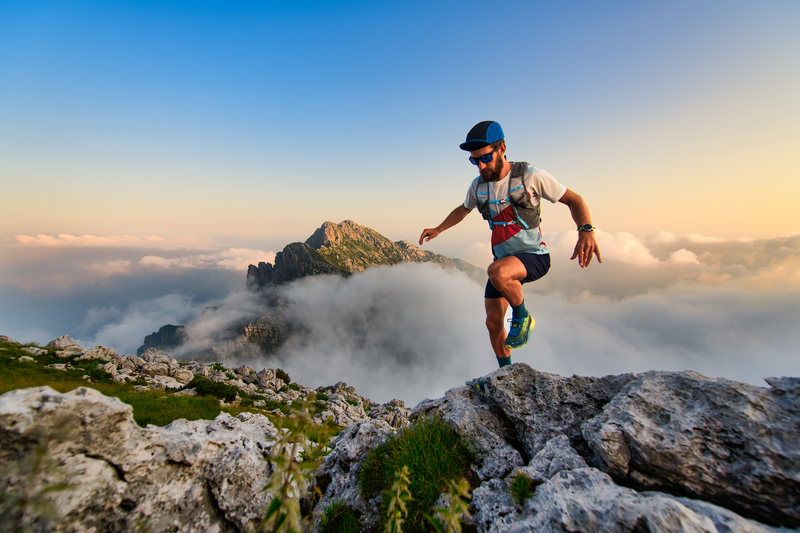
94% of researchers rate our articles as excellent or good
Learn more about the work of our research integrity team to safeguard the quality of each article we publish.
Find out more
PERSPECTIVE article
Front. Public Health , 13 October 2023
Sec. Aging and Public Health
Volume 11 - 2023 | https://doi.org/10.3389/fpubh.2023.1248254
This article is part of the Research Topic Experts' Opinions on Aging and Public Health View all 10 articles
Chronic illnesses are a major threat to global population health through the lifespan into older age. Despite world-wide public health goals, there has been a steady increase in chronic and non-communicable diseases (e.g., cancer, cardiovascular and metabolic disorders) and strong growth in mental health disorders. In 2010, 67% of deaths worldwide were due to chronic diseases and this increased to 74% in 2019, with accelerated growth in the COVID-19 era and its aftermath. Aging and wellbeing across the lifespan are positively impacted by the presence of effective prevention and management of chronic illness that can enhance population health. This paper provides a short overview of the journey to this current situation followed by discussion of how we may better address what the World Health Organization has termed the “tsunami of chronic diseases.” In this paper we advocate for the development, validation, and subsequent deployment of integrated: 1. Polygenic and multifactorial risk prediction tools to screen for those at future risk of chronic disease and those with undiagnosed chronic disease. 2. Advanced preventive, behavior change and chronic disease management to maximize population health and wellbeing. 3. Digital health systems to support greater efficiencies in population-scale health prevention and intervention programs. It is argued that each of these actions individually has an emerging evidence base. However, there has been limited research to date concerning the combined population-level health effects of their integration. We outline the conceptual framework within which we are planning and currently conducting studies to investigate the effects of their integration.
The world is slowly emerging from one of its most challenging periods in modern human history with the COVID-19 pandemic and its aftermath. Before the COVID pandemic the focus was on Chronic/Non-Communicable Diseases (NCDs). The WHO President (1) then warned about the growth in chronic disease burden. The significance of NCDs was recognized in the United Nations 2030 Agenda for Sustainable Development, which set targets to, “reduce by one third premature mortality from noncommunicable diseases through prevention and treatment, and promote mental health and well-being” (2). Unfortunately, it is now clear that COVID-19 has contributed to major upticks in underlying and consequential chronic illnesses and diseases from an already high base (3). We now seem to be in an even worse position than before. We have a syndemic driven by the existing chronic disease pandemic overlaid by the newer COVID-19 pandemic (4). A key challenge in the syndemic is to provide adequately resourced and well-trained public and clinical health workforces (5–7).
The burden of chronic illness globally has increased over time, accounting for the major part of global disease burden (8, 9). The epidemiology of chronic disease burden varies from country to country but most countries whether developed or developing have high chronic disease burden. The Lancet global burden studies chronicle the burden of 369 diseases and injuries in 204 countries and territories. They show that chronic conditions caused 74% of all deaths worldwide in 2019, rising from 67% of deaths in 2010. The mortality data reflect high prevalence’s of chronic conditions across populations. For example, in Australia, the Australian Institute of Health and Welfare (10), has noted 47% of Australians have at least one chronic disease with 20% having 2 or more. 51% of hospitalizations involve chronic disease, 90% of deaths and disease burden is borne disproportionately by adults of lower SES and those living in remote areas. These results are consistent with those globally and in many countries. The USA NIH (11) has noted “currently, some 50% of the US population has a chronic disease, creating an epidemic, and 86% of healthcare costs are attributable to chronic disease.” In the United Kingdom a similar epidemiological pattern is evident with close links between traditional physical chronic diseases and associated mental health disorders (12). These studies show increased prevalence across the lifespan threatening healthy aging.
Mental health disorders including addictions are now an increasing challenge facing humanity (13, 14) with 20 percent of global disease burden. One might be forgiven for labeling recent decades as the “Age of Addiction” (15) with the addiction “traditionals” of alcohol, cannabis, and other substances and new synthesized agents overlaid by newly recognized behavioral addictions (16) (see the WHO ICD (17) and AMA DSM) (18). Of the behavioral addictions, the first was Gambling Disorder (GD) – which continues to be neglected in terms of research and development of new interventions. GD is linked to high levels of mental and physical health comorbidities, health economic costs, homelessness, and suicidality (19, 20). In addition to addictions, there is strong growth in the population prevalence’s of mood disorders and anxiety (21). Interpreting the true growth in mental health disorders has some nuances. Recognition of the importance of mental health disorders (12, 22) has also led to increased prominence in national health surveys and public health epidemiological studies. These changes in population health study content make it difficult to assess the true extent of the underlying growth in the population prevalence of such disorders. Another problem is that the most pressing mental health conditions are seldom appropriately measured in public health epidemiological studies – especially GD. These issues are examples of “what gets measured gets managed” and contrariwise. There are many instances where inclusion of measures has improved health services and policy making (23). For example, mental health measures are now an increasingly prominent component of clinical datasets and such conditions are receiving greater clinical effort and funding. The same is true for chronic diseases in general which are now centrally located in health policy and service design in many countries.
The evidence base provided by disease burden and epidemiological studies strongly reinforces the WHO’s alarm. What therefore is to be done? How can the global chronic diseases/long-term conditions pandemic be better addressed? Below we recommend an integrated population-level approach involving (1) large-scale measurement of polygenic and multifactorial risk factors in order to develop and rigorously validate clinically useful prediction tools and algorithms; (2) early and sustained, effective management of chronic diseases using advanced behavior change interventions and (3) digital health approaches to improve the efficiency and reach of interventions and health services at a wider population level.
Early identification of people at risk of chronic illness and early intervention are key to reducing population chronic disease burden. Unfortunately, this obvious game changer is infrequently implemented in many public health regimens. We now have much better technology available to develop and validate evidence-based risk prediction tools and algorithms, and to demonstrate their value by leveraging digital tools that can be embedded within at-scale screening and treatment programs. We already have the technology to develop and validate useful risk prediction tools and algorithms but we contend that we are not yet systematically conducting such research and implementing resulting tools at the required pace in large-scale studies as outlined in the recent International Common Disease Alliance Polygenic Risk Score Task Force report (24). The first contact with many people with chronic disease risk is after they have already developed it (25, 26). For mental health disorders, delays in presentation and intervention can be particularly long, adding to the burden of disease. Obsessive-compulsive disorder (OCD) is one of the top ten leading causes of disability in the developed world and has a typical duration of untreated illness of 10 years (27). While less well studied, a similar duration of untreated illness has been reported for gambling disorder – around 9 years in affected individuals presenting for treatment (28).
Polygenic and multifactorial risk prediction can play a major role in delivering early warning of impending chronic diseases (29) including traditional chronic diseases such as cardiovascular disorders (30), metabolic disorders (31), and cancers (32). While more research is certainly needed, initial data suggest some promise for mental health disorders (33) including gambling disorder and newer concepts such as gaming disorder (34, 35). Wider implementation of such strategies has the potential to drive down the costs of what are now mature and proven technologies, but already they are affordable. The costs associated with inaction with chronic diseases are substantial (36). The potential cost reductions in health care costs and the net benefits of prevention, early detection and intervention are well established in principle. It is our view that humanity cannot afford to further delay polygenic and multifactorial risk prediction, early diagnosis and intervention.
Beyond the need for data collection and linked rigorous validation, to evaluate the potential value of incorporating polygenic and multifactorial risk prediction into routine practice, the global public health and health care workforces need to be trained to use these tools, to effectively communicate the meaning of risk and risk management to the community. Such training is relatively common for some conditions but neglected in other conditions. In the United Kingdom, there has been recent work to promote the wider use of genomics in General Practice (37, 38).
Lifestyle risk factors make a major contribution to the chronic disease burden over the life course. Many of the same risk factors contribute to multiple chronic diseases. Given the high contribution of these behavioral risk factors to multiple chronic diseases, and, that these diseases comprise such a high proportion of total disease burden, it is obvious that public health and clinical workforces need strong chronic disease program prevention skills. Inclusion of such matters in the medical and clinical health curriculums is an essential and welcome innovation to contemporary chronic disease management (39–41).
It is important to understand that treatment for the various chronic diseases must follow recognized evidence based clinical and population health prevention guidelines. Study of these guidelines show that there is a high degree of commonality in the risk factors that contribute to chronic diseases. Advanced behavior changes skills facilitate more effective prevention and management of chronic disease.
Some of this work on expanding training across health systems has been conducted by members of the authorship team. The training has been directed at public health practitioners and clinical workers in public health programs. The Happy Life Club originated in Australia and was then translated to China in various major cities and provinces where it has grown strongly (42–44). Initial economic evaluation (45) showed that incremental benefit for each patient corresponded to $AUD 16,000 over an 18-month period. The 2020 frontiers special issue devoted to chronic disease and aging (46, 47) built on other work concerning Chronic Disease Management (CDM) programs (48). The Club program trains public health practitioners and clinicians to prevent and manage chronic conditions using Motivational Interviewing (MI) principles (49).
The Happy Life Club coach training program has been (50–52) studied as the subject of evaluations and the program was the subject of a large Randomized Controlled Trial (53) and is a World Bank recommended intervention (54). While MI is a central part of the program, rigorous outcome measurement using validated tools and patient-centered care principles are also key components. These techniques are more broadly applicable across a range of chronic diseases including mental health and addiction disorders (55–57) in controlled trials. It would seem sensible to conduct clinician and public health training so that MI techniques can be more widely applied in public health settings with the aim of further reducing the impact and burden of chronic mental health symptoms.
Digital health platforms hold the potential to facilitate Chronic Disease Management across the lifespan at scale in health systems. A large-scale review of digital health platforms was conducted by WHO and the Cochrane Collaboration to develop the Digital Interventions for Health System Strengthening guideline (58). Eleven new Cochrane reviews were included in the guideline. The guideline highlighted different applications of digital health including conventional public health programs, prevention, clinical delivery and back-office programs. It also highlighted the need for the collection of more evidence for these platforms. A 2022 European review (59) investigating the cost-effectiveness of digital health interventions concluded that the evidence was not yet sufficient to return a positive or negative conclusion. We are committed to providing effective digital health support to public health and clinical workers for chronic disease prevention and intervention programs. The goal is to address the knowledge as to how such tools may be best applied (60–63).
Of course, as noted, it is important to be mindful that specific tools are needed for specific purposes and generalizing across all tools is of limited value. Examples of recent digital tool development work in the field of alcohol use include a tool that can estimate weekly alcohol intake based on responses to the extended AUDIT questionnaire; and a web-app brief intervention to raise awareness about the impact of alcohol on breast cancer in a breast cancer clinic setting (potentially modifiable risk factors account for around 25% of breast cancer cases (64, 65)). Another example is that in work led by UK members of our group, we have developed and are piloting a digital tool for NHS gambling treatment services, which collects validated assessment and outcome data from affected individuals and generates readily interpretable summary reports, which are then discussed by the clinician and their patient. This approach could have potential advantages in terms of streamlining the clinical assessment process, fostering early and sustained patient engagement, and improving quality and volume of research data to improve care pathways.
Overall, digital tools are at different levels of development and only some could be deemed sufficiently validated for current widespread use. However, we feel these examples highlight the potential utility of such tools for public health prevention and interventions, in the management and mitigation of chronic diseases. Smart technologies are now available with much-improved access. Recent data shows that access to the Internet of Things will grow from 15 billion to 30 billion devices in the next 7 years (66). Eight billion of these devices will be smartphone connections (67). It is well understood that the availability of smart devices has been less among economically disadvantaged groups. However, this is not cause to deny the obvious advantages of using smart device technologies in global prevention and management of chronic disease of the majority of people.
As previously stated, there is significant evidence for the efficacy of polygenic and multifactorial risk screening, strong evidence for the efficacy of advanced behavior change principles and a developing evidence base for the efficacy of digital health platforms for some conditions and populations (68–70). Rigorous evaluation of the impact of these approaches in combination and their efficacy across varied populations and chronic diseases and disorders is required. The systematic reviews that have been done of the cost-effectiveness of programs to address chronic diseases show significant advantages (71) but many studies do not include adequate economic analysis or active comparator/control conditions.
The conduct of health economics modeling is a key plank of the valuation of chronic disease management programs. Using tools such as the EuroQoL suite and the use of look up tables developed from major studies credible Disability Adjusted Life Years (DALY) and Quality Adjusted Life Years (QALY) estimations can be constructed from the data (72).
There is the technical issue of what methods of economic evaluation ought to be used to assess the costs and benefits of the study outcomes based on the collected data (73). Many studies use an ICER (incremental cost-effectiveness ratio) or an INMB (incremental net monetary benefit) of the intervention compared with usual programs. Both ICER and INMB methods have limitations, but ICER is currently more widely used. INMB has some appealing aspects. A key one is that it is couched in terms of dollar values whereby costs and benefits are expressed in the same dollar value units. This provides not only ease of interpretation but also the ability to use it to enable direct comparison across different programs. All studies in the program will conform to the design principles outlined in the Bias in Economic Evaluation (ECOBIAS) standards (74) and Consolidated Health Economic Evaluating Reporting Standards (CHEERS) (75) to guide the reporting of study outcomes and rigorous design.
The following chart outlines the logic of the INTEGRATE program approach that aims to enhance improved health outcomes across the lifespan through, prevention, earlier detection and more effective intervention. It is intended that the INTEGRATE model will be applied to a range of chronic physical and mental health conditions at a population level, reflecting the high degree of multi-morbidity identified in population and clinical studies. The INTEGRATE model does not replace guidelines-driven programs and interventions. It provides an organizing framework for important strategic disease risk prediction data based on genomics science and multi-factorial risk assessment, supports public health workers and clinicians using their disciplinary evidence-based prevention/ treatment guidelines to deal with a range of chronic illnesses by enhancing their behavior change skills and assists with the cost-effective delivery of treatment by utilizing advanced digital health platform capabilities. The public health and clinical workers are augmented by powerful support tools (Figure 1).
The INTEGRATE model seeks to combine polygenic genomic and diagnostic testing and history data for target chronic illnesses to identify sub-populations that are low risk, at risk and with diagnosed and undiagnosed conditions. Those with high risk but no diagnosed condition are referred for preventive actions to lower their risk. Those with diagnosed conditions are referred into treatment programs to improve health and wellbeing. All cases in the preventive and treatment programs may be tracked to assess their ongoing health status and wellbeing. These program actions are powered by behavior change science and prevention and treatment guidelines pertinent to their chronic illnesses assisted by digital platform technologies. It is intended that cases and at-risk community members will be detected and enter earlier preventive and treatment programs. Health economics, participant experience and health outcomes studies will be used to evaluate program effectiveness and efficacy. It is intended that health care costs will be lowered, risk reduced, and outcomes improved by the application of this model through its integration into public health and clinical programs targeting chronic diseases.
We have now set ourselves the task of evaluating the efficiency and effectiveness of the INTEGRATE approach we have described for integrated prevention and effective interventions across a range of chronic diseases. We believe that this approach addresses several key problems. Although there have been many exhortations of the virtues of prevention and early invention among at-risk populations, the genomic technologies that practically and expediently this approach are recent, but in some cases are now sufficiently developed to trial and use at scale. However, we must take an evidence-based and skeptical approach using health economics and rigorous clinical efficacy evidence including appropriate control conditions (where feasible). Behavior change science has been with us for several decades, but its power has not been fully implemented due to lack of contemporary training throughout the public health and clinical workforces. This science does not replace, for example, pharmacological and other interventions, it complements and augments them.
We feel these promising technologies are within our grasp and now we have the duty of evaluating and implementing them in an effective integrated way to advantage the targeted populations within our communities. This integrated approach has considerable promise for promoting population health, healthy aging and reducing the current burdens of health care. We also have a commitment to not artificially separate “physical” and “mental” health conditions in a context where they are so demonstrably interdependent.
The original contributions presented in the study are included in the article/supplementary material, further inquiries can be directed to the corresponding author.
ST and CB conceptualized the paper. ST drafted the paper and had final editorial approval. CB assisted with the drafting of the paper and provided editorial input. FC contributed to the genomic sections of the paper. BK contributed to the digital health sections of the paper. MO provided a public health perspective to the drafting of the paper. HB-J and SC assisted with drafting of the mental health sections of the paper. All authors provided editorial comments in addition to their substantive contributions.
The authors thank Liam Thomas for his contribution as a Research Officer to this work, assisting with literature searches and summaries, reference management and document handling.
SRC’s research is funded by the NHS and was previously funded by Wellcome (an independent charity). SRC receives a stipend for editorial work at Elsevier journals (Comprehensive Psychiatry, and Neuroscience & Biobehavioral Reviews). HB-J is the Director of the UK National Problem Gambling Clinic and the UK National Centre for Gaming Disorders which are now fully funded by the NHS. These clinics have previously received funding from NHS England, Central and North West London NHS Trust, and GambleAware. HB-J is Vice President of the Royal Society of Medicine and sits on several national and international Boards. HB-J has been on research teams funded by the Medical Research Council, the Wellcome Trust, and the Wolfson Family Trust.
The remaining authors declare that the research was conducted in the absence of any commercial or financial relationships that could be construed as a potential conflict of interest.
All claims expressed in this article are solely those of the authors and do not necessarily represent those of their affiliated organizations, or those of the publisher, the editors and the reviewers. Any product that may be evaluated in this article, or claim that may be made by its manufacturer, is not guaranteed or endorsed by the publisher.
1. World Health Organization. Ten years in public health, 2007–2017: Report by Dr Margaret Chan, director-general. Geneva: World Health Organization (2017, 2017) Licence: CC BY-NC-SA 3.0 IGO.
2. United Nations. The sustainable development goals report (2022). Available at: https://unstats.un.org/sdgs/report/2022/The-Sustainable-Development-Goals-Report-2022.pdf
4. McGowan, VJ, and Bambra, C. COVID-19 mortality and deprivation: pandemic, syndemic, and endemic health inequalities. Lancet Public Health. (2022) 7:e966–75. doi: 10.1016/S2468-2667(22)00223-7
5. Gibbs, T, and Sabine, N. Chronic disease management and the healthcare workforce. Dela J Public Health. (2022) 8:176–86. doi: 10.32481/djph.2022.12.043
6. Sun, W, Li, Y, Hu, Y, Rao, X, Xu, X, Browning, CJ, et al. Perspectives on the training of Chinese primary health care physicians to reduce chronic diseases and their burden. Front Public Health. (2019) 7:168. doi: 10.3389/fpubh.2019.00168
7. Rao, X, Lai, J, Wu, H, Li, Y, Xu, X, Browning, CJ, et al. The development of a competency assessment standard for general practitioners in China. Front Public Health. (2020) 8:23. doi: 10.3389/fpubh.2020.00023
8. Hajat, C, and Stein, E. The global burden of multiple chronic conditions: a narrative review. Prev Med Rep. (2018) 12:284–93. doi: 10.1016/j.pmedr.2018.10.008
9. GBD 2019 Diseases and Injuries Collaborators. Global burden of 369 diseases and injuries in 204 countries and territories, 1990-2019: a systematic analysis for the global burden of disease study 2019. Lancet (London, England). (2020) 396:1204–22. doi: 10.1016/S0140-6736(20)30925-9
10. Australian Institute of Health and Welfare (2022) Chronic condition multimorbidity, AIHW, Australian government, revised 29/09/ 2022.
11. Holman, HR. The relation of the chronic disease epidemic to the health care crisis. ACR Open Rheumatol. (2020) 2:167–73. doi: 10.1002/acr2.11114
12. Launders, N, Kirsh, L, Osborn, DPJ, and Hayes, JF. The temporal relationship between severe mental illness diagnosis and chronic physical comorbidity: a UK primary care cohort study of disease burden over 10 years. Lancet Psychiatry. (2022) 9:725–35. doi: 10.1016/S2215-0366(22)00225-5
13. Hodgins, DC, Stea, JN, and Grant, JE. Gambling disorders. Lancet. (2011) 378:1874–84. doi: 10.1016/S0140-6736(10)62185-X
14. Roberts, A, Sharman, S, Coid, J, Murphy, R, Bowden-Jones, H, Cowlishaw, S, et al. Gambling and negative life events in a nationally representative sample of UK men. Addict Behav. (2017) 75:95–102. doi: 10.1016/j.addbeh.2017.07.002
15. GBD. 2016 alcohol and drug use collaborators. The global burden of disease attributable to alcohol and drug use in 195 countries and territories, 1990-2016: a systematic analysis for the global burden of disease study 2016. Lancet Psychiatry. (2018) 5:987–102. doi: 10.1016/S2215-0366(18)30337-7
16. Bowden-Jones, H, Hook, RW, Grant, JE, Ioannidis, K, Corazza, O, Fineberg, NA, et al. Gambling disorder in the UK: key research priorities and the urgent need for independent research funding. Lancet Psychiatry. (2022) 9:321–39. doi: 10.1016/S2215-0366(21)00356-4
17. World Health Organization. (2019a). International statistical classification of diseases and related health problems (11th ed.). Available at: https://icd.who.int/
18. American Psychiatric Association. Diagnostic and statistical manual of mental disorders. 5th ed. Virginia: American Psychiatric Association. (2013).
19. Dowling, NA, Cowlishaw, S, Jackson, AC, Merkouris, SS, Francis, KL, and Christensen, DR. Prevalence of psychiatric co-morbidity in treatment-seeking problem gamblers: a systematic review and meta-analysis. Aust N Z J Psychiatry. (2015) 49:519–29. doi: 10.1177/0004867415575774
20. Vandenberg, B, Livingstone, C, Carter, A, and O'Brien, K. Gambling and homelessness: a systematic review and meta-analysis of prevalence. Addict Behav. (2022) 125:107151. doi: 10.1016/j.addbeh.2021.107151
21. World mental health report: Transforming mental health for all. Geneva: World Health Organization (2022) Licence: CC BY-NC-SA 3.0 IGO.
22. GBD. 2019 mental disorders collaborators. Global, regional, and national burden of 12 mental disorders in 204 countries and territories, 1990-2019: a systematic analysis for the global burden of disease study 2019. Lancet Psychiatry. (2022) 9:137. doi: 10.1016/S2215-0366(21)00395-3
23. Connors, EH, Douglas, S, Jensen-Doss, A, Landes, SJ, Lewis, CC, McLeod, BD, et al. What gets measured gets done: how mental health agencies can leverage measurement-based care for better patient care, clinician supports, and organizational goals. Adm Policy Ment Health Ment Health Serv Res. (2021) 48:250–65. doi: 10.1007/s10488-020-01063-w
24. Polygenic risk score task force of the international common disease alliance. Responsible use of polygenic risk scores in the clinic: potential benefits, risks and gaps. Nat Med. (2021) 27:1876–84. doi: 10.1038/s41591-021-01549-6
25. Harris, MI, Klein, R, Welborn, TA, and Knuiman, MW. Onset of NIDDM occurs at least 4-7 yr before clinical diagnosis. Diabetes Care. (1992) 15:815–29. doi: 10.2337/diacare.15.7.815
26. Khou, V, Anderson, JJ, Strange, G, Corrigan, C, Collins, N, Celermajer, DS, et al. Diagnostic delay in pulmonary arterial hypertension: insights from the Australian and New Zealand pulmonary hypertension registry. Respirology. (2020) 25:863–71. doi: 10.1111/resp.13768
27. Fineberg, NA, Dell'Osso, B, Albert, U, Maina, G, Geller, D, Carmi, L, et al. Early intervention for obsessive compulsive disorder: an expert consensus statement. Eur Neuropsychopharmacol. (2019) 29:549–55. doi: 10.1016/j.euroneuro.2019.02.002
28. Grant, JE, and Chamberlain, SR. Duration of untreated illness in gambling disorder. CNS Spectr. (2023):1–21. doi: 10.1017/S1092852923002444
29. Lewis, CM, and Vassos, E. Polygenic risk scores: from research tools to clinical instruments. Genome Med. (2020) 12:44. doi: 10.1186/s13073-020-00742-5
30. Abraham, G, Rutten-Jacobs, L, and Inouye, M. Risk prediction using polygenic risk scores for prevention of stroke and other cardiovascular diseases. Stroke. (2021) 52:2983–91. doi: 10.1161/STROKEAHA.120.032619
31. Hahn, SJ, Kim, S, Choi, YS, Lee, J, and Kang, J. Prediction of type 2 diabetes using genome-wide polygenic risk score and metabolic profiles: a machine learning analysis of population-based 10-year prospective cohort study. EBioMedicine. (2022) 86:104383. doi: 10.1016/j.ebiom.2022.104383
32. Huntley, C, Torr, B, Sud, A, Rowlands, CF, Way, R, Snape, K, et al. Utility of polygenic risk scores in UK cancer screening: a modelling analysis. Lancet Oncol. (2023) 24:658–8. doi: 10.1016/S1470-2045(23)00156-0
33. Meerman, JJ, Ter Hark, SE, Janzing, JGE, and Coenen, MJH. The potential of polygenic risk scores to predict antidepressant treatment response in major depression: a systematic review. J Affect Disord. (2022) 304:1–11. doi: 10.1016/j.jad.2022.02.015
34. Warrier, V, Chamberlain, SR, Thomas, SA, and Bowden-Jones, H. Genetics of gambling disorder and related phenotypes: The potential uses of polygenic and multifactorial risk models to enable early detection and improve clinical outcomes. J. Behav. Addict. (2023) (in press).
35. Piasecki, TM, Gizer, IR, and Slutske, WS. Polygenic risk scores for psychiatric disorders reveal novel clues about the genetics of disordered gambling. Twin Res Hum Genet. (2019) 22:283–99. doi: 10.1017/thg.2019.90
36. National Center for Chronic Disease Prevention and Health Promotion (NCCDPHP) health and economic costs of chronic diseases. Available at: https://www.cdc.gov/chronicdisease/about/costs/index.htm (Accessed 28 April, 2023).
37. Hillman, SC, and Dale, J. Genomics in general practice: generation genome. Br J Gen Pract. (2017) 67:540–51. doi: 10.3399/bjgp17X693533
38. Davies, SC. Annual report of the chief medical officer 2016: Generation genome. London: Department of Health (2017).
39. Reis, C, Dias, S, Rodrigues, AM, Sousa, RD, Gregório, MJ, Branco, J, et al. Sleep duration, lifestyles and chronic diseases: a cross-sectional population-based study. Sleep Sci. (2018) 11:217–20. doi: 10.5935/1984-0063.20180036
40. Ren, Y, Yang, H, Browning, C, Thomas, S, and Liu, M. Therapeutic effects of motivational interviewing on blood pressure control: a meta-analysis of randomized controlled trials. Int J Cardiol. (2014) 172:509–11. doi: 10.1016/j.ijcard.2014.01.051
41. O'Halloran, PD, Blackstock, F, Shields, N, Holland, A, Iles, R, Kingsley, M, et al. Motivational interviewing to increase physical activity in people with chronic health conditions: a systematic review and meta-analysis. Clin Rehabil. (2014) 28:1159–71. doi: 10.1177/0269215514536210
42. Browning, CJ, Chapman, A, Cowlishaw, S, Li, Z, Thomas, SA, Yang, H, et al. The happy life Club™ study protocol: a cluster randomised controlled trial of a type 2 diabetes health coach intervention. BMC Public Health. (2011) 11:90. doi: 10.1186/1471-2458-11-90
43. Browning, CJ, Yang, H, Zhang, T, Chapman, A, Liu, S, Enticott, JC, et al. Implementing a chronic disease self-management program into China: the happy life club™. Front Public Health. (2015) 2:181. doi: 10.3389/fpubh.2014.00181
44. Browning, CJ, Chapman, A, Yang, H, Liu, S, Zhang, T, Enticott, JC, et al. Management of type 2 diabetes in China: the happy life Club, a pragmatic cluster randomised controlled trial using health coaches. BMJ Open. (2016) 6:e009319. doi: 10.1136/bmjopen-2015-009319
45. Mortimer, DS, and Kelly, J. Economic evaluation of the good life Club intervention for diabetes self-management. Aust J Prim Health. (2006) 12:91–100. doi: 10.1071/PY06013
46. Thomas, SA, Qiu, Z, Chapman, A, Liu, S, and Browning, CJ. Editorial: chronic illness and ageing in China. Front Public Health. (2020) 8:104. doi: 10.3389/fpubh.2020.00104
47. Thomas, SA, Browning, CJ, Qiu, Z, Chapman, A, and Liu, S, Chronic illness and ageing in China. Frontiers research Topics, Zurich: Switzerland ISBN 978–2–88963-754-6.
48. Browning, CJ, and Thomas, SA. Implementing chronic disease self-management approaches in Australia and the United Kingdom. Front Public Health. (2015) 2:162. doi: 10.3389/fpubh.2014.00162
49. Browning, CJ, and Thomas, SA. Behavioural change: an evidence-based handbook for social and public health. London: Churchill Livingstone (2005) isbn:ISBN 0443073570.
50. Liu, S, Yang, H, Browning, CJ, Thomas, SA, and Zhang, T. The influence of family responsibility on the self- management behavior of patients with type 2 diabetes mellitus - the happy life Club™. Chin Gen Pract. (2015) 2:1477–82. doi: 10.3969/j.issn.1007-9572.2015.13.001
51. Liu, S, Yang, H, Browning, CJ, Thomas, SA, and Zhang, T. Process analysis of motivational interviewing intervention by primary health care setting staff in China - the happy life Club™. Chin Gen Pract. (2015) 18:2241–8. doi: 10.3969/j.issn.1007−9572.2015.19.001
52. Liu, S, Yang, H, Browning, CJ, Thomas, SA, and Zhang, T. The influence of social connectedness under traditional Chinese culture on the self- management behavior of patients with type 2 diabetes mellitus - the happy life Club™. Chin Gen Pract. (2015) 18:1878–83. doi: 10.3969/j.issn.10079572.2015.16.004
53. Chapman, A, Liu, S, Merkouris, S, Enticott, JC, Yang, Y, Browning, CJ, et al. Psychological interventions for the Management of Glycemic and Psychological Outcomes of type 2 diabetes mellitus in China: a systematic review and Meta-analyses of randomized controlled trials. Front Public Health. (2015) 3:252. doi: 10.3389/fpubh.2015.00252
54. World Bank Health Nutrition and Population Global Practice. Compendium of innovative models of Care for non-Communicable Diseases. Washington: The World Bank Group (2021).
55. Tan, CJ, Shufelt, T, Behan, E, Chantara, J, Koomsri, C, Gordon, AJ, et al. Comparative effectiveness of psychosocial interventions in adults with harmful use of alcohol: a systematic review and network meta-analysis. Addiction. (2023) 118:1414–29. doi: 10.1111/add.16187
56. Yakovenko, I, Quigley, L, Hemmelgarn, BR, Hodgins, DC, and Ronksley, P. The efficacy of motivational interviewing for disordered gambling: systematic review and meta-analysis. Addict Behav. (2015) 43:72–82. doi: 10.1016/j.addbeh.2014.12.011
57. Grant, JE, Donahue, CB, Odlaug, BL, Kim, SW, Miller, MJ, and Petry, NM. Imaginal desensitisation plus motivational interviewing for pathological gambling: randomised controlled trial. Br J Psychiatry. (2009) 195:266–77. doi: 10.1192/bjp.bp.108.062414
58. World Health Organization. WHO guideline: Recommendations on digital interventions for health system strengthening. Geneva: World Health Organization (2019a) Licence: CC BY-NC-SA 3.0 IGO.
59. Gentili, A, Failla, G, Melnyk, A, Puleo, V, Tanna, GLD, Ricciardi, W, et al. The cost-effectiveness of digital health interventions: a systematic review of the literature. Front Public Health. (2022) 10:787135. doi: 10.3389/fpubh.2022.787135
60. Siopis, G, Moschonis, G, Eweka, E, Jung, J, Kwasnicka, D, Asare, BY, et al. Effectiveness, reach, uptake, and feasibility of digital health interventions for adults with hypertension: a systematic review and meta-analysis of randomised controlled trials. Lancet digit. Health. (2023) 5:e144–59. doi: 10.1016/S2589-7500(23)00002-X
61. Gega, L, Jankovic, D, Saramago, P, Marshall, D, Dawson, S, Brabyn, S, et al. Digital interventions in mental health: evidence syntheses and economic modelling. Health Technol Assess. (2022) 26:1–82. doi: 10.3310/RCTI6942
62. Barnett, A, Wright, C, Stone, C, Ho, NY, Adhyaru, P, Kostjasyn, S, et al. Effectiveness of dietary interventions delivered by digital health to adults with chronic conditions: systematic review and meta-analysis. J Hum Nutr Diet. (2022) 36:632–46. doi: 10.1111/jhn.13125
63. Kim, J, Aryee, LMD, Bang, H, Prajogo, S, Choi, YK, Hoch, JS, et al. Effectiveness of digital mental health tools to reduce depressive and anxiety symptoms in low- and middle-income countries: systematic review and Meta-analysis. JMIR Ment Health. (2023) 10:e43066. doi: 10.2196/43066
64. Dutey-Magni, P, Brown, J, Holmes, J, and Sinclair, J. Concurrent validity of an estimator of weekly alcohol consumption (EWAC) based on the extended AUDIT. Addiction. (2022) 117:580–9. doi: 10.1111/add.15662
65. Sinclair, JMA, Dutey-Magni, PF, Anderson, AS, Baird, J, Barker, ME, Cutress, RI, et al. A context-specific digital alcohol brief intervention in symptomatic breast clinics (abreast of health): development and usability study. JMIR Res Protoc. (2020) 9:e14580. doi: 10.2196/14580
66. Statista Search Department (2023). Number of internet of things (IoT) connected devices worldwide from 2019 to 2021, with forecasts from 2022 to 2030. Statista. Available at: https://www.statista.com/statistics/1183457/iot-connected-devices-worldwide/
67. Statista Search Department (2023). Number of smartphone mobile network subscriptions worldwide from 2016 to 2022, with forecasts from 2023 to 2028. Statista. Available at: https://www.statista.com/statistics/330695/number-of-smartphone-users-worldwide/
68. Siegel, KR, Ali, MK, Zhou, X, Ng, BP, Jawanda, S, Proia, K, et al. Cost-effectiveness of interventions to manage diabetes: has the evidence changed since 2008? Diabetes Care. (2020) 43:1557–92. doi: 10.2337/dci20-0017
69. Thomas, C, Brennan, A, Goka, E, Squires, HY, Brenner, G, Bagguley, D, et al. What are the cost-savings and health benefits of improving detection and management for six high cardiovascular risk conditions in England? An economic evaluation. BMJ Open. (2020) 10:e037486. doi: 10.1136/bmjopen-2020-037486
70. Muka, T, Imo, D, Jaspers, L, Colpani, V, Chaker, L, van der Lee, SJ, et al. The global impact of non-communicable diseases on healthcare spending and national income: a systematic review. Eur J Epidemiol. (2015) 30:251–77. doi: 10.1007/s10654-014-9984-2
71. Gallagher, A, Shersher, V, Mortimer, D, Truby, H, and Haines, T. The cost-effectiveness of adjunctive lifestyle interventions for the Management of Cancer: a systematic review. Appl Health Econ Health Policy. (2023) 21:225–32. doi: 10.1007/s40258-022-00759-4
72. Thomas, SA, and Browning, CJ. Burden of disease In: N Pachana, editor. Encyclopedia of Geropsychology. Berlin: Springer-Verlag (2016)
73. Yuan, F, Bangdiwala, SI, Tong, W, and Lamy, A. The impact of statistical properties of incremental monetary net benefit and incremental cost-effectiveness ratio on health economic modeling choices. Expert Rev Pharmacoecon Outcomes Res. (2023) 23:69–78. doi: 10.1080/14737167.2023.2144838
74. Adarkwah, CC, van Gils, PF, Hiligsmann, M, and Evers, SM. Risk of bias in model-based economic evaluations: the ECOBIAS checklist. Expert Rev Pharmacoecon Outcomes Res. (2016) 16:513–3. doi: 10.1586/14737167.2015.1103185
75. Husereau, D, Drummond, M, Augustovski, F, de Bekker-Grob, E, Briggs, AH, Carswell, C, et al. Correction to: consolidated health economic evaluation reporting standards 2022 (CHEERS 2022) statement: updated reporting guidance for health economic evaluations. Appl Health Econ Health Policy. (2022) 20:781–2. doi: 10.1007/s40258-022-00743-y
Keywords: chronic diseases, prevention, genomics, risk prediction, behavior change, digital health, healthy aging
Citation: Thomas SA, Browning CJ, Charchar FJ, Klein B, Ory MG, Bowden-Jones H and Chamberlain SR (2023) Transforming global approaches to chronic disease prevention and management across the lifespan: integrating genomics, behavior change, and digital health solutions. Front. Public Health. 11:1248254. doi: 10.3389/fpubh.2023.1248254
Received: 26 June 2023; Accepted: 18 September 2023;
Published: 13 October 2023.
Edited by:
Kathleen Potempa, University of Michigan, United StatesReviewed by:
Rajvi Jayant Wani, Amgen (Canada), CanadaCopyright © 2023 Thomas, Browning, Charchar, Klein, Ory, Bowden-Jones and Chamberlain. This is an open-access article distributed under the terms of the Creative Commons Attribution License (CC BY). The use, distribution or reproduction in other forums is permitted, provided the original author(s) and the copyright owner(s) are credited and that the original publication in this journal is cited, in accordance with accepted academic practice. No use, distribution or reproduction is permitted which does not comply with these terms.
*Correspondence: Colette J Browning, Yy5icm93bmluZ0BmZWRlcmF0aW9uLmVkdS5hdQ==
Disclaimer: All claims expressed in this article are solely those of the authors and do not necessarily represent those of their affiliated organizations, or those of the publisher, the editors and the reviewers. Any product that may be evaluated in this article or claim that may be made by its manufacturer is not guaranteed or endorsed by the publisher.
Research integrity at Frontiers
Learn more about the work of our research integrity team to safeguard the quality of each article we publish.