- 1Section of Basic Psychology, Department of Military Medical Psychology, Air Force Medical University, Xi'an, Shaanxi, China
- 2Section of Military Psychology, Department of Military Medical Psychology, Air Force Medical University, Xi'an, Shaanxi, China
Background: Anxiety and depression are among the greatest contributors to the global burden of diseases. The close associations of personality traits with anxiety and depression have been widely described. However, the common practice of sum scores in previous studies limits the understanding of the fine-grained connections between different personality traits and anxiety and depression symptoms and cannot explore and compare the risk or protective effects of personality traits on anxiety and depression symptoms.
Objective: We aimed to determine the fine-grained connections between different personality traits and anxiety and depression symptoms and identify the detrimental or protective effects of different personality traits on anxiety and depression symptoms.
Methods: A total of 536 college students from China were recruited online, and the average age was 19.98 ± 1.11. The Chinese version of the Ten-Item Personality Inventory, Generalized Anxiety Disorder-7, and Patient Health Questionnaire-9 was used to investigate the personality traits and symptoms of anxiety and depression of participants after they understood the purpose and filling method of the survey and signed the informed consent. The demographic characteristics were summarized, and the scale scores were calculated. The network model of personality traits and symptoms of anxiety and depression was constructed, and bridge expected influence (BEI) was measured to evaluate the effect of personality traits on anxiety and depression. The edge accuracy and BEI stability were estimated, and the BEI difference and the edge weight difference were tested.
Results: In the network, 29 edges (indicating partial correlations between variables) bridged the personality community and the anxiety and depression community, among which the strongest correlations were extraversion-fatigue, agreeableness-suicidal ideation, conscientiousness-uncontrollable worry, neuroticism-excessive worry, neuroticism-irritability, and openness-feelings of worthlessness. Neuroticism had the highest positive BEI value (0.32), agreeableness had the highest negative BEI value (−0.27), and the BEI values of neuroticism and agreeableness were significantly different from those of most other nodes (p < 0.05).
Conclusion: There are intricate correlations between personality traits and the symptoms of anxiety and depression in college students. Neuroticism was identified as the most crucial risk trait for depression and anxiety symptoms, while agreeableness was the most central protective trait.
1. Introduction
Anxiety and depression are among the greatest contributors to the global burden of diseases (1), with detrimental effects on mental and physical health, such as an increased risk of suicidal thoughts and suicide attempts (2, 3), insomnia (4), daily maladaptive health behaviors (5), Parkinson’s disease, and cardiovascular disease (6, 7). In particular, early adulthood — college undergraduate students are usually in this stage — is a critical period, with heightened susceptibility to anxiety and depressive disorders, with more than 20% of young adults meeting the criteria for anxiety disorders and the prevalence of depression reaching 25% among undergraduate students (8, 9). Recent studies showed that the prevalence of anxiety and depression among Chinese college students was also high (10–13), for example, at 22.7 and 46.8%, respectively, according to a study (13). In addition to common consequences, college students who have developed anxiety or depression may have difficulties in academic functioning and suffer from low quality of life, heightening the necessity for research into this issue in China.
Given that anxiety and depression are prevalent and often accompanied by a decline in quality of life, unpleasant symptoms, and impaired social relationships, it is important to identify the pathogenesis of anxiety and depression. Personality traits have been identified as risk/protective factors for anxiety and depression (9, 14–18). The Big Five (i.e., five facets of personality traits including neuroticism, extraversion, agreeableness, conscientiousness, and openness) is one of the most relevant frameworks when examining the personality trait related to anxiety and depression (17, 19, 20). As the Big Five represents personality traits that usually precede the symptoms of anxiety and depression, they are considered risk/protective factors for anxiety and depression (20, 21). A cross-sectional study on Taiwan college students found that neuroticism was significantly positively associated with anxiety and depression scores while agreeableness was significantly inversely associated with anxiety and depression scores (21). Another study also revealed that agreeableness was inversely associated with depression while neuroticism was significantly associated with depression scores (17). Studies have reported that high levels of conscientiousness and extraversion were protective against the deleterious effects of high levels of neuroticism on depressive mood (22). Consistently, a systematic review showed that neuroticism was considered a risk factor while extraversion and conscientiousness were protective factors for affective disorders such as depression and anxiety (20).
As described above, the relationships between the Big Five personality traits and anxiety and depression have been extensively investigated. Many researchers have used total scores to measure anxiety and depression when investigating the relationships between the Big Five and anxiety and depression, assuming that anxiety or depression is a holistic psychological construct. For example, a previous study used the standardized Depression Anxiety Stress Scale-21 (DASS-21) to assess anxiety and depression and five subscales of the Big Five Inventory (BFI) to assess the Big Five, then examined the associations between the DASS-21 total score and the Big Five (21). According to the cumulative risk hypothesis, the overall risk of a negative outcome, such as depression and anxiety, is magnified by interaction with distinct personality traits (23). For instance, openness can moderate the influence of extraversion, and these two personality traits work together to reduce the risk of anxiety (24). In addition to the fact that personality involves the interaction of different traits, depression and anxiety are subsumptions containing different interacting symptoms (25). However, considering that anxiety and depressive disorders consist of distinct symptoms, the common practice of sum scores, according to previous studies (26, 27), obscures the relative importance of different symptoms and limits the understanding of the fine-grained connections between different personality traits and anxiety and depression symptoms. Imagine two individuals have the same sum score, they would commonly be considered to have the same degree of depression; however, one may have a high score on anhedonia and a low score on fatigue while the other may demonstrate the reverse pattern; their depression actually differs according to the relative importance of anhedonia (core symptom) and fatigue (28). Hence, analysis at a symptom level may provide a way forward, which is essential to understanding psychopathological pathways and effective intervention targets that could not be discovered by relying solely on total scores (27, 29, 30). To the best of our knowledge, there is a paucity of data on symptom-level links between personality traits and symptoms of anxiety and depression, which hampers the identification of more efficacious targets to intervene. This knowledge gap motivated the present study.
One approach to achieve the study objectives is network analysis, which is a data-driven method used to reveal the connections among individual variables, regardless of whether these variables are symptoms (31–35). In the network theory, psychopathological constructs are represented and visualized as networks emerging from interactions between distinct variables, which indicates that the variables (symptoms or otherwise) and their active interactions lead to the development and maintenance of constructs (32, 33, 36). The network commonly consists of nodes, which represent variables, and edges, representing correlations between variables (37). Network analysis can yield important findings. It can be used to examine the fine-grained relationships between individual variables (38–40), shedding light on the important psychopathological pathways between constructs via edge weights. The approach also identifies bridge nodes, which connect to nodes of another community composed of a theory-based group of variables and are key regarding the impacts of one community on another; the identified bridge variables can be considered promising and effective targets for prevention, intervention, and treatment (40–43).
Network analysis has been used to study the relationships between personality traits and psychopathological constructs. For example, a study examined the network structure of substance use disorder, the Big Five personality traits, impulsivity, and psychopathological constructs, including depression and anxiety; however, the presence of anxiety or depression was the sole node in its community, which did not reveal the symptom-level relationships between personality traits and depression and anxiety (44). In another previous study, the network structure of schizotypal personality, autistic traits, obsessive–compulsive traits, depression, and anxiety was investigated; however, this analysis also regarded depression or anxiety as merely one node in the network rather than as a symptom-level construct (45). To the best of our knowledge, there is a lack of network analysis studies investigating the symptom-level relationships of the Big Five personality traits with symptoms of anxiety and depression.
To fill this knowledge gap, we constructed a network consisting of the Big Five personality traits, anxiety symptoms, and depression symptoms. We aimed to elucidate the important pathways linking personality traits with anxiety and depression and to identify important bridge nodes that maximally link nodes among different communities. Based on the findings, we attempted to provide theoretical insights into the specific pathways between distinct personality traits and individual anxiety or depression symptoms and provide implications for prevention and intervention in light of the risk and protective roles that personality traits play.
2. Materials and methods
2.1. Participants
We designed the online survey powered by www.wjx.cn, and the systematically trained investigators sent the quick response code of the survey to the WeChat group of students from three colleges in Xi’an and Shanghai, China. We explained the purpose and the filling method of the survey and obtained the informed consent of the participants. From April to May 2022, 536 students completed the survey. The Chinese version of the Ten-Item Personality Inventory (TIPI-C), Generalized Anxiety Disorder-7 (GAD-7), and Patient Health Questionnaire-9 (PHQ-9) was used to investigate the personality traits and symptoms of anxiety and depression of college students. Questionnaires that were not fully answered were excluded. A total of 507 (94.59%) questionnaires were valid. This study strictly followed the tenets of the Declaration of Helsinki and was approved by the Ethics Committee of the Xijing Hospital of the Air Force Medical University (KY20224106-1).
2.2. Measures
2.2.1. Tipi-c
The TIPI-C is the Chinese version of a scale developed by Li (46) based on the TIPI (47) to assess personality traits, and the reliability and validity of the scale meet the demand of psychometrics for measuring the personality traits of Chinese people (46). It is a short scale with 10 items and five factors, namely, neuroticism, conscientiousness, agreeableness, openness, and extraversion. The scale uses a 7-point Likert scale, ranging from one (completely disagree) to seven (completely agree).
2.2.2. Gad-7
The GAD-7 was compiled by Spitzer et al. (48), as a self-screening tool to assess anxiety symptoms. The Chinese version of GAD-7 used for this study was revised by He et al. (49), and it has good reliability and validity in the Chinese population (49). It contains seven items scored on a 4-point Likert scale, from zero (not at all) to three (almost every day). The GAD-7 total score ranges from zero to 21, and a higher score represents severer anxiety symptoms. The GAD-7 had high reliability in this study (Cronbach’s α coefficient = 0.90).
2.2.3. PHQ-9
The PHQ-9 was developed by Kroenke et al. (50) to measure the severity of depression in the past 2 weeks. The Chinese version of PHQ-9 (51) was used in this study, and it has been proven to have good reliability and validity among Chinese adolescents (52). The scale contains nine items scored on a 4-point Likert scale from zero (not at all) to three (almost every day). The total score of the PHQ-9 ranges from zero to 27, and the higher the score the severer the depression symptoms. Cronbach’s α coefficient of the PHQ-9 in this study was 0.88.
2.3. Statistical analysis
SPSS 22.0 was used to summarize the demographic characteristics of participants and calculate the scale scores. R 4.1.1 software was used to construct the network model, measure bridge centrality, and test the robustness of the network.
2.3.1. Network model construction
The R package qgraph (53) was used for network model construction. In the network, nodes represented dimensions of the TIPI-C and items of the GAD-7 and PHQ-9. The term “community” in network analysis is used to indicate a theory-based group of nodes that correspond to a psychological structure or psychiatric disorder (40). The nodes in this study were divided into two communities, namely, the personality community and the anxiety and depression community. Edge in the network represented a partial correlation of two nodes after statistical control for the interference of other nodes (54). The least absolute shrinkage and selection operator (LASSO) regularization (55) and extended Bayesian information criterion (EBIC) (56) were used in combination to obtain a comprehensible network by setting trivial edges to a weight of zero (37). We set the EBIC hyperparameter γ to 0.5 and used the Fruchterman-Reingold (57) algorithm to lay out the network.
2.3.2. Bridge centrality measurement
The R package network tools were used in the bridge centrality measurement (40). The bridge expected influence (BEI) was used in this study given its suitability for networks with positive and negative edges (58). The BEI of a node is defined as the sum of the edge weights between the node and nodes from another community; the higher the BEI value of a node the stronger the influence of the node on the other community (58).
2.3.3. Network robustness test
The R package bootnet was used to test the robustness of the network (37). The non-parametric bootstrapping method (1,000 bootstrapped samples) was used to estimate the 95% confidence intervals of edge weights to test the edge accuracy. Case-dropping bootstrapping (1,000 bootstrapped samples) was used to test the BEI stability, and the correlation stability (CS) coefficient was calculated to quantify the stability. Ideal stability is indicated by a CS coefficient higher than 0.5 (37). The bootstrapping method (1,000 bootstrapped samples) was used to test the BEI difference of nodes and the edge weight difference of node pairs in the network (α = 0.05).
3. Results
3.1. Demographic characteristics and descriptive statistics
Demographic characteristics of the college students are shown in Table 1. The means, standard deviations, and BEI values of nodes in the network are shown in Table 2.
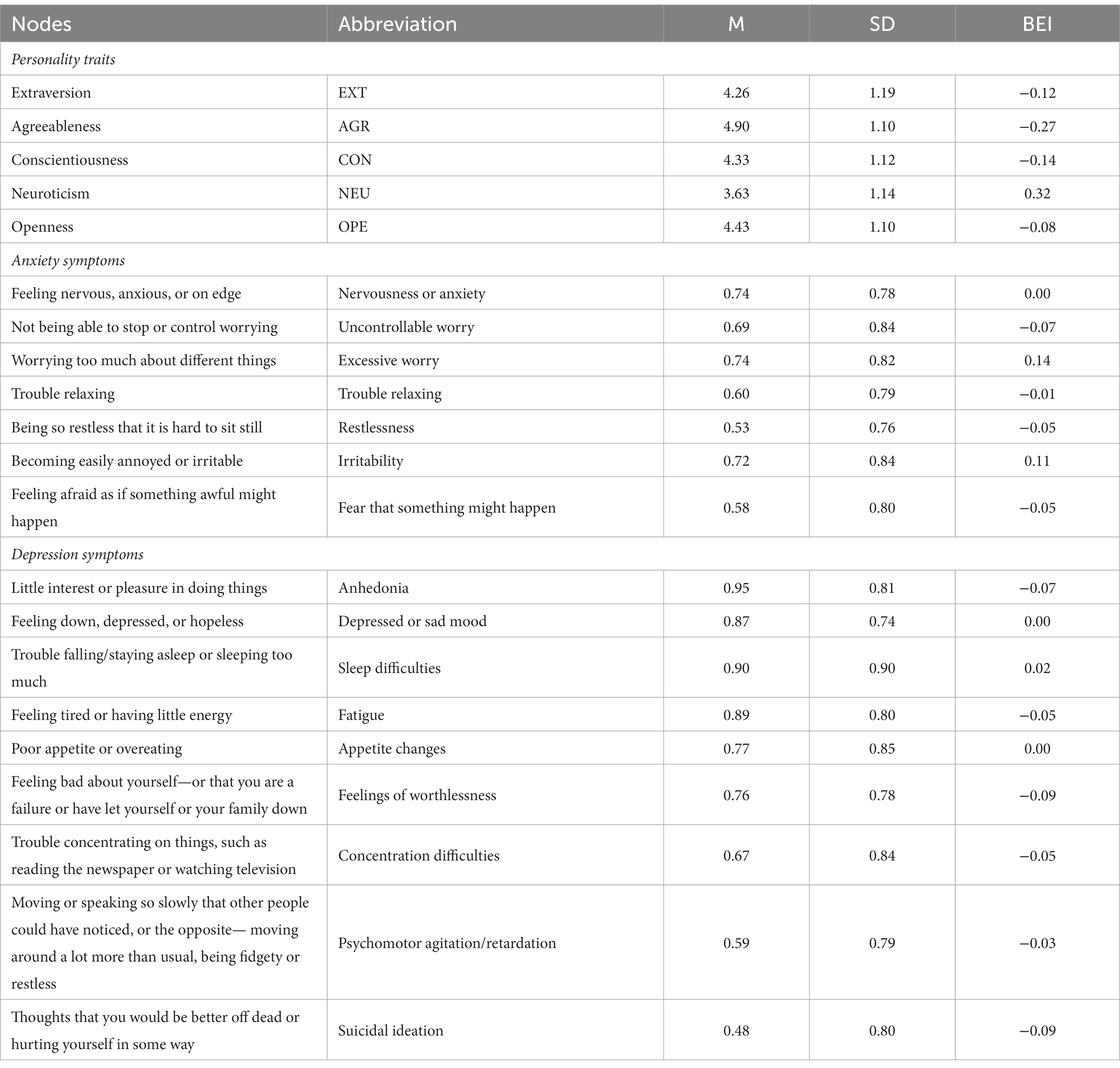
Table 2. The means, standard deviations, and BEI values of nodes in the personality trait–anxiety and depression network.
3.2. The personality trait–anxiety and depression network structure
Figure 1A displays the structure of the personality trait–anxiety and depression network. The network contained 125 non-zero edges (edge weights ranged from-0.15 to 0.23), among which 29 edges bridged the personality community and the anxiety and depression community (23.20%). Of these cross-community edges, EXT was negatively correlated with A7 “fear that something might happen,” D1 “anhedonia,” D4 “fatigue,” D7 “concentration difficulties,” and D9 “suicidal ideation”; the strongest correlation was with D4 “fatigue” (edge weight = −0.06). AGR was positively correlated with D3 “sleep difficulties” (edge weight = 0.02) and negatively correlated with eight nodes of the anxiety and depression community, among which the strongest correlation was with D9 “suicidal ideation” (edge weight = −0.06). CON was negatively correlated with A1 “nervousness or anxiety,” A2 “uncontrollable worry,” D1 “anhedonia,” D4 “fatigue,” and D6 “feelings of worthlessness”; the strongest correlation with CON was A2 “uncontrollable worry” (edge weight = −0.05). NEU was positively correlated with six nodes of the anxiety and depression community, among which the strongest correlations were with A3 “excessive worry” (edge weight = 0.14) and A6 “irritability” (edge weight = 0.11). OPE was negatively correlated with A7 “fear that something might happen,” D1 “anhedonia,” D2 “depressed or sad mood,” and D6 “feelings of worthlessness”; the strongest correlation was with D6 “feelings of worthlessness” (edge weight = −0.06). The correlation matrix of the network is displayed in Supplementary Table S1 of the Supplementary Material.
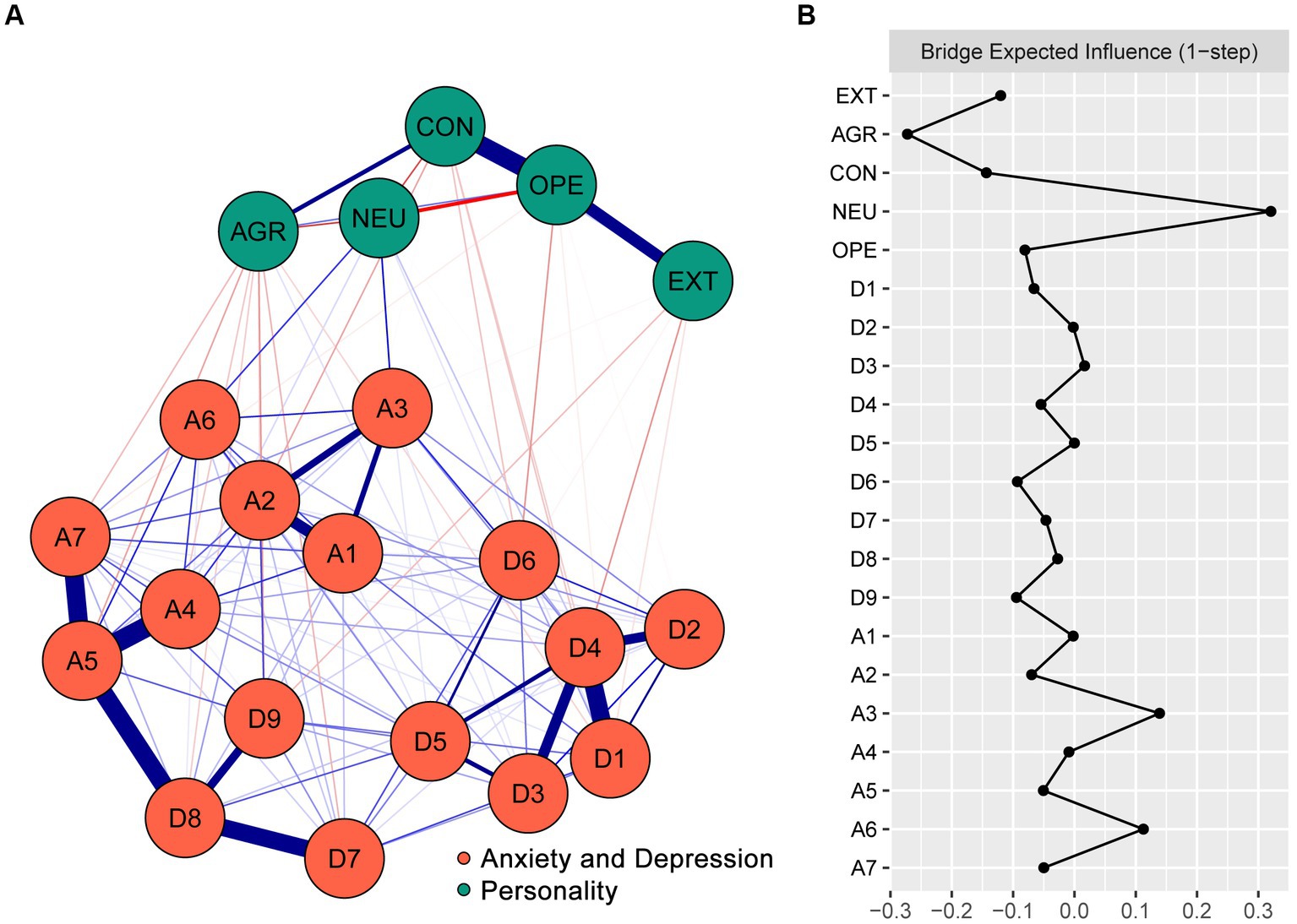
Figure 1. The structure of the personality trait–anxiety and depression network among college students and the BEI values of nodes. (A) The structure of the personality trait–anxiety and depression network. Blue edges represent positive partial correlations, and red edges represent negative partial correlations. The wider the edge, the stronger the partial correlation. (B) The BEI values of nodes in the network (raw scores). EXT, Extraversion; AGR, Agreeableness; CON, Conscientiousness; NEU, Neuroticism; and OPE, Openness. A1, nervousness or anxiety; A2, uncontrollable worry; A3, excessive worry; A4, trouble relaxing; A5, restlessness; A6, irritability; A7, fear that something might happen; D1, anhedonia; D2, depressed or sad mood; D3, sleep difficulties; D4, fatigue; D5, appetite changes; D6, feelings of worthlessness; D7, concentration difficulties; D8, psychomotor agitation/retardation; D9, suicidal ideation.
As shown in Supplementary Figure S1 of the Supplementary Material, the relatively narrow 95% CIs of edge weights indicated that these edge weight estimations were accurate. The results of difference tests on edge weights are shown in Supplementary Figure S2 of the Supplementary Material.
3.3. Bei values of nodes in the network
Figure 1B shows the BEI values of the nodes in the personality trait–anxiety and depression network. In the personality community, NEU had the highest positive BEI value (0.32), and AGR had the highest negative BEI value (−0.27). In the anxiety and depression community, A3 “excessive worry” had the highest BEI value (0.14).
As shown in Supplementary Figure S3 in the Supplementary Material, the BEI values of NEU, AGR, and A3 “excessive worry” were significantly different from the BEI values of most other nodes (p < 0.05). Supplementary Figure S4 in the Supplementary Material displays the results of the BEI stability test. The CS coefficient of BEI in the network was 0.67, suggesting ideal stability.
4. Discussion
There are two prevailing views on the relationship between mental disorders and symptoms. The perspective of classification diagnosis considers symptoms to reflect mental disorders, as in the DSM-5 (32). The dimension diagnosis perspective considers mental disorders as a compound of different symptom dimensions (59). However, both of these perspectives overlook the interactions between symptoms, a fundamental phenomenon in mental disorders (60). According to the network theory, mental disorders are a dynamic system composed of interacting symptoms (61); in the network structure, the edges that bridge communities reveal the psychopathological interactions among different psychological structures and mental disorders (62). As the nodes in the network belong to two different communities measuring personality traits and symptoms of anxiety and depression respectively, the nodes within a community have high consistency; the connections within a community are closer than those across the community. However, the understanding of crucial cross-community edges is based on the network theory of the relationship between mental disorders and symptoms, which can enhance our knowledge of the fine-grained relationships between personality traits and anxiety and depression symptoms. In view of this, we discussed the strongest cross-community edges in the network.
Among symptoms correlated with extraversion, “fatigue” had the strongest negative edge weight. Fatigue is a general feeling of weariness and lack of energy and motivation (63). Extroversion is often accompanied by low allostatic load and great aerobic capacity (64), and high extraversion is correlated with better health outcomes and well-being, such as lower frailty, fewer affective symptoms, and fewer sleeping problems (65–68); thus, individuals high in extraversion are prone to experience low levels of fatigue. Indeed, the advantages of extraversion may explain the positive effects of cognitive behavioral therapy in chronic fatigue syndrome, alleviating daily fatigue and pain, both mentally and physically (68). “Suicidal ideation” was negatively correlated with agreeableness. As one of the most empirically tested theories, the interpersonal-psychological theory of suicide provides a theoretical framework that explains this relationship. The theory assumes that there are three factors underpinning suicidal thoughts and behaviors, namely, perceived burdensomeness, thwarted belongingness, and acquired capability for suicide (the degree to which one is able to enact suicide attempts) (69). Perceived burdensomeness is an individual’s belief that they are a burden to their family, friends, or society, and thwarted belongingness is an unmet need for social connection. Suicidal ideation increases when perceived burdensomeness and thwarted belongingness cooccur (70); however, this cooccurrence is less likely in individuals with high agreeableness, who tend to be good-natured, modest, cooperative, and emotionally satisfied by social interaction (71). Conscientiousness was negatively correlated with “uncontrollable worry” in college students. Conscientiousness is characterized by individual differences in the propensity for self-discipline, orderliness, and reliability in the pursuit of work completion (72). According to Gao et al. (73), self-control is a key component in the structure of conscientiousness, and individuals with high conscientiousness are adept at controlling unnecessary worries. Neuroticism was positively correlated with “irritability” and “excessive worry.” Neuroticism is defined as emotional negativity and instability; people high in neuroticism are irrational perfectionists and tend to catastrophize difficulties (74); they are more prone to irritability when encountering setbacks (75). A correlation between neuroticism and worry was previously reported (76), and the neural basis of excessive worry helps explain how neuroticism contributes to psychopathological vulnerability (77). Openness was negatively correlated with “feelings of worthlessness.” From a cognitive perspective, individuals who tend to seek, comprehend, and utilize complex patterns of information in the world might be more adaptable and therefore less susceptible to depression (78). However, the results of multiple meta-analyzes have revealed no direct association between openness and depression (79, 80). The contradictory results may stem from complicated associations between openness and specific symptoms of depression; the non-correlation between openness and depression on an overall level covered up the internal fine-grained relationships.
BEI reflects the role of a given variable in maintaining the interaction between different psychological structures or mental disorders (81); variables with high BEI values can be regarded as bridges and serve as potential targets to reduce or enhance this interactive influence (58, 81). In the present study, BEI value helped to identify the detrimental or protective effects of different personality traits on anxiety and depression symptoms. Neuroticism was identified as the most crucial risk trait for depression and anxiety symptoms, while agreeableness was the most central protective trait according to their highest positive and negative BEI values, respectively. Neuroticism reflects emotional instability and is a powerful predictor of negative emotional experiences, including depression and anxiety (82–84). A previous study suggested that neuroticism and depression share a common genetic basis (85). A plausible neural mechanism underlying the vulnerability of individuals high in neuroticism to depression is sensitivity to stress-related reductions in response of the ventral striatum to reward (86). Individual differences in neuroticism and trait anxiety were predicted by volume variation in the left amygdala (18). The negative correlations between agreeableness and anxiety and between agreeableness and depression have been confirmed by many studies (87, 88). Individuals high in agreeableness are prone to positive emotions, and agreeableness has been found to be a protective factor against anxiety induced by the COVID-19 pandemic (89). From a network perspective, compared with other intervening personality traits, reducing neuroticism and enhancing agreeableness have more advantages in reducing anxiety and depression. In addition, digital applications have been proven to be an effective method for personality intervention (90).
There are many studies on the relationship between the different Big Five personality traits and anxiety and depression (22, 91–95). However, these studies treated anxiety or depression as a whole and overlooked the interconnections of different symptoms. In addition, due to the limitations of statistical methods, the risk or protective effects of different personality traits on anxiety and depression cannot be compared. Based on network analysis, this study provides us with a clear understanding of the risk or protective effects of each personality trait on different anxiety and depression symptoms and helps quantitatively compare the overall risk or protective effects of different personality traits on anxiety and depression, thus providing a reference for potential intervention targets.
Several limitations should be noted when interpreting the findings of the current study. First, a cross-sectional design was used; thus, the directionality and causality of the relationship between personality traits and symptoms of anxiety and depression could not be determined (96). Second, the study used a convenience sample of Chinese college students, potentially limiting external validity and generalization to the broader population. Third, TIPI-C is a brief scale, and the facets of the personality traits TIPI-C covered may not be as sufficient as other Big Five personality scales. Last, the selection of psychological intervention targets was based on the network analysis theory; relatively weak effects of the relationships between personality traits and symptoms of anxiety and depression may indicate limited practical application of the results, and further intervention research is needed to verify whether interventions targeting the identified bridge variables will be effective.
In summary, the present study represents the first utilization of network analysis to elucidate the relationship between personality traits and anxiety and depression in college students. The intuitive network models help to develop a comprehensive understanding of the fine-grained correlations of personality traits with anxiety and depression. BEI values facilitated the identification of the key variables bridging personality traits with anxiety and depression and highlighted potential targets for psychological intervention.
Data availability statement
The raw data supporting the conclusions of this article will be made available by the authors, without undue reservation.
Ethics statement
The studies involving human participants were reviewed and approved by The Ethics Committee of the Xijing Hospital of the Air Force Medical University (KY20224106-1). Written informed consent to participate in this study was provided by the participants or their legal guardian/next of kin.
Author contributions
YG, XL, and XZ: concept and design and critical revision of the manuscript. TY and ZG: acquisition of data and drafting of the manuscript. ZG and TY: analysis and interpretation of the data. All authors contributed to the article and approved the submitted version.
Funding
This study was funded by the Air Force Medical University (KJZFJ2020-1, 2021JQ-335).
Acknowledgments
The authors thank the participants who contributed to our research. TY sincerely thanks Miss Jiaying Lin for her encouragement and support in research and writing work.
Conflict of interest
The authors declare that the research was conducted in the absence of any commercial or financial relationships that could be construed as a potential conflict of interest.
Publisher’s note
All claims expressed in this article are solely those of the authors and do not necessarily represent those of their affiliated organizations, or those of the publisher, the editors and the reviewers. Any product that may be evaluated in this article, or claim that may be made by its manufacturer, is not guaranteed or endorsed by the publisher.
Supplementary material
The Supplementary material for this article can be found online at: https://www.frontiersin.org/articles/10.3389/fpubh.2023.1204285/full#supplementary-material
Abbreviations
TIPI-C, Chinese version of the Ten-Item Personality Inventory; GAD-7, Generalized Anxiety Disorder-7; PHQ-9, Patient Health Questionnaire-9; LASSO, least absolute shrinkage and selection operator; EBIC, extended Bayesian information criterion; BEI, bridge expected influence; CS coefficient, correlation stability coefficient; DSM, Diagnostic and Statistical Manual of Mental Disorders.
References
1. James, S, Abate, D, Abate, K, Abay, S, Abbafati, C, Abbasi, N, et al. Global, regional, and national incidence, prevalence, and years lived with disability for 354 diseases and injuries for 195 countries and territories, 1990-2017: a systematic analysis for the global burden of disease study 2017. Lancet. (2018) 392:1789–858. doi: 10.1016/S0140-6736(18)32279-7
2. Wei, S, Li, H, Hou, J, Chen, W, Tan, S, Chen, X, et al. Comparing characteristics of suicide attempters with suicidal ideation and those without suicidal ideation treated in the emergency departments of general hospitals in China. Psychiatry Res. (2018) 262:78–83. doi: 10.1016/j.psychres.2018.02.007
3. Yook, V, Kim, H, Kim, EJ, Kim, Y, Lee, G, Choi, JH, et al. Psychological autopsy study comparing suicide decedents with and without a history of suicide attempts in a nationwide sample of South Korea. Suicide Life Threat Behav. (2022) 52:190–8. doi: 10.1111/sltb.12750
4. Wang, X, Wang, N, Zhong, L, Wang, S, Zheng, Y, Yang, B, et al. Prognostic value of depression and anxiety on breast cancer recurrence and mortality: a systematic review and meta-analysis of 282, 203 patients. Mol Psychiatry. (2020) 25:3186–97. doi: 10.1038/s41380-020-00865-6
5. Dalton, ED, and Hammen, CL. Independent and relative effects of stress, depressive symptoms, and affect on college students' daily health behaviors. J Behav Med. (2018) 41:863–74. doi: 10.1007/s10865-018-9945-4
6. Forbes, EJ, Byrne, GJ, O'Sullivan, JD, Yang, J, Marsh, R, and Dissanayaka, NN. Defining atypical anxiety in parkinson's disease. Mov Disord Clin Pract. (2021) 8:571–81. doi: 10.1002/mdc3.13193
7. Roseman, A, Morton, L, and Kovacs, AH. Health anxiety among adults with congenital heart disease. Curr Opin Cardiol. (2021) 36:98–104. doi: 10.1097/HCO.0000000000000811
8. Copeland, WE, Angold, A, Shanahan, L, and Costello, EJ. Longitudinal patterns of anxiety from childhood to adulthood: the great smoky mountains study. J Am Acad Child Adolesc Psychiatry. (2014) 53:21–33. doi: 10.1016/j.jaac.2013.09.017
9. Sheldon, E, Simmonds-Buckley, M, Bone, C, Mascarenhas, T, Chan, N, Wincott, M, et al. Prevalence and risk factors for mental health problems in university undergraduate students: a systematic review with meta-analysis. J Affect Disord. (2021) 287:282–92. doi: 10.1016/j.jad.2021.03.054
10. Liu, X, Ping, S, and Gao, W. Changes in undergraduate students' psychological well-being as they experience university life. Int J Environ Res Public Health. (2019) 16:2864. doi: 10.3390/ijerph16162864
11. Cheng, S, An, D, Yao, Z, Liu, JJ, Ning, X, Wong, JP, et al. Association between mental health knowledge level and depressive symptoms among Chinese college students. Int J Environ Res Public Health. (2021) 18:1850. doi: 10.3390/ijerph18041850
12. Duan, H, Gong, M, Zhang, Q, Huang, X, and Wan, B. Research on sleep status, body mass index, anxiety and depression of college students during the post-pandemic era in Wuhan. China J Affect Disord. (2022) 301:189–92. doi: 10.1016/j.jad.2022.01.015
13. Xu, H, Wang, Z, Peng, L, Mi, Y, Zeng, P, and Liu, X. The study of psychological traits among Chinese college students during the COVID-19 campus lockdown. Front Psychol. (2022) 13:1051770. doi: 10.3389/fpsyg.2022.1051770
14. Bertani, DE, Mattei, G, Ferrari, S, Pingani, L, and Galeazzi, GM. Anxiety, depression and personality traits in Italian medical students. Riv Psichiatr. (2020) 55:342–8. doi: 10.1708/3503.34892
15. Takahashi, Y, Yamagata, S, Ritchie, SJ, Barker, ED, and Ando, J. Etiological pathways of depressive and anxiety symptoms linked to personality traits: A genetically-informative longitudinal study. J Affect Disord. (2021) 291:261–9. doi: 10.1016/j.jad.2021.05.004
16. Tao, Y, Liu, X, Hou, W, Niu, H, Wang, S, Ma, Z, et al. The mediating role of emotion regulation strategies in the relationship between big five personality traits and anxiety and depression among Chinese firefighters. Front Public Health. (2022) 10:901686. doi: 10.3389/fpubh.2022.901686
17. Yasui-Furukori, N, Murakami, H, Otaka, H, Nakayama, H, Murabayashi, M, Mizushiri, S, et al. Personality associated with depressive status in individuals with type 2 diabetes mellitus. Neuropsychiatr Dis Treat. (2019) 15:1133–9. doi: 10.2147/NDT.S200012
18. Hu, Y, Moore, M, Bertels, Z, Phan, KL, Dolcos, F, and Dolcos, S. Smaller amygdala volume and increased neuroticism predict anxiety symptoms in healthy subjects: a volumetric approach using manual tracing. Neuropsychologia. (2020) 145:106564. doi: 10.1016/j.neuropsychologia.2017.11.008
19. Lyon, KA, Juhasz, G, Brown, LJE, and Elliott, R. Big five personality facets explaining variance in anxiety and depressive symptoms in a community sample. J Affect Disord. (2020) 274:515–21. doi: 10.1016/j.jad.2020.05.047
20. Lyon, KA, Elliott, R, Ware, K, Juhasz, G, and Brown, LJE. Associations between facets and aspects of big five personality and affective disorders: a systematic review and best evidence synthesis. J Affect Disord. (2021) 288:175–88. doi: 10.1016/j.jad.2021.03.061
21. Yang, SW, and Koo, M. The big five personality traits as predictors of negative emotional states in university students in Taiwan. Int J Environ Res Public Health. (2022) 19:16468. doi: 10.3390/ijerph192416468
22. Boudouda, NE, and Gana, K. Neuroticism, conscientiousness and extraversion interact to predict depression: a confirmation in a non-western culture. Pers Individ Dif. (2020) 167:110219. doi: 10.1016/j.paid.2020.110219
23. Andersen, AM, and Bienvenu, OJ. Personality and psychopathology. Int Rev Psychiatry. (2011) 23:234–47. doi: 10.3109/09540261.2011.588692
24. Łakuta, P. Personality trait interactions in risk for and protection against social anxiety symptoms. J Psychol. (2019) 153:599–614. doi: 10.1080/00223980.2019.1581723
25. Milaneschi, Y, Lamers, F, Peyrot, WJ, Abdellaoui, A, Willemsen, G, Hottenga, JJ, et al. Polygenic dissection of major depression clinical heterogeneity. Mol Psychiatry. (2016) 21:516–22. doi: 10.1038/mp.2015.86
26. Fried, EI. Problematic assumptions have slowed down depression research: why symptoms, not syndromes are the way forward. Front Psychol. (2015) 6:309. doi: 10.3389/fpsyg.2015.00309
27. Fried, EI, and Nesse, RM. Depression sum-scores don't add up: why analyzing specific depression symptoms is essential. BMC Med. (2015) 13:72. doi: 10.1186/s12916-015-0325-4
28. Guo, Z, Yang, T, Qiu, R, Qiu, H, Ren, L, Liu, X, et al. Network analysis of the relationships between problematic smartphone use and anxiety, and depression in a sample of Chinese college students. Front Psych. (2023) 14:1097301. doi: 10.3389/fpsyt.2023.1097301
29. Fried, EI, and Nesse, RM. Depression is not a consistent syndrome: an investigation of unique symptom patterns in the STAR*D study. J Affect Disord. (2015) 172:96–102. doi: 10.1016/j.jad.2014.10.010
30. Liang, S, Liu, C, Rotaru, K, Li, K, Wei, X, Yuan, S, et al. The relations between emotion regulation, depression and anxiety among medical staff during the late stage of COVID-19 pandemic: a network analysis. Psychiatry Res. (2022) 317:114863. doi: 10.1016/j.psychres.2022.114863
31. Borsboom, D, and Cramer, AO. Network analysis: an integrative approach to the structure of psychopathology. Annu Rev Clin Psychol. (2013) 9:91–121. doi: 10.1146/annurev-clinpsy-050212-185608
32. McNally, RJ. Can network analysis transform psychopathology? Behav Res Ther. (2016) 86:95–104. doi: 10.1016/j.brat.2016.06.006
33. Borsboom, D. A network theory of mental disorders. World Psychiatry. (2017) 16:5–13. doi: 10.1002/wps.20375
34. Marcus, DK, Preszler, J, and Zeigler-Hill, V. A network of dark personality traits: what lies at the heart of darkness? J Res Pers. (2018) 73:56–62. doi: 10.1016/j.jrp.2017.11.003
35. Guo, Z, Yang, T, He, Y, Tian, W, Wang, C, Zhang, Y, et al. The relationships between suicidal ideation, meaning in life, and affect: a network analysis. Int J Ment Health Addict. (2023):1–20. doi: 10.1007/s11469-023-01019-9
36. McNally, RJ. Network analysis of psychopathology: controversies and challenges. Annu Rev Clin Psychol. (2021) 17:31–53. doi: 10.1146/annurev-clinpsy-081219-092850
37. Epskamp, S, Borsboom, D, and Fried, EI. Estimating psychological networks and their accuracy: a tutorial paper. Behav Res Methods. (2018) 50:195–212. doi: 10.3758/s13428-017-0862-1
38. Beard, C, Millner, AJ, Forgeard, MJ, Fried, EI, Hsu, KJ, Treadway, MT, et al. Network analysis of depression and anxiety symptom relationships in a psychiatric sample. Psychol Med. (2016) 46:3359–69. doi: 10.1017/S0033291716002300
39. Galderisi, S, Rucci, P, Kirkpatrick, B, Mucci, A, Gibertoni, D, Rocca, P, et al. Interplay among psychopathologic variables, personal resources, context-related factors, and real-life functioning in individuals with schizophrenia: a network analysis. JAMA Psychiat. (2018) 75:396–404. doi: 10.1001/jamapsychiatry.2017.4607
40. Jones, PJ, Ma, R, and McNally, RJ. Bridge centrality: a network approach to understanding comorbidity. Multivariate Behav Res. (2021) 56:353–67. doi: 10.1080/00273171.2019.1614898
41. Contreras, A, Nieto, I, Valiente, C, Espinosa, R, and Vazquez, C. The study of psychopathology from the network analysis perspective: a systematic review. Psychother Psychosom. (2019) 88:71–83. doi: 10.1159/000497425
42. Guo, Z, He, Y, Yang, T, Ren, L, Qiu, R, Zhu, X, et al. The roles of behavioral inhibition/activation systems and impulsivity in problematic smartphone use: a network analysis. Front Public Health. (2022) 10:1014548. doi: 10.3389/fpubh.2022.1014548
43. Guo, Z, Liang, S, Ren, L, Yang, T, Qiu, R, He, Y, et al. Applying network analysis to understand the relationships between impulsivity and social media addiction and between impulsivity and problematic smartphone use. Front Psych. (2022) 13:993328. doi: 10.3389/fpsyt.2022.993328
44. Poon, JYK, Hu, H-X, Lam, M, Lui, SSY, and Chan, RCK. The interplay between addictive behaviour and psychopathology and personality in substance use disorder: a network analysis in treatment-seeking patients with alcohol and drug use. Int J Ment Health Addict. (2022):1–8. doi: 10.1007/s11469-022-00976-x
45. Zhang, R-T, Zhou, H-Y, Wang, Y-M, Yang, Z-Y, Wang, Y, So, SH, et al. Network analysis of schizotypal personality traits and their association with other subclinical psychiatric features. Asian J Psychiatry. (2019) 44:209–16. doi: 10.1016/j.ajp.2019.08.005
46. Li, J. Psychometric properties of ten-item personality inventory in China. Chin J Health Psychol. (2013) 21:1688–392. doi: 10.13342/j.cnki.cjhp.2013.11.008
47. Gosling, SD, Rentfrow, PJ, and Swann, WB. A very brief measure of the big-five personality domains. J Res Pers. (2003) 37:504–28. doi: 10.1016/S0092-6566(03)00046-1
48. Spitzer, RL, Kroenke, K, Williams, JBW, and Löwe, B. A brief measure for assessing generalized anxiety disorder. Arch Intern Med. (2006) 166:1092. doi: 10.1001/archinte.166.10.1092
49. He, X, Li, C, Qian, J, Cui, H, and Wu, W. Reliability and validity of a generalized anxiety disorder scale in general hospital outpatients. Shanghai Arch Psychiatry. (2010) 22:200–3. doi: 10.3969/j.issn.1002-0829.2010.04.002
50. Kroenke, K, Spitzer, RL, and Williams, JB. The PHQ-9: validity of a brief depression severity measure. J Gen Intern Med. (2001) 16:606–13. doi: 10.1046/j.1525-1497.2001.016009606.x
51. Xu, Y, Wu, H, and Xu, Y. The reliability and validity of patient health questionnaire depression module (PHQ-9) in Chinese elderly. Shanghai Arch Psychiatry. (2007) 05:276.
52. Hu, X, Zhang, Y, Liang, W, Zhang, H, and Yang, S. Reliability and validity of the patient health questionnaire-9 in Chinese adolescents. Sichuan Mental Health. (2014) 27:357–60.
53. Epskamp, S, Cramer, AOJ, Waldorp, LJ, Schmittmann, VD, and Borsboom, D. Qgraph: network visualizations of relationships in psychometric data. J Stat Softw. (2012) 48:1–18. doi: 10.18637/jss.v048.i04
54. Epskamp, S, and Fried, EI. A tutorial on regularized partial correlation networks. Psychol Methods. (2018) 23:617–34. doi: 10.1037/met0000167
55. Tibshirani, R. Regression shrinkage and selection via the lasso. J R Stat Soc. (1996) 58:267–88. doi: 10.1111/j.2517-6161.1996.tb02080.x
56. Chen, J, and Chen, Z. Extended Bayesian information criteria for model selection with large model spaces. Biometrika. (2008) 95:759–71. doi: 10.1093/biomet/asn034
57. Fruchterman, TMJ, and Reingold, EM. Graph drawing by force-directed placement. Softw: Pract Exp. (1991) 21:1129–64. doi: 10.1002/spe.4380211102
58. Robinaugh, DJ, Millner, AJ, and McNally, RJ. Identifying highly influential nodes in the complicated grief network. J Abnorm Psychol. (2016) 125:747–57. doi: 10.1037/abn0000181
60. Schmittmann, VD, Cramer, AOJ, Waldorp, LJ, Epskamp, S, Kievit, RA, and Borsboom, D. Deconstructing the construct: a network perspective on psychological phenomena. New Ideas Psychol. (2013) 31:43–53. doi: 10.1016/j.newideapsych.2011.02.007
61. Robinaugh, DJ, Hoekstra, RHA, Toner, ER, and Borsboom, D. The network approach to psychopathology: a review of the literature 2008-2018 and an agenda for future research. Psychol Med. (2020) 50:353–66. doi: 10.1017/S0033291719003404
62. Mansur-Alves, M, Machado, GM, Rodrigues, WS, Neufeld, CB, and Carvalho, LF. Perfectionism dimensions: a network analysis of their relationships with affect and obsessive-compulsive pathological personality traits. Scand J Psychol. (2022) 63:165–72. doi: 10.1111/sjop.12798
63. Hamilton, W, Watson, J, and Round, A. Investigating fatigue in primary care. BMJ. (2010) 341:c4259. doi: 10.1136/bmj.c4259
64. Stephan, Y, Sutin, AR, Luchetti, M, and Terracciano, A. Allostatic load and personality: a 4-year longitudinal study. Psychosom Med. (2016) 78:302–10. doi: 10.1097/PSY.0000000000000281
65. Hakulinen, C, Elovainio, M, Pulkki-Raback, L, Virtanen, M, Kivimaki, M, and Jokela, M. Personality and depressive symptoms: individual participant meta-analysis of 10 cohort studies. Depress Anxiety. (2015) 32:461–70. doi: 10.1002/da.22376
66. Stephan, Y, Sutin, AR, Canada, B, and Terracciano, A. Personality and frailty: evidence from four samples. J Res Pers. (2017) 66:46–53. doi: 10.1016/j.jrp.2016.12.006
67. Diener, E, Oishi, S, and Lucas, RE. Personality, culture, and subjective well-being: emotional and cognitive evaluations of life. Annu Rev Psychol. (2003) 54:403–25. doi: 10.1146/annurev.psych.54.101601.145056
68. White, PD, Goldsmith, KA, Johnson, AL, Potts, L, Walwyn, R, DeCesare, JC, et al. Comparison of adaptive pacing therapy, cognitive behaviour therapy, graded exercise therapy, and specialist medical care for chronic fatigue syndrome (PACE): a randomised trial. Lancet. (2011) 377:823–36. doi: 10.1016/S0140-6736(11)60096-2
69. Bryan, CJ, Morrow, CE, Anestis, MD, and Joiner, TE. A preliminary test of the interpersonal-psychological theory of suicidal behavior in a military sample. Pers Individ Differ. (2010) 48:347–50. doi: 10.1016/j.paid.2009.10.023
70. Chu, C, Buchman-Schmitt, JM, Hom, MA, Stanley, IH, and Joiner, TE Jr. A test of the interpersonal theory of suicide in a large sample of current firefighters. Psychiatry Res. (2016) 240:26–33. doi: 10.1016/j.psychres.2016.03.041
71. Roccas, S, Sagiv, L, Schwartz, SH, and Knafo, A. The big five personality factors and personal values. Personal Soc Psychol Bull. (2016) 28:789–801. doi: 10.1177/0146167202289008
72. Griffin, SA, and Samuel, DB. A closer look at the lower-order structure of the personality inventory for DSM-5: comparison with the five-factor model. Personal Disord. (2014) 5:406–12. doi: 10.1037/per0000074
73. Gao, K, Zhang, R, Xu, T, Zhou, F, and Feng, T. The effect of conscientiousness on procrastination: the interaction between the self-control and motivation neural pathways. Hum Brain Mapp. (2021) 42:1829–44. doi: 10.1002/hbm.25333
74. Miller, JD, and Pilkonis, PA. Neuroticism and affective instability: the same or different? Am J Psychiatry. (2006) 163:839–45. doi: 10.1176/ajp.2006.163.5.839
75. Castagna, PJ. Structure related to function: prefrontal surface area has an indirect effect on the relationship between amygdala volume and trait neuroticism. Brain Struct Funct. (2019) 224:3309–20. doi: 10.1007/s00429-019-01974-x
76. Muris, P, Roelofs, J, Rassin, E, Franken, I, and Mayer, B. Mediating effects of rumination and worry on the links between neuroticism, anxiety and depression. Pers Individ Differ. (2005) 39:1105–11. doi: 10.1016/j.paid.2005.04.005
77. Servaas, MN, Riese, H, Ormel, J, and Aleman, A. The neural correlates of worry in association with individual differences in neuroticism. Hum Brain Mapp. (2014) 35:4303–15. doi: 10.1002/hbm.22476
78. Meiran, N, Diamond, GM, Toder, D, and Nemets, B. Cognitive rigidity in unipolar depression and obsessive compulsive disorder: examination of task switching, Stroop, working memory updating and post-conflict adaptation. Psychiatry Res. (2011) 185:149–56. doi: 10.1016/j.psychres.2010.04.044
79. Kotov, R, Gamez, W, Schmidt, F, and Watson, D. Linking big personality traits to anxiety, depressive, and substance use disorders: a meta-analysis. Psychol Bull. (2010) 136:768–821. doi: 10.1037/a0020327
80. Markon, KE, Krueger, RF, and Watson, D. Delineating the structure of normal and abnormal personality: an integrative hierarchical approach. J Pers Soc Psychol. (2005) 88:139–57. doi: 10.1037/0022-3514.88.1.139
81. Heeren, A, Jones, PJ, and McNally, RJ. Mapping network connectivity among symptoms of social anxiety and comorbid depression in people with social anxiety disorder. J Affect Disord. (2018) 228:75–82. doi: 10.1016/j.jad.2017.12.003
82. Barlow, DH, Curreri, AJ, and Woodard, LS. Neuroticism and disorders of emotion: a new synthesis. Curr Dir Psychol Sci. (2021) 30:410–7. doi: 10.1177/09637214211030253
83. Chen, X, Pu, J, Shi, W, and Zhou, Y. The impact of neuroticism on symptoms of anxiety and depression in elderly adults: the mediating role of rumination. Curr Psychol. (2020) 39:42–50. doi: 10.1007/s12144-017-9740-3
84. Tonarely, NA, Sherman, JA, Grossman, RA, Shaw, AM, and Ehrenreich-May, J. Neuroticism as an underlying construct in youth emotional disorders. Bull Menn Clin. (2020) 84:214–36. doi: 10.1521/bumc.2020.84.3.214
85. Meng, W, Adams, MJ, Reel, P, Rajendrakumar, A, Huang, Y, Deary, IJ, et al. Genetic correlations between pain phenotypes and depression and neuroticism. Eur J Hum Genet. (2020) 28:358–66. doi: 10.1038/s41431-019-0530-2
86. Bondy, E, Baranger, DA, Balbona, JV, Sputo, K, Paul, SE, Oltmanns, T, et al. Neuroticism and reward-related ventral striatum activity: probing vulnerability to stress-related depression. J Abnorm Psychol. (2021) 130:223–35. doi: 10.1037/abn0000618
87. Nouri, F, Feizi, A, Keshteli, AH, Roohafza, H, Afshar, H, and Adibi, P. Personality traits are differently associated with depression and anxiety: evidence from applying bivariate multiple binary logistic regression on a large sample of general adults. Psychiatr Danub. (2019) 31:448–56. doi: 10.24869/psyd.2019.448
88. Nikčević, AV, Marino, C, Kolubinski, DC, Leach, D, and Spada, MM. Modelling the contribution of the big five personality traits, health anxiety, and COVID-19 psychological distress to generalised anxiety and depressive symptoms during the COVID-19 pandemic. J Affect Disord. (2021) 279:578–84. doi: 10.1016/j.jad.2020.10.053
89. Kumar, VV, and Tankha, G. The relationship between personality traits and COVID-19 anxiety: a mediating model. Behav Sci. (2022) 12:24. doi: 10.3390/bs12020024
90. Allemand, M, and Flückiger, C. Personality change through digital-coaching interventions. Curr Dir Psychol Sci. (2022) 31:41–8. doi: 10.1177/09637214211067782
91. Peñacoba-Puente, C, Marín-Morales, D, Carmona-Monge, FJ, and Velasco, FL. Post-partum depression, personality, and cognitive-emotional factors: a longitudinal study on Spanish pregnant women. Health Care Women Int. (2016) 37:97–117. doi: 10.1080/07399332.2015.1066788
92. Mongrain, M, Barnes, C, Barnhart, R, and Zalan, LB. Acts of kindness reduce depression in individuals low on agreeableness. Transl Issues Psychol Sci. (2018) 4:323–34. doi: 10.1037/tps0000168
93. Carrillo, JM, Rojo, N, Sánchez-Bernardos, ML, and Avia, MD. Openness to experience and depression. Eur J Psychol Assess. (2001) 17:130–6. doi: 10.1027//1015-5759.17.2.130
94. van der Veen, DC, van Dijk, SDM, Comijs, HC, van Zelst, WH, Schoevers, RA, and Oude Voshaar, RC. The importance of personality and life-events in anxious depression: from trait to state anxiety. Aging Ment Health. (2017) 21:1177–83. doi: 10.1080/13607863.2016.1202894
95. Muris, P, Meesters, C, and van Asseldonk, M. Shame on me! Self-conscious emotions and big five personality traits and their relations to anxiety disorders symptoms in young, non-clinical adolescents. Child Psychiatry Hum Dev. (2018) 49:268–78. doi: 10.1007/s10578-017-0747-7
Keywords: personality, anxiety, depression, college students, network analysis
Citation: Yang T, Guo Z, Zhu X, Liu X and Guo Y (2023) The interplay of personality traits, anxiety, and depression in Chinese college students: a network analysis. Front. Public Health. 11:1204285. doi: 10.3389/fpubh.2023.1204285
Edited by:
Kit-Aun Tan, Universiti Putra Malaysia, MalaysiaReviewed by:
Ramayah T., University of Science Malaysia (USM), MalaysiaSoon Li Lee, Monash University Malaysia, Malaysia
Khadijeh Irandoust, Imam Khomeini International University, Iran
Copyright © 2023 Yang, Guo, Zhu, Liu and Guo. This is an open-access article distributed under the terms of the Creative Commons Attribution License (CC BY). The use, distribution or reproduction in other forums is permitted, provided the original author(s) and the copyright owner(s) are credited and that the original publication in this journal is cited, in accordance with accepted academic practice. No use, distribution or reproduction is permitted which does not comply with these terms.
*Correspondence: Xufeng Liu, lxf_fmmu@126.com; Yaning Guo, 13659205403@163.com
†These authors have contributed equally to this work