- 1Department of Medical Sciences, University College of Science and Technology, Gaza, Palestine
- 2School of Public Health, Department of Social and Preventive Medicine, University of Montreal, Montréal, QC, Canada
Even though the Gaza Strip is a low pulmonary tuberculosis (TB) burden region, it is well-known that TB is primarily a socioeconomic problem associated with overcrowding, poor hygiene, a lack of fresh water, and limited access to healthcare, which is the typical case in the Gaza Strip. Therefore, this study aimed at assessing the accuracy of the automatic software computer-aided detection for tuberculosis (CAD4TB) in diagnosing pulmonary TB on chest radiography and compare the CAD4TB software reading with the results of geneXpert. Using a census sampling method, the study was conducted in radiology departments in the Gaza Strip hospitals between 1 December 2022 and 31 March 2023. A digital X-ray, printer, and online X-ray system backed by CAD4TBv6 software were used to screen patients with lower respiratory tract symptoms. GeneXpert analysis was performed for all patients having a score > 40. A total of 1,237 patients presenting with lower respiratory tract symptoms participated in this current study. Chest X-ray readings showed that 7.8% (n = 96) were presumptive for TB. The CAD4TBv6 scores showed that 11.8% (n = 146) of recruited patients were presumptive for TB. GeneXpert testing on sputum samples showed that 6.2% (n = 77) of those with a score > 40 on CAD4TB were positive for pulmonary TB. Significant differences were found in chest X-ray readings, CAD4TBv6 scores, and GeneXpert results among sociodemographic and health status variables (P-value < 0.05). The study showed that the incidence rate of TB in the Gaza Strip is 3.5 per 100,000 population in the Gaza strip. The sensitivity of the CAD4TBv6 score and the symptomatic review for tuberculosis with a threshold score of >40 is 80.2%, and the specificity is 94.0%. The positive Likelihood Ratio is 13.3%, Negative Likelihood Ratio is 0.2 with 7.8% prevalence. Positive Predictive Value is 52.7%, Negative Predictive Value is 98.3%, and accuracy is 92.9%. In a resource-limited country with a high burden of neglected disease, combining chest X-ray readings by CAD4TB and symptomatology is extremely valuable for screening a population at risk. CAD4TB is noticeably more efficient than other methods for TB screening and early diagnosis in people who would otherwise go undetected.
Introduction
Tuberculosis (TB) stands out as the most widespread illness attributed to a single infectious agent, holding a place among the top 10 leading causes of death worldwide. Although TB can be prevented and treated, it impacts individuals of all age groups. In the year 2019 alone, nearly 10 million people across the globe contracted TB. Among them were 5.6 million males, 3.2 million females, and 1.2 million children (1).
Plain chest radiography remains a crucial tool in identifying early-stage pulmonary tuberculosis (TB) and monitoring the progress of treatments (2). Even when TB patients exhibit no symptoms, chest X-rays (CXRs) exhibit a high degree of sensitivity in detecting abnormalities related to pulmonary TB, particularly when interpreted by proficient radiologists. However, despite this capability, only 7.1 million out of an estimated 10 million TB cases worldwide were actually detected and reported in 2019 (3). Despite a decline in the global incidence rates of TB, these rates still fall short of the targets established by the World Health Organization's (WHO) End TB Strategy (3).
While improvements in digital radiography technology have improved the CXR image quality (4), lack of access to these facilities and skilled radiologists continues to be a problem, especially in underdeveloped regions with a high TB prevalence (5). However, the role of artificial intelligence (AI) in enhancing the accuracy of computer-aided diagnosis for pulmonary tuberculosis (TB) on chest radiography has become increasingly pivotal. AI technologies, such as deep learning algorithms, offer a unique capacity to analyze vast amounts of medical imagery with remarkable precision and speed. In the context of TB diagnosis, AI systems can swiftly and accurately detect subtle abnormalities and patterns on chest X-rays that may elude even skilled human radiologists. By providing reliable and consistent assessments, AI-driven computer-aided diagnosis has the potential to significantly expedite the identification of TB cases, especially in asymptomatic patients, ultimately leading to more timely interventions and improved treatment outcomes. This symbiotic integration of AI with medical diagnostics not only augments the overall diagnostic accuracy but also holds promise for more efficient resource utilization within healthcare systems, thus reinforcing its significance in combating pulmonary tuberculosis on a global scale (6–9). If they perform accurately, these CAD systems may facilitate CXR reading for TB screening and advance the WHO's End TB agenda (3, 10). There are only a few studies in this field, and the majority have methodological flaws, focus on a single CAD program, have scant screening data, or are industry-funded (11, 12).
Furthermore, most studies compared performance against a suboptimal reference standard of a single sputum specimen tested with Xpert MTB/RIF evaluated an online CAD processing system or shared images with the CAD vendors (13, 14). This highlights the need for independent and thorough studies. Offline and multiple AI systems have been the focus of more recent research (15–17), but there are still very few. An international meeting by WHO in 2016 concluded that more data on the effectiveness and application of CAD systems for TB screening were needed (18).
Although the Gaza Strip is a low TB burden region, it is well-known that tuberculosis is primarily a socioeconomic problem associated with overcrowding, poor hygiene, a lack of fresh water, and limited access to health care, which is typical in the Gaza Strip (19, 20). There is a lack of well-organized healthcare infrastructure, which affects the finding and treatment of TB cases, complicated disease control in the Gaza Strip, and a lack of statistics on TB after 2016.
Accordingly, this study aims to evaluate the performance of the automatic software computer-aided detection for tuberculosis (CAD4TBv6) in diagnosing pulmonary TB on chest radiography and compare the CAD4TB software reading with the results of radiologists' reports in the Gaza Strip, Palestine.
Methods
Study setting and period
The current study was conducted in radiology departments in the Gaza Strip hospitals between 1 December 2022 and 31 March 2023.
Study design and study participants
We conducted a cross-sectional study to recruit patients with lower respiratory tract symptoms. Data were collected from respiratory patients referred from the chest department who underwent digital CXRs during the study period using a census sampling method. A digital X-ray, printer, and online X-ray system backed by CAD4TBv6 software were used to screen patients. Patients of both sexes ranging in age from 15 to 80 years were included in this study.
Sociodemographic and clinical information tool
The acquisition of sociodemographic and clinical information including signs and symptoms of tuberculosis and a history of respiratory diseases was facilitated through the utilization of a meticulously crafted and rigorously validated questionnaire. The questionnaire's design was rooted in a thorough review of pertinent literature and established health assessment frameworks (21–25). This instrument was developed through a systematic process that involved collaboration with domain experts, iterative refinement, and comprehensive pilot testing.
Assessment of body mass index
Using a measuring rod attached to the balanced beam scale, participants' heights (measured in cm) were recorded to the nearest 0.5 cm while standing barefoot and with their heads up. A common digital weighing scale (SECA, Germany) was used to measure weight (kg). Participants were asked to remove their bulky outerwear before being weighed, and the results were recorded to the nearest 0.1 kg (26).
CXRs scoring procedures
The obtained CXRs were read within 48 h by a radiologist (certified by the Palestinian board) and classified as normal, probable TB, and non-TB-related. Digital CXRs were scored using CAD4TBv6 (Delft Imaging Systems, Veenendaal, The Netherlands), with scores from 0 to 100 (0 being completely normal and 100 very suggestive of TB) (27).
The analysis was based on identifying aberrant lung field shapes and textures using automatically segmented lung fields. The cutoff point of 40 was chosen (28). Patients who had CXRs images with a score equal to or <40 underwent clinical examination. Whereas, patients who had CXRs with a score higher than 40 were assumed to have tuberculosis. Sputum samples for GeneXpert analysis were obtained only from those with a score > 40 and with symptoms strongly suggestive of pulmonary TB such as hemoptysis, night sweat, weight loss, breathlessness, and fever (Figure 1).
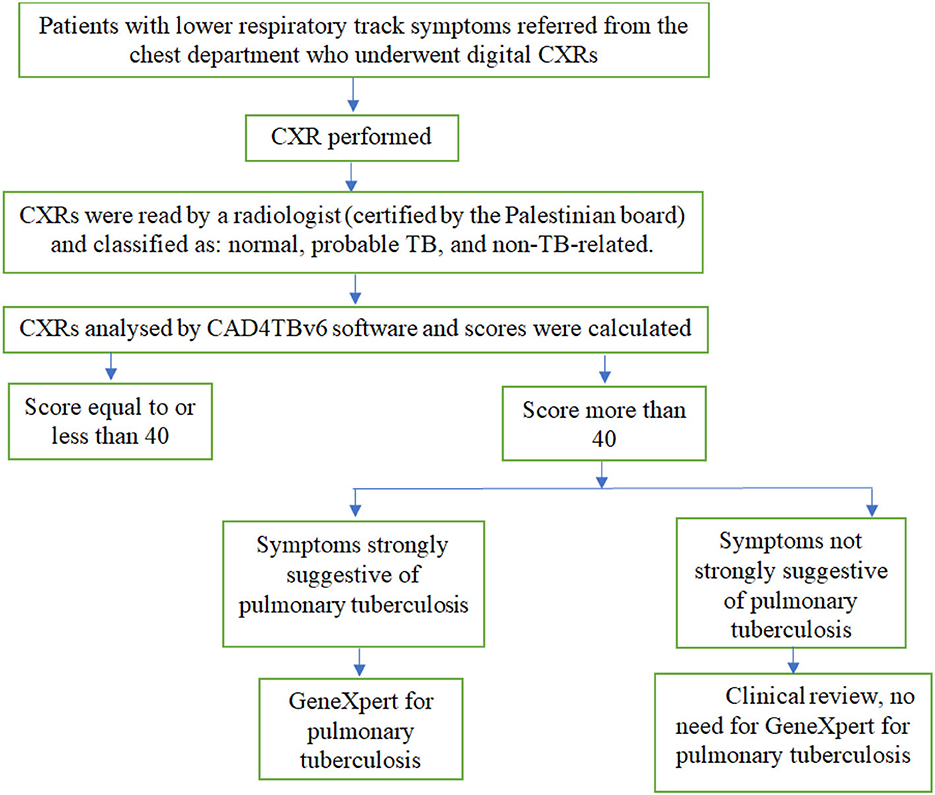
Figure 1. Tuberculosis screening flowchart for patients presenting with lower respiratory tract symptoms.
Statistical analysis
IBM SPSS Statistics for Windows, version 26.0 (IBM Corp., Armonk, NY, USA), was used for statistical analysis. We calculated the frequency and percentage for categorical variables. Continuous variables were analyzed using mean and SD. The chi-square test was used to determine the significant differences between categorical variables. With GeneXpert serving as the reference standard, the following metrics were used for CAD4TB: sensitivity (True Positives x 100/Total Diseased), specificity (True Negatives × 100/Total Non-Diseased), negative predictive value (True Negatives/True Negatives + False Negatives), and positive predictive value (True Positives/True Positives + False Positives).
Results
Sociodemographic and health status characteristics, CAD4TBv6 score, and GeneXpert result
A total of 1,237 patients presenting with lower respiratory tract symptoms participated in this current study. More than half of the study participants (56.4%) were males. 45.1% (n = 558) of study participants were 41–60 years old, with a mean age ± standard deviation of 47.4 ± 14.2 years. Only 22.1% of study participants (n = 273) had a typical BMI of 18.5–24.9, with a mean BMI±std of 30.1 ± 6. 2. 44.9% of the study participants were active tobacco smokers. 75.4% of study participants had a cough of any duration; only 20.0% of them were diabetic patients; 17.5% had hemoptysis; 36.6% had a night sweat; 43.2% experienced weight loss; 79.9% suffered from breathlessness; and 39.7% had a fever. Only 10% of study participants had a past history of TB. Chest X-ray readings showed that 64.8% (n = 801) were normal, 27.5% (n = 340) were abnormal but not TB and only 7.8% (n = 96) were presumptive for TB. There were statistically significant differences in chest X-ray readings between age groups, diabetes status, hemoptysis, night sweats, weight loss, fever, and TB history (P-value 0.05).
The CAD4TBv6 scores showed that 11.8% (n = 146) of recruited patients were presumptive for TB. Significant differences in CAD4TBv6 scores were discovered across age groups, diabetes status, hemoptysis, night sweats, weight loss, fever, and TB history (P-value < 0.05).
GeneXpert testing on sputum samples showed that 6.2% (n = 77) of those with a score > 40 on CAD4TB were positive for pulmonary TB. Significant differences were found in GeneXpert results among age groups, hemoptysis, night sweats, weight loss, fever, and history of TB (P-value < 0.05).
The study showed that the incidence rate of TB in the Gaza Strip is 3.5 per 100,000 population in the Gaza strip (Table 1).
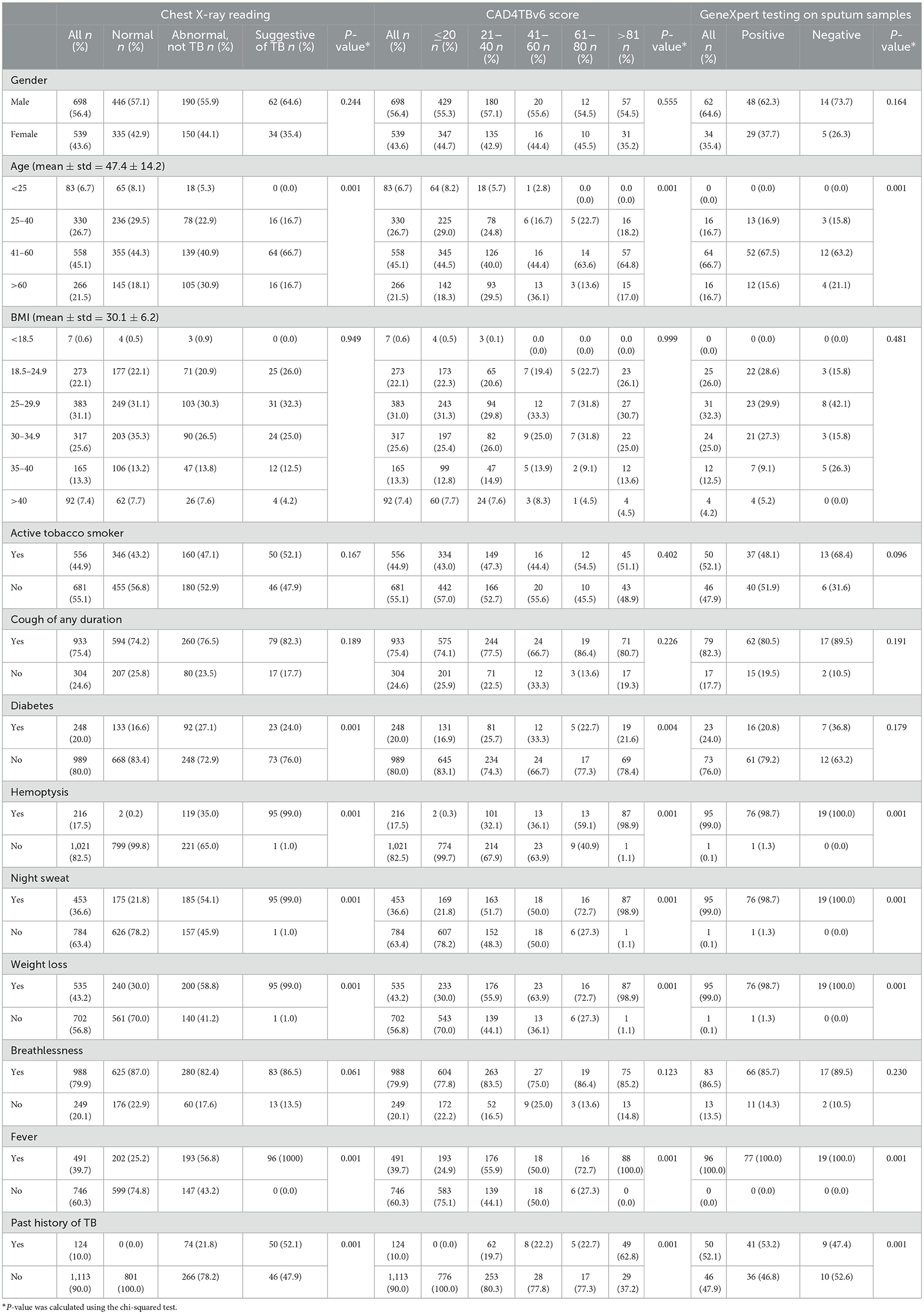
Table 1. Sociodemographic and health status characteristics of patients with presumptive TB by chest x-ray reading, CAD4TB scores, and GeneXpert testing.
The sensitivity of the CAD4TBv6 score and the symptomatic review for tuberculosis with a threshold score of >40 is 80.2%, and the specificity is 94.0%. The positive likelihood ratio is 13.3%, negative likelihood ratio is 0.2, with a 7.8% prevalence. The positive predictive value is 52.7%, the negative predictive value is 98.3%, and the accuracy is 92.9% (Table 2).
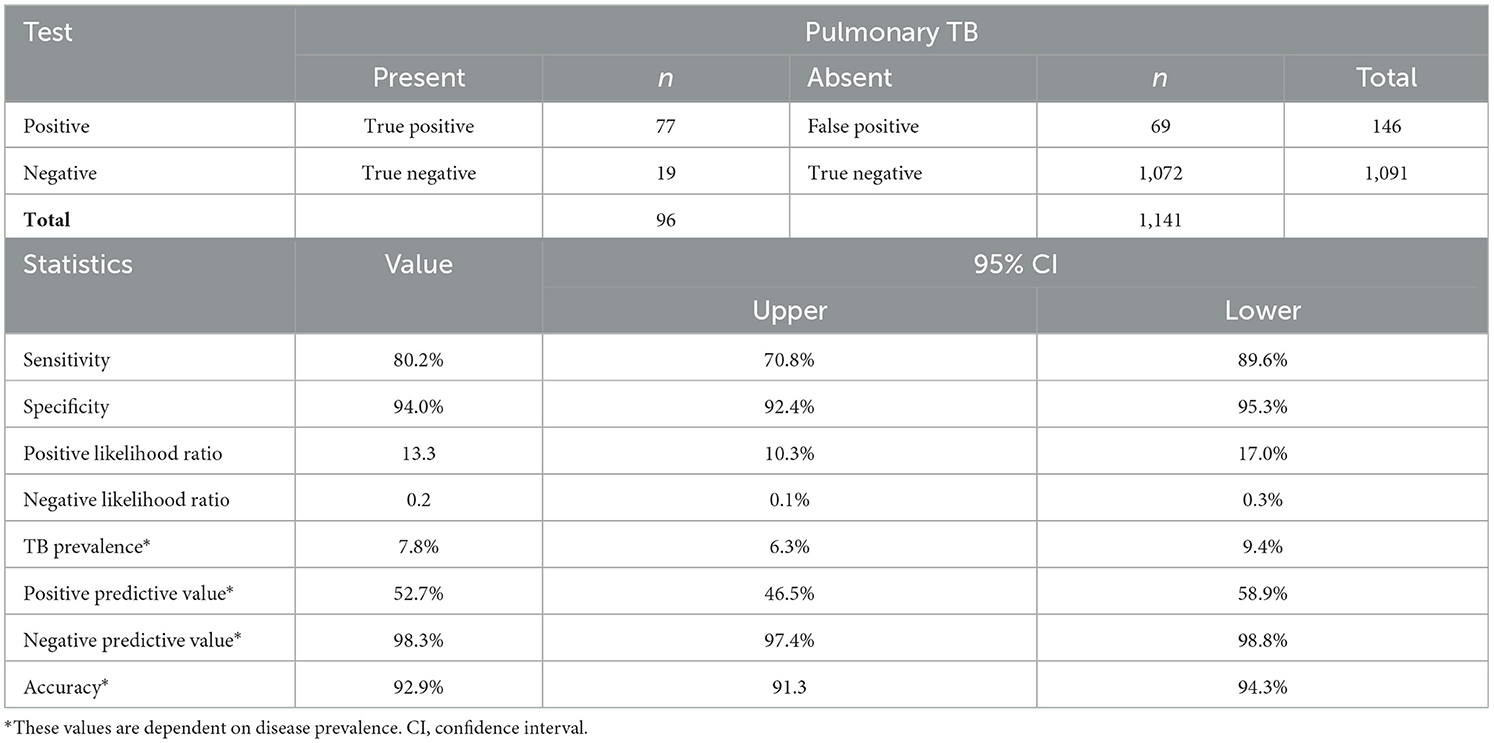
Table 2. Estimated diagnostic accuracy of CAD4TBv6 software in diagnosing pulmonary TB on chest radiography with a threshold score of >40.
CAD4TB analysis
Machine learning methods are used by the commercial software package CAD4TB to automatically identify TB from CXR pictures. Using separate annotated datasets, the software has been trained to recognize recognizable TB features in CXR pictures. It generates a number (0–100) that can be interpreted as the likelihood that the person has active TB that can be seen on CXR. Figure 2 shows an anomaly heatmap showing areas the software deems suspicious.
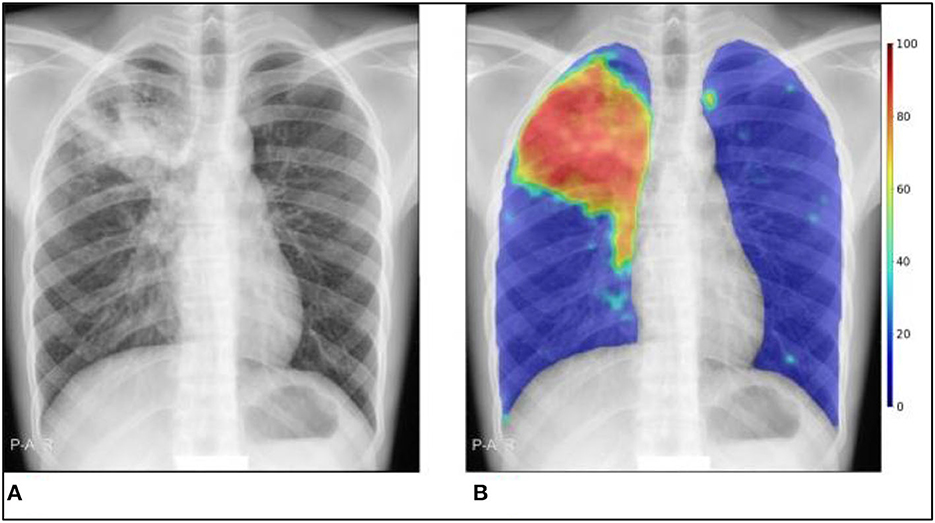
Figure 2. CAD4TB v6 output example. (A) Original CXR, (B) CXR with abnormality heatmap overlay. The Xpert test was positive, and the final composite CAD4TB score for this person was 91.7 (0 = normal, 100 = most abnormal).
Figure 3 shows a few instances in which the radiograph's appearance conflicts with the outcome of the geneXpert test. The first case shows a radiograph that appears consistent with tuberculosis. Still, the geneXpert test was negative, and the second case shows a normal radiograph, but the subject had a positive geneXpert test result. Both times, CAD4TB functions as anticipated and under-qualified observers. The causes of the discrepancy between these specific cases' geneXpert results and radiograph appearance are unknown.
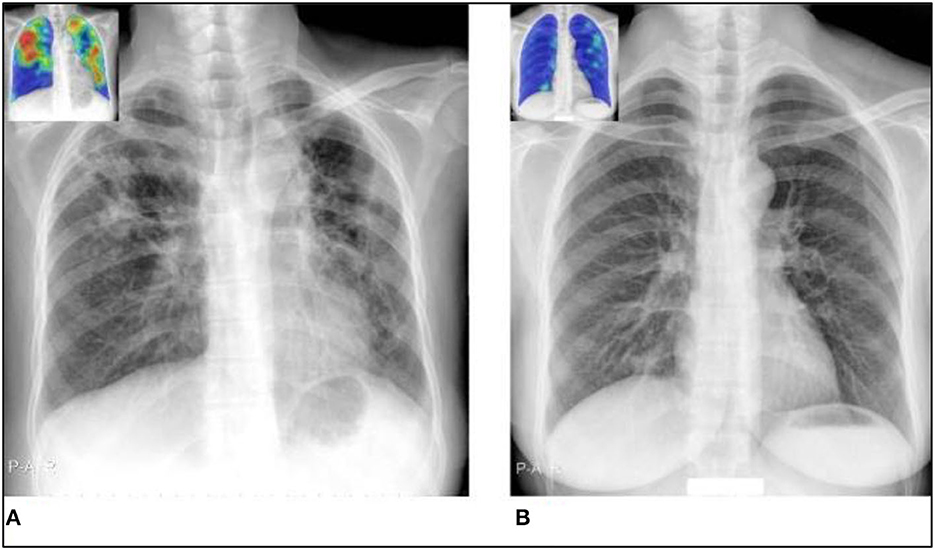
Figure 3. Situations in which the radiograph presentation does not match the geneXpert result, making radiograph-only prediction challenging for both observers and CAD4TB. The CAD4TB heatmaps are displayed in the inset photos, with blue denoting the majority of normal texture and red denoting the majority of abnormal texture. (A) A geneXpert-negative case identified by all five observers as TB positive (score 3) and by CAD4TB v6 (score = 100). (B) A geneXpert-positive case that received a score of 1 (no-TB) from four experts and a score of 2 from the final expert. When a sensitivity of 99% is reached, the 18.7 CAD4TB score for this case indicates that TB has not yet been detected.
In contrast, Figure 4 illustrates two simple situations where the results of the geneXpert and the radiograph (as evaluated by CAD4TB and radiologists) agreed.
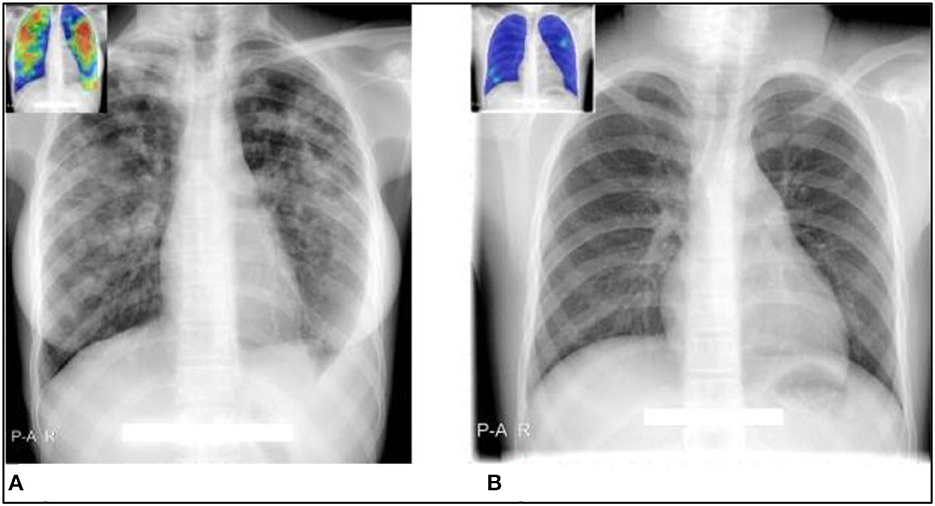
Figure 4. Cases where CAD4TB and observers' interpretations of radiographs agree well with geneXpert results. The CAD4TB heatmaps are displayed in the inset photos, with blue denoting the majority of normal texture and red denoting the majority of abnormal texture. (A) A case that tested positive for TB using geneXpert was rated as such by all five observers and CAD4TB v6 (score = 91.7). (B) A case that received a score of one (no-TB) from all five experts was geneXpert-negative. The case's CAD4TB score is 7.1.
Discussion
To the best of our knowledge, this is the first study to evaluate the performance of the automatic software computer-aided detection for tuberculosis (CAD4TBv6) in diagnosing pulmonary TB on chest radiography and compare the CAD4TB software reading with the results of radiologists' reports in the Gaza strip, Palestine. Sputum samples were obtained from those with a score > 40 for GeneXpert analysis and those with symptoms strongly suggestive of pulmonary TB. A certified radiologist read the obtained CXRs.
Although the utilization of Xpert in programmed applications has increased recently, the WHO has also advocated using screening instruments like CXR that employ more affordable diagnostic algorithms (17, 29–32). Using an automated system to analyze a chest radiograph for the presence of active pulmonary TB produces objective, repeatable results and a consistent format for reporting. Creating software that provides automated CXR interpretation is a significant step toward connecting technology advancements to mass-screening initiatives for TB (23). In addition to increasing case identification in screening programs, using CAD4TB as a triage tool to pre-screen people for Xpert may help lower program expenses (33). Those with low CAD4TB scores had a low likelihood of testing positive for TB, so they might not be prioritized for Xpert testing using this method. Employing a triage tool such as CAD4TB might encourage more judicious use of Xpert by reducing the number of cartridges used in resource-constrained environments where there is not enough money to cover testing for all individuals with presumptive TB. This also applies to settings where onsite radiologists might not always be present to review CXRs. It is important to bear in mind that the costs associated with acquiring and operating digital X-ray devices must be balanced against the potential savings resulting from a reduced need for Xpert exams. This underscores the need for a comprehensive analysis that examines both the financial implications and consequences of widespread mass-screening through chest X-rays (CXR) (25).
According to research by Gautam et al. diabetes has been proven to increase both the likelihood of contracting TB and the severity of the illness (34). Additionally, research has demonstrated that smoking contributes significantly to the development of TB and raises the severity and fatality rates (35). However, our findings were in line with Tavaziva et al. as smoking and diabetes do not seem to make TB more likely to strike or to progress more severely (22).
According to 12 single-center assessment studies, the WHO estimates that Xpert's pooled sensitivity and specificity values for the detection of TB are 92.5 and 98%, respectively (36). However, the accessibility of digital radiography is a need for CAD utilization, which is not yet available in most resource-constrained low-burden settings. Nevertheless, it has been determined to be viable. It produces chest radiography significantly superior to traditional X-ray equipment in regions with low resources, such as Gaza Strip (37).
The findings of the current study of high specificity, high negative predictive value, high sensitivity, high diagnostic accuracy, and relatively low positive predictive value were consistent with findings from other studies in different settings testing CAD4TB (24, 25, 27, 38, 39). Compared to a confirmatory test like the Xpert, triage tests should have a sensitivity of 90% and a specificity of 70%, according to a 2014 WHO consensus meeting to define targets for new TB diagnostic technologies (40).
The findings of this study point to a feasible, effective, and even cost-effective strategy for TB screening in a symptomatic group that combines CAD4TB and symptomatology. Earlier research from different contexts are consistent with our findings (41–43).
The direct comparison between computerized and radiologist reading on the same set of pictures is one of the study's strengths. The extent to which this comparison may be generalized is severely constrained by the inter-reader variance in the reading of chest X-rays and the possibility of involving only one board-certified radiologist. The fact that our short-term study was only done in a single low-burden region presents a second limit that Data gathered from patients who presented with lower respiratory tract symptoms may not accurately reflect the prevalence of illness in the general community.
It is imperative that forthcoming research endeavors direct their attention toward comprehensively examining the multifaceted ramifications encompassing the adoption of Computer-Aided Diagnosis (CAD) within diverse low-burden nations. In particular, a rigorous investigation is warranted to elucidate the intricate interplay of financial, practical, and ethical considerations inherent in the deployment of CAD within these unique contexts. Subsequent investigative trajectories should encompass an exploration of the potential synergies arising from amalgamating CAD-generated outcomes with an array of clinical parameters, encompassing symptomatic manifestations and risk profiling. Furthermore, a profound research agenda should be undertaken to systematically assess the efficacy of CAD not only within the spectrum of operational feasibility but also across various technical dimensions indispensable for its seamless integration into prevailing diagnostic frameworks. Concurrently, an evaluative lens should be directed toward pioneering CAD products that are emergent within the market landscape. This comprehensive inquiry stands to provide a robust foundation for harnessing the maximal potential of CAD, charting its trajectory toward optimized medical diagnostics within the distinctive landscape of low-burden nations.
Conclusion
Combining chest X-ray readings by CAD4TB and symptomatology is extremely valuable for screening a population at risk in a resource-limited country with a high burden of a neglected disease. CAD4TB is noticeably more efficient than other methods for TB screening and early diagnosis in people who would otherwise go undetected. In order to increase case finding and infection control and lower the cost of case detection within triage algorithms, CAD solutions may present an opportunity. This inspires further investigation into the best ways to utilize its potential as a support tool for clinical officers in the diagnostic interpretation of radiographs as well as a stand-alone triage test in systematic screening settings.
Data availability statement
The raw data supporting the conclusions of this article will be made available by the authors, without undue reservation.
Ethics statement
The study protocol was approved by the Palestinian Health Research Council (Helsinki Committee for Ethical Approval research number: PHRC/HC/1175/22). Additional approval was obtained from the included Gaza Strip hospitals. Study participants provided written informed consent for survey activities.
Author contributions
SA: Conceptualization, Data curation, Formal analysis, Funding acquisition, Investigation, Methodology, Project administration, Resources, Software, Supervision, Validation, Visualization, Writing — original draft, Writing — review & editing. KZ: Methodology, Project administration, Software, Validation, Visualization, Writing — original draft, Writing — review & editing.
Funding
This research was funded by the WHO-EMRO, Unit Reference: AP-22-00673.
Acknowledgments
The authors are grateful to the World Health Organization-Regional Office for the Eastern Mediterranean (WHO-EMRO) and Development Pioneers Company for Consultation (Pioneers) for supporting the implementation of the study. The certified radiologist, hospitals, and patients who participated in the study are all thanked for their vital contributions to conducting the study.
Conflict of interest
The authors declare that the research was conducted in the absence of any commercial or financial relationships that could be construed as a potential conflict of interest.
Publisher's note
All claims expressed in this article are solely those of the authors and do not necessarily represent those of their affiliated organizations, or those of the publisher, the editors and the reviewers. Any product that may be evaluated in this article, or claim that may be made by its manufacturer, is not guaranteed or endorsed by the publisher.
References
1. WHO. Tuberculosis. World Health Organization (2022). Available online at: https://www.who.int/en/news-room/fact-sheets/detail/tuberculosis (accessed January 12, 2023).
2. WHO. Chest Radiography in Tuberculosis Detection -Summary of Current WHO Recommendations and Guidance on Programmatic Approaches. World Health Organization (2016). p. 1–44. Available online at: https://www.who.int/tb/publications/Radiography_TB_factsheet.pdf?ua=1 (accessed January 14, 2023).
3. WHO. Global Tuberculosis Report Executive Summary 2020. World Health Organization (2022). p. 1–11. Available online at: https://www.who.int/tb/publications/global_report/TB20_Exec_Sum_20201014.pdf?ua=1 (accessed January 15, 2023).
4. Bansal G. Digital radiography. A comparison with modern conventional imaging. Postgr Med J. (2006) 82:425–8. doi: 10.1136/pgmj.2005.038448
5. Pande T, Pai M, Khan FA, Denkinger CM. Use of chest radiography in the 22 highest tuberculosis burden countries. Eur Respir J. (2015) 46:1816–9. doi: 10.1183/13993003.01064-2015
6. Chartrand G, Cheng PM, Vorontsov E, Drozdzal M, Turcotte S, Pal CJ, et al. Deep learning: a primer for radiologists. Radiographics. (2017) 37:2113–31. doi: 10.1148/rg.2017170077
7. Lakhani P, Sundaram B. Deep learning at chest radiography: automated classification of pulmonary tuberculosis by using convolutional neural networks. Radiology. (2017) 284:574–82. doi: 10.1148/radiol.2017162326
8. Harris M, Qi A, Jeagal L, Torabi N, Menzies D, Korobitsyn A, et al. systematic review of the diagnostic accuracy of artificial intelligence-based computer programs to analyze chest x-rays for pulmonary tuberculosis. PLoS ONE. (2019) 14:e0221339. doi: 10.1371/journal.pone.0221339
9. Gonem S, Janssens W, Das N, Topalovic M. Applications of artificial intelligence and machine learning in respiratory medicine. Thorax. (2020) 75:695–701. doi: 10.1136/thoraxjnl-2020-214556
10. Khan FA, Pande T, Tessema B, Song R, Benedetti A, Pai M, et al. Computer-aided reading of tuberculosis chest radiography: moving the research agenda forward to inform policy. Eur Respiratory Soc. (2017) 50. doi: 10.1183/13993003.00953-2017
11. Pande T, Cohen C, Pai M, Ahmad Khan F. Computer-aided detection of pulmonary tuberculosis on digital chest radiographs: a systematic review. Int J Tuberc Lung Dis. (2016) 20:1226–30. doi: 10.5588/ijtld.15.0926
12. Nash M, Kadavigere R, Andrade J, Sukumar CA, Chawla K, Shenoy VP, et al. Deep learning, computer-aided radiography reading for tuberculosis: a diagnostic accuracy study from a tertiary hospital in India. Sci Rep. (2020) 10:1–10. doi: 10.1038/s41598-019-56589-3
13. Fehr J, Konigorski S, Olivier S, Gunda R, Surujdeen A. Computer-aided interpretation of chest radiography to detect TB in rural South Africa 1. Age. (2021) 4:1–10.
14. Qin ZZ, Sander MS, Rai B, Titahong CN, Sudrungrot S, Laah SN, et al. Using artificial intelligence to read chest radiographs for tuberculosis detection: a multi-site evaluation of the diagnostic accuracy of three deep learning systems. Sci Rep. (2019) 9:1–10. doi: 10.1038/s41598-019-51503-3
15. Khan FA, Majidulla A, Tavaziva G, Nazish A, Abidi SK, Benedetti A, et al. Chest x-ray analysis with deep learning-based software as a triage test for pulmonary tuberculosis: a prospective study of diagnostic accuracy for culture-confirmed disease. Lancet Digital Health. (2020) 2:e573–81. doi: 10.1016/S2589-7500(20)30221-1
16. Qin ZZ, Ahmed S, Sarker MS, Paul K, Adel ASS, Naheyan T, et al. Can artificial intelligence (AI) be used to accurately detect tuberculosis (TB) from chest X-ray? A multiplatform evaluation of five AI products used for TB screening in a high TB-burden setting. medRxiv. (2020). doi: 10.48550/arXiv.2006.05509
17. Gelaw SM, Kik SV, Ruhwald M, Ongarello S, Egzertegegne TS, Gorbacheva O, et al. Diagnostic accuracy of three computer-aided detection systems for detecting pulmonary tuberculosis on chest radiography when used for screening: analysis of an international, multicenter migrants screening study. medRxiv. (2022). doi: 10.1101/2022.03.30.22273191
18. WHO. Chest Radiography in Tuberculosis Detection: Summary of Current WHO Recommendations and Guidance on Programmatic Approaches. World Health Organization (2016). p. 26–8. Available online at: https://apps.who.int/iris/bitstream/handle/10665/252424/9789241511506-eng.pdf?sequence=1&isAllowed=y (accessed January 15, 2023).
19. Abuzerr S, Nasseri S, Yunesian M, Hadi M, Mahvi AH, Nabizadeh R, et al. Prevalence of diarrheal illness and healthcare-seeking behavior by age-group and sex among the population of Gaza strip: a community-based cross-sectional study. BMC Public Health. (2019) 19:1–10. doi: 10.1186/s12889-019-7070-0
20. Abuzerr S, Nasseri S, Yunesian M, Hadi M, Zinszer K, Mahvi AH, et al. Water, sanitation, and hygiene risk factors of acute diarrhea among children under five years in the Gaza Strip. J Water Sanit Hyg Dev. (2020) 10:111–23. doi: 10.2166/washdev.2019.072
21. Mungai B, Ong ‘angò J, Ku CC, Henrion MY, Morton B, Joekes E, Onyango E, Kiplimo R, Kirathe D, Masini E. Accuracy of computer-aided chest X-ray screening in the Kenya National Tuberculosis Prevalence Survey. medRxiv. (2021). doi: 10.1101/2021.10.21.21265321
22. Tavaziva G, Majidulla A, Nazish A, Saeed S, Benedetti A, Khan AJ, et al. Diagnostic accuracy of a commercially available, deep learning-based chest X-ray interpretation software for detecting culture-confirmed pulmonary tuberculosis. Int J Infect Dis. (2022) 122:15–20. doi: 10.1016/j.ijid.2022.05.037
23. Breuninger M, van Ginneken B, Philipsen RH, Mhimbira F, Hella JJ, Lwilla F, et al. Diagnostic accuracy of computer-aided detection of pulmonary tuberculosis in chest radiographs: a validation study from sub-Saharan Africa. PLoS ONE. (2014) 9:e106381. doi: 10.1371/journal.pone.0106381
24. Murphy K, Habib SS, Zaidi SMA, Khowaja S, Khan A, Melendez J, et al. Computer aided detection of tuberculosis on chest radiographs: An evaluation of the CAD4TB v6 system. Sci Rep. (2020) 10:5492. doi: 10.1038/s41598-020-62148-y
25. Zaidi SMA, Habib SS, Van Ginneken B, Ferrand RA, Creswell J, Khowaja S, et al. Evaluation of the diagnostic accuracy of Computer-Aided Detection of tuberculosis on Chest radiography among private sector patients in Pakistan. Sci Rep. (2018) 8:12339. doi: 10.1038/s41598-018-30810-1
26. WHO. Physical Status: The Use and Interpretation of Anthropometry. Geneva: World Health Organization (1995).
27. Maduskar P, Muyoyeta M, Ayles H, Hogeweg L, Peters-Bax L, Van Ginneken B. Detection of tuberculosis using digital chest radiography: automated reading vs. interpretation by clinical officers. Int J Tuberc Lung Dis. (2013) 17:1613–20. doi: 10.5588/ijtld.13.0325
28. Fehr J, Konigorski S, Olivier S, Gunda R, Surujdeen A, Gareta D, et al. Computer-aided interpretation of chest radiography reveals the spectrum of tuberculosis in rural South Africa. NPJ Dig Med. (2021) 4:106. doi: 10.1101/2020.09.04.20188045
29. Philipsen R, Sánchez C, Maduskar P, Melendez J, Peters-Bax L, Peter J, et al. Automated chest-radiography as a triage for Xpert testing in resource-constrained settings: a prospective study of diagnostic accuracy and costs. Sci Rep. (2015) 5:12215. doi: 10.1038/srep12215
30. WHO. Systematic Screening for Active Tuberculosis: Principles And Recommendations. World Health Organization (2013). Available online at: https://books.google.com.pk/books?hl=-en&lr=-&id=-g7EXDAAAQBAJ&oi=-fnd&pg=PP1&dq=-Systematic+screening+for+active+-tuberculosis:+principles+and+recommendations.+&ots=BnNSCcvlv&sig=N5mkmTU0Ke24X9C5Y9Dxw1V83_M#v=onepage&q=Systematic%20screening%20for%20active%20tuberculosis%3A%20principles%20and%20recommendations.&f=false (accessed January 13, 2023).
31. Qin ZZ, Pai M, Van Gemert W, Sahu S, Ghiasi M, Creswell J. How is Xpert MTB/RIF being implemented in 22 high tuberculosis burden countries? Eur Respir J. (2015) 45:549–54. doi: 10.1183/09031936.00147714
32. Kagujje M, Kerkhoff AD, Nteeni M, Dunn I, Mateyo K, Muyoyeta M. The performance of computer-aided detection digital chest X-ray reading technologies for triage of active tuberculosis among persons with a history of previous tuberculosis. Clin Infect Dis. (2023) 76:e894–901. doi: 10.1093/cid/ciac679
33. Story A, Aldridge RW, Abubakar I, Stagg H, Lipman M, Watson J, et al. Active case finding for pulmonary tuberculosis using mobile digital chest radiography: an observational study. Int J Tuberc Lung Dis. (2012) 16:1461–7. doi: 10.5588/ijtld.11.0773
34. Gautam S, Shrestha N, Mahato S, Nguyen TP, Mishra SR, Berg-Beckhoff G. Diabetes among tuberculosis patients and its impact on tuberculosis treatment in South Asia: a systematic review and meta-analysis. Sci Rep. (2021) 11:2113. doi: 10.1038/s41598-021-81057-2
35. Khan AH, Sulaiman SAS, Hassali MA, Khan KU, Ming LC, Mateen O, et al. Effect of smoking on treatment outcome among tuberculosis patients in Malaysia; a multicenter study. BMC Public Health. (2020) 20:1–8. doi: 10.1186/s12889-020-08856-6
36. WHO. Xpert MTB/RIF Implementation Manual. World Health Organization (2014). Available online at: http://apps.who.int/iris/bitstream/handle/10665/112469/9789241506700_eng.pdf (accessed January 12, 2023).
37. Zennaro F, Oliveira Gomes JA, Casalino A, Lonardi M, Starc M, Paoletti P, et al. Digital radiology to improve the quality of care in countries with limited resources: a feasibility study from Angola. PLoS ONE. (2013) 8:e73939. doi: 10.1371/journal.pone.0073939
38. Theron G, Peter J, van Zyl-Smit R, Mishra H, Streicher E, Murray S, et al. Evaluation of the Xpert MTB/RIF assay for the diagnosis of pulmonary tuberculosis in a high HIV prevalence setting. Am J Respir Crit Care Med. (2011) 184:132–40. doi: 10.1164/rccm.201101-0056OC
39. van't Hoog AH, Meme H, Van Deutekom H, Mithika A, Olunga C, Onyino F, Borgdorff M. High sensitivity of chest radiograph reading by clinical officers in a tuberculosis prevalence survey. Int J Tuberc Lung Dis. (2011) 15:1308-1314. doi: 10.5588/ijtld.11.0004
40. WHO. High-Priority Target Product Profles for New Tuberculosis Diagnostics: Report of a Consensus Meeting. Tech. Rep. World Health Organization (2014). Available online at: http://apps.who.int/iris/bitstream/handle/10665/135617/WHO_HTM_TB_2014.18_eng.pdf (accessed January 16, 2023).
41. Fatima R, Qadeer E, Yaqoob A, Haq MU, Majumdar SS, Shewade HD, et al. Extending ‘contact tracing'into the community within a 50-metre radius of an index tuberculosis patient using Xpert MTB/RIF in urban, Pakistan: did it increase case detection? PLoS ONE. (2016) 11:e0165813. doi: 10.1371/journal.pone.0165813
42. Vo LNQ, Vu TN, Nguyen HT, Truong TT, Khuu CM, Pham PQ, et al. Optimizing community screening for tuberculosis: Spatial analysis of localized case finding from door-to-door screening for TB in an urban district of Ho Chi Minh City, Viet Nam. PLoS ONE. (2018) 13:e0209290. doi: 10.1371/journal.pone.0209290
Keywords: computer-aided detection, chest radiography, diagnostic accuracy, GeneXpert, Gaza Strip, pulmonary tuberculosis
Citation: Abuzerr S and Zinszer K (2023) Computer-aided diagnostic accuracy of pulmonary tuberculosis on chest radiography among lower respiratory tract symptoms patients. Front. Public Health 11:1254658. doi: 10.3389/fpubh.2023.1254658
Received: 07 July 2023; Accepted: 09 October 2023;
Published: 27 October 2023.
Edited by:
Ranjan Nanda, International Centre for Genetic Engineering and Biotechnology, IndiaReviewed by:
Mihaela-Simona Subtirelu, University of Medicine and Pharmacy of Craiova, RomaniaDebasis Dash, Institute of Life Sciences (ILS), India
Anjali Agrawal, Teleradiology Solutions, India
Copyright © 2023 Abuzerr and Zinszer. This is an open-access article distributed under the terms of the Creative Commons Attribution License (CC BY). The use, distribution or reproduction in other forums is permitted, provided the original author(s) and the copyright owner(s) are credited and that the original publication in this journal is cited, in accordance with accepted academic practice. No use, distribution or reproduction is permitted which does not comply with these terms.
*Correspondence: Samer Abuzerr, samer_516@hotmail.com