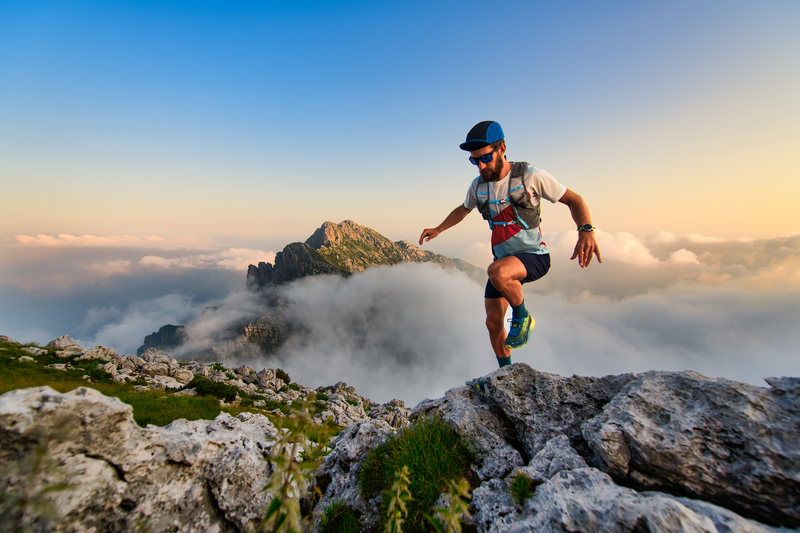
95% of researchers rate our articles as excellent or good
Learn more about the work of our research integrity team to safeguard the quality of each article we publish.
Find out more
ORIGINAL RESEARCH article
Front. Public Health , 20 September 2023
Sec. Public Health and Nutrition
Volume 11 - 2023 | https://doi.org/10.3389/fpubh.2023.1213926
This article is part of the Research Topic Nutrition in the Prevention and Treatment of Cardiovascular Diseases View all 11 articles
Introduction: Mexico ranks second in the global prevalence of obesity in the adult population, which increases the probability of developing dyslipidemia. Dyslipidemia is closely related to cardiovascular diseases, which are the leading cause of death in the country. Therefore, developing tools that facilitate the prediction of dyslipidemias is essential for prevention and early treatment.
Methods: In this study, we utilized a dataset from a Mexico City cohort consisting of 2,621 participants, men and women aged between 20 and 50 years, with and without some type of dyslipidemia. Our primary objective was to identify potential factors associated with different types of dyslipidemia in both men and women. Machine learning algorithms were employed to achieve this goal. To facilitate feature selection, we applied the Variable Importance Measures (VIM) of Random Forest (RF), XGBoost, and Gradient Boosting Machine (GBM). Additionally, to address class imbalance, we employed Synthetic Minority Over-sampling Technique (SMOTE) for dataset resampling. The dataset encompassed anthropometric measurements, biochemical tests, dietary intake, family health history, and other health parameters, including smoking habits, alcohol consumption, quality of sleep, and physical activity.
Results: Our results revealed that the VIM algorithm of RF yielded the most optimal subset of attributes, closely followed by GBM, achieving a balanced accuracy of up to 80%. The selection of the best subset of attributes was based on the comparative performance of classifiers, evaluated through balanced accuracy, sensitivity, and specificity metrics.
Discussion: The top five features contributing to an increased risk of various types of dyslipidemia were identified through the machine learning technique. These features include body mass index, elevated uric acid levels, age, sleep disorders, and anxiety. The findings of this study shed light on significant factors that play a role in dyslipidemia development, aiding in the early identification, prevention, and treatment of this condition.
Dyslipidemia is a metabolic alteration characterized by elevated levels of cholesterol, triglycerides (TGs), and Low-Density Lipoprotein Cholesterol (LDL), as well as a decrease in High-Density Lipoprotein Cholesterol (HDL) levels. Worldwide, dyslipidemia presents as an exponential health problem with severe consequences and is considered one of the main risk factors for ischemic heart disease, cardiovascular disease, stroke, coronary heart disease, and type 2 diabetes mellitus (T2DM), which is the principal cause of death in adults in Mexico (1, 2). Pirillo et al. (3) have pointed out that ischemic heart disease reached a total of 3.78 million deaths in 2019, with high plasma LDL being the principal cause. These authors also reported between 0.61 and 2.73 million deaths due to ischemic stroke, a strongly associated condition. Similarly, there is a high variation in the number of deaths between countries, presumably due to regional differences and types of dyslipidemia. According to the same authors Pirillo et al. (3), low plasma HDL levels have been the most common type of dyslipidemia in Latin America since 2005, followed by hypertriglyceridemia and high plasma LDL levels.
According to the National Cholesterol Education Program Adult Treatment Panel III (ATP III) criteria, the classification of lipid profile dyslipidemias includes four types (see Table 1). Hypertriglyceridemia is a common lipid abnormality characterized by elevated triglyceride (TG) levels, often affecting individuals with visceral obesity, metabolic syndrome, and type 2 diabetes mellitus (T2DM) (4, 5). On the other hand, hypercholesterolemia is associated with high levels of LDL or CHOL and may also be present in individuals with a genetic disorder leading to elevated cholesterol levels (6). Hypoalphalipoproteinemia is frequently observed in people with coronary artery disease and is characterized by low levels of plasma high-density lipoproteins (HDL) (7). Finally, mixed hyperlipidemia, a genetic disorder involving higher cholesterol and triglyceride levels, contributes to the development of coronary artery disease.
In general terms, there are potential risk factors such as increased body mass index (BMI), an excessive dietary intake of saturated fat, and a sedentary lifestyle that contribute to developing a given type of dyslipidemia, a highly complex and heterogeneous set of conditions. This fact complicates the prognosis and diagnostics. In this regard, the widespread use of machine learning (ML) has allowed the application of computational intelligence tools as diagnostic tools for medical issues based on data acquired from analyzed patients. Therefore, such ML models (trained by medical guidance) have been successful in helping doctors to determine medical conditions with improved accuracy in a timely manner (8).
A study proposed by Cui et al. (9) uses ML to predict the risk of dyslipidemia in steelworkers by studying a set of standardized outcomes. They acquired the data by surveying anthropometric data, habits, personal status, and working details. Finally, they apply a Recurrent Neural Network (RNN) and Long Short-Term Memory (LSTM) algorithm, showing excellent performance in predicting dyslipidemia in steel and iron industry employees.
Machine learning has emerged as a valuable tool in predicting dyslipidemia and related conditions based on patient data. For instance, Cui et al. (9) used a recurrent neural network and LSTM algorithm to predict dyslipidemia in steelworkers, achieving excellent accuracy. Lee et al. (10) correlated facial characteristics with hypertriglyceridemia using Naive Bayes classifiers, while Pina et al. (11) showed that a neural network outperformed the Dutch lipid score in predicting dyslipidemia in specialized lipid clinics.
Hatmal et al. (12) used ten ML techniques to predict dyslipidemia with an accuracy of 0.75, considering CD36 protein levels, lipid profile, blood sugar, gender, and age. Similarly, Kim et al. (13) classified and predicted overweight/obesity, dyslipidemia, hypertension, and T2DM using a deep neural network model based on nutritional intake data from Korean citizens. For each disease risk, the accuracies achieved were 0.62496, 0.58654, 0.79958, and 0.80896, respectively.
Dyslipidemia is a complex and heterogeneous condition with potential risk factors such as increased BMI, excessive dietary intake of saturated fat, and a sedentary lifestyle. In this context, machine learning models trained on medical data have shown promising results in improving the diagnosis and prognosis of this condition.
However, recent research indicates that the impact of dyslipidemia on cardiovascular health can vary between men and women due to hormonal, genetic, and lifestyle differences. By analyzing gender-specific differences in dyslipidemia, we can identify unique risk profiles, treatment responses, and underlying mechanisms that may contribute to cardiovascular outcomes. Tailoring interventions based on gender-specific dyslipidemia patterns can lead to more targeted and effective therapies, ultimately improving cardiovascular health for both men and women. This approach also highlights the importance of recognizing and addressing gender-related disparities in dyslipidemia management to optimize patient outcomes and reduce the burden of cardiovascular diseases.
Historically, clinical trials are predominantly done in men, excluding women, even in studies with cells and mice (only male). A review studies the significant causes of diseases by bias in sex and gender. The authors express the influence of differences between sex and gender in genetics, implying affection in diagnosing and treating illnesses (14).
In this context, the present work provides a machine-learning approach to characterize the particularities of men and women with a given type of dyslipidemia (hypertriglyceridemia, hypercholesterolemia, hypoalphalipoproteinemia, as well as mixed hyperlipidemias), identifying the association with clinical factors, biochemical screening, family health history, dietary information, and additional risk factors in order to provide features that can be monitored by health authorities to decrease the risk of long-term complications caused by lipid abnormalities in the study population. While dyslipidemia is a significant risk factor for serious diseases, we acknowledge that our analysis does not incorporate a specific time frame within which an individual might develop the disease. Instead, our study aims to elucidate the underlying risk factors associated with dyslipidemia, providing valuable insights into its etiology and contributing factors. The criteria used in this study to classify dyslipidemia types are shown in Table 1.
The present study investigates the cross-sectional association between various factors and cardiovascular health outcomes utilizing data collected from the baseline assessment of the Tlalpan 2020 cohort (15), a longitudinal research project conducted by the National Institute of Cardiology (Instituto Nacional de Cardiolog-a-Ignacio Chvez) in Mexico City.
The dataset used in this study consists of 2,621 participant records and 137 variables related to anthropometric measurements, clinical parameters, biochemical tests, family health history, physical activity, sleep disorders, smoking habits, alcohol consumption, psychological stress levels, and dietary information. The study identified four types of lipid disorders: 696 cases of hypertriglyceridemia (HTG), 402 cases of hypercholesterolemia (HPLC), 608 cases of hypoalphalipoproteinemia (HPLF), and 548 cases of mixed hyperlipidemia (MIX). Regarding data collection, it was carried out as follows:
• The anthropometric measurements, such as weight, height, and waist circumference (WC), were measured following the International Society for the Advancement of Kinanthropometry (16); the clinical parameters systolic (SBP) and diastolic blood pressure (DBP) were calculated considering three measures of each one, with a duration of the 3-min gap.
• In the case of the biochemical tests, the blood samples: fasting plasma glucose (FPG), TGs, HDL, LDL, CHOL, uric acid (URIC), and atherogenic index of plasma (AIP) were taken after 12 h of overnight fasting.
• The variables of family health history considered diseases the mother and father suffered, such as diabetes, obesity, hypertension, dyslipidemia, and heart attack.
• The physical activity was classified based on International Physical Activity Questionnaire (17) by METs (metabolic equivalents)-minutes/week into three categories low, moderate, and high.
• We used the Medical Outcomes Study-Sleep 12-item scale to determine sleep disorders (18, 19).
• Alcohol consumption was estimated by considering if the participant is a current drinker, the frequency, and the number of cups or beers consumed.
• To classify smoking practice, we consider if the participant is a current smoker, an ex-smoker, or if he/she has never smoked. Supplementary Tables 1–3—presents the variables mentioned in this section.
• Regarding dietary information we applied a software tool called Evaluation of Nutritional Habits and Nutrient Consumption System (20). This system analyzes the meals consumed by the participant during a day in the last year and calculates the amount of nutrients consumed. The variables corresponding to the Evaluation of Nutritional Habits and Nutrient Consumption System are shown in Supplementary Tables 1, 2.
This work utilized several statistical and data analytics methods. Figure 1 presents the general workflow of the model and describes the methodology used to classify participants with a given type of dyslipidemia and identify the risk factors. Dyslipidemia types were classified according to the ATP III criteria. The dataset was divided into two-thirds for training and the rest for testing. We must note that we applied the SMOTE technique to balance the class distribution in the training dataset.
To find the best subset of variables contributing to improving model performance, we used four methods for feature selection: VIM of RF, XGBoost, RPART, and SHAP. For this study, we applied RF to predict the type of dyslipidemia due to its high performance in diagnosing or predicting dyslipidemia and related diseases (21–23). We developed and evaluated RF performance by running 30 executions using different seeds for each one. To measure the effectiveness of the model, we utilized sensitivity (SENS), specificity (SPC), and balanced accuracy (B.ACC), metrics that have been used for imbalanced data learning assessment. Finally, we obtained the best-performing predictive model.
The dataset is divided by individuals distinguished by sex (male or female). To justify this division, we perform the correlation matrix with the characteristic variables in addition to the classifications. Figure 2A shows the correlation matrix for women, and Figure 2B shows it for men. The color variation for the correlation is not evident, which is why the subtraction of both is obtained; the result is shown in Figure 2C, where it is evident that there are characteristics that are more related to one gender than to another, in addition to the importance of the difference in the classification of the diagnosis.
Random Forest, developed by Breiman et al. (24), is an ensemble machine learning algorithm composed of multiple tree-based estimators for solving classification and regression problems. To reduce over-fitting and improve predictions, this algorithm builds multiple tree-based estimators from training data samples using the Gini index. The Gini index measures the purity of the nodes and can be computed using the following equation:
where c is the number of classes and p(i) is the proportion of samples that belong to class c.
In addition, this algorithm can be used for feature selection by calculating the importance score of variables using the permutation feature importance method.
Extreme Gradient Boosting (XGBoost), presented by Chen and Guestrin (25), is a high-performance ensemble machine learning algorithm that calculates the variable importance by providing a score for each feature.
GBM is an ensemble model introduced by Friedman et al. (26) that follows the principle of gradient boosting. It consists of a set of individual decision trees, called weak learners, that are trained sequentially to minimize the loss function of the simple models. This model can be computed using the following equation:
where yi and xi are weak learners, with i∈(1, ..., n) and i∈ℤ+. The constant v (shrinkage factor) is used to control the learning rate, and hm(xi) comes from a decision tree. GBM tries to fit hm(x) by minimizing the loss function:
To evaluate the performance of models and the different subsets of features, we used the following performance metrics: balanced accuracy (B.ACC), sensitivity (SENS), and specificity (SPC)
Where P = Positive, N = Negative, TP = True Positive, FN = False Negative, TN = True Negative, and FP = False Positive, respectively.
We used a 32 GB RAM, 3.50 GHz, Intel Xeon® Dell® Workstation to perform all calculations. R v. 3.6.1 with RStudio and Python v. 3.10.7 were used as programming languages. Purposely, these resources are readily available for implementation in most hospital informatics settings.
The problem of abnormal TG levels can develop based on different factors influencing individuals depending on their lifestyle. Moreover, LDL levels tend to be higher in men than in women until menopause. Hence, in this study, we initially separated the data by gender to obtain the most crucial care features according to the type of dyslipidemia. To identify the potential features by gender and type of dyslipidemia, we applied SMOTE as a resampling method due to class imbalance and three machine learning algorithms, namely VIM of RF, XGBoost, and GBM.
Once we obtained the results from the aforementioned algorithms, we considered displaying at least the top ten most important variables (ranked) that influence each type of dyslipidemia. Each result table shows a different subset of features for each gender and type of dyslipidemia by applying VIM of RF, XGBoost, and GBM.
The results obtained for hypertriglyceridemia are presented in Table 2, followed by the results for hypercholesterolemia in Table 3, as well as the essential variables for hypoalphalipoproteinemia, displayed in Table 4, and finally, the results for mixed hyperlipidemias in Table 5. Summarized general data from the total cohort is presented in Supplementary Table 4.
Subsequently, each algorithm generated subsets of variables, which were used to select the best features. To perform this feature selection process, we applied RF, which was optimized by grid search method (27) (resulting in varying mtry and ntree values for each gender and dyslipidemia type). We employed 10-fold cross-validation with ten repeats to evaluate the performance. Following this, we conducted 30 independent executions with different seeds to ensure robustness and approximate a normal distribution. This approach aligns with similar practices observed in relevant studies (28, 29). The evaluation was based on balanced accuracy, serving as the primary criterion for assessment.
To measure the performance of the RF model, the metrics B.ACC, SENS, and SPC were considered; likewise, it was necessary to apply SMOTE due to the unbalanced dataset. Table 6 shows each result of RF by using the different subset of variables obtained by VIM of RF, XGBoost, RPART, and SHAP, for men and women, as well as the respective parameter tuning and standard deviation (SD).
In the case of men with hypertriglyceridemia, the subset of features obtained by XGBoost achieved the best RF performance with a B.ACC of 82.77% and SD of 1.26. The top variables of this subset showed the influence of overweight, where the first three variables are related to it and body mass index (BMI), followed by age, sleep disturbance (SLPD4) and FYI (SLPSNR1), anxiety as a trait (TR.ANX), smoking practice (SMOKE), somnolence (SLP3), alcohol consumption (ALCOHOL), soy oil consumption (SOYAOIL), glucose levels (FPG), and medical history of the mother with hypertension (M.HPT).
Moreover, for women, the subset of variables obtained by VIM of RF achieved the best performance with a B.ACC of 82.50 and an SD of 1.08, where the principal variable was uric acid levels (URIC) [several studies (30, 31) have found an association between high uric acid and hypertriglyceridemia]. The other variables in this subset include glucose levels (FPG), body mass index (BMI), Systolic blood pressure (SBP), weight, age, Diastolic blood pressure (DBP), Waist circumference (WAIST), sleep disturbance (SLPD4), somnolence (SLP3), height, snoring (SLPSNR1), and smoking practice (SMOKE). All these variables are considered risk factors contributing to the development of hypertriglyceridemia (32, 33).
For hypercholesterolemia, the variables obtained by GBM achieved the best RF performance for men, with a B.ACC of 83.69% and an SD of 1.52. The principal variables found by this model denote a close relation between being overweight as represented by (WEIGHT, WC, AGE, and HEIGHT), sleep disturbances (SLPSNR1, SLP3, and SLPSOB1), anxiety disorders (TR.ANX), and habits such as consumption of flavored soda (FLAVSODA) and smoking (SMOKE).
In the case of women with hypercholesterolemia, the best performance was obtained by the subset generated by VIM or RF with a B.ACC of 79.74% and SD of 1.16, where the anxiety disorders (TR.ANX) and uric acid levels (URIC) were the principal variables, as well as frequently consuming some foods like chicken liver (LIVERSTK), bread (WHBREADSL), oatmeal bowl (OATMEAL), and margarine (MARGARIN), likewise, variables related to sleep disorders like the time to fall asleep (TIM.SLP) and sleep short duration (SLPOP1), followed by low physical activity (METS.low), smoking and history of obese parents (P.OBS).
For men with hypoalphalipoproteinemia, the best subset of variables was presented by GBM with a B.ACC of 80.50% and SD of 1,29, being variables related to overweight the best qualified (WEIGHT, WC, and HEIGHT), as well as age, followed by indicators of sleep disorders like sleep disturbance (SLPD4), snoring (SLPSNR1) and somnolence (SLP3). Likewise, habits of alcohol consumption and smoking, anxiety disorder, cream cheese consumption (CRMCHSPOO) and elevated uric acid levels (URIC).
Similarly, the VIM of RF was the best subset of variables for women with hypoalphalipoproteinemia, with a B.ACC of 83.65% and SD of 1.22. In this case, the principal variable was elevated uric acid levels, followed by snoring (SLPSNR1) and variables closely related to overweight (BMI, WC, WEIGHT), as well as glucose levels (FPG) and blood pressure levels (SBP and DBP) denoted their presence as risk factors, finishing with the consumption of alcohol and bread (WHBREADSL), as well as anxiety.
Finally, the subset of variables obtained by VIM of RF got the best performance for men with mixed hyperlipidemia. In this case, the main variables were closely related to food consumption such as atole without milk (OATMEAL1), oatmeal bowl (OATMEAL), a teaspoon of margarine (MARGARIN), a glass of table wine (TABLEWIN), safflower oil (SAFFLOWR), rum, brandy or tequila (HARDLQUR), zapote (FREQ025), a tablespoon of cream cheese (FREQ005) and olive oil (OLIVEOIL), as well as, ALCOHOL, BMI, history of a parent with dyslipidaemia (P.DSLP), and age.
For women with mixed hyperlipidemia, the variables obtained by VIM of RF with the best-ranked factors were BMI, age, and snoring, followed by glucose levels, waist circumference, sleep short duration, uric acid levels, smoking, height, anxiety, alcohol consumption, Systolic Blood Pressure, and a glass of flavored sugar water (SUGRDRNK).
In what follows, we will discuss the present analysis's expected and novel findings to contextualize the potential value of public health interventions.
In order to determine the significance of studying males and females separately, a significance analysis was conducted using the chi-squared test. The results indicated a strong relationship between gender and the prediction of dyslipidemia types and their critical factors.
The significant associations found for SEX in all dyslipidemias type further emphasize the importance of gender as a significant factor influencing dyslipidemia prediction. Therefore, conducting separate analyses for males and females was crucial to gain a comprehensive understanding of the underlying factors associated with dyslipidemia in each gender group. The results of this significance analysis can be seen in the Supplementary Tables 5–8.
In the case of men with hypertriglyceridemia, several known associations arise. That is the case of overweight (34–37), age (38, 39), and waist circumference (40, 41). Additionally, we discovered a set of relatively new yet significant predictors whose relevance and mechanisms concerning hypertriglyceridemia in men are still to be determined, such as anxiety, tomato sauce consumption, and history of hypertension in the mother. Regarding the association between anxiety and hypertriglyceridemia, van Reedt Dortland and collaborators have identified a potential role of tricyclic antidepressant drugs (42). In contrast, other authors have identified an increased risk of hypertriglyceridemia in patients with psychiatric diseases without relation to specific pharmacological treatment (43).
The case of tomato sauce consumption presents some contradictory features. At the same time, some authors have described a protective role of processed tomato products to post-prandial oxidation and inflammation (both associated with dyslipidemias) in healthy weight subjects (44–46). In contrast, others have related processed foods (including tomato sauce) to hypertriglyceridemia (47, 48).
Since these studies differ in the methods and types of populations under investigation, differences may be explained by such disparate approaches. Hence definite associations need to be further studied with properly defined research methods.
No previous studies have directly linked maternal hypertension history to hypertriglyceridemia. Interestingly however, is the fact that there is an unusual prevalence of hypertriglyceridemia in small populations with known risk factors for pregnancy-associated high blood pressure (49–52), though, at this stage, an actual association is still to be further validated in more extensive population studies.
Similarly, in the case of women with hypertriglyceridemia, the best predictors were some known factors such as AIP (a prominent feature by construction) as well as BMI, age, and cola drink consumption. Other metabolic features appear, such as glucose and uric acid levels and also raw tomato consumption. Regarding the role of high fasting glucose levels in the presence of hypertriglyceridemia, reports have long been made, particularly by driving mechanisms of endogenous hypertriglyceridemia (35, 53, 54). The fact that FPG is a better predictor for hypertriglyceridemia in women than in men may be related to the effects of hormone (in particular, estrogen) metabolism in lipid and glucose processing biochemical pathways (55–57).
Elevated uric acid levels have been previously associated with hypertriglyceridemia, both in extensive cohort studies (31, 52, 58–60), population-based research (61–64), and biochemically-based analyses (65–69). Unlike processed tomato products, whose effects on hypertriglyceridemia are ambiguous (as previously discussed), raw tomato consumption has been acknowledged as a protective factor (44) against dyslipidemia in general and hypertriglyceridemia, in particular, (70–73).
Regarding men with hypercholesterolemia, some of the main predictors are (unsurprisingly) meat-based products with high lipid contents such as tacos al pastor (shepherd style), carnitas, and longaniza (74–76). There is evidence that consuming fatty meats, such as beef, pork, and lamb, may contribute to the development of hypercholesterolemia.
For instance, one study published in the American Journal of Clinical Nutrition found that a diet high in saturated fat, such as that found in fatty meats, was associated with an increase in LDL cholesterol that can, in turn, contribute to the development of cardiovascular disease (77). Another study published in the American Journal of Epidemiology found that individuals who consumed a diet high in red and processed meats had a higher risk of developing hypercholesterolemia than those who consumed a diet low in these types (78).
Aside from fatty meat products, other predictors are foods such as chocolate powder, cream cheese and anthropometrics such as weight and height (79, 80). Some evidence, for instance, suggests that chocolate consumption may be associated with a modest reduction in cholesterol levels, although the effect may be small and may depend on the type of chocolate and the individual.
Several studies published in the American Journal of Clinical Nutrition and the European Journal of Clinical Nutrition found that cocoa and chocolate intake was associated with a slight reduction in total cholesterol and low-density lipoprotein (LDL) cholesterol and that the effect of chocolate on cholesterol levels may be influenced by the type of chocolate consumed, with some studies suggesting that dark chocolate may have a more significant effect on cholesterol levels than milk chocolate (81–83). In contrast, another study recalls that these effects may come via activating flavonoid metabolism and anti-oxidant pathways (84).
In the case of women with hypercholesterolemia, there are well-known factors such as age (85, 86), pork rind (87, 88), mayonnaise consumption (89, 90), and BMI (91, 92). Other less-known predictors emerge from our study. Such is the case of sleep disturbance. Abnormal sleep conditions are gradually being recognized as relevant players in metabolic and cardiovascular diseases (93–95). However, it is noteworthy that most studies relating hypercholesterolemia with sleep disturbances center on the possible effects on sleep induced by drugs such as Pravastatin and Lovastatin (96–100).
The main predictors found for alphalipoproteinemia in men were waist circumference and BMI (101, 102), as well as conditions such as anxiety (103, 104), and consumption of seafood (105, 106) and plums (107). In contrast, in women, selected features were known metabolic state and anthropometric markers such as AIP (108), glucose levels (109, 110), BMI and waist circumference (101, 102), also uric acid levels (111, 112); consumption of high fat or high caloric foods like pork meat, flavored soda, Oaxaca cheese, and bacon (113). Interestingly snoring while sleeping was also a relevant predictor for alphalipoproteinemia in women. Though a direct association of snoring with female alphalipoproteinemia has not been reported, a population-based study has indeed associated self-reported snoring with dyslipidemia, high total cholesterol, and high low-density lipoprotein cholesterol in obese individuals in rural China (114).
Mixed hyperlipidemias in men were best predicted by: AIP (115, 116), waist circumference, BMI (117, 118), age (119), as well as dried chile peppers consumption (DRYCHILES) (120–122), as well as drinking whole milk (MILKGLASS) (123, 124), alcohol (125–127), sweet bread (SWEETBRD) (128, 129), and orange (ORANGE) intake (130–132). In the case of women with mixed hyperlipidemias top predictive features were: BMI (117, 118), age (119), snoring (114), glucose levels (133, 134), uric acid levels (64), smoking (133), and anxiety (135), but also alcohol (125, 136) and flavored sugar water (BACONSLC) (137, 138) consumption.
By focusing on identifying risk factors without a time frame, our study lays the foundation for future investigations that could incorporate temporal aspects for predicting the onset of dyslipidemia or subsequent development of CVD. The findings from our research can serve as a basis for developing predictive models that integrate time-based parameters, enabling more accurate and clinically relevant disease prognosis and management.
In this work, the application of machine learning models in a cohort of Mexico City allowed the identification of subsets of attributes acting as risk factors associated with several types of dyslipidemias. Multi-feature diagnostics, i.e., the diagnosis based on different aspects, is considered essential to support healthcare providers as it allows early detection of patients at the most significant risk of developing a type of dyslipidemia, which supports the development of strategies for prevention, treatment, and prognosis the condition.
The separation by gender allowed the discovery of differences between subsets of risk factors associated with each type of dyslipidemia.
Even when we obtained high-performance models with this particular data and the support of SMOTE, it is possible to note that the best classifiers identified risk factors in men with hypercholesterolemia (with a B.ACC of 83.69%) and women with hypoalphalipoproteinemia (with a B.ACC of 83.65%). Therefore, the exploration of other ML models and the continuous update of the data set may not be ruled out in future work to improve the values of the metrics and predict the development of dyslipidemia types.
The original contributions presented in the study are included in the article/Supplementary material, further inquiries can be directed to the corresponding author/s.
The studies involving humans were approved by Research Ethics Board for Biomedical Research in Humans by the National Institute of Cardiology Ignacio Chavez-Protocol approved with key 13-802. The studies were conducted in accordance with the local legislation and institutional requirements. Written informed consent for participation was not required from the participants or the participants' legal guardians/next of kin in accordance with the national legislation and institutional requirements.
GG-E designed computational strategy, implemented computing code and algorithmics, evaluated performance measures, co-supervised the project, and drafted the manuscript. TRP-Z guided the clinical approach and provided feedback to the modeling. TR-d implemented computing code and algorithmics and contributed to drafting the manuscript. MM-G performed clinical, sociomedical, and health policy research contributed to drafting the manuscript. LG-M supported data curation. MFM-M performed a clinical assessment. LMA-G contributed to clinical assessment. LMA-G, TRP-Z, and GV-A reviewed clinical results. EH-L devised the overall study strategy, co-supervised the project, performed the technical assessment, and revised and edited the manuscript. All authors read and approved the submitted version of the manuscript.
This research was supported by the National Council of Humanities, Sciences and Technology (CONAHCYT) and Cátedras CONAHCYT (Researchers for Mexico).
We would like to express our gratitude to the National Council of Humanities, Sciences and Technology (CONAHCYT) for their support under the Cátedras CONAHCYT program, No. 1591. Additionally, we extend our thanks to Dr. Maite Vallejo Allende for her logistical support for this study.
The authors declare that the research was conducted in the absence of any commercial or financial relationships that could be construed as a potential conflict of interest.
All claims expressed in this article are solely those of the authors and do not necessarily represent those of their affiliated organizations, or those of the publisher, the editors and the reviewers. Any product that may be evaluated in this article, or claim that may be made by its manufacturer, is not guaranteed or endorsed by the publisher.
The Supplementary Material for this article can be found online at: https://www.frontiersin.org/articles/10.3389/fpubh.2023.1213926/full#supplementary-material
1. Furgione A, Sánchez D, Scott G, Luti Y, Arraiz N, Bermúdez V, et al. Dislipidemias primarias como factor de riesgo para la enfermedad coronaria. Rev Latinoamericana Hipertensión. (2009) 4:18–25.
2. Narindrarangkura P, Bosl W, Rangsin R, Hatthachote P. Prevalence of dyslipidemia associated with complications in diabetic patients: a nationwide study in Thailand. Lipids Health Dis. (2019) 18:1–8. doi: 10.1186/s12944-019-1034-3
3. Pirillo A, Casula M, Olmastroni E, Norata GD, Catapano AL. Global epidemiology of dyslipidaemias. Nat Rev Cardiol. (2021) 18:689–700. doi: 10.1038/s41569-021-00541-4
4. Yuan G, Al-Shali KZ, Hegele RA. Hypertriglyceridemia: its etiology, effects and treatment. CMAJ. (2007) 176:1113–20. doi: 10.1503/cmaj.060963
6. Ibrahim MA, Asuka E, Jialal I. Hypercholesterolemia. In: StatPearls. StatPearls Publishing (2022). p. NBK459188.
7. Vega GL, Grundy SM. Hypoalphalipoproteinemia (low high density lipoprotein) as a risk factor for coronary heart disease. Curr Opin Lipidol. (1996) 7:209–16. doi: 10.1097/00041433-199608000-00007
8. Bhavsar KA, Abugabah A, Singla J, AlZubi AA, Bashir AK, et al. A comprehensive review on medical diagnosis using machine learning. Comput Mater Continua. (2021) 67:1997. doi: 10.32604/cmc.2021.014943
9. Cui S, Li C, Chen Z, Wang J, Yuan J. Research on risk prediction of dyslipidemia in steel workers based on recurrent neural network and lstm neural network. IEEE Access. (2020) 8:34153–61. doi: 10.1109/ACCESS.2020.2974887
10. Lee J, Lee BJ. Prediction model for hypertriglyceridemia based on naive bayes using facial characteristics. KIPS Trans Softw Data Eng. (2019) 8:433–40. doi: 10.3745/KTSDE.2019.8.11.433
11. Pina A, Helgadottir S, Mancina RM, Pavanello C, Pirazzi C, Montalcini T, et al. Virtual genetic diagnosis for familial hypercholesterolemia powered by machine learning. Eur J Prev Cardiol. (2020) 27:1639–46. doi: 10.1177/2047487319898951
12. Hatmal MM, Alshaer W, Mahmoud IS, Al-Hatamleh MA, Al-Ameer HJ, Abuyaman O, et al. Investigating the association of CD36 gene polymorphisms (rs1761667 and rs1527483) with T2DM and dyslipidemia: Statistical analysis, machine learning based prediction, and meta-analysis. PLoS ONE. (2021) 16:e0257857. doi: 10.1371/journal.pone.0257857
13. Kim H, Lim DH, Kim Y. Classification and prediction on the effects of nutritional intake on overweight/obesity, dyslipidemia, hypertension and type 2 diabetes mellitus using deep learning model: 4-7th Korea national health and nutrition examination survey. Int J Environ Res Public Health. (2021) 18:5597. doi: 10.3390/ijerph18115597
14. Mauvais-Jarvis F, Merz NB, Barnes PJ, Brinton RD, Carrero JJ, DeMeo DL, et al. Sex and gender: modifiers of health, disease, and medicine. Lancet. (2020) 396:565–82. doi: 10.1016/S0140-6736(20)31561-0
15. Colín-Ramírez E, Rivera-Mancía S, Infante-Vázquez O, Cartas-Rosado R, Vargas-Barrón J, Madero M, et al. Protocol for a prospective longitudinal study of risk factors for hypertension incidence in a Mexico City population: the Tlalpan 2020 cohort. BMJ Open. (2017) 7:e016773. doi: 10.1136/bmjopen-2017-016773
16. Marfell-Jones MJ, Stewart A, De Ridder J. International Standards for Anthropometric Assessment. Wellington, New Zealand: International Society for the Advancement of Kinanthropometry. (2012).
17. Craig CL, Marshall AL, Sjöström M, Bauman AE, Booth ML, Ainsworth BE, et al. International physical activity questionnaire: 12-country reliability and validity. Med Sci Sports Exer. (2003) 35:1381–95. doi: 10.1249/01.MSS.0000078924.61453.FB
18. Stewart AL, Ware JE. Measuring Functioning and Well-being: The Medical Outcomes Study Approach. Duke: Duke University Press. (1992). doi: 10.7249/CB361
19. Spritzer K, Hays R. MOS sleep scale: a manual for use and scoring, version 1.0. Los Angeles, CA. (2003). p. 1–8.
20. Hernández-Avila J, gonzález-Avilés L, Rosales-Mendoza E. Manual de usuario SNUT Sistema de Evaluación de Hábitos Nutricionales y Consumo de Nutrimentos. México: Instituto Nacional de Salud Pública. (2003).
21. Su X, Xu Y, Tan Z, Wang X, Yang P, Su Y, et al. Prediction for cardiovascular diseases based on laboratory data: an analysis of random forest model. J Clin Lab Anal. (2020) 34:e23421. doi: 10.1002/jcla.23421
22. Saheb-Honar M, Dehaki MG, Kazemi-Galougahi MH, Soleiman-Meigooni S. A comparison of three research methods: logistic regression, decision tree, and random forest to reveal association of type 2 diabetes with risk factors and classify subjects in a military population. J Arch Milit Med. (2022) 10:e118525. doi: 10.5812/jamm-118525
23. Liu J, Sun Y, Ma J, Tu J, Deng Y, He P, et al. Analysis and classification of main risk factors causing stroke in Shanxi Province. arXiv preprint arXiv:210600002. (2021). doi: 10.1016/j.imu.2021.100712
25. Chen T, Guestrin C. Xgboost: A scalable tree boosting system. In: Proceedings of the 22nd ACM SIGKDD International Conference on Knowledge Discovery and Data Mining. (2016). p. 785–794. doi: 10.1145/2939672.2939785
26. Friedman JH. Greedy function approximation: a gradient boosting machine. Ann Statist. (2001) 29:1189–1232. doi: 10.1214/aos/1013203451
27. Hsu CW, Chang CC, Lin CJ. A practical guide to support vector classification. Taipei, Taiwan (2003).
28. Alarcón-Narváez D, Hernández-Torruco J, Hernández-Oca na B, Chávez-Bosquez O, Marchi J, Méndez-Castillo JJ. Toward a machine learning model for a primary diagnosis of Guillain-Barré syndrome subtypes. Health Inf J. (2021) 27:14604582211021471. doi: 10.1177/14604582211021471
29. de la Cruz-Ruiz F, Canul-Reich J, Rivera-López R, de la Cruz-Hernández E. Impact of data balancing a multiclass dataset before the creation of association rules to study bacterial vaginosis. Intell Med. (2023) in press. doi: 10.1016/j.imed.2023.02.001
30. Feig DI, Kang DH, Johnson RJ. Uric acid and cardiovascular risk. New England J Med. (2008) 359:1811–21. doi: 10.1056/NEJMra0800885
31. Kuwabara M, Borghi C, Cicero AF, Hisatome I, Niwa K, Ohno M, et al. Elevated serum uric acid increases risks for developing high LDL cholesterol and hypertriglyceridemia: A five-year cohort study in Japan. Int J Cardiol. (2018) 261:183–8. doi: 10.1016/j.ijcard.2018.03.045
32. Ruiz-García A, Arranz-Martínez E, Morales-Cobos LE, García-Álvarez JC, Iturmendi-Martínez N, Rivera-Teijido M, et al. Prevalence rates of overweight and obesity and their associations with cardiometabolic and renal factors. SIMETAP-OB study. Clín Invest Arterioscler. (2022) 34:291–302. doi: 10.1016/j.artere.2022.10.001
33. Vallejo Quinones CS, Macias Coello CA, Suarez Hurtado LA. The sleep apnea in obese people as a predisposing factor of cardiovasvulares disorders: importance for physicians. Opuntia Brava. (2018) 10:271.
34. Parhofer KG, Laufs U. The diagnosis and treatment of hypertriglyceridemia. Deutsches Ärzteblatt Int. (2019) 116:825. doi: 10.3238/arztebl.2019.0825
35. Subramanian S, Chait A. Hypertriglyceridemia secondary to obesity and diabetes. Biochim Biophys Acta Molec Cell Biol Lipids. (2012) 1821:819–25. doi: 10.1016/j.bbalip.2011.10.003
36. Taskinen MR, Adiels M, Westerbacka J, Söderlund S, Kahri J, Lundbom N, et al. Dual metabolic defects are required to produce hypertriglyceridemia in obese subjects. Arterioscler Thromb Vasc Biol. (2011) 31:2144–50. doi: 10.1161/ATVBAHA.111.224808
37. Fried SK, Rao SP. Sugars, hypertriglyceridemia, and cardiovascular disease. Am J Clin Nutr. (2003) 78:873S–80S. doi: 10.1093/ajcn/78.4.873S
38. Brunzell JD. Hypertriglyceridemia. New England J Med. (2007) 357:1009–17. doi: 10.1056/NEJMcp070061
39. Assmann G, Schulte H, von Eckardstein A. Hypertriglyceridemia and elevated lipoprotein (a) are risk factors for major coronary events in middle-aged men. Am J Cardiol. (1996) 77:1179–84. doi: 10.1016/S0002-9149(96)00159-2
40. Lemieux I, Pascot A, Couillard C, Lamarche B, Tchernof A, Alméras N, et al. Hypertriglyceridemic waist: a marker of the atherogenic metabolic triad (hyperinsulinemia; hyperapolipoprotein B; small, dense LDL) in men? Circulation. (2000) 102:179–84. doi: 10.1161/01.CIR.102.2.179
41. Sam S, Haffner S, Davidson MH, D'Agostino Sr RB, Feinstein S, Kondos G, et al. Hypertriglyceridemic waist phenotype predicts increased visceral fat in subjects with type 2 diabetes. Diab Care. (2009) 32:1916–20. doi: 10.2337/dc09-0412
42. van Reedt Dortland AK, Giltay EJ, Van Veen T, Zitman FG, Penninx BW. Metabolic syndrome abnormalities are associated with severity of anxiety and depression and with tricyclic antidepressant use. Acta Psychiatr Scand. (2010) 122:30–9. doi: 10.1111/j.1600-0447.2010.01565.x
43. Glueck CJ, Kuller FE, Hamer T, Rodriguez R, Sosa F, Sieve-Smith L, et al. Hypocholesterolemia, hypertriglyceridemia, suicide, and suicide ideation in children hospitalized for psychiatric diseases. Pediatr Res. (1994) 35:602–10. doi: 10.1203/00006450-199405000-00013
44. Burton-Freeman B, Talbot J, Park E, Krishnankutty S, Edirisinghe I. Protective activity of processed tomato products on postprandial oxidation and inflammation: a clinical trial in healthy weight men and women. Molec Nutr Food Res. (2012) 56:622–31. doi: 10.1002/mnfr.201100649
45. Kelley DS, Siegel D, Fedor DM, Adkins Y, Mackey BE, DHA. supplementation decreases serum C-reactive protein and other markers of inflammation in hypertriglyceridemic men. J Nutr. (2009) 139:495–501. doi: 10.3945/jn.108.100354
46. Babio N, Bulló M, Basora J, Martínez-González M, Fernández-Ballart J, Márquez-Sandoval F, et al. Adherence to the Mediterranean diet and risk of metabolic syndrome and its components. Nutr Metab Cardiov Dis. (2009) 19:563–70. doi: 10.1016/j.numecd.2008.10.007
47. Mottaghi A, Bahadoran Z, Mirmiran P, Mirzaei S, Azizi F. Is dietary phytochemical index in association with the occurrence of hypertriglyceridemic waist phenotype and changes in lipid accumulation product index? A prospective approach in Tehran Lipid and Glucose Study. Int J Pharmacog Phytochem Res. (2015) 7:16–21.
48. Lim M, Kim J. Association between fruit and vegetable consumption and risk of metabolic syndrome determined using the Korean Genome and Epidemiology Study (KoGES). Eur J Nutr. (2020) 59:1667–78. doi: 10.1007/s00394-019-02021-5
49. Li Q, Zhang D, Guo C, Zhou Q, Tian G, Liu D, et al. Association of hypertriglyceridemic waist-to-height ratio and its dynamic status with incident hypertension: the Rural Chinese Cohort Study. J Hypertens. (2019) 37:2354–60. doi: 10.1097/HJH.0000000000002186
50. Zhang Z, Xue Z, Chen H, Wang T, Li Y, Chao X, et al. Prevalence of hypertension and risk factors in Uygur population in Kashgar area of Xinjiang Uygur Autonomous Region. Zhonghua liu Xing Bing xue za zhi= Zhonghua Liuxingbingxue Zazhi. (2017) 38:709–14. doi: 10.3760/cma.j.issn.0254-6450.2017.06.004
51. Wang A, Li Z, Zhou Y, Wang C, Luo Y, Liu X, et al. Hypertriglyceridemic waist phenotype and risk of cardiovascular diseases in China: results from the Kailuan Study. Int J Cardiol. (2014) 174:106–9. doi: 10.1016/j.ijcard.2014.03.177
52. Nagahama K, Inoue T, Iseki K, Touma T, Kinjo K, Ohya Y, et al. Hyperuricemia as a predictor of hypertension in a screened cohort in Okinawa, Japan. Hypert Res. (2004) 27:835–41. doi: 10.1291/hypres.27.835
53. Reaven GM, Lerner RL, Stern MP, Farquhar JW. Role of insulin in endogenous hypertriglyceridemia. J Clin Invest. (1967) 46:1756–67. doi: 10.1172/JCI105666
54. Grundy SM. Hypertriglyceridemia, insulin resistance, and the metabolic syndrome. Am J Cardiol. (1999) 83:25–9. doi: 10.1016/S0002-9149(99)00211-8
55. Mauvais-Jarvis F, Clegg DJ, Hevener AL. The role of estrogens in control of energy balance and glucose homeostasis. Endocr Rev. (2013) 34:309–38. doi: 10.1210/er.2012-1055
56. Nadal A, Alonso-Magdalena P, Soriano S, Quesada I, Ropero AB. The pancreatic β-cell as a target of estrogens and xenoestrogens: implications for blood glucose homeostasis and diabetes. Mol Cell Endocrinol. (2009) 304:63–8. doi: 10.1016/j.mce.2009.02.016
57. Gürsoy A, Kulaksizoglu M, Sahin M, Ertugrul DT, Ozer F, Tutuncu NB, et al. Severe hypertriglyceridemia-induced pancreatitis during pregnancy. J Nat Med Assoc. (2006) 98:655.
58. Zheng R, Ren P, Chen Q, Yang T, Chen C, Mao Y. Serum uric acid levels and risk of incident hypertriglyceridemia: a longitudinal population-based epidemiological study. Ann Clin Labor Sci. (2017) 47:586–91.
59. Wen CP, Cheng TYD, Chan HT, Tsai MK, Chung WSI, Tsai SP, et al. Is high serum uric acid a risk marker or a target for treatment? Examination of its independent effect in a large cohort with low cardiovascular risk. Am J Kidney Dis. (2010) 56:273–88. doi: 10.1053/j.ajkd.2010.01.024
60. Zhang Y, Zhang M, Yu X, Wei F, Chen C, Zhang K, et al. Association of hypertension and hypertriglyceridemia on incident hyperuricemia: an 8-year prospective cohort study. J Transl Med. (2020) 18:1–8. doi: 10.1186/s12967-020-02590-8
61. Lippi G, Montagnana M, Targher G, Salvagno GL, Guidi GC, et al. Relationship between uric acid, hyperglycemia and hypertriglyceridemia in general population. Biochemia Medica. (2008) 18:37–41. doi: 10.11613/BM.2008.005
62. Hou YL, Yang XL, Wang CX, Zhi LX, Yang MJ, You CG. Hypertriglyceridemia and hyperuricemia: a retrospective study of urban residents. Lipids Health Dis. (2019) 18:1–5. doi: 10.1186/s12944-019-1031-6
63. Conen D, Wietlisbach V, Bovet P, Shamlaye C, Riesen W, Paccaud F, et al. Prevalence of hyperuricemia and relation of serum uric acid with cardiovascular risk factors in a developing country. BMC Public Health. (2004) 4:1–9. doi: 10.1186/1471-2458-4-9
64. Chen S, Yang H, Chen Y, Wang J, Xu L, Miao M, et al. Association between serum uric acid levels and dyslipidemia in Chinese adults: A cross-sectional study and further meta-analysis. Medicine. (2020) 99:e19088. doi: 10.1097/MD.0000000000019088
65. Cardona F, Morcillo S, Gonzalo-Marin M, Tinahones F. The apolipoprotein E genotype predicts postprandial hypertriglyceridemia in patients with the metabolic syndrome. J Clin Endocrinol Metab. (2005) 90:2972–5. doi: 10.1210/jc.2004-1912
66. Fox IH, John D, DeBruyne S, Dwosh I, Marliss EB. Hyperuricemia and hypertriglyceridemia: metabolic basis for the association. Metabolism. (1985) 34:741–6. doi: 10.1016/0026-0495(85)90025-3
67. Bastow M, Durrington P, Ishola M. Hypertriglyceridemia and hyperuricemia: effects of two fibric acid derivatives (bezafibrate and fenofibrate) in a double-blind, placebo-controlled trial. Metabolism. (1988) 37:217–20. doi: 10.1016/0026-0495(88)90098-4
68. Cibičková L, Langová K, Vaverková H, Kubíčková V, Karásek D. Correlation of uric acid levels and parameters of metabolic syndrome. Physiol Res. (2017) 66:481. doi: 10.33549/physiolres.933410
69. Tsouli SG, Liberopoulos EN, Mikhailidis DP, Athyros VG, Elisaf MS. Elevated serum uric acid levels in metabolic syndrome: an active component or an innocent bystander? Metabolism. (2006) 55:1293–301. doi: 10.1016/j.metabol.2006.05.013
70. Cuevas-Ramos D, Almeda-Valdés P, Chávez-Manzanera E, Meza-Arana CE, Brito-Córdova G, Mehta R, et al. Effect of tomato consumption on high-density lipoprotein cholesterol level: a randomized, single-blinded, controlled clinical trial. Diab Metab Syndr Obes. (2013) 6:263. doi: 10.2147/DMSO.S48858
71. Alam P, Raka MA, Khan S, Sarker J, Ahmed N, Nath PD, et al. A clinical review of the effectiveness of tomato (Solanum lycopersicum) against cardiovascular dysfunction and related metabolic syndrome. J Herbal Med. (2019) 16:100235. doi: 10.1016/j.hermed.2018.09.006
72. Yanai H. Anti-atherosclerotic effects of tomatoes. Funct Foods Health Dis. (2017) 7:411–28. doi: 10.31989/ffhd.v7i6.351
73. Yuan C, Lee H, Shin H, Stampfer M, Cho E. Fruit and vegetable consumption and hypertriglyceridemia: Korean national health and nutrition examination surveys (KNHANES) 2007-2009. Eur J Clin Nutr. (2015) 69:1193–9. doi: 10.1038/ejcn.2015.77
74. Martinez-Lopez E, Curiel-Lopez F, Hernandez-Nazara A, Moreno-Luna LE, Ramos-Marquez ME, Roman S, et al. Influence of ApoE and FABP2 polymorphisms and environmental factors in the susceptibility to gallstone disease. Ann Hepatol. (2015) 14:515–23. doi: 10.1016/S1665-2681(19)31173-1
75. Jeong IY, Shim JE, Song S. Association of saturated fatty acid intake and its food sources with hypercholesterolemia in middle-aged Korean men and women. Cardio Metab Syndr J. (2022) 2:142–53. doi: 10.51789/cmsj.2022.2.e12
76. Febriani D. The effect of lifestyle on hypercholesterolemia. Open Public Health J. (2018) 11:526–532. doi: 10.2174/1874944501811010526
77. Dreon DM, Fernstrom HA, Campos H, Blanche P, Williams PT, Krauss RM. Change in dietary saturated fat intake is correlated with change in mass of large low-density-lipoprotein particles in men. Am J Clin Nutr. (1998) 67:828–36. doi: 10.1093/ajcn/67.5.828
78. Jakobsen MU, Overvad K, Dyerberg J, Schroll M, Heitmann BL. Dietary fat and risk of coronary heart disease: possible effect modification by gender and age. Am J Epidemiol. (2004) 160:141–9. doi: 10.1093/aje/kwh193
79. Gidding SS. Special commentary: is diet management helpful in familial hypercholesterolemia? Curr Opin Clin Nutr Metab Care. (2019) 22:135–40. doi: 10.1097/MCO.0000000000000538
80. Lee MY, Nam GE, Han K, Kim DH, Kim YH, Cho KH, et al. Association between height and hypercholesterolemia in adults: a nationwide population-based study in Korea. Lipids Health Dis. (2019) 18:1–7. doi: 10.1186/s12944-019-1148-7
81. Wan Y, Vinson JA, Etherton TD, Proch J, Lazarus SA, Kris-Etherton PM. Effects of cocoa powder and dark chocolate on LDL oxidative susceptibility and prostaglandin concentrations in humans. Am J Clin Nutr. (2001) 74:596–602. doi: 10.1093/ajcn/74.5.596
82. Jia L, Liu X, Bai YY Li SH, Sun K, He C, et al. Short-term effect of cocoa product consumption on lipid profile: a meta-analysis of randomized controlled trials. Am J Clin Nutr. (2010) 92:218–25. doi: 10.3945/ajcn.2009.28202
83. Tokede O, Gaziano J, Djousse L. Effects of cocoa products/dark chocolate on serum lipids: a meta-analysis. Eur J Clin Nutr. (2011) 65:879–86. doi: 10.1038/ejcn.2011.64
84. Galleano M, Oteiza PI, Fraga CG. Cocoa, chocolate and cardiovascular disease. J Cardiovasc Pharmacol. (2009) 54:483. doi: 10.1097/FJC.0b013e3181b76787
85. Trapani L, Pallottini V. Age-related hypercholesterolemia and HMG-CoA reductase dysregulation: sex does matter (a gender perspective). Curr Gerontol Geriatr Res. (2010) 2010:420139. doi: 10.1155/2010/420139
86. Larosa JC. Understanding risk in hypercholesterolemia. Clin Cardiol. (2003) 26:3–6. doi: 10.1002/clc.4960261303
87. Keenan JM, Morris DH. Hypercholesterolemia: dietary advice for patients regarding meat. Postgrad Med. (1995) 98:113–28. doi: 10.1080/00325481.1995.11946059
88. Cahill LE, Pan A, Chiuve SE, Sun Q, Willett WC, Hu FB, et al. Fried-food consumption and risk of type 2 diabetes and coronary artery disease: a prospective study in 2 cohorts of US women and men. Am J Clin Nutr. (2014) 100:667–75. doi: 10.3945/ajcn.114.084129
89. Matsuoka R, Masuda Y, Takeuchi A, Marushima R, Hasegawa M, Sakamoto A, et al. A double-blind, placebo-controlled study on the effects of mayonnaise containing free plant sterol on serum cholesterol concentration; safety evaluation for normocholesterolemic and mildly hypercholesterolemic Japanese subjects. J Oleo Sci. (2004) 53:79–88. doi: 10.5650/jos.53.79
90. Saito S, Takeshita M, Tomonobu K, Kudo N, Shiiba D, Hase T, et al. Dose-dependent cholesterol-lowering effect of a mayonnaise-type product with a main component of diacylglycerol-containing plant sterol esters. Nutrition. (2006) 22:174–8. doi: 10.1016/j.nut.2005.05.013
91. Loffredo L, Martino F, Carnevale R, Pignatelli P, Catasca E, Perri L, et al. Obesity and hypercholesterolemia are associated with NOX2 generated oxidative stress and arterial dysfunction. J Pediatr. (2012) 161:1004–9. doi: 10.1016/j.jpeds.2012.05.042
92. Van Itallie TB. Health implications of overweight and obesity in the United States. Ann Internal Med. (1985) 103:983–988. doi: 10.7326/0003-4819-103-6-983
93. Muscogiuri G, Tuccinardi D, Nicastro V, Barrea L, Colao A, Savastano S. Sleep disturbances: one of the culprits of obesity-related cardiovascular risk? Int J Obes Supplem. (2020) 10:62–72. doi: 10.1038/s41367-020-0019-z
94. Bidulescu A, Din-Dzietham R, Coverson DL, Chen Z, Meng YX, Buxbaum SG, et al. Interaction of sleep quality and psychosocial stress on obesity in African Americans: the Cardiovascular Health Epidemiology Study (CHES). BMC Public Health. (2010) 10:1–10. doi: 10.1186/1471-2458-10-581
95. Gangwisch JE, Malaspina D, Babiss LA, Opler MG, Posner K, Shen S, et al. Short sleep duration as a risk factor for hypercholesterolemia: analyses of the National Longitudinal Study of Adolescent Health. Sleep. (2010) 33:956–61. doi: 10.1093/sleep/33.7.956
96. Ehrenberg BL, Lamon-Fava S, Corbett KE, McNamara JR, Dallal GE, Schaefer EJ. Comparison of the effects of pravastatin and lovastatin on sleep disturbance in hypercholesterolemic subjects. Sleep. (1999) 22:117–21. doi: 10.1093/sleep/22.1.117
97. Kostis JB, Rosen RC, Wilson AC. Central nervous system effects of HMG CoA reductase inhibitors: lovastatin and pravastatin on sleep and cognitive performance in patients with hypercholesterolemia. J Clin Pharmacol. (1994) 34:989–96. doi: 10.1002/j.1552-4604.1994.tb01971.x
98. Partinen M, Pihl S, Strandberg T, Vanhanen H, Murtomäki E, Block G, et al. Comparison of effects on sleep of lovastatin and pravastatin in hypercholesterolemia. Am J Cardiol. (1994) 73:876–80. doi: 10.1016/0002-9149(94)90814-1
99. The Simvastatin Pravastatin Study Group. Comparison of the efficacy, safety and tolerability of simvastatin and pravastatin for hypercholesterolemia. Am J Cardiol. (1993) 71:1408–14. doi: 10.1016/0002-9149(93)90601-8
100. Takada M, Fujimoto M, Yamazaki K, Takamoto M, Hosomi K. Association of statin use with sleep disturbances: data mining of a spontaneous reporting database and a prescription database. Drug safety. (2014) 37:421–31. doi: 10.1007/s40264-014-0163-x
101. Kuller LH. Hyperlipidaemia and cardiovascular disease. Curr Opin Lipidol. (2002) 13:449–51. doi: 10.1097/00041433-200208000-00014
102. Yoshino G, Hirano T, Kazumi T. Atherogenic lipoproteins and diabetes mellitus. J Diab Complic. (2002) 16:29–34. doi: 10.1016/S1056-8727(01)00199-4
103. Lee JW, Lim HK, Kim JY, Ryoo S. Stress-Induced Cardiomyopathy: Clinical Observations. Intech Open Access Publisher. (2012). doi: 10.5772/30067
104. Tabatabaei P, Gilani B, Pournaqash Tehrani SS. Study of the relationship between lipids and lipoproteins with depression. Contemp Psychol Biannual J Iranian Psychol Assoc. (2007) 1:23–32.
105. De Buyzere M, Delanghe J, Labeur C, Noens L, Benoit Y, Baert J, et al. Acquired hypolipoproteinemia. Clin Chem. (1992) 38:776–81. doi: 10.1093/clinchem/38.5.776
106. Kuo P. Management of blood lipid abnormalities in coronary heart disease patients. Clin Cardiol. (1989) 12:553–60. doi: 10.1002/clc.4960121002
107. Srinivasan S. Scientific validations of anti-hyperlipidermic activity of ethanol extract of Elaecarpus variabilis. JKK Nattraja College of Pharmacy, Komarapalayam. (2017).
108. Dumon MF, Freneix-Clerc M, Maviel MJ, Clerc M. Familial hypocholesterolemia and HDL deficiency. In: Hypercholesterolemia, Hypocholesterolemia, Hypertriglyceridemia, in Vivo Kinetics. Springer (1990). p. 161–171. doi: 10.1007/978-1-4684-5904-3_21
109. Zhang Q, Jiang Z, Xu Y. HDL and Oxidation. In: HDL Metabolism and Diseases. Springer (2022). p. 63–77. doi: 10.1007/978-981-19-1592-5_5
110. Abdelkafi EOH. Evaluation of Serum Triglyceride, Cholesterol and High Density Lipoprotein Cholesterol levels among Sudanese Females with Polycystic Ovary Syndrome in Aljazeera State. Sudan University of Science & Technology (2021).
111. Chiang KM, Tsay YC, Vincent Ng TC, Yang HC, Huang YT, Chen CH, et al. Is Hyperuricemia, an early-onset metabolic disorder, causally associated with cardiovascular disease events in Han Chinese? J Clin Med. (2019) 8:1202. doi: 10.3390/jcm8081202
112. Çelık RGG, Köksal A, Şahın B, Şen A, Sakalli NK, Nalbantoğlu M. The relationship between serum uric acid levels and clinical features in essential tremor. Arch Neuropsych. (2020) 57:33. doi: 10.29399/npa.24761
113. Peters WL, Hegsted DM, Leaf A. Lipids, nutrition, and coronary heart disease. Cardiol Clin. (1985) 3:179–91. doi: 10.1016/S0733-8651(18)30679-9
114. Zhang N, Chen Y, Chen S, Jia P, Guo X, Sun G, et al. Self-reported snoring is associated with dyslipidemia, high total cholesterol, and high low-density lipoprotein cholesterol in obesity: a cross-sectional study from a rural area of China. Int J Environ Res Public Health. (2017) 14:86. doi: 10.3390/ijerph14010086
115. Kammar-García A, López-Moreno P, Hernández-Hernández ME, Ortíz-Bueno AM, Martínez-Montaño MdLC. Atherogenic index of plasma as a marker of cardiovascular risk factors in Mexicans aged 18 to 22 years. In: Baylor University Medical Center Proceedings. Taylor & Francis (2021). p. 22–27. doi: 10.1080/08998280.2020.1799479
116. Rosolova H, Dobiasova M, Soska V, Blaha V, Ceska R, Nussbaumerova B, et al. Combined therapy of mixed dyslipidemia in patients with high cardiovascular risk and changes in the lipid target values and atherogenic index of plasma. Cor Vasa. (2014) 56:e133–9. doi: 10.1016/j.crvasa.2014.01.003
117. Aguilar-Salinas CA, Olaiz G, Valles V, Torres JMR, Pérez FJG, Rull JA, et al. High prevalence of low HDL cholesterol concentrations and mixed hyperlipidemia in a Mexican nationwide survey. J Lipid Res. (2001) 42:1298–307. doi: 10.1016/S0022-2275(20)31581-9
118. Bello-Chavolla OY, Kuri-García A, Ríos-Ríos M, Vargas-Vázquez A, Cortés-Arroyo JE, Tapia-González G, et al. Familial combined hyperlipidemia: current knowledge, perspectives, and controversies. Rev Invest Clin. (2018) 70:224–36. doi: 10.24875/RIC.18002575
119. Guan C, Fu S, Zhen D, Li X, Niu J, Cheng J, et al. Correlation of serum vitamin D with lipid profiles in middle-aged and elderly Chinese individuals. Asia Pac J Clin Nutr. (2020) 29:839–45. doi: 10.6133/apjcn.202012_29(4).0020
120. Chaudhary A, Gour JK, Rizvi SI. Capsaicin has potent anti-oxidative effects in vivo through a mechanism which is non-receptor mediated. Arch Physiol Biochem. (2022) 128:141–7. doi: 10.1080/13813455.2019.1669056
121. Sanati S, Razavi BM, Hosseinzadeh H, A. review of the effects of Capsicum annuum L. and its constituent, capsaicin, in metabolic syndrome Iranian. J Basic Med Sci. (2018) 21:439. doi: 10.22038/IJBMS.2018.25200.6238
122. Li R, Xiao J, Cao Y, Huang Q, Ho CT, Lu M. Capsaicin attenuates oleic acid-induced lipid accumulation via the regulation of circadian clock genes in HepG2 cells. J Agric Food Chem. (2021) 70:794–803. doi: 10.1021/acs.jafc.1c06437
123. Hidaka H, Takiwaki M, Yamashita M, Kawasaki K, Sugano M, Honda T. Consumption of nonfat milk results in a less atherogenic lipoprotein profile: a pilot study. Ann Nutr Metab. (2012) 61:111–6. doi: 10.1159/000339261
124. Lopez-Huertas E. Health effects of oleic acid and long chain omega-3 fatty acids (EPA and DHA) enriched milks. A review of intervention studies. Pharmacol Res. (2010) 61:200–7. doi: 10.1016/j.phrs.2009.10.007
125. Rouillier P, Boutron-Ruault MC, Bertrais S, Arnault N, Daudin JJ, Bacro JN, et al. Alcohol and atherosclerotic vascular disease risk factors in French men: relationships are linear, J-shaped, and U-shaped. Alcoholism. (2005) 29:84–8. doi: 10.1097/01.ALC.0000150005.52605.FA
126. Martina B, Weinbacher M, Kiener S, Keller U, Battegay E. Reproducibility of fasting serum cholesterol and triglycerides in ambulatory patients with mixed hyperlipidemia. Schweiz Med Wochenschr. (1996) 126:2175–80.
127. Kudzma D, Schonfeld G. Alcoholic hyperlipidemia: induction by alcohol but not by carbohydrate. J Lab Clin Med. (1971) 77:384–95.
128. Bermudez OI, Toher C, Montenegro-Bethancourt G, Vossenaar M, Mathias P, Doak C, et al. Dietary intakes and food sources of fat and fatty acids in Guatemalan schoolchildren: a cross-sectional study. Nutr J. (2010) 9:1–15. doi: 10.1186/1475-2891-9-20
129. Denova-Gutiérrez E, Casta nón S, Talavera JO, Gallegos-Carrillo K, Flores M, Dosamantes-Carrasco D, et al. Dietary patterns are associated with metabolic syndrome in an urban Mexican population. J Nutr. (2010) 140:1855–1863. doi: 10.3945/jn.110.122671
130. Abdo EM, Shaltout OES, Ali S, Mansour HM. A functional orange juice fortified with beetroot by-products attenuates hyperlipidemia and obesity induced by a high-fat diet. Antioxidants. (2022) 11:457. doi: 10.3390/antiox11030457
131. Mallick N, Khan RA. Antihyperlipidemic effects of Citrus sinensis, Citrus paradisi, and their combinations. J Pharm Bioall Sci. (2016) 8:112. doi: 10.4103/0975-7406.171727
132. Huang YL, Ma YS, Tsai YH, Chang SK. In vitro hypoglycemic, cholesterol-lowering and fermentation capacities of fiber-rich orange pomace as affected by extrusion. Int J Biol Macromol. (2019) 124:796–801. doi: 10.1016/j.ijbiomac.2018.11.249
133. Korth RM. Women with overweight, mixed hyperlipidemia, intolerance to glucose and diastolic hypertension. Health. (2014) 6:64. doi: 10.4236/health.2014.65064
134. Zhang Y, Ding X, Hua B, Liu Q, Gao H, Chen H, et al. High triglyceride-glucose index is associated with poor cardiovascular outcomes in nondiabetic patients with ACS with LDL-C below 1.8 mmol/L. J Atheroscler Thromb. (2022) 29:268–81. doi: 10.5551/jat.61119
135. Lim LF, Solmi M, Cortese S. Association between anxiety and hypertension in adults: a systematic review and meta-analysis. Neurosci. Biobehav Rev. (2021) 131:96–119. doi: 10.1016/j.neubiorev.2021.08.031
136. Talpur MTH, Katbar MT, Shabir KU, Shabir KU, Yaqoob U, Jabeen S, et al. Prevalence of dyslipidemia in young adults. Profess Med J. (2020) 27:987–93. doi: 10.29309/TPMJ/2020.27.05.4040
137. Chen L, Caballero B, Mitchell DC, Loria C, Lin PH, Champagne CM, et al. Reducing consumption of sugar-sweetened beverages is associated with reduced blood pressure: a prospective study among United States adults. Circulation. (2010) 121:2398–406. doi: 10.1161/CIRCULATIONAHA.109.911164
Keywords: hypertriglyceridemia, hypercholesterolemia, hypoalphalipoproteinemia, mixed hyperlipidemias, feature selection, machine learning, Tlalpan 2020 cohort, Mexico City
Citation: Gutiérrez-Esparza G, Pulido T, Martínez-García M, Ramírez-delReal T, Groves-Miralrio LE, Márquez-Murillo MF, Amezcua-Guerra LM, Vargas-Alarcón G and Hernández-Lemus E (2023) A machine learning approach to personalized predictors of dyslipidemia: a cohort study. Front. Public Health 11:1213926. doi: 10.3389/fpubh.2023.1213926
Received: 28 April 2023; Accepted: 23 August 2023;
Published: 20 September 2023.
Edited by:
Zhendong Liu, Shandong First Medical University, ChinaReviewed by:
Suman Kundu, Vanderbilt University Medical Center, United StatesCopyright © 2023 Gutiérrez-Esparza, Pulido, Martínez-García, Ramírez-delReal, Groves-Miralrio, Márquez-Murillo, Amezcua-Guerra, Vargas-Alarcón and Hernández-Lemus. This is an open-access article distributed under the terms of the Creative Commons Attribution License (CC BY). The use, distribution or reproduction in other forums is permitted, provided the original author(s) and the copyright owner(s) are credited and that the original publication in this journal is cited, in accordance with accepted academic practice. No use, distribution or reproduction is permitted which does not comply with these terms.
*Correspondence: Enrique Hernández-Lemus, ZWhlcm5hbmRlekBpbm1lZ2VuLmdvYi5teA==; Guadalupe Gutiérrez-Esparza, Z2d1dGllcnJlemVAY29uYWN5dC5teA==
†These authors share first authorship
Disclaimer: All claims expressed in this article are solely those of the authors and do not necessarily represent those of their affiliated organizations, or those of the publisher, the editors and the reviewers. Any product that may be evaluated in this article or claim that may be made by its manufacturer is not guaranteed or endorsed by the publisher.
Research integrity at Frontiers
Learn more about the work of our research integrity team to safeguard the quality of each article we publish.