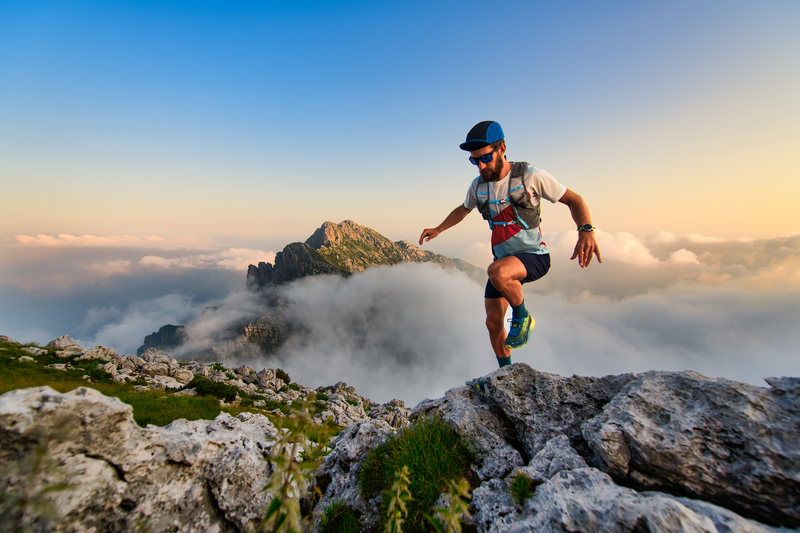
95% of researchers rate our articles as excellent or good
Learn more about the work of our research integrity team to safeguard the quality of each article we publish.
Find out more
PERSPECTIVE article
Front. Public Health , 22 September 2023
Sec. Digital Public Health
Volume 11 - 2023 | https://doi.org/10.3389/fpubh.2023.1265050
This article is part of the Research Topic Utilizing Big Data and Deep Learning to improve Healthcare Intelligence and Biomedical Service Delivery View all 8 articles
In the information age, real-world data-based evidence can help extrapolate and supplement data from randomized controlled trials, which can benefit clinical trials and drug development and improve public health decision-making. However, the legitimate use of real-world data in China is limited due to concerns over patient confidentiality. The use of personal information is a core element of data governance in public health. In China’s public health data governance, practical problems exist, such as balancing personal information protection and public value conflict. In 2021, China adopted the Personal Information Protection Law (PIPL) to provide a consistent legal framework for protecting personal information, including sensitive medical health data. Despite the PIPL offering critical legal safeguards for processing health data, further clarification is needed regarding specific issues, including the meaning of “separate consent,” cross-border data transfer requirements, and exceptions for scientific research. A shift in the law and regulatory framework is necessary to advance public health research further and realize the potential benefits of combining real-world evidence and digital health while respecting privacy in the technological and demographic change era.
Despite the absence of a globally agreed-upon definition in recent years, the term real-world data (RWD) quickly morphed into a buzzword (1). Definitions of RWD differ depending on the source or environment from which the data were generated or collected (2). Given its enhanced popularity, China’s National Medical Products Administration (NMPA) introduced a series of policies to standardize and guide the application of RWD. Per the NMPA’s definition, RWD are “various data related to patients’ health status and/or diagnosis and health care that are collected on a daily basis, opposed to data gathered in experimental settings such as randomized controlled trials” (3). RWD contains digital and non-digital data from various sources, including hospital electronic medical records (EMRs), classical cohort studies, administrative databases, daily wearable devices, and surveillance databases (4). Using RWD to inform health-related decisions is referred to as real-world evidence (RWE). NMPA further defines RWE as “the clinical evidence about the use and potential benefit–risk of a drug obtained through appropriate and adequate analysis of applicable RWD.” This definition can be used to support drug development and regulatory decisions and for other scientific purposes (3, 5).
The RWD-based study (RWS) (6, 7) has been frequently used to address recent public health issues. This is because addressing urgent public health requirements today requires access to and cross-referencing of several types of data (8). For example, the COVID-19 pandemic demonstrated that promptly capturing and using RWD from community testing can support real-time monitoring and decision-making by public health agencies (9). When threats to public health arise from epidemic outbreaks, government agencies should have access to RWD from various sources like EMRs, pharmacy claims, medical claims, and labs to understand disease spread, predict potential outbreaks, create surveillance systems, allocate resources, and make informed decisions (10). RWS is characterized by a vast research population, diverse study scenarios, substantial heterogeneity among a wide range of data sources, and high external validity. Other RWS applications to address public health issues can be found in the literature, such as a scoping review that demonstrated potential for applying RWD to address noncommunicable diseases with greater precision (11). Recently, Sarovar et al. (12) conducted a study using air pollution and fetal death certificate data to provide evidence for associations between prenatal short-term air pollution exposure and stillbirth.
In China, Du (13) first introduced the concept of RWS, describing its use in evaluating the safety of Traditional Chinese Medicine injections. In response to the increasing interest in conducting RWS, the NMPA organized representatives from academia, the pharmaceutical industry, and relevant institutions to formulate policies for using RWD in the medical and healthcare field and to promote RWS-based regulatory science (14). Chinese researchers have made efforts in the field of RWS related to public health research. Yin et al. (15) conducted a RWS using EMRs collected on 1,446 inpatients with neurosurgery operations in a grade A tertiary hospital during a 5-year period to address the public health threat from antibiotic resistance. On June 9, 2023, China launched a RWS on COVID-19-listed drugs in Beijing. Data from nearly 40,000 patients at more than 100 large and grassroots hospitals across 30 provinces, cities, and autonomous regions will be included in the RWS (16). This RWS will provide more solid evidence for COVID-19 drugs’ efficacy, effectiveness, and safety, and assist in validating their use in clinical settings. Moreover, this RWS is an essential exercise for China to strengthen the construction of the public health emergency system at the grassroots level.
Real-world data-based study have tremendous potential to promote medical research, regulatory decision-making, and public health in China. However, a few challenges remain (17–20), including inconsistent terminology and non-standardized coding, a lack of longitudinal data, an inability to process and validate data transparently, and barriers to medical health data (MHDs) access, sharing, and linkage across research institutions (21–25). This article focuses only on the challenges raised by the data protection law. The processing of RWD triggers patient privacy concerns and, therefore, must adhere to relevant data laws and regulations (26). Consequently, researchers conducting RWS in China face significant compliance challenges related to personal information (PI) protection, which generate possible legal impediments. This article suggests how best to handle these obstacles and overcome them.
China’s Personal Information Protection Law (PIPL), enacted in November 2021, defines PI as “various information related to identified or identifiable natural persons recorded electronically or by other means.” It distinguishes between PI and sensitive PI and constructs a two-layered (a risk-based general clause + an opened list) style (27) criteria to identify sensitive PI (Table 1). Unlike the European Union’s (EU) General Data Protection Regulation (GDPR), which adopted both an objective context-based and a subjective purposeful approach to determine if information included sensitive data (28), the PIPL takes a consequentialist approach. The PI disclosure risk and its potential damages play decisive roles in recognizing sensitive characteristics.
Real-world data related to MHDs falls within the scope of sensitive PI. Individuals could be at risk of data exposure without appropriate legislative measures to protect these data (29). In general, the GDPR forbids processing “special categories of personal data,” including MHDs, but preserves certain exemptions to the prohibition. Unlike the GDPR, the PIPL does not prohibit the processing of sensitive PI; instead, it provides stricter duties for PI processors. Accordingly, acceptable RWD processing requires either individualized “separate” consent from each person connected with the data or anonymized PI or the research must possess a specific purpose, be sufficiently necessary, implement strict protective measures, and conduct a protection impact assessment (PIA).
An inherent challenge of any data-related research involves balancing data privacy protection with data availability, especially MHDs that are highly sensitive in nature (30). Overly strict or unclear data protection rules hinder data access and sharing. Therefore, they reduce the quality and availability of data in RWS. For Chinese researchers, the particularity of China’s medical environment increases the difficulty of accessing, sharing, and linking MHDs. For example, as mentioned above, the lack of longitudinal follow-up data is an obstacle to conduct RWS. This is owing to the fact that patients often seek treatment in multiple hospitals (not through a referral system), which makes it difficult for medical institutions to obtain complete EMRs for the patient (24). To make use of longitudinal RWD, integrating fragmented data from various sources and breaking down data silos while ensuring patients’ PI security is urgently required (31). Although research institutions and technology companies have attempted to build databases and integrate patients’ EMRs and electronic health records across multiple institutions into one system (32), they face serious compliance risks under the PIPL. Since China’s PIPL does not provide for scientific research exemptions for the reuse of MHDs, the utilization and reuse of RWD should strictly comply with the PIPL, including obtaining re-consent from the patients. Data fragmentation and high legal compliance costs degrade RWD accessibility and usability for research (33).
The primary obstacle to processing RWD is obtaining the PI subject’s consent. This requirement resolves the ethical data quandary associated with RWS involving humans. It is rooted in the right to informational self-determination, which aims to increase individual control over one’s PI. This right is guaranteed in both the GDPR (34) and U.S. California Consumer Privacy Act (35) and is also recognized in China’s PIPL.
Under PIPL, PI processors are obligated to obtain specific, informed consent from PI subjects regarding their PI handling activities. The individual should voluntarily and explicitly give such consent on a fully informed basis. As for sensitive PI processing, the PIPL sets higher standards concerning informed consent and notification duties; in such instances, separate consent is mandatory for certain processing activities.
The PIPL does not define “separate consent.” However, the semantic interpretation clearly indicates that it does not permit “broad/blanket consent.” This means that RWS researchers must inform personal data subjects about the specific purpose, necessity, and impact of PI processing for specific research projects and obtain explicit consent from each individual. This process can be difficult, time-consuming, and costly. However, many medical breakthroughs rely on large-scale data collection of sensitive MHDs (36). Without broad access to and use of such data, RWS is not feasible. Ergo, PIPL’s separate consent requirement limits big data analytics as it reduces the volume of data available for analysis.
Data desensitization technologies, such as anonymization and de-identification, are critical to ensuring the legal compliance of RWD processing. Anonymity exists on a continuum from complete anonymity to fully identifiable data (37); de-identified data lies somewhere in the middle.
Anonymized PI is outside the regulatory scope of the PIPL, so its use would not violate the PIPL. However, the anonymization of rich MHDs may limit data linkage possibilities and exclude information crucial to RWS, reducing the data’s usefulness (38). For example, the anonymized data set of “John Doe, 14 years old” could be “Male, 10–20 years,” which distorts the data.
In contrast, de-identification seeks to preserve as much of the underlying data as possible, as a precursor to anonymization (39). According to the U.S. Health Insurance Portability and Accountability Act (HIPAA) de-identified PI under PIPL is not identical to de-identified protected health information. Instead, it is consistent with the GDPR’s definition of pseudonymization. The de-identification technology separates data from personal identity by removing identifying attributes. This action reduces the significant identifying risks to an acceptably small level. However, the de-identified RWD still falls into PIPL’s protection scope since the re-identification risk remains. Therefore, the current PIPL de-identification rule is too simplistic to support RWS since it neither clarifies the criteria for lawful de-identification nor establishes when de-identified PI can be considered “anonymous.”
Real-world data-based study researchers should conduct a prior PI-PIA (Table 2). A PI-PIA must be conducted if PI processors want to transmit RWD abroad. This institution is somewhat similar to the “data protection impact assessment” required under GDPR, which helps negate risk.
The RWD processing risk is not static or binary. Instead, it exists on a spectrum that changes with the processing scenario. The PI-PIA conducts a case-by-case, context-based analysis to evaluate the risk degree and the effectiveness of the protective measures taken, which structurally embeds the legal and ethical considerations of regulators into the PI processing. It introduces legal obligations to force PI processors to “identify, assess, and ultimately manage the high risks to rights and freedoms” (40) posed by PI processing beforehand, ultimately shifting the PI protection model from an ex-post regulation to an ex-ante prevention model based on risk management.
Legitimate cross-border data transfer (CBDT) requires the PI processor to pass a security assessment organized by the Cyberspace Administration of China (CAC), obtain a PI protection certification from a body recognized by the CAC, or sign a standard contract formulated by the CAC with the overseas recipient (article 38 PIPL). In addition, the PIPL imposes stricter restrictions on CBDT than most data protection laws by requiring certain entities to comply with PI localization obligations (article 40 PIPL). To carry out CBDT, the PI processor must also notify PI subjects of the specific information about the CBDT and obtain their separate consent (article 39 PIPL). Unlike the GDPR, the PIPL does not have an “adequacy decision” or “appropriate safeguards” mechanism for CBDT or various exceptions for specific situations from the prohibition. These requirements have made CBDT challenging to execute.
This article has demonstrated the legal dilemmas of conducting RWS in China, mainly the compliance hurdles related to PI protection. The primary factor impeding effective data utilization and weakening individual rights and interests protection is the lag of relevant legislation and regulations in data governance in public health. This article proposes several countermeasures to strike a balance between data utility and privacy protection.
In RWS based on big data, researchers can uncover patterns of human physiology and pathology by comparing data from different races, disease spectrums, and environments. These data inevitably contain sensitive PI. Strict consent obligations hinder such data collection, limiting opportunities for RWS. Accordingly, a consent model must be developed to address the potential conflict between active data use and informed consent principles.
The level of privacy risk depends upon how the PI is used in varying scenarios. Thus, consent models should adjust accordingly (41). Legislators should construct a dynamic, context-based meta-consent model for scientific research to enable participants to give either specific or broad consent depending on personal preferences for the secondary use of their PI (42). Compared to broad consent, which may not meet ethical requirements in some medical studies involving humans, the meta-consent model better balances the legal and ethical obligations of the researcher. It suggests, for example, establishing a web-based portal or application that allows individual research participants to dynamically provide blanket or specific consents for different personal data types based on their preferences, depending on the different RWS project categories they are involved in (43). Specifically, the research participant might choose to give blanket consent for RWS in the public interest, to provide separate consent for commercial RWD use, or to refuse consent to transfers of personal data across borders; moreover, participants can modify their initial choices (44–48). Active participation in the tiered-consent process, which improves interactions between healthcare providers and patients (49), along with increased transparency regarding PI use, are the main advantages of this model. Opponents argue that its implementation costs are higher than broad consent or dynamic consent model (44, 50). However, implementing meta-consent would reduce the average costs of obtaining consent from PI subjects compared to the current specific, separate informed model while improving the availability of MHDs in RWS and respect for participants’ autonomy. Therefore, this is a pragmatic approach.
To overcome the legal barriers in RWD processing, we recommend regulators adopt a pragmatic, risk-based approach to clarify the criteria for “adequately” de-identifying PI in different scenarios to preserve as much data utility as possible. China’s regulators should consider adopting HIPAA-like privacy rules, which allow sharing and using health data without informed consent as long as the data has been de-identified by expert determination or the safe harbor method. To prevent the weakening of de-identification criteria due to rapid technological advances, PI processors should be contractually prohibited from re-identifying PI and be held liable for non-compliance.
Medical health data have unique public interest attributes (25). RWS that processes large amounts of MHDs can promote the prevention and control of infectious diseases, drug development, and more, which greatly enhance public health interests. Strict de-identified criteria is more biased toward PI protection and partially sacrifices the data utilization, given that different data types have different requirements for protection and utilization. This suggests that the homogeneous de-identified criteria cannot be simultaneously applied to the de-identification needs of different PI types, such as financial data, sensitive PI such as religious beliefs or sexual orientation, and MHDs. On this basis, in particular, the de-identification of MHDs should be regulated. Specifically, China should consider enacting separate regulations on MHDs in addition to the PIPL. Meanwhile, the government should authenticate specialized institutions with ultimate responsibility for MHD processing and de-identification (51). This makes it easier for research institutions to choose suitable de-identification service providers; moreover, it reduces their compliance risks and encourages them to share research data, ultimately improving the availability and quality of MHDs, while balancing data protection and utilization.
Maximizing RWD potential and protecting patients’ PI are critical values. If they are in conflict, the regulatory framework must be adjusted to balance them.
The PIPL improves accountability for protecting PI with strict regulations like those on CBDT. However, it does not sufficiently balance research promotion with the global trend toward responsible data sharing. We cannot ignore that the MHDs is not only a matter of patient welfare but also a matter of public interest. The EU recently introduced a new concept of data altruism in the Data Governance Act and published a proposal for the European Health Data Space to improve MHDs availability. In contrast, China lacks similar legislation and a unified, effective PI protection agency with independent authority, which may place China at an international disadvantage for RWD use.
China’s National Standard “Information Security Technology—Guide for Health Data Security 2020” (GHDS) introduced the concept of a “limited data set,” which stipulates that de-identified data could be used or disclosed without the PI subject’s consent when used for scientific research, medical/health education, and public health purposes. However, the GHDS is a recommended national standard without legal force and inconsistent with current PIPL rules. To address this, reasonable proportionate legal derogations and exceptions for processing PI should be enacted for scientific and medical purposes, as well as for the public good (52). We urge legislators to revise the PI regulatory framework to balance “risk control” and “industry promotion” (53). The NMPA could create a negative list of PI processing practices for RWS to protect citizens’ PI rights and avoid unclear regulations. Likewise, international organizations (e.g., WHO) should lead in strengthening cooperation and collaboration among member countries to protect MHDs within multilateral frameworks.
Information technology-derived RWS is promoting a new round of digital revolution in the health field. At the same time, it brings PI challenges which urgently need to be solved. To promote the RWS to support public health efforts, we should clarify the regulatory model of utilitarianism of RWD in public health care and resolve the tension between active utilization and strict data protection. China’s legislation ought to encourage the active use of data in public health care through the introduction of the flexible meta-consent model, the adoption of a risk-based approach to interpreting and clarifying the de-identifying PI criteria, and weakening the current strict regulatory framework by introducing other supplementary mechanisms to balance the protection of PI and conflict of public value.
The original contributions presented in the study are included in the article/supplementary material, further inquiries can be directed to the corresponding author.
YY: Conceptualization, Data curation, Supervision, Writing – original draft, Writing – review & editing. FY: Conceptualization, Data curation, Supervision, Writing – original draft, Writing – review & editing.
The author(s) declare financial support was received for the research, authorship, and/or publication of this article. This work was supported by National Social Science Fund of China Youth Project under Grant (19CFX063). The funder was not involved in the study design, collection, analysis, interpretation of data, the writing of this article, or the decision to submit it for publication.
The authors declare that the research was conducted in the absence of any commercial or financial relationships that could be construed as a potential conflict of interest.
All claims expressed in this article are solely those of the authors and do not necessarily represent those of their affiliated organizations, or those of the publisher, the editors and the reviewers. Any product that may be evaluated in this article, or claim that may be made by its manufacturer, is not guaranteed or endorsed by the publisher.
1. Klimek, P, Baltic, D, Brunner, M, Degelsegger-Marquez, A, Garhöfer, G, Gouya-Lechner, G, et al. Quality criteria for real-world data in pharmaceutical research and health care decision-making: austrian expert consensus. JMIR Med Inform. (2022) 10:e34204. doi: 10.2196/34204
2. Grimberg, F, Asprion, PM, Schneider, B, Miho, E, Babrak, L, and Habbabeh, A. The real-world data challenges radar: a review on the challenges and risks regarding the use of real-world data. Digit Biomark. (2021) 5:148–57. doi: 10.1159/000516178
3. National Medical Products Administration Guidelines for real-world evidence to support drug development and review (trial). (2020). Available at: https://www.nmpa.gov.cn/yaopin/ypggtg/ypqtgg/20200107151901190.html (Accessed September 2, 2023).
4. Sun, X, Tan, J, Tang, L, Guo, JJ, and Li, XL. Real world evidence: experience and lessons from China. BMJ. (2018) 360:j5262. doi: 10.1136/bmj.j5262
5. National Medical Products Administration (2020). Guideline on use of real-world data in medical device clinical evaluation (trial). Available at: https://www.nmpa.gov.cn/xxgk/ggtg/qtggtg/20201126090030150.html (Accessed September 2, 2023).
6. Wen, Y. Real-world study: from real-world data to real-world evidence. Transl Breast Cancer Res. (2020) 1:21. doi: 10.21037/tbcr-20-19
7. Togo, K, and Yonemoto, N. Real world data and data science in medical research: present and future. Jpn J Statis Data Sci. (2022) 5:769–81. doi: 10.1007/s42081-022-00156-0
8. Diallo, G, and Bordea, G. Novelty in public health and epidemiology informatics. Yearb Med Inform. (2022) 31:273–5. doi: 10.1055/s-0042-1742526
9. Arora, J, Mega, JL, Abernethy, A, and Stadtlander, W. Connecting real-world data to support public health efforts. NEJM Catalyst. (2022). doi: 10.1056/CAT.22.0040
10. Branda, F, Pierini, M, and Mazzoli, S. Monkeypox: EpiMPX surveillance system and open data with a special focus on European and Italian epidemic. J Clin Virol Plus. (2022) 2:100114. doi: 10.1016/j.jcvp.2022.100114
11. Canfell, OJ, Kodiyattu, Z, Eakin, E, Burton-Jones, A, Wong, I, Macaulay, C, et al. Real-world data for precision public health of noncommunicable diseases: a scoping review. BMC Public Health. (2022) 22:2166. doi: 10.1186/s12889-022-14452-7
12. Sarovar, V, Malig, BJ, and Basu, R. A case-crossover study of short-term air pollution exposure and the risk of stillbirth in California, 1999-2009. Environ Res. (2020) 191:110103. doi: 10.1016/j.envres.2020.110103
14. Li, P, Wang, S, and Chen, Y. Use of real-world evidence for drug regulatory decisions in China: current status and future directions. Ther Innov Regul Sci. (2023). doi: 10.1007/s43441-023-00555-9
15. Yin, Y, Gong, J, and Li, JS. Application of antibacterial drugs in neurosurgery patients in Shenyang city, 2015-2020: a retrospective single-center study. Chin J Public Health. (2021) 37:568–9. doi: 10.11847/zgggws1134565
16. Cheng, S. China launches huge COVID drugs study. (2023). Available at: https://www.chinadaily.com.cn/a/202306/09/WS64832d09a31033ad3f7bb75f.html (Accessed June 30, 2023).
17. Li, J, Liu, L, Cao, H, Yang, M, and Sun, X. Use of real-world evidence to support regulatory decisions on medical devices in China and a unique opportunity to gain accelerated approval in “Boao Lecheng pilot zone”. Cost Eff Resour Alloc. (2023) 21:7. doi: 10.1186/s12962-022-00412-w
18. Aljunid, SM, Srithamrongsawat, S, Chen, W, Bae, SJ, Pwu, RF, Ikeda, S, et al. Health-care data collecting, sharing, and using in Thailand, China mainland, South Korea, Taiwan, Japan, and Malaysia. Value Health. (2012) 15:S132–8. doi: 10.1016/j.jval.2011.11.004
19. Li, M, Chen, S, Lai, Y, Liang, Z, Wang, J, Shi, J, et al. Integrating real-world evidence in the regulatory decision-making process: a systematic analysis of experiences in the US, EU, and China using a logic model. Front Med. (2021) 8:669509. doi: 10.3389/fmed.2021.669509
20. d'Elia, A, Gabbay, M, Rodgers, S, Kierans, C, Jones, E, Durrani, I, et al. Artificial intelligence and health inequities in primary care: a systematic scoping review and framework. Fam Med Commun Health. (2022) 10:e001670. doi: 10.1136/fmch-2022-001670
21. Xie, J, Wu, EQ, Wang, S, Cheng, T, Zhou, Z, Zhong, J, et al. Real-world data for healthcare research in China: call for actions. Value Health Reg Issues. (2022) 27:72–81. doi: 10.1016/j.vhri.2021.05.002
22. Storm, NE, Chang, W, Lin, TC, Lange, JL, Bradbury, B, Critchlow, CW, et al. A novel case study of the use of real-world evidence to support the registration of an osteoporosis product in China. Ther Innov Regul Sci. (2022) 56:137–44. doi: 10.1007/s43441-021-00342-4
23. He, ZC. When data protection norms meet digital health technology: China’s regulatory approaches to health data protection. Comput Law Secur Rev. (2022) 47:105758. doi: 10.1016/j.clsr.2022.105758
24. Zhong, J, Zhang, J, Fang, H, Liu, L, Xie, J, and Wu, E. Advancing the development of real-world data for healthcare research in China: challenges and opportunities. BMJ Open. (2022) 12:e063139. doi: 10.1136/bmjopen-2022-063139
25. Muscat, D, Hinton, R, Nutbeam, D, Kenney, E, Kuruvilla, S, and Jakab, Z. Universal health information is essential for universal health coverage. Fam Med Commun Health. (2023) 11:e002090. doi: 10.1136/fmch-2022-002090
26. Center of Drug Evaluation of National Medical Products Administration. Technical guideline for real-world data to generate real-world evidence (trial) (2021). Available at: https://www.cde.org.cn/main/news/viewInfoCommon/2a1c437ed54e7b838a7e86f4ac21c539 (Accessed September 2, 2023).
27. Wang, LM. Basic issues in the protection of sensitive personal information. Contemp Law Rev. (2022) 1:3–14.
28. Quinn, P, and Malgieri, G. The difficulty of defining sensitive data—the concept of sensitive data in the EU data protection framework. German Law J. (2021) 22:1583–612. doi: 10.1017/glj.2021.79
29. Van Kolfschooten, H, and De Ruijter, A. COVID-19 and privacy in the European Union: a legal perspective on contact tracing. Contemp Secur Pol. (2020) 41:478–91. doi: 10.1080/13523260.2020.1771509
30. Wang, K, and Muennig, P. Realizing the promise of big data: how Taiwan can help the world reduce medical errors and advance precision medicine. Appl Comput Inform. (2022). doi: 10.1108/ACI-11-2021-0298
31. Crane, G, Lim, JCW, Gau, CS, Xie, J, and Chu, L. The challenges and opportunities in using real-world data to drive advances in healthcare in East Asia: expert panel recommendations. Curr Med Res Opin. (2022) 38:1543–51. doi: 10.1080/03007995.2022.2096354
32. LinkDoc (2023). Artificial intelligence and medical big data solution provider. Available at: https://www.linkdoc.com (Accessed September 3, 2023).
33. Hjollund, NHI, Valderas, JM, Kyte, D, and Calvert, MJ. Health data processes: a framework for analyzing and discussing efficient use and reuse of health data with a focus on patient-reported outcome measures. J Med Internet Res. (2019) 21:e12412. doi: 10.2196/12412
34. Thouvenin, F. Informational self-determination: a convincing rationale for data protection law? J Intellect Property Inform Technol Electron Commerce Law. (2021) 12:246–56.
35. California Legislative Information (2023). Assembly Bill No. 375. Available at: https://leginfo.legislature.ca.gov/faces/billTextClient.xhtml?bill_id=201720180AB375 (Accessed June 30, 2023).
37. Scott, CR. Anonymity in applied communication research: tensions between IRBs, researchers, and human subjects. J Appl Commun Res. (2005) 33:242–57. doi: 10.1080/00909880500149445
38. Bentzen, HB, Castro, R, Fears, R, Griffin, G, Ter Meulen, V, and Ursin, G. Remove obstacles to sharing health data with researchers outside of the European Union. Nat Med. (2021) 27:1329–33. doi: 10.1038/s41591-021-01460-0
39. Ohno-Machado, L, Vinterbo, S, and Dreiseitl, S. Effects of data anonymization by cell suppression on descriptive statistics and predictive modeling performance. J Am Med Inform Assoc. (2002) 9:115S–9S. doi: 10.1197/jamia.M1241
40. Demetzou, K. Data protection impact assessment: a tool for accountability and the unclarified concept of 'high risk' in the general data protection regulation. Comput Law Secur Rev. (2019) 35:105342. doi: 10.1016/j.clsr.2019.105342
41. Sun, QB. The Speicial institutional logics of sensitive personal information protection and its regulation strategy. Adm Law Rev. (2022) 1:119–30.
42. Ploug, T, and Holm, S. Meta consent—a flexible solution to the problem of secondary use of health data. Bioethics. (2016) 30:721–32. doi: 10.1111/bioe.12286
43. Cumyn, A, Barton, A, Dault, R, Safa, N, Cloutier, AM, and Ethier, JF. Meta-consent for the secondary use of health data within a learning health system: a qualitative study of the public’s perspective. BMC Med Ethics. (2021) 22:81. doi: 10.1186/s12910-021-00647-x
44. Manson, NC. The biobank consent debate: why ‘meta-consent’ is not the solution? J Med Ethics. (2019) 45:291–4. doi: 10.1136/medethics-2018-105007
45. Ploug, T, and Holm, S. Meta consent: a flexible and autonomous way of obtaining informed consent for secondary research. BMJ. (2015) 350:h2146. doi: 10.1136/bmj.h2146
46. Ploug, T, and Holm, S. The biobank consent debate: why 'meta-consent' is still the solution! J Med Ethics. (2019) 45:295–7. doi: 10.1136/medethics-2018-105258
47. Ploug, T, and Holm, S. The four dimensions of contestable AI diagnostics—a patient-centric approach to explainable AI. Artif Intell Med. (2020) 107:101901. doi: 10.1016/j.artmed.2020.101901
48. Kaye, J, Whitley, EA, Lund, D, Morrison, M, Teare, H, and Melham, K. Dynamic consent: a patient interface for twenty-first century research networks. Eur J Hum Genet. (2015) 23:141–6. doi: 10.1038/ejhg.2014.71
49. Liu, C, Wang, D, Liu, C, Jiang, J, Wang, X, Chen, H, et al. What is the meaning of health literacy? A systematic review and qualitative synthesis. Fam Med Commun Health. (2020) 8:e000351. doi: 10.1136/fmch-2020-000351
50. Manson, NC. The case against meta-consent: not only do Ploug and Holm not answer it, they make it even stronger. J Med Ethics. (2020) 46:627–8. doi: 10.1136/medethics-2019-105955
51. Li, RS. On the mechanism of anonymization of personal medical information: with the relevant provisions of personal information protection law. SJTU Law Rev. (2022) 4:122–36. doi: 10.19375/j.cnki.31-2075/d.2022.04.001
52. Li, XJ, Cong, YL, and Liu, RS. Research under China’s personal information law. Science. (2022) 378:713–5. doi: 10.1126/science.abq7402
Keywords: personal information, health data, cross-border data transfer, privacy, real world data
Citation: Yao Y and Yang F (2023) Overcoming personal information protection challenges involving real-world data to support public health efforts in China. Front. Public Health. 11:1265050. doi: 10.3389/fpubh.2023.1265050
Received: 21 July 2023; Accepted: 11 September 2023;
Published: 22 September 2023.
Edited by:
Ulises Cortés, Universitat Politecnica de Catalunya, SpainReviewed by:
Yibo Wu, Peking University, ChinaCopyright © 2023 Yao and Yang. This is an open-access article distributed under the terms of the Creative Commons Attribution License (CC BY). The use, distribution or reproduction in other forums is permitted, provided the original author(s) and the copyright owner(s) are credited and that the original publication in this journal is cited, in accordance with accepted academic practice. No use, distribution or reproduction is permitted which does not comply with these terms.
*Correspondence: Fei Yang, aXRsYXdfeWFuZ0AxNjMuY29t
Disclaimer: All claims expressed in this article are solely those of the authors and do not necessarily represent those of their affiliated organizations, or those of the publisher, the editors and the reviewers. Any product that may be evaluated in this article or claim that may be made by its manufacturer is not guaranteed or endorsed by the publisher.
Research integrity at Frontiers
Learn more about the work of our research integrity team to safeguard the quality of each article we publish.