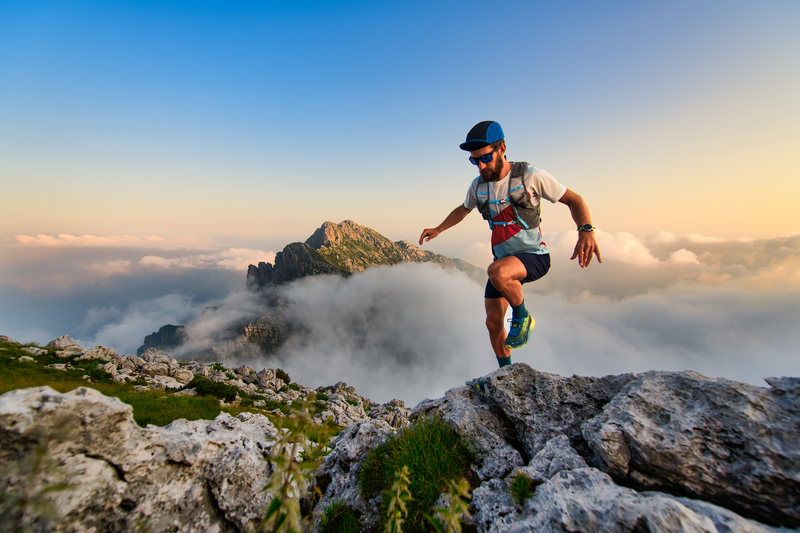
95% of researchers rate our articles as excellent or good
Learn more about the work of our research integrity team to safeguard the quality of each article we publish.
Find out more
TECHNOLOGY AND CODE article
Front. Public Health , 29 June 2023
Sec. Infectious Diseases: Epidemiology and Prevention
Volume 11 - 2023 | https://doi.org/10.3389/fpubh.2023.1215574
Recurrent outbreaks of zoonotic infectious diseases highlight the importance of considering the interconnections between human, animal, and environmental health in disease prevention and control. This has given rise to the concept of One Health, which recognizes the interconnectedness of between human and animal health within their ecosystems. As a contribution to the One Health approach, this study aims to develop an indicator system to model the facilitation of the spread of zoonotic diseases. Initially, a literature review was conducted using the Preferred Reporting Items for Systematic Reviews and Meta-Analyses (PRISMA) statement to identify relevant indicators related to One Health. The selected indicators focused on demographics, socioeconomic aspects, interactions between animal and human populations and water bodies, as well as environmental conditions related to air quality and climate. These indicators were characterized using values obtained from the literature or calculated through distance analysis, geoprocessing tasks, and other methods. Subsequently, Multi-Criteria Decision-Making (MCDM) techniques, specifically the Entropy and Technique for Order of Preference by Similarity to Ideal Solution (TOPSIS) methods, were utilized to combine the indicators and create a composite metric for assessing the spread of zoonotic diseases. The final indicators selected were then tested against recorded zoonoses in the Valencian Community (Spain) for 2021, and a strong positive correlation was identified. Therefore, the proposed indicator system can be valuable in guiding the development of planning strategies that align with the One Health principles. Based on the results achieved, such strategies may prioritize the preservation of natural landscape features to mitigate habitat encroachment, protect land and water resources, and attenuate extreme atmospheric conditions.
Zoonotic diseases pose a significant public health concern, with over 70% of emerging diseases being transmitted from animals to humans and 60% of human infectious diseases being shared with animals (1). This means that zoonotic diseases have played a role in recent outbreaks, including Ebola and coronavirus pandemics, as well as in well-known foodborne illnesses. These diseases can be transmitted not only through direct contact with animals or vectors, or the ingestion of animal products, but also through the consumption of contaminated vegetables grown in areas where domestic or wild animal manure or irrigation water is used (2).
The transmission of zoonotic diseases is a complex and multifactorial process, making it difficult to manage and predict due to the interconnected elements involved. Therefore, an interdisciplinary approach is necessary; focusing not only on disease surveillance but also on the development of predictive models (3).
The concept of One Health is crucial for today’s sustainable human development. One Health is “an approach that recognizes people’s health, closely connected to the health of animals and our shared environment” (4). The recurrence of outbreaks of emerging and re-emerging zoonotic diseases has emphasized the importance of the One Health approach, which acknowledges the interconnectedness of human, animal, and environmental health (5, 6). Achieving One Health requires a comprehensive understanding of the complex interactions and feedback between these systems and the identification of interventions that can promote positive outcomes for all (7). However, measuring progress toward One Health goals remains challenging, and various initiatives have employed different indicator systems to address this issue (8–10).
There is an ongoing debate on the most effective way to measure progress toward One Health goals (11–14), but there is a clear relationship between contagious diseases and spatial configurations and conditions (15, 16). The exponential growth of human population has disrupted the interface between humans, animals, and the environment through increased urbanization and the expansion of livestock and agricultural areas (17). These processes lead to the fragmentation of wildlife habitats that increase interspecies friction and the spread of pathogens. For example, it has been argued that certain infectious diseases are exacerbated by factors such as rapid urbanization, large migrant workers populations, climate change, ecological changes, and policies like deforestation (18).
Spatial assessment of disease susceptibility or transmissibility is crucial for the One Health approach as it helps identify areas where zoonotic diseases are more likely to occur and spread. Understanding the relationship between different spatial configurations and the spread of pathogens is essential to reduce the transmission of infectious diseases (17, 19, 20). However, it is a complex and challenging subject.
The number of publications examining the relationship between spatial configuration and the spread of infectious diseases increased from 100 in 2000 to over 700 publications in 2017 (20). This highlights the growing interest in this field and the motivation behind conducting a systematic literature review to better understand what had been done previously.
Previous research has addressed this issue from different perspectives. There is a general trend toward interdisciplinary strategies (17), although the focus has often been on program implementation rather than contextual research (21). More specifically, the analysis diverges in multiple directions. The interest in modeling methodologies has been of particular interest, but different approaches have been taken. Some authors have incorporated census data, land use information, and population mobility into their model design (22), while others have examined multiple cases to understand how mathematical models can generate robust evidence and shape effective public health policies at local and global levels (23). Recent research shows a notable trend toward automation and the development of more complex models.
Significant research has attempted to understand how proximity to Green Infrastructure (GI), which refers to a network of natural and semi-natural areas, can help improve human health (24). Other studies have focused on zoonoses related to ecosystems, assessing their impact on human health while examining the existing evidence of ecological responses to global changes (25). Recent studies have emphasized the need for a holistic approach within the concept of One Health to predict and prevent future pandemics (26, 27). Some models have considered different spatial conditions (28), while others have focused on specific species (29). An important percentage analyzed specific outbreaks in detail (e.g. (30–33),). In these cases, the proliferation of Geographic Information Systems (GIS) has contributed to a better understanding of the role of space in pathogen spillover (34, 35). For more details, refer to (36–39).
In general, the study of previous research has provided insights into the strong relationship between the spread of diseases and various spatial conditions. However, we could not find any studies specifically addressing how these spatial conditions could represent susceptibility or weaknesses that contribute to the faster, stronger, or broader spread of zoonotic diseases. Apart from the previously mentioned approaches, some authors have worked on developing indicators to better understand One Health conditions (8, 9, 40), but they used qualitative research based on binary logic, were conducted at the regional/national scale, or were somehow not holistic and complex enough to capture all the conditions prevailing in the area. In other words, to address a given area using the One Health framework, holistic tools to assess its spatial susceptibility need to be developed.
This research helps to fill this knowledge gap by developing a spatial indicator system that models the facilitation of zoonotic diseases spread, providing insights into a region’s contribution to One Health. The proposed indicator system takes a multidimensional approach for One Health, incorporating indicators of human, animal, and environmental health, as well as their interactions. We apply this indicator system to the region of Valencia, Spain, which exhibits diverse ecosystems and land uses including agriculture, urban areas, and natural areas. The novelty of this research lies in the development of a practical tool for measuring a region’s contribution to One Health.
As mentioned earlier, One Health requires collaboration among different sectors and stakeholders (41–43). This case study aims to provide policymakers, researchers, and practitioners with a practical tool for monitoring progress toward One Health goals and identifying areas for intervention and improvement. Furthermore, our study seeks to evaluate the usefulness and validity of the proposed indicator system by comparing its results with infectious disease records in the case study area and assessing the potential role of GI in achieving One Health.
This document is structured as follows: first, we review the literature on One Health and indicator systems. Second, we describe the methodology employed to develop the indicator system and apply it to the region of Valencia, Spain. Third, we present the results of our case study and evaluate the utility and validity of the proposed indicator system. Finally, we discuss the implications of our findings and provide recommendations for future research.
The steps for designing and applying the proposed indicator system are depicted in Figure 1. A systematic literature review was first conducted to gain insights into the breadth of prior research on using indicators for One Health goals. The outcomes of the literature review were used to select a list of indicators that encompassed aspects related to animal, human, and environmental health. GIS and Multi-Criteria Decision-Making (MCDM) methods were employed to characterize, weight, and aggregate the shortlisted indicators. This resulted in a composite index that reflects the contributions of a region’s spatial context to One Health. These index values were then compared with the area covered by GI to assess its impact on One Health.
Figure 1. Components of the indicator system to measure the facilitation of the spread of zoonotic diseases.
The indicators were selected based on the results of a literature review conducted according to the Preferred Reporting Items for Systematic Reviews and Meta-Analyses (PRISMA) statement (44). The literature review aimed to answer the research question: are there any indicators or metrics that have been consistently used to address One Health issues? Finding an answer to this question should result in a set of indicators ranked by their frequency of use in previous research. The inclusion criteria for the review were as follows:
• The publications are original research articles.
• The articles are indexed in the Scopus, Web of Science or PubMed databases.
• The year of publication of the documents is equal to or later than 2004.
• The articles are published in English.
Review articles, conference papers, and books were excluded from the eligible items to focus on original research contributions that utilized indicators to address the One Health initiative. The search included the Scopus, Web of Science, and PubMed databases because of their extensive journal coverage, temporal range, and relevance to medical research, respectively (45). The time frame was limited to 2004 onwards, aligning with the year when the term One Health was coined (46, 47). The documents had to be written in English because of its consideration as the language of science (48).
The search query included the terms “one health,” and either “indicator*” or “metric*” in the title, abstract, or keywords of the documents. Additional specific terms related to different facets of the One Health concept, particularly those with spatial implications, were also incorporated into the search query. Eq. (1) presents the search query used in the Scopus database.
TITLE-ABS-KEY [“one health” AND (“indicator*” OR “metric*”)] AND [“agricultur*” OR “air” OR “biodiversity” OR “climate change*” OR “disease*” OR “ecologic*” OR “ecosystem*” OR “epidemi*” OR “food” OR “forest*” OR “habitat” OR “land use*” OR “livestock” OR “pollution” OR “population” OR “soil” OR “urban*” OR “warming” OR “water” OR “wildlife”] AND LIMIT-TO (DOCTYPE, “ar”)] AND [LIMIT-TO (PUBYEAR, 2022–2004) AND LIMIT-TO (LANGUAGE, “English”]. (1)
The results from the databases were combined to eliminate duplicates. Aside from the fields returned directly from the query (authors, year, title, abstract, and keywords), a few others were added manually to gather more specific information. One of these fields was used to track the reasons for discarding initially eligible articles. This could be for a variety of reasons, including the article being a review that is not labeled as such, misinterpretation of search query terms (e.g., “one’s health” instead of “one health”), or the absence of suggested indicators. Invalid documents could be identified through reading the abstracts or full texts.
Six additional fields were related to the dimensions of One Health. Three fields indicated whether articles emphasized animal, human, or environmental health, while the other three fields recorded the specific terms used for each dimension. Two binary fields were included to indicate whether the articles considered GI and whether they had a spatial component. Another pair of fields compiled the indicators proposed in the papers and derived from reading them.
The screened documents were processed to generate frequency counts from the words contained in some fields, particularly keywords, and indicators, as well as the binary data on the dimensions of One Health and the presence of GI and spatial approaches. After reclassification and standardization, this analysis revealed the main trends found in the articles and created a hierarchy of the most frequently recurring indicators for modeling One Health.
Due to the orientation of the study on the role of spatial planning in the facilitation of zoonotic disease spread, the indicators were designed in a way that allowed them to be characterized using GIS. This involved applying geoprocessing tools to conduct spatial analyses regarding densities, distances, or algebraic operations. The resulting maps were then used to calculate descriptive statistics (mean or sum) per administrative unit through zonal calculations.
The outputs of this characterization process enabled the creation of a matrix of indicators assessed with values across administrative units. This arrangement resembles an MCDM problem, where multiple alternatives are evaluated depending on a set of criteria (49). MCDM problems broadly consist of two steps: weighting of criteria (indicators) and assessment of alternatives (administrative units) across the weighted criteria (50). Given the multiple branches that stem from the concept of One Health, the selection of indicators aimed to capture different aspects of environmental, human, and animal health.
The weighting of indicators was performed using the Entropy Method (EM), which was proposed by Zeleny (51) to objectively calculate the weights of criteria in decision-making processes. The importance of a criterion is assumed to be proportional to the amount of information it provides about the alternatives. The idea is to give more weight to the criterion that can better discriminate the other options, i.e., the criterion that shows greater diversity when evaluating the other options. The higher the entropy ( j), the lower the diversity ( ).
In this study, the EM was employed to determine the weights of indicators based on their differentiation. Indicators that exhibited more distinct values across the administrative units contained more information and had lower entropy (52). This implies large weights for indicators with low entropy values and vice versa. For example, if all administrative units had very similar values (e.g., from 0.5 to 0.6) regarding a particular indicator, that indicator would be given a low weight. Conversely, if another indicator exhibited a wide range of values (e.g., from 0.1 to 0.9) across the administrative units, it would be assigned a higher weight.
To enable a proper comparison of the index dimensions within the decision-making matrix a max-min transformation was applied to normalize the values of the indicators across the administrative units, as shown in Eq. (2). Transformation ensured that the indicator values were on a comparable scale.
where and are the maximum and minimum values among the alternatives for indicator . The entropy of each indicator was determined from the normalized values as formulated in Eq. (3).
where . For the applicability of the method, the entire term is set to if . To give more importance to those indicators that contain more information, the weights were computed as defined in Eq. (4).
Once the weights were determined, the Technique for Order of Preference by Similarity to Ideal Solution (TOPSIS) (53) was utilized to aggregate them and generate a composite measure of each administrative unit’s contribution to One Health. Here, TOPSIS was used to evaluate the proximity of a set of alternatives (administrative units in this case) to an ideal solution in terms of One Health.
The ideal administrative unit represents a theoretical scenario with the best scores for all the indicators related to the facilitation of zoonotic disease spread. In practice, this scenario is highly unlikely, as it should usually be the case that the highest values of the indicators are distributed over several administrative units. Therefore, TOPSIS calculates the distance between these real solutions and the ideal solution through a series of steps. Again, the first one was to normalize the decision-making matrix. In this case, a vector normalization as shown in Eq. (5) is proposed for the TOPSIS method (54).
The weights yielded by Eq. (4) were then multiplied by the normalized values in Eq. (5) to result in a set of normalized weighted values . These were in turn used to determine the positive ( ) and negative ( ) ideal solutions through Eqs. (6) and (7), respectively.
where and represent indicators that are beneficial and harmful to the spread of zoonotic diseases, respectively. The distances ( and ) from the actual administrative units to these ideal solutions were calculated by applying Eqs. (8) and (9).
Finally, the Relative Closeness ( ) from the administrative units to the ideal solution was computed using Eq. (10). The higher the value of , the more susceptible the administrative unit is to zoonoses (the less it contributes to One Health), and vice versa.
The validity of the values of obtained from Eq. (10) was assessed by comparing them with the records of infectious disease counts in the case study area. This comparison was conducted using Pearson’s correlation coefficient (55) since both variables were quantitative (continuous). The presence of a statistically significant association was determined at a significance level ( ) of 0.05. Therefore, the proposed indicator system was considered valid if it exhibited a strong positive correlation with the infectious disease count, and the value of p obtained from Pearson’s was less than .
Moreover, the results were also analyzed in terms of their relationship with Green Infrastructure (GI). The potential benefits of GI for the three pillars of One Health have been discussed in previous literature (24). GI can be defined as “a strategically planned network of natural and semi-natural areas with other environmental features designed and managed to deliver a wide range of ecosystem services. It incorporates green spaces (or blue if aquatic ecosystems are concerned) and other physical features in terrestrial (including coastal) and marine areas” (56).
The correlation between One Health and GI was determined by the correlation coefficient between these values of and the area covered by GI in each administrative unit. The latter was determined based on the following classes in the Corine Land Cover (CLC) (57): 1.4 (artificial, non-agricultural vegetated areas), 2 (agricultural areas), 3.1 (forest), 3.2 (shrub and/or herbaceous vegetation associations), 4 (wetlands), and 5.1 (inland waters). These categories allowed for differentiation between artificial (class 1.4) and natural and semi-natural (classes 2, 3.1, 3.2, 4, and 5) GI.
The indicator system was tested in the 33 counties of the Valencian Community as shown in Figure 2. The Valencian Community is located in eastern Spain and has a predominantly Mediterranean, arid, and semi-arid climate. Regions with Mediterranean ecosystems, like the Valencian Community, are known to experience high levels of environmental degradation due to biophysical factors such as wildfires, drought, erosion, as well as social factors such as tourism, urbanization, and deforestation (58, 59).
In terms of biophysical factors, it is noteworthy that 17% of the forest area in the region has experienced at least one wildfire, 29% suffers from serious erosion problems, and 46% is at risk of desertification. In addition, the effects of climate change significantly affect the regularity of precipitation, which can have implications for areas prone to desertification (58, 60).
Concerning social factors, the region is characterized by a dominance of the service sector, which accounts for more than 65% of total employment, and tourism activities, such as real estate, gastronomy, and transportation, which have an employment rate of over 12% and contribute 15% to the GDP (61, 62). In particular, the Valencian Community has cultivated a tourism model focused on second homes linked to construction that has resulted in serious environmental impacts, high space requirements, and an unsustainable approach (63).
All these activities have been boosted by lax political guidelines and favorable economic incentives, leading to land degradation (58) (EVR, 2020). Thus, insufficient budgetary resources further contribute to the challenge of meeting the goal of recovering 15% of degraded ecosystems. Besides, other studies indicate that the Valencian Community has primarily focused on reforestation projects with limited impact on biodiversity, while neglecting medium and long-term-ecological restoration projects (64, 65).
The systematic literature review conducted following the PRISMA statement produced the results presented in Figure 3, which shows the number of records removed at each step. The search query formulated in Eq. (1) initially returned 463 records from the three databases considered. This number gradually decreased through the different steps of the PRISMA statement until a final number of 99 articles was obtained for data analysis.
Figure 3. Number of records retained and removed after each step of the systematic literature review.
The extraction of information from these articles allowed for the identification of several indicators that could potentially influence the spread of diseases impacting human, animal, and environmental health. The vast majority of these indicators were not used as direct metrics to model spatial facilitation of disease spread but were instead discussed as factors that may affect One Health. Table 1 compiles the list of indicators extracted from the review and provides the main references that supported their use as surrogates for aspects that go against the One Health concept (9, 14, 40, 66–161).
Table 1. List of proposed or derivable indicators from the systematic literature review on metrics used to address One Health.
Certainindicators were discarded due to limited data availability, low frequency, and/or the impossibility to characterize them spatially. Based on the frequency of the indicators found to be valid, the list in Table 2 was compiled for subsequent calculations. The first subset of indicators (from to ) focused on population demographics (either human or animal) and socioeconomic aspects such as health facility density, education level, and financial resources. Another group consisted of indicators related to land use (from to ). The interactions between animal and human populations and water bodies were addressed in the indicators ranging from to . The final group of indicators pertained to environmental conditions, including air quality ( and ) and climate ( and ).
The rationale behind the influence of these indicators on One Health is outlined below. The impact of Antimicrobial Resistance (AMR) and its implications for One Health has been linked to reduced accessibility to adequate healthcare and human health ( ) as well as veterinary ( ) professionalism (83). Moreover, high population densities ( , , and ) can contributeto the spread of zoonotic diseases and increased intra-interactions within and between populations and ecosystems (81). In the case of human populations, factors such as poverty ( ) can support the evolution of pathogen life cycles, while education ( ) can aid in controlling global zoonotic pathogens (75).
The impact of agriculture ( ) is associated with freshwater, which serves as a common drinking source for wildlife (69), as reflected in . Agricultural runoff can be a source of pollution, especially if antibiotics are utilized in farming practices (68). The use of agrochemicals in agriculture contributes to reduced biodiversity and promotes mutation among microbial populations in soil and water bodies ( ) (162). Other forms of anthropogenic land use changes such as deforestation ( ) are linked to the increased adaptability of disease vectors and the creation of the conditions for increased interactions at the wildlife-human interface (163). and relate to aspects like the role of hunted animals as carriers of bacteria with specific resistance traits (92) and livestock movement as a contributing factor to the emergence of zoonotic diseases (164).
Increased global connectivity between humans and animals (either livestock or wildlife; and ) has been identifiedas a source of acceleratedand exacerbated AMR (70). Surface water (streams, rivers, lakes, and ponds) used as drinking water for dairy cattle can serve as a source of pathogens (72) ( ), posingbilateral implications for farm animals and humans ( ) due to the dissemination and maintenance of resistance genes in the environment (165).
The impact of air pollution ( and ) is indirect as it stems from its contribution to biodiversity decline (166), which in turn has been argued to increase human exposure to zoonotic pathogens (167). Moisture resulting from precipitation ( ) results in dense vegetation that provides suitable conditions for vector proliferation (168). Floods caused by heavy rainfall also increase the risk of waterborne diseases (169). Temperature ( ) is proportional to vector distribution and disease risk too. High temperatures promote increased activity of mosquitoes, ticks, and sandflies, while they can lead to the migration of rodents into human habitats (170, 171).
Some indicators in Table 2 were obtained directly as single values per administrative unit, while others were available as either vector (point or polygon) or raster layers. These data had to be processed to express them as a single value per administrative unit. The indicators in point format were processed to obtain densities per county. Instead, polygon data were used to determine the proportion of the area corresponding to the indicators covered by the counties.
The indicators related to the interactions between point and polygon layers ( , and ) were determined through a three-step process: transform the point layer into a raster using a Kernel density function (shape = quartic, radius = 25 km), calculate the interaction of the gridded density and the polygon layer by multiplication, and aggregate the values per county by taking the median of the interaction values per raster unit into a polygon layer.
Apart from these general procedures, two indicators required specific calculations. Deforestation ( ) was determined based on the variations in class 3.1 (Forests) in the 2006, 2012, and 2018 CLC maps in the Valencian Community. The CLC map was also used to characterize water and soil pollution ( ), which was computed by assigning scores depending on the land cover classes (172).
Figure 4 shows various steps in the processing of indicators as an example. Figure 4A depicts the location of health and veterinary centers in the study area, which were used to obtain their density per county ( and ). Figure 4B applies the aforementioned scores for water and soil pollution to the different land covers in the study area. Figures 4C,D represent the presence of wild animals and livestock areas in the region along with the human population values per county, which were the inputs used to characterize and . The values per county for each indicator considered ( - ) are provided as Supplementary material.
Figure 4. Processing of indicators before their calculation per county (A) Health and veterinary centers ( and ); (B) Water and soil pollution ( ); (C) Interaction between human population and wildlife ( ); and (D) Interaction between human population and livestock ( ).
The application of the EM algorithm according to Eqs. (2)–(4) resulted in the weights shown in Figure 5. These weights indicate the importance of various indicators for this study. Some indicators were found to have reduced importance (weights less than 0.025 out of 1): including healthcare facilities (for humans or animals), temperature, and NOx concentration, deforestation, hunting territory, and water and soil pollution. According to the EM principle, these variables are not sufficiently discriminatory among the counties in the Valencian Community, indicating that most counties are relatively homogeneous in relation to these indicators.
Instead, the indicators related to human and animal populations and their interactions obtained the highest weights. In particular, the presence of wildlife and humans was identified as the two most important indicators. This highlights the significance of distinct habitats and the risks associated with phenomena such as urban sprawl. The next most important indicators were also aligned with this focus, involving domestic and farm animals and their interactions with wildlife, humans, and water bodies, with the latter also being represented by precipitation. The ozone uptake by wheat was also identified as an important environmental indicator due to its impact on food safety.
Apart from the rationale behind them, all of these indicators with the highest weights exhibited spatial variability among the counties in the study area. In addition, they accounted for the three dimensions of One Health. Among them, animal-related variables were the most important ( - , - ), followed by those focused on humans ( , , and ) and the environment ( , and ).
The use of Eqs. (5)–(10) following the steps of the TOPSIS method resulted in the map shown in Figure 6A. The results are consistent with the significance of indicators associated with humans and wildlife (Figure 5), as well as the distribution of these populations (Figure 4A). According to this map, the primary focus of disease spread is observed in the county of València (Figure 2), where the region’s capital is situated, highlighting the implications of population movement in this area.
Figure 6. (A) Susceptibility to zoonoses according to the indicator system; (B) Infectious disease density (cases/km2); (C) Proportion of artificial GI (%); and (D) Proportion of natural and semi-natural GI (%).
The second county in the ranking of zoonotic disease susceptibility was el Baix Segura, located in the southern of the Valencian Community. Although the human population density is not as high in this county, it attained the highest scores in terms of wildlife-livestock-water interactions. Instead, counties in the western and northern regions exhibited lower susceptibility. As can be seen from the results provided as Supplementary material, these counties only obtained high scores in indicators of lesser importance (Figure 5), such as illiterate population ( ), average income per consumption unit ( ), or hunting territory ( ).
Overall, the results presented in Figure 6A are instructive in terms of indicating hotspots linked to susceptibility to infectious diseases. The indicator system based on the EM and TOPSIS methods enables the derivation of discriminative results, highlighting substantial differences between the most critical counties and the others. Consequently, this facilitates the implementation of strategies to strengthen One Health through measures aimed at safeguarding the interface between humans, animals, and the environment.
The Valencian Animal Identification Registry (RIVIA in Spanish) provides a report on the cases notified in 2021 for the following diseases: Leishmaniosis, Ehrlichiosis, Dirofilariosis, Leptospirosis, Toxoplasmosis, and Babesiosis. Figure 6B shows how these cases are distributed across the counties in the Valencian Community.
Figure 7A demonstrates that the value of Pearson’s obtained between disease density (number of records per county area) and the values of was 0.72 (value of p < 0.05). This strong and positive correlation coefficient reinforces the effectiveness of the proposed indicator system in representing a region’s susceptibility to the emergence of infectious diseases.
Figure 7. Correlation coefficient between the results of applying the indicator system to the Valencian Community and (A) Infectious disease density reported in 2021 (cases/km2); (B) Proportion of artificial GI (%); and (C) Proportion of natural and semi-natural GI (%).
A similar analysis was carried out to examine the relationship between and the proportion of artificial (Figure 6C) and natural and semi-natural GI (Figure 6D) in the study area. Again, the p values were below the significance level of 0.05 in both cases, while the values of Pearson’s obtained were 0.78 (Figure 7B) and − 0.73 (Figure 7C), respectively. These results indicate that more developed and urbanized counties exhibit a higher susceptibility to the emergence of infectious diseases, while the presence of natural and semi-natural areas may contribute to the One Health initiative.
The results obtained in this study address some key demands in the field of landscape epidemiology, including the incorporation of spatial interactions between individuals and environmental gradients in large-scale studies (173). Spatial dimensions such as distances between humans, animals, and environments have been found to be associated with both directly and indirectly transmitted infectious diseases (174). Overall, the spatial processing of indicators proposed in this study aligns with these premises.
The results are also consistent with the notion that urbanization contributes to increased encounters with wildlife, leading to challenges in infectious disease epidemiology due to amplified and faster spread (175). Although the interface between human and wildlife populations ( ) carries moderate weight, wild animals ( ) and human population ( ) were identified as the two most important indicators according to Figure 5.
Other authors have emphasized that land urbanization, rather than population urbanization is a key driver of infectious disease morbidity and mortality (176). This does not necessarily contradict the results obtained in this study but underscores the importance of using appropriate metrics. Population density goes beyond population size by considering how the accumulation of people and animals can facilitate disease transmission.
Urbanization promotes the occurrence of zoonoses through demographic growth and density, socioeconomic inequalities, increased movement of people and animals, and land use change (177). All these factors are included in the list of indicators presented in Table 1. The results in Figure 7 would be supported by this line of thought, since considering these aspects together correlates positively with the number of infectious diseases and the presence of natural and semi-natural GI, while correlating negatively with artificial GI in more urbanized areas.
The role of GI is linked to biodiversity, which is a crucial factor to consider when pursuing One Health goals. Zoonotic pathogens are more likely to originate from specific taxa that often reproduce due to human influences, i.e., in urbanized areas lacking biodiversity values (167). This may explain the lower susceptibility to infectious diseases in counties with higher proportions of natural and semi-natural GI. Notably, the provision of suitable habitats for vector and zoonotic reservoir populations is one of the regulating ecosystem services supported by GI (178).
Therefore, the results of the indicator system can support the development of planning strategies aimed at promoting the principles of One Health. Strategies could focus on the implementation of natural GI, as the presence of these areas showed a negative correlation with zoonoses. Preserving and/or restoring natural landscape features can help minimize habitat encroachment, improve land and water quality, and mitigate extreme atmospheric conditions. Instead, artificial green spaces, commonly found in urban areas, showed a positive correlation with zoonoses. This is associated with urban sprawl and its effects on increased interactions between populations through greater movement of people, animals, and wildlife between developed and undeveloped areas.
Although these conclusions stem from validated results, this investigation had some limitations that constrain its impact. First, the data used to characterize certain indicators were site-specific, which hampers the usability of the indicator system in other parts of the world where such data may be lacking. Second, the methodology behind the indicator system could benefit from automation, which would result in a web-based application where users only need to input the data to obtain the composite metric of zoonotic disease susceptibility, while calculating the GIS and MCDA tasks would be in the background. Finally, this indicator system may have limitations in modeling diseases transmitted by vectors such as ticks or mosquitoes, whose distribution and survival are influenced by complex dynamics determined by climatic and seasonal factors, as well as by the presence of specific hosts, with certain conditions being favorable for some species but not for others (179, 180).
This research consisted of the development, application, and validation of an indicator system to model spatial susceptibility to zoonotic disease facilitation, thus providing an indirect measurement of contributions to the One Health initiative. The study focused on the counties of the Valencian Community (Spain) as a case study. The methods used to achieve this goal included a systematic literature review, the combination of GIS and MCDM techniques, and the use of statistical testing.
The systematic literature review resulted in the identification of 22 spatial indicators that encompassed population, land use, and atmospheric variables. These indicators represented the risks associated with direct or indirect interactions at the interfaces between humans, wildlife, livestock, and ecosystems, thereby addressing the three pillars of the One Health approach: human, animal, and environmental health.
Processing these indicators using GIS and MCDM methods resulted in a composite metric for zoonotic disease susceptibility. The results showed that the indicators concerning human and animal populations and their interactions are the most important ones, underlining the relevance of controlling urban sprawl to mitigate habitat encroachment. The impact of urbanization was also further supported by the county level analysis, with the highest susceptibility to zoonotic diseases observed in the county corresponding to the capital and most populated city in the region.
This trend was confirmed by the strong positive correlation between the results of the indicator system and the presence of artificial GI. Conversely, the association with natural and semi-natural GI exhibited an opposite relationship, underscoring the importance of these areas for habitat preservation and biodiversity protection, thus aligning with the principles of One Health. The validity of these findings was verified by comparing the results of the indicator system with the records reported in the Valencian Community in 2021, showing a strong positive correlation. Therefore, the indicator system is proposed as a tool for implementing the principles of the One Health approach when designing strategies for better public space planning.
The original contributions presented in the study are included in the article/Supplementary material; further inquiries can be directed to the corresponding author.
DJ-E conceived the research, contributed to the literature review, analyzed the data, discussed the results, and wrote the manuscript. FM-V contributed to the literature review, produced the results, and wrote the manuscript. VM contributed to the literature review and produced the results. FC-R contributed to the literature review, wrote the manuscript, and reviewed the manuscript. LB contributed to the literature review and reviewed the manuscript. All authors contributed to the article and approved the submitted version.
This study was financed through the research projects ECOVAL (grant number CIGE/2021/079) and ECOHEALTH (grant number PII2022_04), funded by the Conselleria for Innovation, Universities, Science and Digital Society of the Generalitat Valenciana and the Valencian International University (VIU), respectively.
The authors declare that the research was conducted in the absence of any commercial or financial relationships that could be construed as a potential conflict of interest.
All claims expressed in this article are solely those of the authors and do not necessarily represent those of their affiliated organizations, or those of the publisher, the editors and the reviewers. Any product that may be evaluated in this article, or claim that may be made by its manufacturer, is not guaranteed or endorsed by the publisher.
The Supplementary material for this article can be found online at: https://www.frontiersin.org/articles/10.3389/fpubh.2023.1215574/full#supplementary-material
1. Salyer, SJ, Silver, R, Simone, K, and Barton, BC. Prioritizing Zoonoses for Global Health capacity building—themes from one health zoonotic disease workshops in 7 countries, 2014–2016. Emerg Infect Dis. (2017) 23:S55–64. doi: 10.3201/eid2313.170418
2. Iwu, CD, and Okoh, AI. Preharvest transmission routes of fresh produce associated bacterial pathogens with outbreak potentials: a review. IJERPH. (2019) 16:4407. doi: 10.3390/ijerph16224407
3. Allen, T, Murray, KA, Zambrana-Torrelio, C, Morse, SS, Rondinini, C, Di Marco, M, et al. Global hotspots and correlates of emerging zoonotic diseases. Nat Commun. (2017) 8:1124. doi: 10.1038/s41467-017-00923-8
4. CDC One health basics. Centers for Disease Control and Prevention. (2022) Available at: https://www.cdc.gov/onehealth/basics/index.html (Accessed April 4, 2023)
5. Rüegg, SR, Nielsen, LR, Buttigieg, SC, Santa, M, Aragrande, M, Canali, M, et al. A systems approach to evaluate one health initiatives. Front Vet Sci. (2018) 5:23. doi: 10.3389/fvets.2018.00023
6. Zinsstag, J, Schelling, E, Waltner-Toews, D, and Tanner, M. From “one medicine” to “one health” and systemic approaches to health and well-being. Prev Vet Med. (2011) 101:148–56. doi: 10.1016/j.prevetmed.2010.07.003
7. Destoumieux-Garzón, D, Mavingui, P, Boetsch, G, Boissier, J, Darriet, F, Duboz, P, et al. The one health concept: 10 years old and a long road ahead. Front Vet Sci. (2018) 5:14. doi: 10.3389/fvets.2018.00014
8. Feng, J, Guo, Z, Ai, L, Liu, J, Zhang, X, Cao, C, et al. Establishment of an indicator framework for global one health intrinsic drivers index based on the grounded theory and fuzzy analytical hierarchy-entropy weight method. Infect Dis Poverty. (2022) 11:121. doi: 10.1186/s40249-022-01042-3
9. Zhang, X-X, Liu, J-S, Han, L-F, Xia, S, Li, S-Z, Li, OY, et al. Towards a global one health index: a potential assessment tool for one health performance. Infect Dis Poverty. (2022) 11:57. doi: 10.1186/s40249-022-00979-9
10. Zhao, H-Q, Fei, S-W, Yin, J-X, Li, Q, Jiang, T-G, Guo, Z-Y, et al. Assessment of performance for a key indicator of one health: evidence based on one health index for zoonoses in sub-Saharan Africa. Infect Dis Poverty. (2022) 11:109. doi: 10.1186/s40249-022-01020-9
11. Barton, BC. Introduction One Health: over a decade of progress on the road to sustainability: -EN- -FR-Introduction. Une seule santé: plus d’une décennie d’avancées vers la durabilité-ES-Introducción. Una sola salud: más de un decenio de avances en la senda de la sostenibilidad. Rev Sci Tech OIE. (2019) 38:21–50. doi: 10.20506/rst.38.1.2939
12. Li, P, Wu, J, and Shukla, S. Achieving the one health goal: highlighting groundwater quality and public health. WaterSA. (2022) 14:3540. doi: 10.3390/w14213540
13. Ung, L, Stothard, JR, Phalkey, R, Azman, AS, Chodosh, J, Hanage, WP, et al. Towards global control of parasitic diseases in the Covid-19 era: one health and the future of multisectoral global health governance. Adv Parasitol Elsevier. (2021):1–26. doi: 10.1016/bs.apar.2021.08.007
14. Wilcox, BA, Aguirre, AA, De Paula, N, Siriaroonrat, B, and Echaubard, P. Operationalizing one health employing social-ecological systems theory: lessons from the greater Mekong sub-region. Front Public Health. (2019) 7:85. doi: 10.3389/fpubh.2019.00085
15. Moore, SM, ten Bosch, QA, Siraj, AS, Soda, KJ, España, G, Campo, A, et al. Local and regional dynamics of chikungunya virus transmission in Colombia: the role of mismatched spatial heterogeneity. BMC Med. (2018) 16:152. doi: 10.1186/s12916-018-1127-2
16. Sattenspiel, L, and Lloyd, A. The Geographic Spread of Infectious Diseases: Models and Applications. Princeton, New Jersey, U.S.: Princeton University Press (2009). 304 p.
17. The Lancet. Zoonoses: beyond the human–animal–environment interface. Lancet. (2020) 396:1. doi: 10.1016/S0140-6736(20)31486-0
18. Liu, Q, Xu, W, Lu, S, Jiang, J, Zhou, J, Shao, Z, et al. Landscape of emerging and re-emerging infectious diseases in China: impact of ecology, climate, and behavior. Front Med. (2018) 12:3–22. doi: 10.1007/s11684-017-0605-9
19. Alirol, E, Getaz, L, Stoll, B, Chappuis, F, and Loutan, L. Urbanisation and infectious diseases in a globalised world. Lancet Infect Dis. (2011) 11:131–41. doi: 10.1016/S1473-3099(10)70223-1
20. Chowell, G, and Rothenberg, R. Spatial infectious disease epidemiology: on the cusp. BMC Med. (2018) 16:192. doi: 10.1186/s12916-018-1184-6
21. Tasker, A, and Braam, D. Positioning zoonotic disease research in forced migration: a systematic literature review of theoretical frameworks and approaches. PLoS One. (2021) 16:e0254746. doi: 10.1371/journal.pone.0254746
22. Eubank, S, Guclu, H, Anil Kumar, VS, Marathe, MV, Srinivasan, A, Toroczkai, Z, et al. Modelling disease outbreaks in realistic urban social networks. Nature. (2004) 429:180–4. doi: 10.1038/nature02541
23. Heesterbeek, H, Anderson, RM, Andreasen, V, Bansal, S, De Angelis, D, Dye, C, et al. Modeling infectious disease dynamics in the complex landscape of global health. Science. (2015) 347:aaa4339. doi: 10.1126/science.aaa4339
24. Felappi, JF, Sommer, JH, Falkenberg, T, Terlau, W, and Kötter, T. Green infrastructure through the lens of “one health”: a systematic review and integrative framework uncovering synergies and trade-offs between mental health and wildlife support in cities. Sci Total Environ. (2020) 748:141589. doi: 10.1016/j.scitotenv.2020.141589
25. Wilson, R, Tiedt, S, and Murray, K. Zoonotic infectious diseases as ecosystem disservices: a retrospective data review. Lancet Planet Health. (2021) 5:S23. doi: 10.1016/S2542-5196(21)00107-8
26. Petrovan, SO, Aldridge, DC, Bartlett, H, Bladon, AJ, Booth, H, Broad, S, et al. Post COVID-19: a solution scan of options for preventing future zoonotic epidemics. Biol Rev. (2021) 96:2694–715. doi: 10.1111/brv.12774
27. Zhou, X-N, and Tanner, M. Science in one health: a new journal with a new approach. Sci One Health. (2022) 1:100001. doi: 10.1016/j.soh.2022.100001
28. Arino, J, and van den Driessche, P. A multi-city epidemic model. Math Popul Stud. (2003) 10:175–93. doi: 10.1080/08898480306720
29. Arino, J, Davis, JR, Hartley, D, Jordan, R, Miller, JM, and van den Driessche, P. A multi-species epidemic model with spatial dynamics. Math Med Biol J IMA. (2005) 22:129–42. doi: 10.1093/imammb/dqi003
30. Bashir, RSE, and Hassan, OA. A one health perspective to identify environmental factors that affect Rift Valley fever transmission in Gezira state. Central Sudan Trop Med Health. (2019) 47:54. doi: 10.1186/s41182-019-0178-1
31. Bhattacharya, D, Kshatri, JS, Choudhary, HR, Parai, D, Shandilya, J, Mansingh, A, et al. One health approach for elimination of human anthrax in a tribal district of Odisha: study protocol. PLoS One. (2021) 16:e0251041. doi: 10.1371/journal.pone.0251041
32. Sharun, K, Tiwari, R, Natesan, S, and Dhama, K. SARS-CoV-2 infection in farmed minks, associated zoonotic concerns, and importance of the one health approach during the ongoing COVID-19 pandemic. Vet Q. (2021) 41:50–60. doi: 10.1080/01652176.2020.1867776
33. Taetzsch, SJ, Bertke, AS, and Gruszynski, KR. Zoonotic disease transmission associated with feral cats in a metropolitan area: a geospatial analysis. Zoonoses Public Health. (2018) 65:412–9. doi: 10.1111/zph.12449
34. Dietrich, D, Dekova, R, Davy, S, Fahrni, G, and Geissbühler, A. Applications of space technologies to Global Health: scoping review. J Med Internet Res. (2018) 20:e230. doi: 10.2196/jmir.9458
35. Olugasa, BO, and Fasunla, AJ. One health data audit: a spatio-temporal approach to cultivating sustainable multi-disciplinary collaboration and communication in zoonoses surveillance, control and stepwise elimination. PAMJ One Health. (2020) 1:5. doi: 10.11604/pamj-oh.2020.1.5.22350
36. Guptill, SC, and Moore, CG. Investigating vector-borne and zoonotic diseases with remote sensing and GIS In: O Selinus, editor. Essentials of Medical Geology. Dordrecht, The Netherlands: Springer Netherlands (2013). 647–63.
37. Jahanfar, A, Drake, J, Sleep, B, and Margolis, L. Evaluating the shading effect of photovoltaic panels on green roof discharge reduction and plant growth. J Hydrol. (2019) 568:919–28. doi: 10.1016/j.jhydrol.2018.11.019
38. Mollalo, A, and Khodabandehloo, E. Zoonotic cutaneous leishmaniasis in northeastern Iran: a GIS-based spatio-temporal multi-criteria decision-making approach. Epidemiol Infect. (2016) 144:2217–29. doi: 10.1017/S0950268816000224
39. Souza IP de O, Uberti MS, Tassinari W de SGeoprocessing and spatial analysis for identifying leptospirosis risk areas: a systematic review. Rev Inst Med trop S Paulo. (2020) 62:e35. doi: 10.1590/s1678-9946202062035
40. de Moura, RR, Chiba de Castro, WA, Farinhas, JH, Pettan-Brewer, C, Kmetiuk, LB, dos Santos, AP, et al. One health index (OHI) applied to Curitiba, the ninth-largest metropolitan area of Brazil, with concomitant assessment of animal, environmental, and human health indicators. One Health. (2022) 14:100373. doi: 10.1016/j.onehlt.2022.100373
41. Errecaborde, KM, Macy, KW, Pekol, A, Perez, S, O’Brien, MK, Allen, I, et al. Factors that enable effective one health collaborations—a scoping review of the literature. PLoS One. (2019) 14:e0224660. doi: 10.1371/journal.pone.0224660
42. Vesterinen, HM, Dutcher, TV, Errecaborde, KM, Mahero, MW, Macy, KW, Prasarnphanich, O-O, et al. Strengthening multi-sectoral collaboration on critical health issues: one health systems mapping and analysis resource toolkit (OH-SMART) for operationalizing one health. PLoS One. (2019) 14:e0219197. doi: 10.1371/journal.pone.0219197
43. Henley, P, Igihozo, G, and Wotton, L. One health approaches require community engagement, education, and international collaborations—a lesson from Rwanda. Nat Med. (2021) 27:947–8. doi: 10.1038/s41591-021-01350-5
44. Liberati, A, Altman, DG, Tetzlaff, J, Mulrow, C, Gøtzsche, PC, Ioannidis, JPA, et al. The PRISMA statement for reporting systematic reviews and Meta-analyses of studies that evaluate health care interventions: explanation and elaboration. PLoS Med. (2009) 6:e1000100. doi: 10.1371/journal.pmed.1000100
45. Falagas, ME, Pitsouni, EI, Malietzis, GA, and Pappas, G. Comparison of PubMed, Scopus, web of science, and Google scholar: strengths and weaknesses. FASEB J. (2008) 22:338–42. doi: 10.1096/fj.07-9492LSF
46. Evans, BR, and Leighton, FA. A history of one health: -EN-A history of one health-FR-histoire du concept « Une seule santé » -ES-Historia de «Una sola salud». Rev Sci Tech OIE. (2014) 33:413–20. doi: 10.20506/rst.33.2.2298
47. Mackenzie, JS, and Jeggo, M. The one health approach—why is it so important? Trop Med. (2019) 4:88. doi: 10.3390/tropicalmed4020088
48. Elnathan, R. English is the language of science — but precision is tough as a non-native speaker. Nature (2021) doi: 10.1038/d41586-021-00899-y (Epub ahead of print).
49. Ma, W, Du, Y, Liu, X, and Shen, Y. Literature review: multi-criteria decision-making method application for sustainable deep-sea mining transport plans. Ecol Indic. (2022) 140:109049. doi: 10.1016/j.ecolind.2022.109049
50. Jato-Espino, D, Castillo-Lopez, E, Rodriguez-Hernandez, J, and Canteras-Jordana, JC. A review of application of multi-criteria decision making methods in construction. Autom Constr. (2014) 45:151–62. doi: 10.1016/j.autcon.2014.05.013
52. Zhu, Y, Tian, D, and Yan, F. Effectiveness of entropy weight method in decision-making. Math Probl Eng. (2020) 2020:1–5. doi: 10.1155/2020/3564835
53. Hwang, C-L, and Yoon, K. Multiple Attribute Decision Making. Berlin, Heidelberg: Springer Berlin Heidelberg (1981).
54. Çelen, A. Comparative analysis of normalization procedures in TOPSIS method: with an application to Turkish deposit banking market. Informatica. (2014) 25:185–208. doi: 10.15388/Informatica.2014.10
55. Kirch, W. Pearson’s correlation coefficient In:. Encyclopedia of Public Health. Dordrecht: Springer Netherlands (2008). 1090–1.
56. European Commission Green infrastructure (GI)—Enhancing Europe’s natural capital. (2013). Available at: https://eur-lex.europa.eu/legal-content/EN/TXT/?uri=CELEX:52013DC0249 (Accessed September 29, 2022).
57. European Union Copernicus land monitoring service. CORINE Land Cover (2015). Available at: https://land.copernicus.eu/pan-european/corine-land-cover (Accessed September 2, 2022).
58. Generalitat Valenciana Plan de Acción Territorial Forestal de la Comunitat Valenciana–PATFOR. Medio Natural (2019) Available at: https://agroambient.gva.es/ca/web/medio-natural/patfor (Accessed March 3, 2023).
59. Zdruli, P. Land resources of the Mediterranean: status, pressures, trends and impacts on future regional development. Land Degrad Dev. (2014) 25:373–84. doi: 10.1002/ldr.2150
60. Millán Muñoz, M. Sequía en el Mediterréneo e inundaciones en el Reino Unido y Centroeuropa: cosas que los modelos climáticos globales no ven del ciclo hídrico en Europa, y por qué. Cuides Cuaderno Interdisciplinar de Desarrollo Sostenible. (2010) 7–100. Available at: https://dialnet.unirioja.es/servlet/articulo?codigo=3201315 (Accessed March 3, 2023).
61. EVR. Generalitat Valenciana. Propuesta de Estrategia Valenciana para la Recuperación, EVR: documento de trabajo. Acuerdos para la recuperación de la Comunidad Valenciana (2020) https://gvaoberta.gva.es/es/acords-per-a-la-recuperacio-de-la-comunitat-valenciana-alcem-nos (Accessed June 20, 2023).
62. INE. Distribución porcentual de los activos por sector económico y provincia. Instituto Nacional de Estadística (2022) https://www.ine.es/jaxiT3/Tabla.htm?t=3994&L=0 (Accessed June 20, 2023).
63. Aledo, A, Climent-Gil, E, and Mañas-Navarro, JJ. “Aportaciones teóricas, metodológicas y aplicadas de la sociología a la planificación del turismo.,” Sociología del turismo. Madrid (Spain): Centro de Investigaciones Sociológicas (2019). 93–125. Available at: http://rua.ua.es/dspace/handle/10045/120010 (Accessed March 3, 2023)
64. Gann, GD, McDonald, T, Walder, B, Aronson, J, Nelson, CR, Jonson, J, et al. International principles and standards for the practice of ecological restoration. Restor Ecol. (2019) 27:S1–S46. doi: 10.1111/rec.13035
65. WWF. Recuperando paisajes: un nuevo camino para la restauración ecológica. World Wide Fund for Nature (2016) https://www.wwf.es/?38160/Espaa-va-camino-de-incumplir-las-metas-europeas-de-restauracin-ecolgica (Accessed March 3, 2023).
66. Mencía-Ares, O, Argüello, H, Puente, H, Gómez-García, M, Manzanilla, EG, Álvarez-Ordóñez, A, et al. Antimicrobial resistance in commensal Escherichia coli and Enterococcus spp. is influenced by production system, antimicrobial use, and biosecurity measures on Spanish pig farms. Porc Health Manag. (2021) 7:27. doi: 10.1186/s40813-021-00206-1
67. Stentiford, GD, Bateman, IJ, Hinchliffe, SJ, Bass, D, Hartnell, R, Santos, EM, et al. Sustainable aquaculture through the one health lens. Nat Food. (2020) 1:468–74. doi: 10.1038/s43016-020-0127-5
68. Zira, S, Röös, E, Ivarsson, E, Friman, J, Møller, H, Samsonstuen, S, et al. An assessment of scenarios for future pig production using a one health approach. Livest Sci. (2022) 260:104929. doi: 10.1016/j.livsci.2022.104929
69. Dias, D, Fonseca, C, Caetano, T, and Mendo, S. Oh, deer! How worried should we be about the diversity and abundance of the faecal resistome of red deer? Sci Total Environ. (2022) 825:153831. doi: 10.1016/j.scitotenv.2022.153831
70. Lambraki, IA, Majowicz, SE, Parmley, EJ, Wernli, D, Léger, A, Graells, T, et al. Building social-ecological system resilience to tackle antimicrobial resistance across the one health spectrum: protocol for a mixed methods study. JMIR Res Protoc. (2021) 10:e24378. doi: 10.2196/24378
71. Zana, B, Erdélyi, K, Nagy, A, Mezei, E, Nagy, O, Takács, M, et al. Multi-approach investigation regarding the West Nile virus situation in Hungary, 2018. Viruses. (2020) 12:123. doi: 10.3390/v12010123
72. Dow, CT, and Alvarez, BL. Mycobacterium paratuberculosis zoonosis is a one health emergency. Ecosyst Health. (2022) 19:164–74. doi: 10.1007/s10393-022-01602-x
73. Kamaruzzaman, EA, Aziz, SA, Bitrus, AA, Zakaria, Z, and Hassan, L. Occurrence and characteristics of extended-spectrum β-lactamase-producing Escherichia coli from dairy cattle, milk, and farm environments in peninsular Malaysia. Pathogens. (2020) 9:1–10. doi: 10.3390/pathogens9121007
74. Nel, LH. Discrepancies in data reporting for rabies. Africa Emerg Infect Dis. (2013) 19:529–33. doi: 10.3201/eid1904.120185
75. Singh, BB, and Gajadhar, AA. Role of India’s wildlife in the emergence and re-emergence of zoonotic pathogens, risk factors and public health implications. Acta Trop. (2014) 138:67–77. doi: 10.1016/j.actatropica.2014.06.009
76. Mercato, A, Cortimiglia, C, Abualshaar, A, Piazza, A, Marchesini, F, Milani, G, et al. Wild boars as an Indicator of environmental spread of ESβL-producing Escherichia coli. Front Microbiol. (2022) 13:838383. doi: 10.3389/fmicb.2022.838383
77. Rostal, MK, Olival, KJ, Loh, EH, and Karesh, WB. Wildlife: the need to better understand the linkages. Curr Top Microbiol Immunol. (2013) 365:101–25. doi: 10.1007/82-2012-271
78. Benitez, ADN, Martins, FDC, Mareze, M, Santos, NJR, Ferreira, FP, Martins, CM, et al. Spatial and simultaneous representative seroprevalence of anti-toxoplasma gondii antibodies in owners and their domiciled dogs in a major city of southern Brazil. PLoS One. (2017) 12:e0192570. doi: 10.1371/journal.pone.0180906
79. Muramoto, J, Parr, DM, Perez, J, and Wong, DG. Integrated soil health Management for Plant Health and one Health: lessons from histories of soil-borne disease Management in California Strawberries and Arthropod Pest Management. Front Sustain Food Syst. (2022) 6:839648. doi: 10.3389/fsufs.2022.839648
80. Paris, JMG, Falkenberg, T, Nöthlings, U, Heinzel, C, Borgemeister, C, and Escobar, N. Changing dietary patterns is necessary to improve the sustainability of Western diets from a one health perspective. Sci Total Environ. (2022) 811:151437. doi: 10.1016/j.scitotenv.2021.151437
81. McAlester, J, and Kanazawa, Y. Situating zoonotic diseases in peacebuilding and development theories: prioritizing zoonoses in Jordan. PLoS One. (2022) 17:e0265508. doi: 10.1371/journal.pone.0265508
82. Murray, AG, Ives, SC, Smith, RJ, and Moriarty, M. A preliminary assessment of indirect impacts on aquaculture species health and welfare in Scotland during COVID-19 lockdown. Veterinary and animal. Science. (2021) 11:100167. doi: 10.1016/j.vas.2021.100167
83. Iwu, CD, and Patrick, SM. An insight into the implementation of the global action plan on antimicrobial resistance in the WHO African region: a roadmap for action. Int J Antimicrob Agents. (2021) 58:106411. doi: 10.1016/j.ijantimicag.2021.106411
84. Molia, S, Saillard, J, Dellagi, K, Cliquet, F, Bart, J-M, Rotureau, B, et al. Practices in research, surveillance and control of neglected tropical diseases by one health approaches: a survey targeting scientists from french-speaking countries. PLoS Negl Trop Dis. (2021) 15:e0009246. doi: 10.1371/journal.pntd.0009246
85. Scott, LC, Wilson, MJ, Esser, SM, Lee, NL, Wheeler, ME, Aubee, A, et al. Assessing visitor use impact on antibiotic resistant bacteria and antibiotic resistance genes in soil and water environments of Rocky Mountain National Park. Sci Total Environ. (2021) 785:147122. doi: 10.1016/j.scitotenv.2021.147122
86. Cardoso, MD, Santos, AFDM, Rodrigues, MDS, Pribul, BR, Grael, AS, Pedroso, VM, et al. Salmonella spp. profiles isolated from seabird samples from the Brazilian coast. Prev Vet Med. (2021) 193:105413. doi: 10.1016/j.prevetmed.2021.105413
87. Ewbank, AC, Esperón, F, Sacristán, C, Sacristán, I, Neves, E, Costa-Silva, S, et al. Occurrence and quantification of antimicrobial resistance genes in the gastrointestinal microbiome of two wild seabird species with contrasting behaviors. Front Vet Sci. (2021) 8:651781. doi: 10.3389/fvets.2021.651781
88. Ewbank, AC, Esperón, F, Sacristán, C, Sacristán, I, Krul, R, Macedo Cavalcanti, E, et al. Seabirds as anthropization indicators in two different tropical biotopes: a one health approach to the issue of antimicrobial resistance genes pollution in oceanic islands. Sci Total Environ. (2021) 754:142141. doi: 10.1016/j.scitotenv.2020.142141
89. Chen, Q, Ma, X, Rainey, JJ, Li, Y, Mu, D, Tao, X, et al. Findings from the initial stepwise approach to rabies elimination (SARE) assessment in China, 2019. PLoS Negl Trop Dis. (2021) 15:e0009274. doi: 10.1371/journal.pntd.0009274
90. Meyers, AC, Auckland, L, Meyers, HF, Rodriguez, CA, Kontowicz, E, Petersen, CA, et al. Epidemiology of vector-borne pathogens among U.S. government working dogs. Vector-Borne Zoo Dis. (2021) 21:358–68. doi: 10.1089/vbz.2020.2725
91. Alcoba, G, Ochoa, C, Martins, SB, de Castañeda, RR, Bolon, I, Wanda, F, et al. Novel transdisciplinary methodology for cross-sectional analysis of snakebite epidemiology at national scale. PLoS Negl Trop Dis. (2021) 15:1–19. doi: 10.1371/journal.pntd.0009023
92. Plaza-Rodríguez, C, Alt, K, Grobbel, M, Hammerl, JA, Irrgang, A, Szabo, I, et al. Wildlife as sentinels of antimicrobial resistance in Germany? Front Vet Sci. (2021) 7:627821. doi: 10.3389/fvets.2020.627821
93. Silva, RBS, Franco-Silva, LF, Lima, DA, de Andrade Freitas, ABA, Ramalho, WM, and de Melo, MA. Spatial analysis of canine leishmaniasis in an area of transmission of the semi-arid region of the state of Paraíba. Brazil Rev Brasil Parasitol Vet. (2021) 30:1–10. doi: 10.1590/s1984-296120201089
94. Catapan, DC, Borges, TD, Müller, MO, and Pimpão, CT. Public policies for population management of dogs and cats and social indicators of the Curitiba metropolitan region in Brazil. Acta Vet Brasil. (2019) 13:215–23. doi: 10.21708/avb.2019.13.4.8504
95. Harvey, TV, Heukelbach, J, Assunção, MS, Fernandes, TM, da Rocha, CMBM, and Carlos, RSA. Seasonal variation and persistence of tungiasis infestation in dogs in an endemic community, Bahia state (Brazil): longitudinal study. Parasitol Res. (2019) 118:1711–8. doi: 10.1007/s00436-019-06314-w
96. Innes, GK, Lambrou, AS, Thumrin, P, Thukngamdee, Y, Tangwangvivat, R, Doungngern, P, et al. Enhancing global health security in Thailand: strengths and challenges of initiating a one health approach to avian influenza surveillance. One Health. (2022) 14:100397. doi: 10.1016/j.onehlt.2022.100397
97. Schaufler, K, Nowak, K, Düx, A, Semmler, T, Villa, L, Kourouma, L, et al. Clinically relevant ESBL-producing K. pneumoniae ST307 and E. coli ST38 in an urban west African rat population. Front Microbiol. (2018) 9:150. doi: 10.3389/fmicb.2018.00150
98. Alegría-Morán, R, Pastenes, Á, Cabrera, G, Fredes, F, and Ramírez-Toloza, G. Urban public squares as potential hotspots of dog-human contact: a spatial analysis of zoonotic parasites detection in gran Santiago, Chile. Vet Parasitol Reg Stud Rep. (2021) 24:100579. doi: 10.1016/j.vprsr.2021.100579
99. Martín-Díaz, J, García-Aljaro, C, Pascual-Benito, M, Galofré, B, Blanch, AR, and Lucena, F. Microcosms for evaluating microbial indicator persistence and mobilization in fluvial sediments during rainfall events. Water Res. (2017) 123:623–31. doi: 10.1016/j.watres.2017.07.017
100. Orusa, T, Orusa, R, Viani, A, Carella, E, and Mondino, EB. Geomatics and EO data to support wildlife diseases assessment at landscape level: a pilot experience to map infectious keratoconjunctivitis in chamois and phenological trends in Aosta Valley (NW Italy). Remote Sens. (2020) 12:1–22. doi: 10.3390/rs12213542
101. Rostal, MK, Ross, N, Machalaba, C, Cordel, C, Paweska, JT, and Karesh, WB. Benefits of a one health approach: an example using Rift Valley fever. One Health. (2018) 5:34–6. doi: 10.1016/j.onehlt.2018.01.001
102. Ladbury, GAF, Van Leuken, JPG, Swart, A, Vellema, P, Schimmer, B, Ter Schegget, R, et al. Integrating interdisciplinary methodologies for one health: goat farm re-implicated as the probable source of an urban Q fever outbreak, the Netherlands, 2009. BMC Infect Dis. (2015) 15:372. doi: 10.1186/s12879-015-1083-9
103. Howe, AC, and Soupir, ML. Antimicrobial resistance in integrated agroecosystems: state of the science and future opportunities. J Environ Qual. (2021) 50:1255–65. doi: 10.1002/jeq2.20289
104. Nelson, A, Manandhar, S, Ruzante, J, Gywali, A, Dhakal, B, Dulal, S, et al. Antimicrobial drug resistant non-typhoidal Salmonella enterica in commercial poultry value chain in Chitwan, Nepal. One Health Outlook. (2020) 2:18. doi: 10.1186/s42522-020-00025-4
105. Pearce, N, and Douwes, J. Research at the interface between human and veterinary health. Prev Vet Med. (2013) 111:187–93. doi: 10.1016/j.prevetmed.2013.05.010
106. Melo, AM, Stevens, DA, Tell, LA, Veríssimo, C, Sabino, R, and Xavier, MO. Aspergillosis, avian species and the one health perspective: the possible importance of birds in azole resistance. Microorganisms. (2020) 8:1–22. doi: 10.3390/microorganisms8122037
107. Mahefarisoa, KL, Simon Delso, N, Zaninotto, V, Colin, ME, and Bonmatin, JM. The threat of veterinary medicinal products and biocides on pollinators: a one health perspective. One Health. (2021) 12:100237. doi: 10.1016/j.onehlt.2021.100237
108. Alba, P, Leekitcharoenphon, P, Franco, A, Feltrin, F, Ianzano, A, Caprioli, A, et al. Molecular epidemiology of mcr-encoded colistin resistance in Enterobacteriaceae from food-producing animals in Italy revealed through the EU harmonized antimicrobial resistance monitoring. Front Microbiol. (2018) 9:1217. doi: 10.3389/fmicb.2018.01217
109. Alders, RG, Chadag, MV, Debnath, NC, Howden, M, Meza, F, Schipp, MA, et al. Planetary boundaries and veterinary services. OIE Rev Sci Tech. (2021) 40:439–53. doi: 10.20506/rst.40.2.3236
110. Amato, L, Dente, MG, Calistri, P, and Declich, S, Medi Lab Secure WG. Integrated early warning surveillance: achilles′ heel of one health? Microorganisms. (2020) 8:84. doi: 10.3390/microorganisms8010084
111. Anjum, MF, Schmitt, H, Börjesson, S, Berendonk, TU, Donner, E, Stehling, EG, et al. The potential of using E. coli as an indicator for the surveillance of antimicrobial resistance (AMR) in the environment. Curr Opin Microbiol. (2021) 64:152–8. doi: 10.1016/j.mib.2021.09.011
112. Badul, S, Abia, ALK, Amoako, DG, Perrett, K, Bester, LA, and Essack, SY. From the farms to the dining table: the distribution and molecular characteristics of antibiotic-resistant enterococcus spp. in intensive pig farming in South Africa. Microorganisms. (2021) 9:882. doi: 10.3390/microorganisms9050882
113. Bastos, V, Mota, R, Guimarães, M, Richard, Y, Lima, AL, Casseb, A, et al. Challenges of rabies surveillance in the eastern Amazon: the need of a one health approach to predict rabies spillover. Front Public Health. (2021) 9:624574. doi: 10.3389/fpubh.2021.624574
114. Butcher, A, Cañada, JA, and Sariola, S. How to make noncoherent problems more productive: towards an AMR management plan for low resource livestock sectors. Humanities and social sciences. Communications. (2021) 8:287. doi: 10.1057/s41599-021-00965-w
115. Cardoen, S, De Clercq, K, Vanholme, L, De Winter, P, Thiry, E, and Van Huffei, X. Preparedness activities and research needs in addressing emerging infectious animal and zoonotic diseases. OIE Rev Sci Tech. (2017) 36:557–68. doi: 10.20506/rst.36.2.2674
116. Carvelli, A, Scaramozzino, P, Iacoponi, F, Condoleo, R, and Marta, UD. Size, demography, ownership profiles, and identification rate of the owned dog population in Central Italy. PLoS One. (2020) 15:e0240551. doi: 10.1371/journal.pone.0240551
117. Cilia, G, Fratini, F, Turchi, B, Ebani, VV, Turini, L, Bilei, S, et al. Presence and characterization of zoonotic bacterial pathogens in wild boar hunting dogs (Canis lupus familiaris) in tuscany (Italy). Animals. (2021) 11:1139. doi: 10.3390/ani11041139
118. Compri, M, Mader, R, Mazzolini, E, De-Angelis, G, Mutters, NT, Rajendran, NB, et al. White paper: bridging the gap between surveillance data and antimicrobial stewardship in the animal sector—practical guidance from the JPIAMR ARCH and COMBACTE-MAGNET EPI-net networks. J Antimicrob Chemother. (2020) 75:II52–II66. doi: 10.1093/jac/dkaa429
119. Cuong, NV, Ly, NPC, Van, NTB, Phu, DH, Kiet, BT, Hien, VB, et al. Feasibility study of a field survey to measure antimicrobial usage in humans and animals in the Mekong Delta region of Vietnam. JAC Antimicrob Resist. (2021) 3:dlab107. doi: 10.1093/jacamr/dlab107
120. Denis-Robichaud, J, Aenishaenslin, C, Richard, L, Desmarchelier, M, and Carabin, H. Association between pet ownership and mental health and well-being of Canadians assessed in a cross-sectional study during the COVID-19 pandemic. Int J Environ Res Public Health. (2022) 19:2215. doi: 10.3390/ijerph19042215
121. Duffy, SC, Srinivasan, S, Schilling, MA, Stuber, T, Danchuk, SN, Michael, JS, et al. Reconsidering Mycobacterium bovis as a proxy for zoonotic tuberculosis: a molecular epidemiological surveillance study. Lancet Microb. (2020) 1:e66–73. doi: 10.1016/S2666-5247(20)30038-0
122. Eddy, C, and Sase, E. Part 1: the zika virus threat and prevention challenges: an all-hazards and one health approach to pandemic and global epidemic prevention and mitigation. J Environ Health. (2021) 84:8–18.
123. Elton, L, Haider, N, Kock, R, Thomason, MJ, Tembo, J, Arruda, LB, et al. Zoonotic disease preparedness in sub-Saharan African countries. One Health Outlook. (2021) 3:5. doi: 10.1186/s42522-021-00037-8
124. Fusi, F, Lorenzi, V, Franceschini, G, Compiani, R, Harper, V, Ginestreti, J, et al. Animal welfare and biosecurity assessment: a comparison between Italian and Irish beef cattle rearing systems. Anim Prod Sci. (2020) 61:55–63. doi: 10.1071/AN19611
125. Gomes, S, Fernandes, C, Monteiro, S, Cabecinha, E, Teixeira, A, Varandas, S, et al. The role of aquatic ecosystems (river Tua, Portugal) as reservoirs of multidrug-resistant Aeromonas spp. water (Switzerland). WaterSA. (2021) 13:698. doi: 10.3390/w13050698
126. Gostin, LO, and Katz, R. The international health regulations: the governing framework for Global Health security. Milbank Q. (2016) 94:264–313. doi: 10.1111/1468-0009.12186
127. Guo, B, Huang, J, and Porterfield, SL. Food security and health in transition to adulthood for individuals with disabilities. Disabil Health J. (2020) 13:100937. doi: 10.1016/j.dhjo.2020.100937
128. Häsler, B, Hiby, E, Gilbert, W, Obeyesekere, N, Bennani, H, and Rushton, J. A one health framework for the evaluation of rabies control programmes: a case study from Colombo City, Sri Lanka. PLoS Negl Trop Dis. (2014) 8:e03270. doi: 10.1371/journal.pntd.0003270
129. Hosseini Jebeli, SS, Rezapour, A, Hajebi, A, Moradi-Lakeh, M, and Damari, B. Scaling-up a new socio-mental health service model in Iran to reduce burden of neuropsychiatric disorders: an economic evaluation study. Int J Ment Heal Syst. (2021) 15:47. doi: 10.1186/s13033-021-00468-w
130. Houe, H, Nielsen, SS, Nielsen, LR, Ethelberg, S, and Mølbak, K. Opportunities for improved disease surveillance and control by use of integrated data on animal and human health. Front Vet Sci. (2019) 6:301. doi: 10.3389/fvets.2019.00301
131. Huntington, B, Bernardo, TM, Bondad-Reantaso, M, Bruce, M, Devleesschauwer, B, Gilbert, W, et al. Global burden of animal diseases: a novel approach to understanding and managing disease in livestock and aquaculture. OIE Rev Sci Tech. (2021) 40:567–84. doi: 10.20506/rst.40.2.3246
132. Imanishi, M, Rotstein, DS, Reimschuessel, R, Schwensohn, CA, Woody, DH, Davis, SW, et al. Public veterinary medicine: public health outbreak of salmonella enterica serotype Infantis infection in humans linked to dry dog food in the United States and Canada, 2012. J Am Vet Med Assoc. (2014) 244:545–53. doi: 10.2460/javma.244.5.545
133. Jans, C, Sarno, E, Collineau, L, Meile, L, Stärk, KDC, and Stephan, R. Consumer exposure to antimicrobial resistant bacteria from food at Swiss retail level. Front Microbiol. (2018) 9:362. doi: 10.3389/fmicb.2018.00362
134. Krecek, RC, Rabinowitz, PM, and Conrad, PA. Demystifying and demonstrating the value of a one health approach to parasitological challenges. Vet Parasitol. (2020) 287:109202. doi: 10.1016/j.vetpar.2020.109202
135. Léchenne, M, Traore, A, Hattendorf, J, Kallo, V, Oussiguere, A, Tetchi, M, et al. Increasing rabies data availability: the example of a one health research project in Chad, Côte d’Ivoire and Mali. Acta Trop. (2021) 215:105808. doi: 10.1016/j.actatropica.2020.105808
136. Machalaba, C, Smith, KM, Awada, L, Berry, K, Berthe, F, Bouley, TA, et al. One health economics to confront disease threats. Trans R Soc Trop Med Hyg. (2017) 111:235–7. doi: 10.1093/trstmh/trx039
137. Mantovani, A. Human and veterinary medicine: the priority for public health synergies. Vet Ital. (2008) 44:577–82.
138. Mantovani, A, Aquilina, G, Cubadda, F, and Marcon, F. Risk-benefit assessment of feed additives in the one health perspective. Front Nutr. (2022) 9:843124. doi: 10.3389/fnut.2022.843124
139. Mason, C, and Weber, J. What predictors are associated with the social inclusion of people with disabilities? A comparison of community-based rehabilitation participants to the general population in Vietnam. Disabil Rehabil. (2021) 43:815–22. doi: 10.1080/09638288.2019.1643413
140. McIntyre, KM, Setzkorn, C, Hepworth, PJ, Morand, S, Morse, AP, and Baylis, M. A quantitative prioritisation of human and domestic animal pathogens in Europe. PLoS One. (2014) 9:e0103529. doi: 10.1371/journal.pone.0103529
141. Mirzaei, H, and Damari, B. Establishing calendar for health observatories studies: Islamic Republic of Iran’s experience. Iran Red Crescent Med J. (2020) 22:e100363. doi: 10.32592/ircmj.Crossmark
142. Mo, SS, Urdahl, AM, Madslien, K, Sunde, M, Nesse, LL, Slettemeås, JS, et al. What does the fox say? Monitoring antimicrobial resistance in the environment using wild red foxes as an indicator. PLoS One. (2018) 13:e198019. doi: 10.1371/journal.pone.0198019
143. Moran, D. A framework for improved one health governance and policy making for antimicrobial use. BMJ. Glob Health. (2019) 4:e001807. doi: 10.1136/bmjgh-2019-001807
144. Nguyen, NT, Liu, M, Katayama, H, Takemura, T, and Kasuga, I. Association of the colistin resistance gene mcr-1 with faecal pollution in water environments in Hanoi. Vietnam Lett Appl Microbiol. (2021) 72:275–82. doi: 10.1111/lam.13421
145. Nieto-Claudin, A, Deem, SL, Rodríguez, C, Cano, S, Moity, N, Cabrera, F, et al. Antimicrobial resistance in Galapagos tortoises as an indicator of the growing human footprint. Environ Pollut. (2021) 284:117453. doi: 10.1016/j.envpol.2021.117453
146. Nwafor, CD, Ilori, E, Olayinka, A, Ochu, C, Olorundare, R, Edeh, E, et al. The one health approach to incident management of the 2019 Lassa fever outbreak response in Nigeria. One Health. (2021) 13:100346. doi: 10.1016/j.onehlt.2021.100346
147. Ortmeyer, HK, and Katzel, LI. Effects of proximity between companion dogs and their caregivers on heart rate variability measures in older adults: a pilot study. Int J Environ Res Public Health. (2020) 17:2674. doi: 10.3390/ijerph17082674
148. Purseid, BV, Darshan, N, Kasabi, GS, Gerard, F, Samrat, A, George, C, et al. Predicting disease risk areas through co-production of spatial models: the example of kyasanur forest disease in india’s forest landscapes. PLoS Negl Trop Dis. (2020) 14:1–20. doi: 10.1371/journal.pntd.0008179
149. Ramsamy, Y, Mlisana, KP, Amoako, DG, Abia, ALK, Allam, M, Ismail, A, et al. Comparative pathogenomics of aeromonas veronii from pigs in South Africa: dominance of the novel st 657 clone. Microorganisms. (2020) 8:1–16. doi: 10.3390/microorganisms8122008
150. Rebelo, A, Mourão, J, Freitas, AR, Duarte, B, Silveira, E, Sanchez-Valenzuela, A, et al. Diversity of metal and antibiotic resistance genes in Enterococcus spp. from the last century reflects multiple pollution and genetic exchange among phyla from overlapping ecosystems. Sci Total Environ. (2021) 787:147548. doi: 10.1016/j.scitotenv.2021.147548
151. Rocha, ADDL, Ferrari, RG, Pereira, WE, Lima, LAD, Givisiez, PEN, Moreno-Switt, AI, et al. Revisiting the biological behavior of Salmonella enterica in hydric resources: a Meta-analysis study addressing the critical role of environmental water on food safety and public health. Front Microbiol. (2022) 13:802625. doi: 10.3389/fmicb.2022.802625
152. Rousham, E, Unicomb, L, Wood, P, Smith, M, Asaduzzaman, M, and Islam, MA. Spatial and temporal variation in the community prevalence of antibiotic resistance in Bangladesh: an integrated surveillance study protocol. BMJ Open. (2018) 8:e023158. doi: 10.1136/bmjopen-2018-023158
153. Rudy, JE, Khan, Y, Bower, JK, Patel, S, and Foraker, RE. Cardiovascular health trends in electronic health record data (2012–2015): a cross-sectional analysis of the guideline advantage™. eGEMs. (2019) 7:30. doi: 10.5334/egems.268
154. Scoppetta, F, Chiovoloni, M, Spernanzoni, G, Filippini, G, and Capuccella, M. Pharmaco-epidemiological evaluation of veterinary antimicrobial prescriptions for cattle, swine, small ruminants, poultry, rainbow trout, and food-producing horses in Umbria in 2014. Vet Ital. (2018) 54:305–15. doi: 10.12834/VetIt.1174.6524.2
155. Sinclair, JR. Importance of a one health approach in advancing global health security and the sustainable development goals. Rev Sci Tech. (2019) 38:145–54. doi: 10.20506/rst.38.1.2949
156. Smoglica, C, Vergara, A, Angelucci, S, Festino, AR, Antonucci, A, Marsilio, F, et al. Evidence of linezolid resistance and virulence factors in Enterococcus spp. Antibiotics. (2022) 11:223. doi: 10.3390/antibiotics11020223
157. Sweileh, WM. Global research activity on antimicrobial resistance in food-producing animals. Arch Public Health. (2021) 79:49. doi: 10.1186/s13690-021-00572-w
158. Taruscio, D, Bermejo-Sánchez, E, Salerno, P, and Mantovani, A. Primary prevention as an essential factor ensuring sustainability of health systems: the example of congenital anomalies. Ann Istitut Super Sanita. (2019) 55:258–64. doi: 10.4415/ANN_19_03_11
159. Tekola, B, Myers, L, Lubroth, J, Plee, L, Calistri, P, and Pinto, J. International health threats and global early warning and response mechanisms. OIE Rev Sci Tech. (2017) 36:657–70. doi: 10.20506/rst.36.2.2683
160. Valadez-Noriega, M, Estévez-Moreno, LX, Rayas-Amor, AA, Rubio-Lozano, MS, Galindo, F, and Miranda-de la Lama, GC. Livestock hauliers’ attitudes, knowledge and current practices towards animal welfare, occupational wellbeing and transport risk factors: a Mexican survey. Prev Vet Med. (2018) 160:76–84. doi: 10.1016/j.prevetmed.2018.09.023
161. Zrnčić, S. European union’s action plan on antimicrobial resistance and implications for trading partners with example of national action plan for Croatia. Asian Fish Sci. (2020) 33:75–82. doi: 10.33997/j.afs.2020.33.S1.011
162. Arora, NK. Agricultural sustainability and food security. Environ Sustain. (2018) 1:217–9. doi: 10.1007/s42398-018-00032-2
163. Silva, RBS, Franco-Silva, LF, and Lima, DA. Spatial analysis of canine leishmaniasis in an area of transmission of the semi-arid region of the state of Paraíba. Brazil Rev Bras Parasitol Vet. (2021) 30:e018620. doi: 10.1590/s1984-296120201089,Freitas ABA de A, Ramalho WM, Melo MA de
164. Plowright, RK, Parrish, CR, McCallum, H, Hudson, PJ, Ko, AI, Graham, AL, et al. Pathways to zoonotic spillover. Nat Rev Microbiol. (2017) 15:502–10. doi: 10.1038/nrmicro.2017.45
165. Kamaruzzaman, EA, Abdul Aziz, S, Bitrus, AA, Zakaria, Z, and Hassan, L. Occurrence and characteristics of extended-Spectrum β-lactamase-producing Escherichia coli from dairy cattle, milk, and farm environments in peninsular Malaysia. Pathogens. (2020) 9:1007. doi: 10.3390/pathogens9121007
166. Saurabh Sonwani, SS, and Vandana Maurya, VM. Impact of air pollution on the environment and economy In: P Saxena and V Naik, editors. Air Pollution: Sources, Impacts and Controls. UK: CAB International (2019). 113–34.
167. Keesing, F, and Ostfeld, RS. Impacts of biodiversity and biodiversity loss on zoonotic diseases. Proc Natl Acad Sci U S A. (2021) 118:e2023540118. doi: 10.1073/pnas.2023540118
168. Githeko, AK, Lindsay, SW, Confalonieri, UE, and Patz, JA. Climate change and vector-borne diseases: a regional analysis. Bull World Health Organ. (2000) 78:1136–47.
169. Naicker, PR. The impact of climate change and other factors on zoonotic diseases. Arch Clin Microbiol. (2011) 2:4. doi: 10.3823/226
170. Brownstein, JS, Holford, TR, and Fish, D. Effect of climate change on Lyme disease risk in North America. Ecosyst Health. (2005) 2:38–46. doi: 10.1007/s10393-004-0139-x
171. Evander, M, and Ahlm, C. Milder winters in northern Scandinavia may contribute to larger outbreaks of haemorrhagic fever virus. Glob Health Action. (2009) 2:2020. doi: 10.3402/gha.v2i0.2020
172. Cecchi, G, Munafò, M, Baiocco, F, Andreani, P, and Mancini, L. Estimating river pollution from diffuse sources in the Viterbo province using the potential non-point pollution index. Ann Istitut Super Sanita. (2007) 43:295–301.
173. Meentemeyer, RK, Haas, SE, and Václavík, T. Landscape epidemiology of emerging infectious diseases in natural and human-altered ecosystems. Annu Rev Phytopathol. (2012) 50:379–402. doi: 10.1146/annurev-phyto-081211-172938
174. Lin, C-H, and Wen, T-H. How spatial epidemiology helps understand infectious human disease transmission. Trop Med. (2022) 7:164. doi: 10.3390/tropicalmed7080164
175. Neiderud, C-J. How urbanization affects the epidemiology of emerging infectious diseases. Infect Ecol Epidemiol. (2015) 5:27060. doi: 10.3402/iee.v5.27060
176. Yu, D, Li, X, Yu, J, Shi, X, Liu, P, and Tian, P. Whether urbanization has intensified the spread of infectious diseases—renewed question by the COVID-19 pandemic. Front Public Health. (2021) 9:699710. doi: 10.3389/fpubh.2021.699710
177. Ahmed, S, Dávila, JD, Allen, A, Haklay, M, Tacoli, C, and Fèvre, EM. Does urbanization make emergence of zoonosis more likely? Evidence, myths and gaps. Environ Urban. (2019) 31:443–60. doi: 10.1177/0956247819866124
178. Coutts, C, and Hahn, M. Green infrastructure, ecosystem services, and human health. IJERPH. (2015) 12:9768–98. doi: 10.3390/ijerph120809768
179. Chandrasegaran, K, Lahondère, C, Escobar, LE, and Vinauger, C. Linking mosquito ecology, traits, behavior, and disease transmission. Trends Parasitol. (2020) 36:393–403. doi: 10.1016/j.pt.2020.02.001
Keywords: geographic information system, multi-criteria decision-making, green infrastructure, indicators, one health, systematic literature review
Citation: Jato-Espino D, Mayor-Vitoria F, Moscardó V, Capra-Ribeiro F and Bartolomé del Pino LE (2023) Toward One Health: a spatial indicator system to model the facilitation of the spread of zoonotic diseases. Front. Public Health. 11:1215574. doi: 10.3389/fpubh.2023.1215574
Received: 04 May 2023; Accepted: 14 June 2023;
Published: 29 June 2023.
Edited by:
Juarez Antonio Simões Quaresma, Federal University of Pará, BrazilReviewed by:
Taif Shah, Kunming University of Science and Technology, ChinaCopyright © 2023 Jato-Espino, Mayor-Vitoria, Moscardó, Capra-Ribeiro and Bartolomé del Pino. This is an open-access article distributed under the terms of the Creative Commons Attribution License (CC BY). The use, distribution or reproduction in other forums is permitted, provided the original author(s) and the copyright owner(s) are credited and that the original publication in this journal is cited, in accordance with accepted academic practice. No use, distribution or reproduction is permitted which does not comply with these terms.
*Correspondence: Daniel Jato-Espino, ZGphdG9AdW5pdmVyc2lkYWR2aXUuY29t
Disclaimer: All claims expressed in this article are solely those of the authors and do not necessarily represent those of their affiliated organizations, or those of the publisher, the editors and the reviewers. Any product that may be evaluated in this article or claim that may be made by its manufacturer is not guaranteed or endorsed by the publisher.
Research integrity at Frontiers
Learn more about the work of our research integrity team to safeguard the quality of each article we publish.