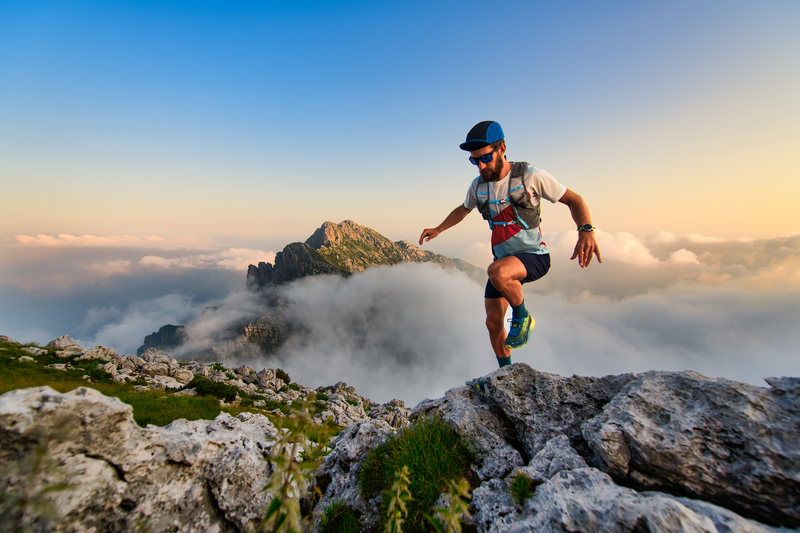
94% of researchers rate our articles as excellent or good
Learn more about the work of our research integrity team to safeguard the quality of each article we publish.
Find out more
ORIGINAL RESEARCH article
Front. Public Health , 23 November 2023
Sec. Substance Use Disorders and Behavioral Addictions
Volume 11 - 2023 | https://doi.org/10.3389/fpubh.2023.1275975
This article is part of the Research Topic Computational Methods in Substance Use and Addiction Research View all 4 articles
Introduction: Substances and the people who use them have been dehumanized for decades. As a result, lawmakers and healthcare providers have implemented policies that subjected millions to criminalization, incarceration, and inadequate resources to support health and wellbeing. While there have been recent shifts in public opinion on issues such as legalization, in the case of marijuana in the U.S., or addiction as a disease, dehumanization and stigma are still leading barriers for individuals seeking treatment. Integral to the narrative of “substance users” as thoughtless zombies or violent criminals is their portrayal in popular media, such as films and news.
Methods: This study attempts to quantify the dehumanization of people who use substances (PWUS) across time using a large corpus of over 3 million news articles. We apply a computational linguistic framework for measuring dehumanization across three decades of New York Times articles.
Results: We show that (1) levels of dehumanization remain high and (2) while marijuana has become less dehumanized over time, attitudes toward other substances such as heroin and cocaine remain stable.
Discussion: This work highlights the importance of a holistic view of substance use that places all substances within the context of addiction as a disease, prioritizes the humanization of PWUS, and centers around harm reduction.
Defined as the treatment or perception of individuals as less than human (1), dehumanization can cause harm in various contexts. Although direct consequences are often difficult to draw out, dehumanization has been shown to feed intergroup bias, abusive language, and violence (1, 2), such as in the cases of dehumanizing media campaigns against Jewish people in Nazi Germany, Rwandan Tutsis, and Arab leaders post-9/11 (3, 4). Moreover, dehumanization of patients with physical and mental health conditions has been documented extensively (1, 5–7). Most famously, people with a substance use disorder (SUD) and more broadly people who use substances (PWUS) were dehumanized throughout the War on Drugs from the 1970s to today with varying degrees of severity based on demographics and the type of drug (8). The War on Drugs, in particular, embedded dehumanizing policies into both the criminal justice and healthcare systems, dramatically increasing incarceration and negatively impacting the health and well-being of communities (9). Since the dehumanization of PWUS contributes to stigma, it is thus associated with lower support for non-discriminatory drug laws (10), inhibited help-seeking behavior (11, 12), and worse health outcomes (13). Understanding historical patterns of dehumanization and consequently discovering mitigation protocols are significant public health and public policy concerns.
Language is a key component of dehumanization. The lexicon for health problems, particularly SUDs, both reveals and affects societal responses and treatment strategies (14). Several studies have shown that dehumanizing labels induce and perpetuate explicit and implicit biases among the general public and well-trained health professionals (15–17). For instance, McGinty et al. (18) finds that stigmatizing language in American print and television news platforms about the opioid epidemic increased from 2008 to 2018, contributing to public stigma toward people with opioid use disorders. Moreover, Brown (19) argues that PWUS more effectively recognize potential harms if information is shared in a nonjudgmental way. The label of “substance abuser” conveys that the patient is the problem while “person with an SUD” conveys that the patient is not the problem but instead has a problem (17). Systematic approaches to changing the language of addiction have been spearheaded by addiction research journals and the American Society of Addiction Medicine (20, 21). While language changes more quickly in response to new information, Kelly et al. (14) shows that language evolution is slow and opts for more efficient terms, which poses barriers to adopting less dehumanizing language. Hence, it is imperative to detect, understand, and minimize dehumanizing language so people with SUDs have one less barrier to recovery (22).
Given the media's well-established role in dehumanizing social groups via sensationalist writing, Mendelsohn et al. (2) developed a computational linguistic framework for analyzing dehumanizing language. Focused on media portrayals of the LGBTQ community, their work represents the first large-scale quantitative analysis of dehumanization, allowing them to comprehensively capture media attitudes, track dehumanization over time, and capture previously untapped variations in language. Similar large-scale, multi-decade studies have measured gender and ethnic stereotypes (23), public perception of artificial intelligence (24), and the framing of immigration in political speeches (25), among others.
Based on the claim of Mendelsohn et al. (2) that the framework generalizes to other groups, we aim to apply their model to the dehumanization of PWUS. Although the dehumanization of PWUS has been well-studied, no project has quantified dehumanizing language about PWUS by American institutions across time. To do this, we use a multi-dimensional linguistic measure of dehumanization (which includes negative evaluations of a target group, denial of agency, moral disgust, and a vermin metaphor) to identify trends in dehumanization toward both PWUS (e.g., addict and alcoholic) and people who use specific substances (e.g., marijuana and heroin). This is measured across a data set of over 32 million New York Times articles from 1986 to 2020. This work contributes to our understanding of how American institutions and, in particular, mainstream media express attitudes toward marginalized populations. Furthermore, understanding these attitudes has implications for both policymakers and healthcare professionals as they respond to public health issues such as the opioid epidemic and emerging substances (26).
Following Mendelsohn et al. (2), we use a multi-dimensional measure of dehumanization which consists of Negative Evaluation of a Target Group, Denial of Agency, Moral Disgust, and Vermin as a Dehumanizing Metaphor, which are key elements of dehumanization (1, 27). Attributing negative characteristics to dehumanized groups contributes to moral exclusion, delegitimization, and psychological distancing (28, 29). Particularly effective at distancing an outgroup is equating members of the outgroup to nonhuman entities like vermin who are portrayed as threatening, thoughtless, and emotionless (30). As such, the outgroup is perceived as undeserving of the fair rules and moral values that apply to fellow humans, which leads to abuse and violence (2, 29). A key contributor to outgroup exclusion and the negative perception of its members in many dehumanizing metaphors is moral disgust (27, 31). Groups lacking sanctity and purity are perceived as mindless and thus allowed to be hurt (32). Indeed, dehumanization involves denying agency—the ability to control one's affective, behavioral, and cognitive states—to outgroup members (1, 30). Outgroup members are thought to be incapable of rational thought or controlling their actions and are thus excluded on the basis of lacking uniquely human traits (1). While dehumanization exaggerates intergroup differences by categorically distinguishing social groups (1), it can also take subtle, involuntary, and unconscious forms (33).
Previous work has brought attention to how American media coverage of individuals who use drugs has historically been and continues to be dehumanizing (34). Mass media partakes in what Reinarman and Duskin (35) call, “the routinization of caricature—rhetorically recrafting worst cases into typical cases, and profoundly distorting the nature of drug problems in the interest of dramatic stories.” Durham et al. (36) showed how newspapers and television historically paint misleading crime images. Similarly, Coomber et al. (37) showed that drug-related stories are particularly fraught with stereotypical images to increase viewership. For instance, Boyd et al. (38) explained how drug traffickers in movies assume the role of an out-of-control “outsider” who “threatens the world order of white, middle-class protestant morality.” Young et al. (39) argue that certain people, such as police officers, are particularly susceptible to believing in media stereotypes. Media influences public opinion and vice versa (40), and as Gentzkow and Shapiro (41) states, “news content has a powerful impact on politics, with ideologically diverse content producing socially desirable outcomes”. More than misleading, Murji (42) argues that the media can do harm by instigating drug crackdowns.
Computational linguistics and natural language processing methods have been used in several substance use related tasks. These include public perceptions of medical cannabis use (43), Reddit-based self-reported barriers to treatment seeking (12), and identifying emerging drug-related words and slang (44). At the population level (e.g., U.S. counties and states), a handful of studies have examined social media language and substance use rates, such as excessive drinking (45, 46), opioid mortality (47, 48), and, more generally, pharmacovigilance (49). Similar to dehumanization, there is a growing body of work focused on computational work identifying stigma toward people who use substances (50–52).
We use a corpus of 3.05 million New York Times articles spanning from 1986 to 2015, first collected by Fast and Horvitz (24) and used by Mendelsohn et al. (2) to assess dehumanization of LGBTQ people. We further supplement this data set with 229,235 more recent articles from 2016 to 2020. To collect this additional data, we first repeatedly queried the New York Times Archive API1 to list the metadata of all articles published for the years 2016–2020. This metadata included the URL of each article, which we used to download each article's full text. We then scraped the contents of these webpages using the Beautiful Soup Python package.2 Following Mendelsohn et al. (2), we retained articles related to news such as those coming from the World, Politics, Sports, Opinions, and Health sections and removed articles from the Arts and Movies sections as these are not typically news-related.
The final data set thus spanned from January 1986 to December 2020 and included 3.28 million articles, containing 39.6 million paragraphs, which can be further broken down into 96.9 million sentences. All articles are date stamped to allow for time-based collation.
In order to identify New York Times articles about substance use, we consider two classes of keywords: (1) keywords associated with PWUS and (2) keywords representing substances themselves, which we assume is a proxy for people using that specific substance. For the first class, we consider addict(s), addiction, alcoholic(s), and alcoholism.3 For the second class, we consider cocaine, heroin, marijuana, methamphetamine(s) , opioid(s), opiate(s), oxycontin, percocet, and xanax. Figure 1 shows the frequency of several keywords.4 Due to their low frequency, we excluded methamphetamine(s) , opioid(s), opiate(s), oxycontin, percocet, and xanax from further analysis. In particular, the terms opioid(s) did not become popular until roughly 2010, while the other terms (oxycontin, percocet, and xanax) remained rare across all decades.
Since the goal of the study is to understand attitudes toward people who use substances, a natural comparison group would be people who do not use substances. This comparison is done to contrast both overall levels of dehumanization and compare trends over time. Unfortunately, due to the keyword approach, there is no way to accurately identify this group as there are no common single words or phrases which refer to people who do not use substances. Thus, following Mendelsohn et al. (2), we compare the substance use keywords to the keyword american(s). Results from Mendelsohn et al. (2) showed that there was little change in dehumanization toward american(s) across time and, therefore, we will use these keywords to compare overall levels of dehumanization to the substance use keywords.
Here we use the computational framework outlined by Mendelsohn et al. (2) and, unless otherwise stated, use their recommended algorithmic settings.
Several of the methods developed by Mendelsohn et al. (2) rely on word embeddings (i.e., vector representations of words) to measure their semantic change across time. As such, we begin by examining the words closest in embedding (or semantic) space to our substance use keywords and how these neighbors change over time.
We begin by training a word embedding model over the entire New York Times corpus, using the word2vec skip-gram model (53). This is done via the Gensim software package (54) using all default parameters except that models are trained for five iterations and with a window size of 10 words. The resulting word embeddings are then used to initialize subsequent word2vec models trained for each year of the data (i.e., 35 separate word2vec models). This process is repeated 10 times and, for each year, results are averaged over the 10 models, in order to smooth out any randomness in each training.
Substance use keyword vectors are then created using a weighted average of all forms of the keyword. For example, vectors for addict and addicts are combined into a single vector by weighting individual vectors by their frequency. We then find each substance use keyword's closest neighbors in the yearly embedding space by computing the cosine distance between the keyword vector and all other vectors in the embedding space. We report the 10 words with the smallest average distance.
Negative evaluation of a target group is operationalized via three measures: paragraph-level valence, word embedding valence, and connotation frames of perspective. These methods are applied at the paragraph, word, and sentence level, respectively, in order to measure dehumanization across different lengths of context. Each measure is applied to yearly segments of the corpus from 1986 to 2020.
Valence is measured using the valence dimension of the NRC Valence, Arousal, and Dominance (VAD) lexicon (55). This lexicon contains 20,000 words with valence scores ranging from 0 (most negative valence) to 1 (most positive valence). The negative end of the lexicon contains words such as “shit,” “nightmare,” and “toxic,” while the positive end contains words such as “enjoyable,” “generous,” and “happy.” We then calculate the average valence of each paragraph, considering only paragraphs which contain a substance use keyword. Paragraphs are used as the unit of analysis in order to give more context to the substance use keywords (as opposed to sentences, for example).
This method measures valence at the word level by looking at the valence of the nearest neighbors to each substance use keyword. Similar to the methods outlined in Section 4.1, we identify the 500 closest words to each keyword by finding the minimal cosine distance across all words. We then assign each neighbor a valence score using the valence dimension of the NRC VAD lexicon, taking the average valence score across all 500 neighbors.
This method enables us to measure directed sentiment through a lexicon of 900 English verbs (56). Each verb is weighted to represent the writer's perspective toward the verb's subject and object. For example, the verb harm has a negative weight (−0.87) toward the subject (i.e., the person doing the harm) and a positive weight (0.20) toward the object (i.e., the receiver of the harm). We extract all subject-verb-object tuples containing at least one of the substance use keywords. This is done using spaCy's dependency parser.5 We then use the lexicon to measure the writer's perspective toward the keyword and average the verb weights over all tuples.
Denial of Agency is operationalized via two measures: connotation frames and word embedding dominance. These measure Denial of Agency at both the sentence and word level, respectively.
Here we use the same methods outlined in Section 4.2.3 but use a lexicon designed to measure agency (57). Similar to the Connotation Frames of Perspective, this lexicon consists of verbs used to measure agency between a subject and an object. Words such as “harm” and “fires” are labeled as high agency for the subject, whereas words such as “relishes” and “inherits” represent low agency. This lexicon uses binary scores for each verb, whereas the Connotation Frames of Perspective contained real-valued scores. Since we are interested in the agency of the individual using the substance, we only consider subject-verb-object tuples where the subject is a substance use keyword. Therefore, we calculate the fraction of subject-verb-object tuples where the subject has high agency.
We use the dominance dimension from the NRC VAD lexicon (55). This lexicon represents dominance via 20,000 English words, which are each weighted between 0 and 1. The highest-weighted words in the lexicon (representing high dominance) are “power,” “leadership,” and “success,” while the lowest-weighted words (representing low dominance) are “weak,” “frail,” and “empty.” To measure dominance, we use the same approach as outlined in Section 4.2.2. Specifically, we compute the average dominance of the 500 nearest neighbors for each substance use keyword.
To measure Moral Disgust, we use the sanctity/purity dimension of the Moral Foundations lexicon (58), taking the negative (or vice) end of this dimension. This dimension contains 46 words such as “disgust*,” “gross,” and “wretched*” (where an asterisk will match any word that, for example, begins with “disgust” such as “disgusting”). Unlike the NRC VAD lexicon, the words in the Moral Foundations lexicon are not weighted. Using this lexicon, we create a moral disgust vector by taking the average word2vec embedding of all words within the negative sanctity/purity dimension, weighting each embedding by the word's frequency. This is done for each year, resulting in 35 moral disgust vectors. We then calculate the yearly semantic similarity between the moral disgust vectors and each substance use keyword vector using a cosine similarity metric. A larger cosine similarity represents higher semantic similarity.
The association between substance use and vermin is measured by calculating the semantic similarity between the substance use keywords and vermin. First, we create a vermin vector by taking the average word2vec embedding for “bedbug(s),” “cockroach(es),” “fleas,” “rat(s),” “rodent(s),” termite(s),” and “vermin,” where each vector is weighted by its frequency. Then, for each year, we calculate the cosine similarity between the vermin vector and the substance use keyword vectors, where a larger value represents higher semantic similarity.
We end with two qualitative error analyses. First, we manually inspect the paragraphs with the highest and lowest valence scores to see if our methods correctly identify dehumanization. To do this, we identify paragraphs with the highest (≥0.7; i.e., lower dehumanization) and lowest ( ≤ 0.3; i.e., higher dehumanization) normalized valence scores from the NRC Valence lexicon (on a scale from 0 to 1). Valence is the average valence of the paragraph, and all paragraphs have a minimum of 15 words in order to provide a larger context to the keywords. To determine if the valence scores match the direction of dehumanization, we annotated each paragraph as being correctly labeled by the lexicon. In other words, we determined if each paragraph showed decreased dehumanization for high valence scores and increased dehumanization for low valence scores. Three authors separately annotated each paragraph as being correctly (valence score correctly matches the level of dehumanization) or incorrectly (valence score incorrectly matches the level of dehumanization) labeled by the lexicon, or not relevant (NR) when the paragraph is not referring to substances or substance use. The final labels of correct, incorrect, and NR were assigned by a majority vote across the three annotations.
Second, we note that the NRC Valence lexicon contains all of the keywords used in this study and several related words which reference substance use (e.g., methadone, lsd, and amphetamines). In particular, these words were all negatively valenced, and therefore any conversation around substance use will tend toward more dehumanization when measured with the NRC lexicon. Therefore, we measure paragraph-level sentiment using the positive emotions category in the 2015 version of the Linguistic Inquiry and Word Count (LIWC) dictionary, since this category does not contain substance-related words (59). LIWC is a manually curated dictionary that measures constructs such as psychological processes (e.g., anxiety and sadness) and linguistic dimensions (e.g., pronouns and verbs). To measure paragraph-level sentiment using LIWC we count the number of words within each paragraph that are in LIWC's positive emotions category and divide the count by the total number of words in the paragraph.
Table 1 shows the most similar words (or nearest neighbors) as measured by the (minimum) cosine distance between the word2vec representations of the keywords addict and addiction and all other words in the embedding space. In 1986, we see words associated with specific substances (“heroin”), connections to alcoholism, “abuse” and “abusers”, as well as mental (“psychosis” and “schizophrenia”) and eating disorders (“bulimia”). In 2000, we see further mentions of eating disorders (“anorexia” and “bulimia”) as well as “obesity”, words related to sex (“venereal” and “cybersex”). This continues in 2010, with “hypersexuality”/ “prostitutes” and “bulimia”/“bulimic”. Finally, in 2020 we see a larger number of words related to mental disorders: “bipolar,” “schizophrenia,” and “adhd.” Notably, “abuser” drops out of the top 10 results in 2020. Across all years, we see “heroin” closely related to addict.
In Table 2, we compare marijuana to heroin. Again, in 1986 the most similar words are other substances: “cocaine,” “heroin,” “marijuana,” and “hashish.” We also see words related to legality: “illicit” for both marijuana and heroin and “trafficking” for heroin. As time progresses, starting in 2010, marijuana becomes more closely related to legality (“decriminalizing” and “legalizing”), “dispensaries” (i.e., places to legally purchase marijuana), and other legal substances, such as “alcohol,” “nicotine,” and “tobacco.” Marijuana is also still closely related to “cocaine” and “methamphetamine(s)”, neither of which are legal. Heroin continues to be closely related to other substances as time progresses. Across all 4 years, “cocaine” remains the most related word. Other substances such as “methamphetamine(s),” “vicodin,” and “opiates” appear in 2000. In 2010, we see “oxycodone” and “oxycontin”, both of which are prescription painkillers. Finally, in 2020, we see “overdose” and “fentanyl”, a synthetic opioid.
In Figure 2, we see the temporal trends in the linguistic dehumanization measures for the addict and addiction keywords as well as the control keyword american. Note that smaller values on the vertical axis represent more dehumanization, while larger values represent less dehumanization. Across five of the seven measures, we find that american has larger values than both addict and addiction, suggesting increased dehumanization in the language discussing PWUS and matching results from Mendelsohn et al. For both connotation-frame measures (agency and perspective), we see all three keywords close to each other when compared to the remaining measures.
Figure 2. Comparison of addiction (blue triangles) and addict (purple squares) to the control keyword american (red circles) across all four measures of dehumanization: negative evaluation of a target group (A–C), denial of agency (D, E), moral disgust (F), and vermin metaphor (G). A significant increase or decrease over time is indicated with ↑ and ↓, respectively, next to the label in the legend in each subplot. In all plots, smaller values on the vertical axis represent increased dehumanization.
Figure 3 compares marijuana to heroin. Across four (out of seven) measures, we see the two keywords diverge: (a) paragraph-level sentiment, (b) average neighbor valence, (d) average neighbor dominance, and (f) distance from moral disgust. In each of these four plots, we see, over time, more humanization in language surrounding marijuana and more dehumanization in the language around heroin. Similar to the results we saw in Figures 2C, E, the connotation-frames measures show both keywords as having relatively similar values of linguistic dehumanization across time.
Figure 3. Comparison of marijuna (green stars) and heroin (brown hexagons) across all four measures of dehumanization: negative evaluation of a target group (A–C), denial of agency (D, E), moral disgust (F), and vermin metaphor (G). A significant increase or decrease over time is indicated with ↑ and ↓, respectively, next to the label in the legend in each subplot. In all plots, smaller values on the vertical axis represent increased dehumanization.
Turning to the components of dehumanization, we see: (1) all four keywords moving away from vermin, and (2) three out of four keywords becoming more distanced from disgust (heroin has no change). Thus, consistently across both Moral Disgust and Vermin Metaphor, we see more linguistic humanization regardless of the substance keyword being examined. Across the two Denial of Agency measures, we see mixed results, which depend on both the measure and the keyword: (1) addiction shows no change, (2) addict goes up in one measure (average dominance) and down in another (connotation frames of agency), (3) marijuana increases in one measure (average dominance) and shows no change in another (connotation frames of agency), and (4) heroin decreases in one measure (average dominance) and shows no change in another (connotation frames of agency). Similarly, Negative Evaluation of a Target Group shows mixed results for some keywords. Both addiction and marijuana increase for two of the three measures, while no change is seen for the third measure. The keyword addict differs across all three measures, while heroin shows no change in two out of the three measures (and decreases on the third measure).
Since all of the proposed measures are proxies for some component of dehumanization (e.g., distance from the vermin embedding is a proxy for vermin metaphors) and no single component is proof of humanization/dehumanization, we report summaries across each keyword. These results are summarized in Table 3. For each keyword, we summarize the temporal trend for each measure of dehumanization. Blue up arrows indicate an increase in humanization (i.e., a positive product-moment correlation, significant at p < 0.05), whereas red down arrows indicate an increase in dehumanization (i.e., a negative product-moment correlation, significant at p < 0.05). We then add the number of significant results, where up arrows (humanization) are counted as positive 1 and down arrows (dehumanization) are counted as −1 (thus, totals can range from −7 to 7). The resulting total provides a measure for the strength of the change in dehumanization over time. Results show that people who use marijuana, in particular, have become less dehumanized over time, with an increase in linguistic humanization across five out of seven measures. Articles using general terms for PWUS (addict, addiction, and alcohol keywords) saw slight increases in linguistic humanization (increases in two measures each). Discussions around the remaining keywords saw no change (alcoholism), small increases (alcoholic), or small decreases (cocaine and heroin) in linguistic humanization.
In Table 4 (addict and addiction), Table 5 (marijuana), and Table 6 (heroin), we show the top- and bottom-most valenced paragraphs in the data set. Words within the example paragraphs are highlighted red (negative) when the word has a valence score of ≤ 0.3 and highlighted blue when the word has a valence score of ≥0.7 in the NRC Valence lexicon. The final labels consist of (valence score correctly matches the level of dehumanization),
(valence score incorrectly matches the level of dehumanization), and NR (not relevant).
Table 4. Addict and addiction paragraphs with highest (top five rows) and lowest (bottom five rows) valence, as measured by the NRC Valence lexicon.
Table 5. Marijuana paragraphs with highest (top five rows) and lowest (bottom five rows) valence, as measured by the NRC Valence lexicon.
Table 6. Heroin paragraphs with highest (top five rows) and lowest (bottom five rows) valence, as measured by the NRC Valence lexicon.
Across all keywords, we see examples of the valence lexicon correctly and incorrectly identifying linguistic dehumanizing content. Several examples where linguistic dehumanization is incorrectly identified are a result of the following categories of words being negatively valenced: (1) substances and substance use (“drug” and “withdrawal”), (2) criminal justice (“arrest,” “incarceration,” and “sentencing”), and (3) mental and physical health (“pain,” “illness,” and “anxiety”). For addict and addiction in Table 4, we see that other types of addiction (skating and swimming) are being classified as related to substance use due to the ambiguity in the keyword approach. These two examples are thus labeled as not relevant (NR).
Table 7 compares the paragraph-level sentiment analysis using both the NRC Valence lexicon and LIWC. Here we see that the two sentiment measures agree on six out of eight keywords. The measures disagree on addict, where NRC shows an increase in valence and LIWC exhibits no change, and cocaine, where NRC shows a decrease in valence and LIWC increases.
Taken together, while the NRC lexicon by default drives results toward dehumanization and thus causes misclassifications, overall trends hold when using an alternative lexicon (LIWC) that does not have the same limitations.
America's War on Drugs extended to dehumanizing the people who use them. Our findings suggest that PWUS have been dehumanized in popular media for decades. Overall levels of dehumanization remain high when compared to baselines (e.g., americans). While temporal trends suggest that conversations have been shifting since the 1980s (i.e., toward decriminalizing and systemic issues such as mental health), there seem to be differences across substances. Marijuana, in particular, has become less dehumanized over time. These trends dovetail with annual polls showing increased support for legalizing marijuana (60). On the other hand, substances such as cocaine and heroin show little change.
While the results show that people who use marijuana have become less dehumanized over time, we note that there a long history of stigmatization and dehumanization toward this group. This dates back to the 1930s where it was connected to violent crimes and immigration. This switched contexts in the 1960s to dehumanize the hippie movement as “dropouts” and includes increased criminalization under the Nixon administration (34). It was not until the 1990s when support for legalization began to grow, which mostly overlaps with the NYT data used in the current study.
It has been shown that negative media coverage of substances declined in the 1990s, which coincides with positive public perceptions of marijuana. It is important to note that no causality has been established, as media coverage could be reflective of or driving public perceptions. Stringer and Maggard (61) note that there is also an increase in coverage of medical marijuana during the 1990s, which could be driving this increase in public perception.
Another possible reason for the difference in dehumanization toward people who use marijuana vs. those who use heroin or cocaine is they way these substances are consumed. Both cocaine and heroin can be smoked, snorted, or injected, whereas marijuana is typically smoked or consumed in an edible format. Research has shown that injectable substances are highly stigmatized (62) which can then be operationalized via dehumanization.
Rather than the narrative pushed by the War on Drugs of people with an SUD being cold, incompetent, and subhuman (63), policymakers, providers, and media outlets should ensure that drug policies and everyday healthcare practices counter the components of dehumanization. First, people with an SUD must be reidentified from nonhuman entities like zombies and trash to humans. Second, granting people with an SUD agency requires involving them in paving their road to recovery and recognizing their ability to comprehend potential harms once informed. For instance, the promotion of using drugs only in groups acknowledges the agency of people with an SUD to monitor each other; Good Samaritan laws similarly provide people using drugs immunity and thus the agency to call emergency services for someone else (19). Third, benevolent attitudes rather than negative evaluations of people suffering from SUDs could protect against dehumanization in both clinical and social settings (64). Fourth, blame should primarily be attributed to context and addiction as a disease rather than to the individual to minimize moral disgust. What is tricky is acknowledging agency while shifting blame away from people with SUDs (65). Blaming patients' behavior for their disease plays a role in increasing negative evaluations and moral disgust (66). Disgust evolved to motivate self-monitoring and punish people who threaten others with their disease (67). Disgust has been used to drive the stigmatization of outgroups and limit social interactions when needed most, thus, disgust responses should not always be trusted (68). Some of the disgust mitigation strategies for people with other diseases may apply to people with SUDs. During the AIDS epidemic, for instance, patients fought moral disgust by rejecting self-blame, proving that they do not pose a threat, working together with professionals, and raising awareness about the social effects of disease (67, 69, 70). Finally, research on media depictions of substance users has shown that media outlets are already capable of humanizing PWUS. This split between dehumanizing and humanizing typically falls along racial lines, with urban black and brown people criminalized for injecting heroin while suburban white people who “misuse” prescription drugs are shown in a sympathetic light (71). In sum, the shift away from dehumanization and toward harm reduction can be facilitated by meeting people with SUDs where they are and promoting humanistic treatment strategies to which people with SUDs can more feasibly adhere.
The analysis presented here is limited in several ways. First, the selected keywords are by no means exhaustive in terms of variation of substances or substances that are typically dehumanized. For example, we do not consider stimulants, despite the fact that people who use methamphetamines have been referred to as “meth zombies” in popular media and anti-drug ads (34). The keyword opioid(s) is perhaps the most obvious missing keyword, given the recent attention the opioid epidemic has received. This term did not gain popularity until after 2010 and, thus, could not be used in a multi-decade analysis. Similarly, keywords such as xanax, percocet, and oxycontin were never frequent enough to analyze, and we were therefore unable to examine prescription opioids. The second limitation is that the keywords do not explicitly refer to substance users, with the exception of addict and alcoholic, but rather the substances themselves. That said, when examining a random selection of articles, we did not find any examples that referred to specific substances such as marijuana and heroin that were not in the context of use. One could use more sophisticated keyword matching (e.g., “cocaine users”) or dependency parsing to identify people who use substances, which may result in a high precision and low recall matching. In the end, we decided on the simpler and more general approach (using substance keywords), since word embeddings and related measures depend on data frequency (72). Third, the baseline of american is not ideal, as the New York Times is a U.S. institution and, therefore, this keyword represents an ingroup. Thus, it may be the case that the reported levels of dehumanization toward PWUS are only high compared to this low baseline. That said, the levels of dehumanization reported here for the substance use keywords are similar or higher (e.g., lower distance from vermin) than those reported in Mendelsohn et al. (2) who examined dehumanization toward LGBTQ people, another historically dehumanized group. Fourth, the data was collected using two sampling methods. The data from 1986 to 2015 was collected outside of this manuscript, whereas the data from 2016 to 2020 was collected separately for this study. These collections may have used different sampling strategies and, thus, biased the data from the last 5 years. Finally, we only consider data from a national, highly respected, and liberal-leaning news source. One might expect to see different patterns of dehumanization when using local newspapers, which may report more on drug-related arrests or whose opinion pieces may more accurately reflect the local population. Similarly, right-leaning news sources may also show different patterns of dehumanization.
The full dataset is available from the New York Times and can be downloaded using our code: https://osf.io/uya29/. Requests for the 1986–2015 data can be sent to Mendelson et al. (2020) while requests for the 2016–2020 data can be sent to the corresponding author.
SG: Conceptualization, Data curation, Formal analysis, Methodology, Visualization, Writing—original draft. DH: Writing—original draft. DB: Data curation, Formal analysis, Software, Writing—review & editing. GS: Data curation, Writing—review & editing. BC: Conceptualization, Funding acquisition, Methodology, Supervision, Writing—review & editing.
The author(s) declare financial support was received for the research, authorship, and/or publication of this article. This research was supported in part by the Intramural Research Program of the NIH, National Institute on Drug Abuse (ZIA DA000628).
We thank Julia Mendelsohn for sharing data and code, as well as for conducting an inspirational study. We also thank Zachary Fried and Zachary Demko for their help with this manuscript.
The authors declare that the research was conducted in the absence of any commercial or financial relationships that could be construed as a potential conflict of interest.
All claims expressed in this article are solely those of the authors and do not necessarily represent those of their affiliated organizations, or those of the publisher, the editors and the reviewers. Any product that may be evaluated in this article, or claim that may be made by its manufacturer, is not guaranteed or endorsed by the publisher.
1. ^https://developer.nytimes.com/docs/archive-product/1/overview.
2. ^https://www.crummy.com/software/BeautifulSoup/.
3. ^To aid the reader throughout the paper, we italicize keywords in order to distinguish keywords from results, which may be quoted. For example, Table 2 shows that “marijuana” is related to the keyword heroin and “heroin” is related to the keyword marijuana.
4. ^For legibility, we only consider a subset of the keywords.
1. Haslam N. Dehumanization: an integrative review. Person Soc Psychol Rev. (2006) 10:252–64. doi: 10.1207/s15327957pspr1003_4
2. Mendelsohn J, Tsvetkov Y, Jurafsky D. A framework for the computational linguistic analysis of dehumanization. Front Artif Intell. (2020) 3:55. doi: 10.3389/frai.2020.00055
3. Harris LT, Fiske ST. Dehumanized Perception: A Psychological Means to Facilitate Atrocities, Torture, and Genocide? Z Psychol. (2011) 219:175–81. doi: 10.1027/2151-2604/a000065
4. Steuter E, Wills D. “The vermin have struck again”: dehumanizing the enemy in post 9/11 media representations. Media War Conflict. (2010) 3:152–67. doi: 10.1177/1750635210360082
5. Haque OS, Waytz A. Dehumanization in medicine: causes, solutions, and functions. Perspect Psychol Sci. (2012) 7:176–86. doi: 10.1177/1745691611429706
6. Kersbergen I, Robinson E. Blatant dehumanization of people with obesity. Obesity. (2019) 27:1005–12. doi: 10.1002/oby.2246
7. Szasz T. Ideology and Insanity: Essays on the Psychiatric Dehumanization of Man. New York, NY: Syracuse University Press (1991).
8. Reinarman C. The Social Construction of Drug Scares. Constructions of Deviance: Social Power, Context, and Interaction. Boston, MA: Cengage Learning (1994). p. 92–105.
9. Cohen A, Vakharia SP, Netherland J, Frederique K. How the war on drugs impacts social determinants of health beyond the criminal legal system. Ann Med. (2022) 54:2024–38. doi: 10.1080/07853890.2022.2100926
10. Sumnall H, Atkinson A, Gage S, Hamilton I, Montgomery C. Less than human: dehumanisation of people who use heroin. Health Educ. (2021) 121:649–69. doi: 10.1108/HE-07-2021-0099
11. Clement S, Schauman O, Graham T, Maggioni F, Evans-Lacko S, Bezborodovs N, et al. What is the impact of mental health-related stigma on help-seeking? A systematic review of quantitative and qualitative studies. Psychol Med. (2015) 45:11–27. doi: 10.1017/S0033291714000129
12. Bremer W, Plaisance K, Walker D, Bonn M, Love JS, Perrone J, et al. Barriers to opioid use disorder treatment: a comparison of self-reported information from social media with barriers found in literature. Front Public Health. (2023) 11:1141093. doi: 10.3389/fpubh.2023.1141093
13. Byrne SK. Healthcare avoidance: a critical review. Holist Nurs Pract. (2008) 22:280–92. doi: 10.1097/01.HNP.0000334921.31433.c6
14. Kelly JF, Saitz R, Wakeman S. Language, substance use disorders, and policy: the need to reach consensus on an “addiction-ary”. Alcohol Treat Q. (2016) 34:116–23. doi: 10.1080/07347324.2016.1113103
15. Ashford RD, Brown AM, Curtis B. Substance use, recovery, and linguistics: the impact of word choice on explicit and implicit bias. Drug Alcohol Depend. (2018) 189:131–8. doi: 10.1016/j.drugalcdep.2018.05.005
16. Ashford RD, Brown AM, McDaniel J, Curtis B. Biased labels: an experimental study of language and stigma among individuals in recovery and health professionals. Subst Use Misuse. (2019) 54:1376–84. doi: 10.1080/10826084.2019.1581221
17. Kelly JF, Westerhoff CM. Does it matter how we refer to individuals with substance-related conditions? A randomized study of two commonly used terms. Int J Drug Policy. (2010) 21:202–7. doi: 10.1016/j.drugpo.2009.10.010
18. McGinty EE, Stone EM, Kennedy-Hendricks A, Barry CL. Stigmatizing language in news media coverage of the opioid epidemic: implications for public health. Prev Med. (2019) 124:110–4. doi: 10.1016/j.ypmed.2019.03.018
19. Brown TR. The role of dehumanization in our response to people with substance use disorders. Front Psychiatry. (2020) 11:372. doi: 10.3389/fpsyt.2020.00372
20. American Psychiatric Association American Psychological Association. Diagnostic and Statistical Manual of Mental Disorders: DSM-5. Vol 5. Washington, DC: American Psychiatric Association (2013).
21. Broyles LM, Binswanger IA, Jenkins JA, Finnell DS, Faseru B, Cavaiola A, et al. Confronting inadvertent stigma and pejorative language in addiction scholarship: a recognition and response. Subst Abuse. (2014) 35:217–21. doi: 10.1080/08897077.2014.930372
22. Volkow ND, Gordon JA, Koob GF. Choosing appropriate language to reduce the stigma around mental illness and substance use disorders. Neuropsychopharmacology. (2021) 46:2230–2. doi: 10.1038/s41386-021-01069-4
23. Garg N, Schiebinger L, Jurafsky D, Zou J. Word embeddings quantify 100 years of gender and ethnic stereotypes. Proc Nat Acad Sci USA. (2018) 115:E3635–44. doi: 10.1073/pnas.1720347115
24. Fast E, Horvitz E. Long-term trends in the public perception of artificial intelligence. In: Proceedings of the AAAI Conference on Artificial Intelligence. Vol. 31 (San Francisco, CA) (2017). doi: 10.1609/aaai.v31i1.10635
25. Card D, Chang S, Becker C, Mendelsohn J, Voigt R, Boustan L, et al. Computational analysis of 140 years of US political speeches reveals more positive but increasingly polarized framing of immigration. Proc Nat Acad Sci USA. (2022) 119:e2120510119. doi: 10.1073/pnas.2120510119
26. Gupta R, Holtgrave DR, Ashburn MA. Xylazine—medical and public health imperatives. N Engl J Med. (2023). doi: 10.1056/NEJMp2303120
27. Sherman GD, Haidt J. Cuteness and disgust: the humanizing and dehumanizing effects of emotion. Emot Rev. (2011) 3:245–51. doi: 10.1177/1754073911402396
28. Bar-Tal D. Causes and consequences of delegitimization: models of conflict and ethnocentrism. J Soc Iss. (1990) 46:65–81. doi: 10.1111/j.1540-4560.1990.tb00272.x
29. Opotow S. Moral exclusion and injustice: an introduction. J Soc Iss. (1990) 46:1–20. doi: 10.1111/j.1540-4560.1990.tb00268.x
30. Tipler C, Ruscher JB. Agency's role in dehumanization: non-human metaphors of out-groups. Soc Personal Psychol Compass. (2014) 8:214–28. doi: 10.1111/spc3.12100
31. Buckels EE, Trapnell PD. Disgust facilitates outgroup dehumanization. Group Process Intergroup Relat. (2013) 16:771–80. doi: 10.1177/1368430212471738
32. Monroe AE, Plant EA. The dark side of morality: prioritizing sanctity over care motivates denial of mind and prejudice toward sexual outgroups. J Exp Psychol. (2019) 148:342. doi: 10.1037/xge0000537
33. Haslam N, Loughnan S. Dehumanization and infrahumanization. Annu Rev Psychol. (2014) 65:399–423. doi: 10.1146/annurev-psych-010213-115045
34. Habib DRS, Giorgi S, Curtis B. Role of the media in promoting the dehumanization of people who use drugs. Am J Drug Alcohol Abuse. (2023) 49:1–10. doi: 10.1080/00952990.2023.2180383
35. Reinarman C, Duskin C. Dominant ideology and drugs in the media. In:Ferrell J, Websdale N, , editors. Making Trouble. New York, NY: Routledge (2017). p. 73–88.
36. Durham III AM, Elrod HP, Kinkade PT. Images of crime and justice: Murder and the “true crime” genre. J Crim Just. (1995) 23:143–52. doi: 10.1016/0047-2352(95)00002-8
37. Coomber R. Drugs and Drug Use in Society: a Critical Reader. Vol 1. Dartford: Greenwich University Press (1994).
38. Boyd S. Media constructions of illegal drugs, users, and sellers: a closer look at Traffic. Int J Drug Policy. (2002) 13:397–407. doi: 10.1016/S0955-3959(02)00079-8
39. Young J. The role of the police as amplifiers of deviancy, negotiators of reality and translators of fantasy. Images Deviance. (1971) 37:27–61.
40. Baum MA, Potter PB. The relationships between mass media, public opinion, and foreign policy: Toward a theoretical synthesis. Annu Rev Polit Sci. (2008) 11:39–65. doi: 10.1146/annurev.polisci.11.060406.214132
41. Gentzkow M, Shapiro JM. What drives media slant? Evidence from US daily newspapers. Econometrica. (2010) 78:35–71. doi: 10.3982/ECTA7195
42. Murji K. The agony and the ecstasy: drugs, media and morality. In: The Control of Drugs and Drug Users. Boca Raton, FL: CRC Press (2020). p. 69–85.
43. Khademi S, Hallinan CM, Conway M, Bonomo Y. Using social media data to investigate public perceptions of cannabis as a medicine: narrative review. J Med Internet Res. (2023) 25:e36667. doi: 10.2196/36667
44. Lavertu A, Altman RB. RedMed: extending drug lexicons for social media applications. J Biomed Inform. (2019) 99:103307. doi: 10.1016/j.jbi.2019.103307
45. Curtis B, Giorgi S, Buffone AE, Ungar LH, Ashford RD, Hemmons J, et al. Can Twitter be used to predict county excessive alcohol consumption rates? PLoS ONE. (2018) 13:e0194290. doi: 10.1371/journal.pone.0194290
46. Giorgi S, Yaden DB, Eichstaedt JC, Ashford RD, Buffone AE, Schwartz HA, et al. Cultural differences in Tweeting about drinking across the US. Int J Environ Res Public Health. (2020) 17:1125. doi: 10.3390/ijerph17041125
47. Sarker A, Gonzalez-Hernandez G, Ruan Y, Perrone J. Machine learning and natural language processing for geolocation-centric monitoring and characterization of opioid-related social media chatter. JAMA Netw Open. (2019) 2:e1914672-e1914672. doi: 10.1001/jamanetworkopen.2019.14672
48. Giorgi S, Yaden DB, Eichstaedt JC, Ungar LH, Schwartz HA, Kwarteng A, et al. Predicting US county opioid poisoning mortality from multi-modal social media and psychological self-report data. Sci Rep. (2023) 13:9027. doi: 10.1038/s41598-023-34468-2
49. Sarker A, Ginn R, Nikfarjam A, O'Connor K, Smith K, Jayaraman S, et al. Utilizing social media data for pharmacovigilance: a review. J Biomed Informat. (2015) 54:202–12. doi: 10.1016/j.jbi.2015.02.004
50. Chen AT, Johnny S, Conway M. Examining stigma relating to substance use and contextual factors in social media discussions. Drug Alcohol Depend Rep. (2022) 3:100061. doi: 10.1016/j.dadr.2022.100061
51. Conway M, Citrenbaum C, Chen AT. Substance use-related stigma: an exploratory study of search behavior using Google trends (2004-2021). Am J Drug Alcohol Abuse. (2022) 48:504–6. doi: 10.1080/00952990.2022.2068422
52. Giorgi S, Bellew D, Habib DRS, Sherman G, Sedoc J, Smitterberg C, et al. Lived experience matters: automatic detection of stigma on social media toward people who use substances. In: Proceedings of the International AAAI Conference on Web and Social Media (in press).
53. Mikolov T, Sutskever I, Chen K, Corrado GS, Dean J. Distributed representations of words and phrases and their compositionality. In:Burges CJ, Bottou L, Welling M, Ghahramani Z, Weinberger KQ, , editors. Proceedings of the 26th International Conference on Neural Information Processing Systems - Vol 2 (Lake Tahoe, NV: Curran Associates, Inc.) (2013).
54. Řehřek R, Sojka P. Software framework for topic modelling with large corpora. In: Proceedings of the LREC 2010 Workshop on New Challenges for NLP Frameworks. Valletta: ELRA (2010). p. 45-50. Available online at: http://is.muni.cz/publication/884893/en (accessed August 31, 2023).
55. Mohammad S. Obtaining reliable human ratings of valence, arousal, and dominance for 20,000 English words. In: Proceedings of the 56th Annual Meeting of the Association for Computational Linguistics. Vol. 1 (Melbourne, VIC) (2018). p. 174–84.
56. Rashkin H, Singh S, Choi Y. Connotation frames: a data-driven investigation. In: Proceedings of the 54th Annual Meeting of the Association for Computational Linguistics. Vol. 1. Berlin: Association for Computational Linguistics (2016). p. 311–21. Available online at: https://aclanthology.org/P16-1030 (accessed August 31, 2023).
57. Sap M, Prasettio MC, Holtzman A, Rashkin H, Choi Y. Connotation frames of power and agency in modern films. In: Proceedings of the 2017 Conference on Empirical Methods in Natural Language Processing. Copenhagen, Denmark: Association for Computational Linguistics (2017). p. 2329–34. Available online at: https://aclanthology.org/D17-1247 (accessed August 31, 2023).
58. Graham J, Haidt J, Nosek BA. Liberals and conservatives rely on different sets of moral foundations. J Pers Soc Psychol. (2009) 96:1029. doi: 10.1037/a0015141
59. Pennebaker JW, Francis ME, Booth RJ. Linguistic Inquiry and Word Count: LIWC 2001. Mahway: Lawrence Erlbaum Associates (2001). 71 p.
60. Gallup. Support for Legal Marijuana Holds at Record High of 68% (2021). Available online at: https://news.gallup.com/poll/356939/support-legal-marijuana-holds-record-high.aspx (accessed August 6, 2023).
61. Stringer RJ, Maggard SR. Reefer madness to marijuana legalization: media exposure and American attitudes toward marijuana (1975-2012). J Drug Issues. (2016) 46:428–45. doi: 10.1177/0022042616659762
62. Biancarelli DL, Biello KB, Childs E, Drainoni M, Salhaney P, Edeza A, et al. Strategies used by people who inject drugs to avoid stigma in healthcare settings. Drug Alcohol Depend. (2019) 198:80–6. doi: 10.1016/j.drugalcdep.2019.01.037
63. Fiske ST. From dehumanization and objectification to rehumanization: neuroimaging studies on the building blocks of empathy. Ann N Y Acad Sci. (2009) 1167:31–4. doi: 10.1111/j.1749-6632.2009.04544.x
64. Fontesse S, Demoulin S, Stinglhamber F, Maurage P. Dehumanization of psychiatric patients: experimental and clinical implications in severe alcohol-use disorders. Addict Behav. (2019) 89:216–23. doi: 10.1016/j.addbeh.2018.08.041
65. Pickard H. Responsibility without blame for addiction. Neuroethics. (2017) 10:169–80. doi: 10.1007/s12152-016-9295-2
66. Shepherd MA, Gerend MA. The blame game: cervical cancer, knowledge of its link to human papillomavirus and stigma. Psychol Health. (2014) 29:94–109. doi: 10.1080/08870446.2013.834057
67. Curtis V. Why disgust matters. Philos Transact R Soc B Biol Sci. (2011) 366:3478–90. doi: 10.1098/rstb.2011.0165
68. Nussbaum MC. Hiding from Humanity: Disgust, Shame, and the Law. Princeton, NJ: Princeton University Press (2009).
69. Brandt AM. AIDS in historical perspective: four lessons from the history of sexually transmitted diseases. Am J Public Health. (1988) 78:367–71. doi: 10.2105/AJPH.78.4.367
70. Brown L, Macintyre K, Trujillo L. Interventions to reduce HIV/AIDS stigma: what have we learned? AIDS Educ Prev. (2003) 15:49–69. doi: 10.1521/aeap.15.1.49.23844
71. Netherland J, Hansen HB. The war on drugs that wasn't: wasted whiteness, “dirty doctors,” and race in media coverage of prescription opioid misuse. Cult Med and Psychiatry. (2016) 40:664–86. doi: 10.1007/s11013-016-9496-5
72. Zhou K, Ethayarajh K, Card D, Jurafsky D. Problems with cosine as a measure of embedding similarity for high frequency words. In: Proceedings of the 60th Annual Meeting of the Association for Computational Linguistics. Vol. 2. Dublin: Association for Computational Linguistics (2022). p. 401–23. Available online at: https://aclanthology.org/2022.acl-short.45 (accessed August 31, 2023).
Keywords: dehumanization, substance use, addiction, New York Times, computational linguistics
Citation: Giorgi S, Habib DRS, Bellew D, Sherman G and Curtis B (2023) A linguistic analysis of dehumanization toward substance use across three decades of news articles. Front. Public Health 11:1275975. doi: 10.3389/fpubh.2023.1275975
Received: 01 September 2023; Accepted: 09 October 2023;
Published: 23 November 2023.
Edited by:
Mike Conway, The University of Utah, United StatesReviewed by:
Darshan Singh Darshan Singh, University of Science Malaysia (USM), MalaysiaCopyright © 2023 Giorgi, Habib, Bellew, Sherman and Curtis. This is an open-access article distributed under the terms of the Creative Commons Attribution License (CC BY). The use, distribution or reproduction in other forums is permitted, provided the original author(s) and the copyright owner(s) are credited and that the original publication in this journal is cited, in accordance with accepted academic practice. No use, distribution or reproduction is permitted which does not comply with these terms.
*Correspondence: Brenda Curtis, YnJlbmRhLmN1cnRpc0BuaWguZ292
Disclaimer: All claims expressed in this article are solely those of the authors and do not necessarily represent those of their affiliated organizations, or those of the publisher, the editors and the reviewers. Any product that may be evaluated in this article or claim that may be made by its manufacturer is not guaranteed or endorsed by the publisher.
Research integrity at Frontiers
Learn more about the work of our research integrity team to safeguard the quality of each article we publish.