCV-19S items is shown in Figure 1. The mean predictability in this sample was 0.544, indicating that 54.4% of each node’s variance could be explained by its neighboring nodes. The three nodes that had the highest centrality measured by EI were FOC7 (“Palpitation when thinking about COVID-19”), FOC6 (“Sleep difficulties caused by worried about COVID-19”), and FOC5 (“Nervous when watching news about COVID-19”). Supplementary Table S1 shows descriptive information and network centrality indices for each symptom of COVID-19 fear, and Supplementary Table S2 presents the correlation matrix regarding the correlation coefficient between the seven items of the FCV-19S.
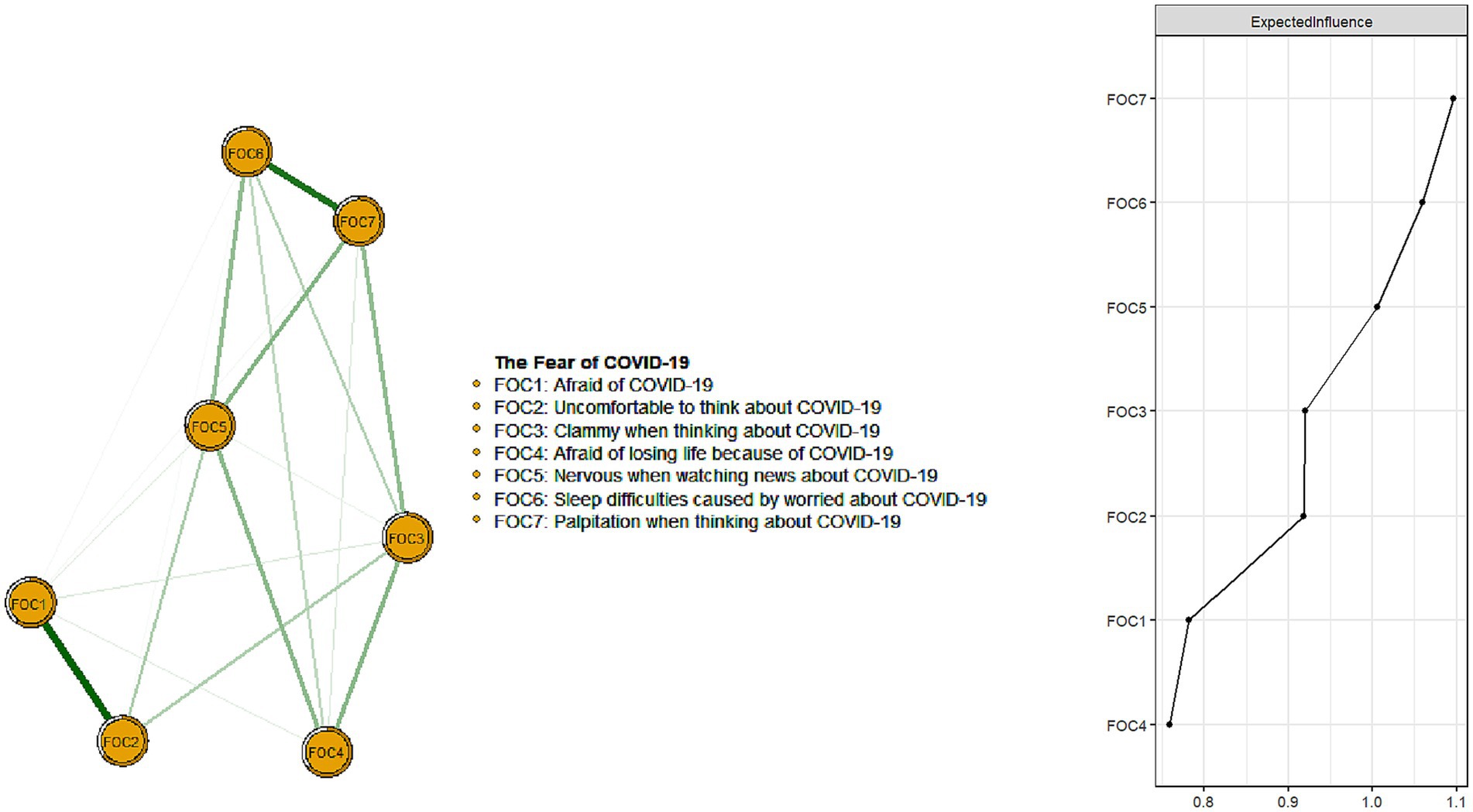
Figure 1. Network structure of the fear of COVID-19 infection among Chinese mental health professionals. Number of nodes: 8; Number of non-zero edges: 24/28; Mean weight/3ht: 0.1116963.
The flow network of QoL with symptoms of COVID-19 fear is presented in Figure 2. The FOC2 (“Uncomfortable to think about COVID-19”; average edge weight = −0.048) had the strongest negative association with QoL, followed by FOC7 (“Palpitation when thinking about COVID-19”; average edge weight = −0.043) and FOC5 (“Nervous when watching news about COVID-19”; average edge weight = −0.038).
Figure 3 illustrates the result of network stability. The CS-coefficient of EI was 0.75 based on the case-dropping bootstrap procedure, indicating that the network model was stable even if 75% of the sample drooped without significantly affecting the network structure. For the network accuracy, bootstrap 95% CIs for estimated edge weights revealed a narrow range, as shown in Supplementary Figure S1. Most edge weights were non-zero, suggesting that the network was accurate and stable. Supplementary Figure S2 shows that most edge-weight comparisons were statistically significant using bootstrapped difference tests, indicating that the network model was reliable.
4. Discussion
This was the first study to explore the prevalence, correlates, and network structure of COVID-19 fear among MHPs. The prevalence of COVID-19 fear among MHPs was 60.8% (95% CI: 59.9–61.8%), which was similar to previous findings (63.2, 95% CI: 61–65.3%) among frontline nurses in Wuhan, China, during the Dynamic Zero-COVID policy in China (18). However, this figure exceeded those reported in studies of MHPs in Greece (23.7%; 95% CI:18.3–29.0%) (13), HPs in Bangladesh (27.3%; 95% CI:24.3–30.5%) (68), HPs and general population in Australia (31.9%; 95% CI:28.0–35.9%) (69), and also HPs and general population in India (54.8, 95% CI:52.3–57.3%) (70). The mean total score of FCV-19S was 17.36 ± 6.147 in this study (95% CI = 17.25–17.48%), which was higher than the mean total score of FCV-19S across 35 countries (13.11, 95% CI: 11.57–14.65%) among general and HPs population according to a recent meta-analysis (7). This finding indicates that there have been an escalation of COVID-19 fear among MHPs in China after the end of the Dynamic Zero-COVID policy. The high prevalence of COVID-19 fear may be explained by factors similar to those found in previous research among HPs, including the rapid and extensive spread of infection (4), increased work burden, inadequate Protective Equipment (PPE) and supports (71, 72), having conflicting information (73), and sense of stigmatization (73, 74). Taken together, these factors likely contributed to the increased fear of COVID-19 (75, 76). An appropriate fear response could decrease at-risk behavior or promote compliance with infection prevention strategies like social distancing and handwashing (77). However, an overreaction to COVID-19 fear would often lead to erratic behavior during infectious epidemics regardless of gender and social status (78), such as panic buying of household items (78, 79) and medical supplies (77). Measures that could reduce fear include having adequate infection control training, clear COVID-19 related protocols and precise communication to all employees (73, 78), priorities on work safety, support and a manageable workload, as well as peer support systems to assist with mental health issues (73).
The study also identified several correlates of COVID-19 fear among MHPs in China. We found that people who were married, had experienced COVID-19 infection or quarantine during COVID-19 pandemic were more likely to experience fear of COVID-19, which is consistent with previous findings (8, 11, 70, 80, 81). Reasons for their fear may include concerns about spreading the infection to their families (80, 81) and having increased sense of responsibility for their family during quarantine (80, 82). In addition, HPs who fear returning home due to the risk of infecting their family members have almost a twofold higher risk of experiencing psychological reactions, anxiety, and obsessive-compulsive symptoms (83). Participants with better economic and health status were less likely to fear COVID-19, which is aligned with previous research that linked lower income levels to higher rates of psychological distress among HPs (83), and found a higher level of fear among individuals with chronic diseases (16). The greater level of fear may be explained by the fact that individuals with low income were more vulnerable to having psychological distress (84), while individuals with physical comorbidities who were infected with COVID-19 were at higher risk of life-threatening complications (85).
The network structure of the fear of COVID-19 was also examined. “Palpitations when thinking about COVID-19” (FOC7) was the most central symptom of the fear of COVID-19 network model and had the strongest connection to other symptoms. Other influential nodes included “Sleep difficulties caused by worried about COVID-19” (FOC6) and “Nervous when watching news about COVID-19” (FOC5). These findings are aligned with previous network research on COVID-19 fear among the general population in Iran, Bangladesh, and Norway (46). The most common symptom of FCV-19S, palpitations, was observed in this study and also previous research among the general population (86) and HPs (87). Additionally, recent research showed that having palpitations was a significant predictor of anxiety, depression, and insomnia (88). They were more common in patients with anxiety or depression symptoms after COVID-19 infection due to COVID-19 fear (89).
“Sleep difficulty” refers to insomnia or hypersomnia (90). “Sleep difficulties caused by worries about COVID-19” (FOC6) was a central symptom, which supports the results of a recent meta-analysis that a high prevalence of insomnia (44.1, 95% CI: 31.3–57.0%) was observed among HPs during the COVID-19 pandemic (91). More than half of MHPs experienced insomnia in a United Kingdom study during COVID-19 pandemic (92). Sleep quality is an indicator of anxiety and depressive symptoms among HPs (93). Furthermore, palpitations and sleep difficulties are part of physical aspects of FCV-19S (4) as well as somatic symptoms (94). In particular, the prevalence rate of somatic symptoms among HPs was 16% (95% CI: 3–36%) during the COVID-19 pandemic in a previous meta-analysis (95), which may be triggered by the fear of COVID-19 (96). HPs were twice more likely to experience somatic symptoms if they were fearful of returning home due to the risk of infecting their family members (83). Somatic symptoms, sometimes referred to as somatization, are psychological defense mechanisms against the recognition or expression of psychological distress (i.e., fear) (97), particularly in cultures where psychiatric disorders are highly stigmatized (98, 99). Signs of somatization, such as palpation and sleep difficulty, may suggest that individuals have irrational fears associated with COVID-19 that should be addressed (46).
“Nervous when watching news about COVID-19” (FOC5) was another central symptom in our study. Certain news about COVID-19 may cause nervousness and pose a hindrance to reduce the fear of COVID-19, which may be related to the characteristics of the media. The relationship between HPs and media is complicated and can be both a source of support and stress (73). For example, the media has a role to advocate for healthcare workers, which could assist in mobilizing medical resources (i.e., PPE) (73). However, mass media also could enhance the fear of COVID-19, such as “coronavirus infodemic” defined as excessive reporting of inaccurate news over social media platforms which bred fear (100, 101). It could exacerbate psychological distress including anxiety phobia, panic, and depression (78, 102, 103). By portraying the news catastrophically during pandemics, the media perpetuated racism, stigma and xenophobia against particular communities (6). It was difficult for HPs to admit having psychological needs and to engage in psychological intervention due to the potential stigma exacerbated by the media representation of militarism among HPs (73). Thus, rather than focusing on specific treatment of COVID-19 fear, early screening on somatic symptoms (i.e., palpations and sleep difficulty), eradicating stigma around the mental health distress of MHPs and reducing the negative impact of fake news on social media as central symptoms may be more effective for prevention of COVID-19 fear.
There was a negative relationship between fear of COVID-19 and QoL, as observed in this study and also other studies (15, 16). In the flow network model, the fear of COVID-19 symptoms of “Uncomfortable to think about COVID-19” (FOC2), “Palpitation when thinking about COVID-19” (FOC7), and “Nervous when watching news about COVID-19” (FOC5) had the most robust, direct negative connection to QoL and would be potential targets for reducing fear and enhancing QoL in this population. Previous research indicated that psychological and emotional distress had a major effect on QoL among HPs during the COVID-19 pandemic (104). Dysfunctional worry (i.e., worry which affects QoL) corresponds to adverse emotional outcomes that might be detrimental to mental health (105). Additionally, somatic symptoms such as palpations are also key indicators of poor health-related QoL by mediating anxiety and depression (106). The three flow symptoms identified in this study are part of physical and psychological responses among FCV-19S (54), indicating that physiological and mental subconscious responses are probably triggered by fear arising from being confronted with possible harm associated with the specific threat (i.e., infectious diseases) (107). These fear responses are directly associated with an individual’s psychological adjustment skills (i.e., experimental avoidance and psychological resilience), which could be predicted by fear of COVID-19 (108). A previous study reported that HPs with avoidance experience had a higher risk of COVID-19 fear, whereas psychological resilience was a key protector for reducing these fears (108). Therefore, strengthening avoidance coping skills and psychological resilience might play an essential role in maintaining QoL (108, 109). For example, cognitive-behavioral therapy could significantly reduce experiential avoidance among patients with anxiety disorders (110), stress (111, 112), and depression among HPs, as well as result in a profound increase in psychological resilience among HPs (112). In tandem with those studies, intervention to reduce fear of COVID-19 among MPHs could provide an effective approach for enhancing the QoL of MPHs who suffer from excessive fear.
The strengths of this study included the large sample size and use of network analysis to identify central symptoms of COVID-19 fear and those with strong correlations with QoL. However, several limitations should be acknowledged. First, this study was cross-sectional, therefore, causal relationships between fear of COVID-19 and other factors could not be inferred. To analyze the causal relationships and dynamic changes in fear of COVID-19 over time, future longitudinal studies are necessary. Second, this study was conducted in China, so the results may not be representative of other regions due to differences in COVID-19 policies and trajectory. Third, this study did not collect data regarding rural and urban areas. Data on geographical areas and hospital types should be examined in future studies on COVID-19 fear among HPs. Fourth, snowball sampling via an online survey was used to reduce the risk of COVID-19 infection, which might have resulted in selection biases. Finally, the assessment based on self-report, might lead to recall bias and social desirability bias.
In conclusion, this study found a high prevalence of COVID-19 fear among Chinese MPHs immediately following the end of China’s Dynamic Zero-COVID policy, which was associated with poor quality of life. Being married, having COVID-19 infection, quarantine experience, lower economic and health status were observed to significantly increase the risk of COVID-19 fear. “Palpitation when thinking about COVID-19” (FOC7) was the most central symptom in the network model while “Uncomfortable to think about COVID-19” (FOC2) had the most robust correlation with poor QoL in this study. These symptoms might serve as possible targets in developing preventive strategies and treatments for MPHs with excessive fear of COVID-19. Future research should prioritize early screening of somatic symptoms, mental health stigma among MHPs and negative impact of social media to address the fear of COVID-19.
Data availability statement
The datasets presented in this article are not readily available because The Ethics Committee of Beijing Anding Hospital in China that approved the study prohibits the authors from making publicly available the research dataset of clinical studies. Requests to access the datasets should be directed to xyutly@gmail.com.
Ethics statement
The studies involving humans were approved by The Ethics Committee of the Beijing Anding Hospital in China. The studies were conducted in accordance with the local legislation and institutional requirements. The participants provided their written informed consent to participate in this study.
Author contributions
ML: Data curation, Formal Analysis, Methodology, Writing – original draft. PC: Data curation, Methodology, Writing – review & editing. QZ: Methodology, Writing – review & editing. SS: Methodology, Writing – review & editing. F-RA: Methodology, Writing – review & editing. ZS: Data curation, Writing – review & editing. TC: Data curation, Writing – review & editing. GU: Data curation, Writing – review & editing. CN: Writing – review & editing. Y-TX: Methodology, Writing – original draft, Writing – review & editing. YF: Methodology, Writing – review & editing.
Funding
The author(s) declare financial support was received for the research, authorship, and/or publication of this article. The study was supported by Beijing High Level Public Health Technology Talent Construction Project (Discipline Backbone-01-028 & Discipline Backbones-02-38) and the University of Macau (MYRG2019-00066-FHS; MYRG2022-00187-FHS).
Acknowledgments
The authors are grateful to all participants and clinicians involved in this study.
Conflict of interest
The authors declare that the research was conducted in the absence of any commercial or financial relationships that could be construed as a potential conflict of interest.
Publisher’s note
All claims expressed in this article are solely those of the authors and do not necessarily represent those of their affiliated organizations, or those of the publisher, the editors and the reviewers. Any product that may be evaluated in this article, or claim that may be made by its manufacturer, is not guaranteed or endorsed by the publisher.
Supplementary material
The Supplementary material for this article can be found online at: https://www.frontiersin.org/articles/10.3389/fpubh.2023.1280688/full#supplementary-material
SUPPLEMENTARY FIGURE S1 | Bootstrapped confidence intervals of edge weights.
SUPPLEMENTARY FIGURE S2 | Estimation of edge weight difference by bootstrapped difference test.
SUPPLEMENTARY TABLE S1 | Descriptive information and network centrality indices of COVID-19 fear.
SUPPLEMENTARY TABLE S2 | Correlation matrix of the FCV-19S items.
References
1. World Health Organization (2020). Rolling updates on coronavirus disease (COVID-19). Available at: https://www.who.int/emergencies/diseases/novel-coronavirus-2019/events-as-they-happen
2. World Health Organization (2020). Mental health and psychosocial considerations during the COVID-19 outbreak. Available at: https://www.who.int/docs/default-source/coronaviruse/mental-health-considerations.pdf
3. Grover, S, Dua, D, Sahoo, S, Mehra, A, Nehra, R, and Chakrabarti, S. Why all COVID-19 hospitals should have mental health professionals: the importance of mental health in a worldwide crisis! Asian J Psychiatr. (2020) 51:102147. doi: 10.1016/j.ajp.2020.102147
4. Ahorsu, DK, Lin, CY, Imani, V, Saffari, M, Griffiths, MD, and Pakpour, AH. The fear of COVID-19 scale: development and initial validation. Int J Ment Heal Addict. (2020) 20:1537–45. doi: 10.1007/s11469-020-00270-8
5. Chakraborty, N. The COVID -19 pandemic and its impact on mental health. Prog Neurol Psychiatry. (2020) 24:21–4. doi: 10.1002/pnp.666
6. Dubey, S, Biswas, P, Ghosh, R, Chatterjee, S, Dubey, MJ, Chatterjee, S, et al. Psychosocial impact of COVID-19. Diabetes Metab Syndr. (2020) 14:779–88. doi: 10.1016/j.dsx.2020.05.035
7. Alimoradi, Z, Ohayon, MM, Griffiths, MD, Lin, CY, and Pakpour, AH. Fear of COVID-19 and its association with mental health-related factors: systematic review and meta-analysis. BJPsych Open. (2022) 8:e73. doi: 10.1192/bjo.2022.26
8. Quadros, S, Garg, S, Ranjan, R, Vijayasarathi, G, and Mamun, MA. Fear of COVID 19 infection across different cohorts: a scoping review. Front Psychol. (2021) 12:708430. doi: 10.3389/fpsyt.2021.708430
9. Cabarkapa, S, Nadjidai, SE, Murgier, J, and Ng, CH. The psychological impact of COVID-19 and other viral epidemics on frontline healthcare workers and ways to address it: a rapid systematic review. Brain Behav Immun Health. (2020) 8:100144. doi: 10.1016/j.bbih.2020.100144
10. de los Santos, JAA, and Labrague, LJ. The impact of fear of COVID-19 on job stress, and turnover intentions of frontline nurses in the community: a cross-sectional study in the Philippines. Traumatology. (2021) 27:52–9. doi: 10.1037/trm0000294
11. Alnazly, E, Khraisat, OM, Al-Bashaireh, AM, and Bryant, CL. Anxiety, depression, stress, fear and social support during COVID-19 pandemic among Jordanian healthcare workers. PLoS One. (2021) 16:e0247679. doi: 10.1371/journal.pone.0247679
12. Luo, F, Ghanei Gheshlagh, R, Dalvand, S, Saedmoucheshi, S, and Li, Q. Systematic review and Meta-analysis of fear of COVID-19. Front Psychol. (2021) 12:661078. doi: 10.3389/fpsyg.2021.661078
13. Stefanatou, P, Xenaki, LA, Karagiorgas, I, Ntigrintaki, AA, Giannouli, E, Malogiannis, IA, et al. Fear of COVID-19 impact on professional quality of life among mental health workers. Int J Environ Res Public Health. (2022) 19:9949. doi: 10.3390/ijerph19169949
14. Simsir, Z, Koc, H, Seki, T, and Griffiths, MD. The relationship between fear of COVID-19 and mental health problems: a meta-analysis. Death Stud. (2022) 46:515–23. doi: 10.1080/07481187.2021.1889097
15. Demirbas, N, and Kutlu, R. Effects of COVID-19 fear on Society's quality of life. Int J Ment Heal Addict. (2022) 20:2813–22. doi: 10.1007/s11469-021-00550-x
16. Kontodimopoulos, N, Poulaki, E, Fanourgiakis, J, and Talias, MA. The association between fear of COVID-19 and health-related quality of life: a cross-sectional study in the Greek general population. J Pers Med. (2022) 12:1891. doi: 10.3390/jpm12111891
17. Ahorsu, DK, Lin, CY, and Marznaki, ZH. The association between fear of COVID-19 and mental health: the mediating roles of burnout and job stress among emergency nursing staff. Nurs Open. (2022) 9:1147–54. doi: 10.1002/nop2.1154
18. Hu, D, Kong, Y, Li, W, Han, Q, Zhang, X, Zhu, LX, et al. Frontline nurses' burnout, anxiety, depression, and fear statuses and their associated factors during the COVID-19 outbreak in Wuhan, China: a large-scale cross-sectional study. EClinicalMedicine. (2020) 24:100424. doi: 10.1016/j.eclinm.2020.100424
19. Khattak, SR, Saeed, I, Rehman, SU, and Fayaz, M. Impact of fear of COVID-19 pandemic on the mental health of nurses in Pakistan. J Loss Trauma. (2020) 26:421–35. doi: 10.1080/15325024.2020.1814580
20. Labrague, LJ, and de Los Santos, JAA. Fear of COVID-19, psychological distress, work satisfaction and turnover intention among frontline nurses. J Nurs Manag. (2021) 29:395–403. doi: 10.1111/jonm.13168
21. Fitzpatrick, KM, Drawve, G, and Harris, C. Facing new fears during the COVID-19 pandemic: the state of America's mental health. J Anxiety Disord. (2020) 75:102291. doi: 10.1016/j.janxdis.2020.102291
22. Bai, W, Sha, S, Cheung, T, Su, Z, Jackson, T, and Xiang, YT. Optimizing the dynamic zero-COVID policy in China. Int J Biol Sci. (2022) 18:5314–6. doi: 10.7150/ijbs.75699
23. Suzuki, R, Yamasoba, D, Kimura, I, Wang, L, Kishimoto, M, Ito, J, et al. Attenuated fusogenicity and pathogenicity of SARS-CoV-2 omicron variant. Nature. (2022) 603:700–5. doi: 10.1038/s41586-022-04462-1
24. The Lancet Regional Health-Western P. The end of zero-COVID-19 policy is not the end of COVID-19 for China. Lancet Reg Health West Pac. (2023) 30:100702. doi: 10.1016/j.lanwpc.2023.100702
25. Araf, Y, Akter, F, Tang, YD, Fatemi, R, Parvez, MSA, Zheng, C, et al. Omicron variant of SARS-CoV-2: genomics, transmissibility, and responses to current COVID-19 vaccines. J Med Virol. (2022) 94:1825–32. doi: 10.1002/jmv.27588
26. Zheng, L, Liu, S, and Lu, F. Impact of National Omicron Outbreak at the end of 2022 on the future outlook of COVID-19 in China. Emerg Microbes Infect. (2023) 12:2191738. doi: 10.1080/22221751.2023.2191738
27. Burki, T. Moving away from zero COVID in China. Lancet Respir Med. (2023) 11:132. doi: 10.1016/S2213-2600(22)00508-2
28. Sher, L. The impact of the COVID-19 pandemic on suicide rates. QJM. (2020) 113:707–12. doi: 10.1093/qjmed/hcaa202
29. Liberati, E, Richards, N, Willars, J, Scott, D, Boydell, N, Parker, J, et al. A qualitative study of experiences of NHS mental healthcare workers during the Covid-19 pandemic. BMC Psychiatry. (2021) 21:250. doi: 10.1186/s12888-021-03261-8
30. Grover, S, Mehra, A, Sahoo, S, Avasthi, A, Tripathi, A, D'Souza, A, et al. State of mental health services in various training centers in India during the lockdown and COVID-19 pandemic. Indian J Psychiatry. (2020) 62:363–9. doi: 10.4103/psychiatry.IndianJPsychiatry_567_20
31. Li, W, Yang, Y, Liu, ZH, Zhao, YJ, Zhang, Q, Zhang, L, et al. Progression of mental health services during the COVID-19 outbreak in China. Int J Biol Sci. (2020) 16:1732–8. doi: 10.7150/ijbs.45120
32. Bell, V, and Wade, D. Mental health of clinical staff working in high-risk epidemic and pandemic health emergencies a rapid review of the evidence and living meta-analysis. Soc Psychiatry Psychiatr Epidemiol. (2021) 56:1–11. doi: 10.1007/s00127-020-01990-x
33. Gonzalez-Nuevo, C, Postigo, A, Gonzalez-Menendez, A, Alonso-Perez, F, Cuesta, M, and Gonzalez-Pando, D. Professional quality of life and fear of COVID-19 among Spanish nurses: a longitudinal repeated cross-sectional study. J Clin Nurs. (2023). doi: 10.1111/jocn.16688
34. Centers for Disease Control and Prevention (2018). Health-related quality of life (HRQOL). Available at: https://www.cdc.gov/hrqol/concept.htm.
35. Felce, D, and Perry, J. Quality of life: its definition and measurement. Res Dev Disabil. (1995) 16:51–74. doi: 10.1016/0891-4222(94)00028-8
36. Guyatt, GH, Feeny, DH, and Patrick, DL. Measuring health-related quality of life. Ann Intern Med. (1993) 118:622–9. doi: 10.7326/0003-4819-118-8-199304150-00009
37. Fisher, B, Costanza, R, Ali, S, Beer, C, Bond, L, Boumans, R, et al. An integrative approach to quality of life measurement. Res Policy Sap. (2008) 1:11–15. doi: 10.5194/sapiens-1-11-2008
38. Borsboom, D, and Cramer, AO. Network analysis: an integrative approach to the structure of psychopathology. Annu Rev Clin Psychol. (2013) 9:91–121. doi: 10.1146/annurev-clinpsy-050212-185608
39. Hofmann, SG, Curtiss, J, and McNally, RJ. A complex network perspective on clinical science. Perspect Psychol Sci. (2016) 11:597–605. doi: 10.1177/1745691616639283
40. Fried, EI, Epskamp, S, Nesse, RM, Tuerlinckx, F, and Borsboom, D. What are 'good' depression symptoms? Comparing the centrality of DSM and non-DSM symptoms of depression in a network analysis. J Affect Disord. (2016) 189:314–20. doi: 10.1016/j.jad.2015.09.005
41. Hevey, D. Network analysis: a brief overview and tutorial. Health Psychol Behav Med. (2018) 6:301–28. doi: 10.1080/21642850.2018.1521283
42. Borsboom, D. A network theory of mental disorders. World Psychiatry. (2017) 16:5–13. doi: 10.1002/wps.20375
43. Cai, H, Chow, IHI, Lei, S-M, Lok, GKI, Su, Z, Cheung, T, et al. Inter-relationships of depressive and anxiety symptoms with suicidality among adolescents: A network perspective. J Affect Disord. (2023) 324:480–488. doi: 10.1016/j.jad.2022.12.093
44. Chen, P, Zhang, L, Sha, S, Lam, MI, Lok, KI, Chow, IHI, et al. Prevalence of insomnia and its association with quality of life among Macau residents shortly after the summer 2022 COVID-19 outbreak: A network analysis perspective. Front Psychol. (2023) 14:1113122. doi: 10.3389/fpsyt.2023.1113122
45. Liu, J, Hao, J, Sun, Y, and Shi, Z. Network analysis of population flow among major cities and its influence on COVID-19 transmission in China. Cities. (2021) 112:103138. doi: 10.1016/j.cities.2021.103138
46. Lecuona, O, Lin, CY, Rozgonjuk, D, Norekval, TM, Iversen, MM, Mamun, MA, et al. A network analysis of the fear of COVID-19 scale (FCV-19S): A large-scale Cross-cultural study in Iran, Bangladesh, and Norway. Int J Environ Res Public Health. (2022) 19:6824. doi: 10.3390/ijerph19116824
47. Jin, Y, Sha, S, Tian, T, Wang, Q, Liang, S, Wang, Z, et al. Network analysis of comorbid depression and anxiety and their associations with quality of life among clinicians in public hospitals during the late stage of the COVID-19 pandemic in China. J Affect Disord. (2022) 314:193–200. doi: 10.1016/j.jad.2022.06.051
48. An, Y, Yang, Y, Wang, A, Li, Y, Zhang, Q, Cheung, T, et al. Prevalence of depression and its impact on quality of life among frontline nurses in emergency departments during the COVID-19 outbreak. J Affect Disord. (2020) 276:312–5. doi: 10.1016/j.jad.2020.06.047
49. Shen, YJ, Wei, L, Li, Q, Li, LQ, and Zhang, XH. Mental health and social support among nurses during the COVID-19 pandemic. Psychol Health Med. (2022) 27:444–52. doi: 10.1080/13548506.2021.1944653
50. Li, Y, Cheng, XH, Xu, WT, Tan, LP, and Gou, XJ. A WeChat-based Mobile platform for perioperative health education for gastrointestinal surgery. Emerg Med Int. (2021) 2021:6566981. doi: 10.1155/2021/6566981
51. Luan, H, Wang, M, Sokol, RL, Wu, S, Victor, BG, and Perron, BE. A scoping review of WeChat to facilitate professional healthcare education in mainland China. Med Educ Online. (2020) 25:1782594. doi: 10.1080/10872981.2020.1782594
52. Yang, Y, Li, Y, An, Y, Zhao, YJ, Zhang, L, Cheung, T, et al. Workplace violence against Chinese frontline clinicians during the COVID-19 pandemic and its associations with demographic and clinical characteristics and quality of life: a structural equation modeling investigation. Front Psychol. (2021) 12:649989. doi: 10.3389/fpsyt.2021.649989
53. Luo, H, Lie, Y, and Prinzen, FW. Surveillance of COVID-19 in the general population using an online questionnaire: report from 18,161 respondents in China. JMIR Public Health Surveill. (2020) 6:e18576. doi: 10.2196/18576
54. Chi, X, Chen, S, Chen, Y, Chen, D, Yu, Q, Guo, T, et al. Psychometric evaluation of the fear of COVID-19 scale among Chinese population. Int J Ment Heal Addict. (2022) 20:1273–88. doi: 10.1007/s11469-020-00441-7
55. Feng, QY, Huang, CW, Jia, YP, Liu, T, Jia, HY, Wang, KC, et al. Reliability and validity of the Chinese version of fear of coronavirus disease 2019 scale. Acad J Second Mil Univ. (2021) 42:778–82.
56. The WHOQOL Group. Development of the World Health Organization WHOQOL-BREF quality of life assessment. Psychol Med. (1998) 28:551–8. doi: 10.1017/S0033291798006667
57. Cheung, YB, Yeo, KK, Chong, KJ, Khoo, EYH, and Wee, HL. Measurement equivalence of the English, Chinese and Malay versions of the World Health Organization quality of life (WHOQOL-BREF) questionnaires. Health Qual Life Outcomes. (2019) 17:67. doi: 10.1186/s12955-019-1130-0
58. Xia, P, Li, N, Hau, KT, Liu, C, and Lu, Y. Quality of life of Chinese urban community residents: a psychometric study of the mainland Chinese version of the WHOQOL-BREF. BMC Med Res Methodol. (2012) 12:37. doi: 10.1186/1471-2288-12-37
59. Yang, S-C, Kuo, P-W, Su, S, Wang, J-D, and Lin, M-l. Development and psychometric properties of the Dialysis module of the WHOQOL-BREF Taiwan version. J Formos Med Assoc. (2006) 105:299–309. doi: 10.1016/S0929-6646(09)60121-2
60. R Core Team (2020). R: A language and environment for statistical computing. R Foundation for Statistical Computing, Vienna, Austria.
61. Epskamp, S, Borsboom, D, and Fried, EI. Estimating psychological networks and their accuracy: a tutorial paper. Behav Res Methods. (2018) 50:195–212. doi: 10.3758/s13428-017-0862-1
62. Yang, Y, Zhang, DY, Li, YL, Zhang, M, Wang, PH, Liu, XH, et al. Prevalence, correlates, and network analysis of internet addiction symptoms among Chinese pregnant and postpartum women. J Affect Disord. (2022) 298:126–33. doi: 10.1016/j.jad.2021.10.092
63. Epskamp, S, Cramer, AOJ, Waldorp, LJ, Schmittmann, VD, and Borsboom, D. Qgraph: network visualizations of relationships in psychometric data. J Stat Softw. (2012) 48:1–18. doi: 10.18637/jss.v048.i04
64. Villanueva, RAM, and Chen, ZJ. ggplot2: Elegant graphics for data analysis. Measur Interdiscip Res Perspect. (2019) 17:160–7. doi: 10.1080/15366367.2019.1565254
65. Robinaugh, DJ, Millner, AJ, and McNally, RJ. Identifying highly influential nodes in the complicated grief network. J Abnorm Psychol. (2016) 125:747–57. doi: 10.1037/abn0000181
66. Bai, W, Zhao, YJ, Cai, H, Sha, S, Zhang, Q, Lei, SM, et al. Network analysis of depression, anxiety, insomnia and quality of life among Macau residents during the COVID-19 pandemic. J Affect Disord. (2022) 311:181–8. doi: 10.1016/j.jad.2022.05.061
67. Haslbeck, JMB, and Waldorp, LJ. How well do network models predict observations? On the importance of predictability in network models. Behav Res Methods. (2018) 50:853–61. doi: 10.3758/s13428-017-0910-x
68. Sakib, N, Akter, T, Zohra, F, Bhuiyan, A, Mamun, MA, and Griffiths, MD. Fear of COVID-19 and depression: a comparative study among the general population and healthcare professionals during COVID-19 pandemic crisis in Bangladesh. Int J Ment Heal Addict. (2023) 21:976–92. doi: 10.1007/s11469-020-00477-9
69. Rahman, MA, Hoque, N, Alif, SM, Salehin, M, Islam, SMS, Banik, B, et al. Factors associated with psychological distress, fear and coping strategies during the COVID-19 pandemic in Australia. Glob Health. (2020) 16:95. doi: 10.1186/s12992-020-00624-w
70. Doshi, D, Karunakar, P, Sukhabogi, JR, Prasanna, JS, and Mahajan, SV. Assessing coronavirus fear in Indian population using the fear of COVID-19 scale. Int J Ment Heal Addict. (2021) 19:2383–91. doi: 10.1007/s11469-020-00332-x
71. Lai, J, Ma, S, Wang, Y, Cai, Z, Hu, J, Wei, N, et al. Factors associated with mental health outcomes among health care workers exposed to coronavirus disease 2019. JAMA Netw Open. (2020) 3:e203976. doi: 10.1001/jamanetworkopen.2020.3976
72. Paiano, M, Jaques, AE, Nacamura, PAB, Salci, MA, Radovanovic, CAT, and Carreira, L. Mental health of healthcare professionals in China during the new coronavirus pandemic: an integrative review. Rev Bras Enferm. (2020) 73:e20200338. doi: 10.1590/0034-7167-2020-0338
73. Billings, J, Ching, BCF, Gkofa, V, Greene, T, and Bloomfield, M. Experiences of frontline healthcare workers and their views about support during COVID-19 and previous pandemics: a systematic review and qualitative meta-synthesis. BMC Health Serv Res. (2021) 21:923. doi: 10.1186/s12913-021-06917-z
74. El-Hage, W, Hingray, C, Lemogne, C, Yrondi, A, Brunault, P, Bienvenu, T, et al. Health professionals facing the coronavirus disease 2019 (COVID-19) pandemic: what are the mental health risks? L'Encéphale. (2020) 46:S73–80. doi: 10.1016/j.encep.2020.04.008
75. Khan, KS, Mamun, MA, Griffiths, MD, and Ullah, I. The mental health impact of the COVID-19 pandemic across different cohorts. Int J Ment Heal Addict. (2022) 20:380–6. doi: 10.1007/s11469-020-00367-0
76. Moretti, M, De Geyter, D, Van Cutsem, E, Van Laere, S, Pierard, D, and Allard, SD. Fear for CoViD-19 and reluctance to work among health care workers during the epidemic, a prospective monocentric cohort study. Am J Infect Control. (2022) 50:312–8. doi: 10.1016/j.ajic.2021.10.042
77. Harper, CA, Satchell, LP, Fido, D, and Latzman, RD. Functional fear predicts public health compliance in the COVID-19 pandemic. Int J Ment Heal Addict. (2021) 19:1875–88. doi: 10.1007/s11469-020-00281-5
78. Ho, CS, Chee, CY, and Ho, RC. Mental health strategies to combat the psychological impact of coronavirus disease 2019 (COVID-19) beyond paranoia and panic. Ann Acad Med Singap. (2020) 49:155–60. doi: 10.47102/annals-acadmedsg.202043
79. Scottie, A. (2020). The psychology behind why toilet paper, of all things, is the latest coronavirus panic buy by Scottie Andrew, CNN. Available at: https://edition.cnn.com/2020/03/09/health/toilet-paper-shortages-novel-coronavirus-trnd/index.html
80. Kumar, J, Katto, MS, Siddiqui, AA, Sahito, B, Ahmed, B, Jamil, M, et al. Predictive factors associated with fear faced by healthcare workers during COVID-19 pandemic: A questionnaire-based study. Cureus. (2020) 12:e9741. doi: 10.7759/cureus.9741
81. Alharthi, MH, Alshomrani, AT, Bazaid, K, Sonpol, HMA, Ibrahim, IAE, and Alashkar, AM. Factors affecting the psychological well-being of health care workers during the COVID-19 crisis. Psychol Res Behav Manag. (2022) 15:1931–42. doi: 10.2147/PRBM.S370456
82. Ahmed, MA, Jouhar, R, Ahmed, N, Adnan, S, Aftab, M, Zafar, MS, et al. Fear and practice modifications among dentists to combat novel coronavirus disease (COVID-19) outbreak. Int J Environ Res Public Health. (2020) 17:2821. doi: 10.3390/ijerph17082821
83. Juan, Y, Yuanyuan, C, Qiuxiang, Y, Cong, L, Xiaofeng, L, Yundong, Z, et al. Psychological distress surveillance and related impact analysis of hospital staff during the COVID-19 epidemic in Chongqing. China Compr Psychiatry. (2020) 103:152198. doi: 10.1016/j.comppsych.2020.152198
84. Sugawara, D, Masuyama, A, and Kubo, T. Socioeconomic impacts of the COVID-19 lockdown on the mental health and life satisfaction of the Japanese population. Int J Ment Heal Addict. (2022) 20:1560–74. doi: 10.1007/s11469-020-00461-3
85. Sanyaolu, A, Okorie, C, Marinkovic, A, Patidar, R, Younis, K, Desai, P, et al. Comorbidity and its impact on patients with COVID-19. SN Compr Clin Med. (2020) 2:1069–76. doi: 10.1007/s42399-020-00363-4
86. Chalhoub, Z, Koubeissy, H, Fares, Y, and Abou-Abbas, L. Fear and death anxiety in the shadow of COVID-19 among the Lebanese population: a cross-sectional study. PLoS One. (2022) 17:e0270567. doi: 10.1371/journal.pone.0270567
87. Couto, RN, Medeiros, ED, Sousa, ÍM, Medeiros, PCB, and Carvalho, TA. Fear and anxiety in professionals about COVID-19: Brazilian versions of measures. Estud Psicol. (2022):39. doi: 10.1590/1982-0275202239200136
88. Vilca, LW, Chávez, BV, Fernández, YS, Caycho-Rodríguez, T, and White, M. Impact of the fear of catching COVID-19 on mental health in undergraduate students: a predictive model for anxiety, depression, and insomnia. Curr Psychol. (2022). doi: 10.1007/s12144-021-02542-5
89. Huang, B, Yan, H, Hu, L, Cao, G, Wang, G, Meng, J, et al. The contribution of psychological distress to resting palpitations in patients who recovered from severe COVID-19. Int J Gen Med. (2021) 14:9371–8. doi: 10.2147/ijgm.S334715
90. Oliván-Blázquez, B, Rubio, E, García-Sanz, O, and Magallón-Botaya, R. Correlation between diagnosis of depression and symptoms present in primary care patients. Actas Esp Psiquiatr. (2016) 44:55–63.
91. Hao, Q, Wang, D, Xie, M, Tang, Y, Dou, Y, Zhu, L, et al. Prevalence and risk factors of mental health problems among healthcare workers during the COVID-19 pandemic: a systematic review and meta-analysis. Front Psychol. (2021) 12:567381. doi: 10.3389/fpsyt.2021.567381
92. Pappa, S, Barnett, J, Berges, I, and Sakkas, N. Tired, worried and burned out, but still resilient: a cross-sectional study of mental health workers in the UK during the COVID-19 pandemic. Int J Environ Res Public Health. (2021) 18:4457. doi: 10.3390/ijerph18094457
93. Stojanov, J, Malobabic, M, Stanojevic, G, Stevic, M, Milosevic, V, and Stojanov, A. Quality of sleep and health-related quality of life among health care professionals treating patients with coronavirus disease-19. Int J Soc Psychiatry. (2021) 67:175–81. doi: 10.1177/0020764020942800
94. Zhang, L, Fritzsche, K, Liu, Y, Wang, J, Huang, M, Wang, Y, et al. Validation of the Chinese version of the PHQ-15 in a tertiary hospital. BMC Psychiatry. (2016) 16:89. doi: 10.1186/s12888-016-0798-5
95. Yan, H, Ding, Y, and Guo, W. Mental health of medical staff during the coronavirus disease 2019 pandemic: A systematic review and Meta-analysis. Psychosom Med. (2021) 83:387–96. doi: 10.1097/PSY.0000000000000922
96. Colizzi, M, Bortoletto, R, Silvestri, M, Mondini, F, Puttini, E, Cainelli, C, et al. Medically unexplained symptoms in the times of COVID-19 pandemic: A case-report. Brain Behav Immun Health. (2020) 5:100073. doi: 10.1016/j.bbih.2020.100073
97. Simon, GE, VonKorff, M, Piccinelli, M, Fullerton, C, and Ormel, J. An international study of the relation between somatic symptoms and depression. N Engl J Med. (1999) 341:1329–35. doi: 10.1056/NEJM199910283411801
98. Kleinman, AM. Depression, somatization and the "new cross-cultural psychiatry". Soc Sci Med. (1977) 11:3–10. doi: 10.1016/0037-7856(77)90138-x
99. Ryder, AG, and Chentsova-Dutton, YE. Depression in cultural context: “Chinese somatization,” revisited. Psychiatr Clin N Am. (2012) 35:15–36. doi: 10.1016/j.psc.2011.11.006
100. Zarocostas, J. How to fight an infodemic. Lancet. (2020) 395:676. doi: 10.1016/S0140-6736(20)30461-X
101. Kumar, A, and Nayar, KR. COVID 19 and its mental health consequences. J Ment Health. (2021) 30:1–2. doi: 10.1080/09638237.2020.1757052
102. Asmundson, GJG, and Taylor, S. Coronaphobia: fear and the 2019-nCoV outbreak. J Anxiety Disord. (2020) 70:102196. doi: 10.1016/j.janxdis.2020.102196
103. Rocha, YM, de Moura, GA, Desidério, GA, de Oliveira, CH, Lourenço, FD, and de Figueiredo Nicolete, LD. The impact of fake news on social media and its influence on health during the COVID-19 pandemic: a systematic review. J Public Health. (2023) 31:1007–1016. doi: 10.1007/s10389-021-01658-z
104. Suman, S, Tomar, B, Singh, P, Raj, P, and Nathiya, D. Mental health outcome and professional quality of life among healthcare workers during COVID-19 pandemic: a frontline-COVID survey. Hamdan Med J. (2020) 13:196–202. doi: 10.4103/HMJ.HMJ_53_20
105. Solymosi, R, Jackson, J, Posch, K, Yesberg, JA, Bradford, B, and Kyprianides, A. Functional and dysfunctional fear of COVID-19: a classification scheme. Crime Sci. (2021) 10:4. doi: 10.1186/s40163-020-00137-2
106. Hyphantis, TN, Tomenson, B, Bai, M, Tsianos, E, Mavreas, V, and Creed, F. Psychological distress, somatization, and defense mechanisms associated with quality of life in inflammatory bowel disease patients. Dig Dis Sci. (2009) 55:724–32. doi: 10.1007/s10620-009-0762-z
107. Pappas, G, Kiriaze, IJ, Giannakis, P, and Falagas, ME. Psychosocial consequences of infectious diseases. Clin Microbiol Infect. (2009) 15:743–7. doi: 10.1111/j.1469-0691.2009.02947.x
108. Secer, I, Ulas, S, and Karaman-Ozlu, Z. The effect of the fear of COVID-19 on healthcare professionals' psychological adjustment skills: mediating role of experiential avoidance and psychological resilience. Front Psychol. (2020) 11:561536. doi: 10.3389/fpsyg.2020.561536
109. Sfeir, M, Akel, M, Hallit, S, and Obeid, S. Factors associated with general well-being among Lebanese adults: the role of emotional intelligence, fear of COVID, healthy lifestyle, coping strategies (avoidance and approach). Curr Psychol (2022) doi: 10.1007/s12144-021-02549-y [Epub ahead of print].
110. Eustis, EH, Cardona, N, Nauphal, M, Sauer-Zavala, S, Rosellini, AJ, Farchione, TJ, et al. Experiential avoidance as a mechanism of change across cognitive-behavioral therapy in a sample of participants with heterogeneous anxiety disorders. Cogn Ther Res. (2019) 44:275–86. doi: 10.1007/s10608-019-10063-6
111. Yoo, IG. The effects of the type of delivery of cognitive-behavioral therapy for healthcare workers: a systematic review. J Clin Psychol. (2022) 78:149–66. doi: 10.1002/jclp.23215
Keywords: fear, quality of life, COVID-19, mental health professionals, network analysis
Citation: Lam MI, Chen P, Zhang Q, Sha S, An F-R, Su Z, Cheung T, Ungvari GS, Ng CH, Xiang Y-T and Feng Y (2023) Prevalence of COVID-19 fear and its association with quality of life and network structure among Chinese mental health professionals after ending China’s dynamic zero-COVID policy: a national survey. Front. Public Health. 11:1280688. doi: 10.3389/fpubh.2023.1280688
Edited by:
Renato de Filippis, University Magna Graecia of Catanzaro, ItalyReviewed by:
Yuna Koyama, Tokyo Medical and Dental University, JapanHsueh-Han Yeh, Henry Ford Health System, United States
Copyright © 2023 Lam, Chen, Zhang, Sha, An, Su, Cheung, Ungvari, Ng, Xiang and Feng. This is an open-access article distributed under the terms of the Creative Commons Attribution License (CC BY). The use, distribution or reproduction in other forums is permitted, provided the original author(s) and the copyright owner(s) are credited and that the original publication in this journal is cited, in accordance with accepted academic practice. No use, distribution or reproduction is permitted which does not comply with these terms.
*Correspondence: Yu-Tao Xiang, xyutly@gmail.com; Yuan Feng, 19558051@qq.com; Chee H. Ng, cng@unimelb.edu.au
†These authors have contributed equally to this work