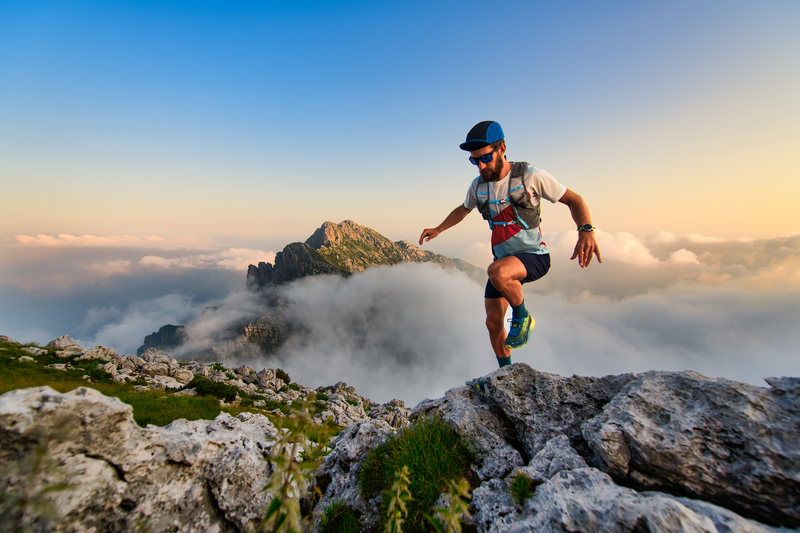
94% of researchers rate our articles as excellent or good
Learn more about the work of our research integrity team to safeguard the quality of each article we publish.
Find out more
REVIEW article
Front. Public Health , 04 May 2023
Sec. Aging and Public Health
Volume 11 - 2023 | https://doi.org/10.3389/fpubh.2023.1171243
This article is part of the Research Topic Novel Indicators and Strategies for Prevention and Management of Physical and Cognitive Frailty in Aging Population View all 14 articles
This review examines the current literature to identify biomarkers of frailty across patients with solid tumors. We conducted the systematic review using preferred reporting items for systematic reviews and meta-analysis guidelines (PRISMA). PubMed, Web of Science, and Embase databases were searched from their inception to December 08, 2021, for reports of biomarkers and frailty. Two reviewers independently screened titles, abstracts, and full-text articles. A quality assessment was conducted using NHLBI Quality Assessment Tool for Observational Cohort and Cross-Sectional Studies, and Quality Assessment of Case-Control Studies. In total, 915 reports were screened, and 14 full-text articles were included in the review. Most studies included breast tumors, were cross-sectional in design, and measured biomarkers at baseline or pre-treatment. Frailty tools varied with Fried Frailty Phenotype and the geriatric assessment most frequently used. Increased inflammatory parameters (i.e., Interleukin-6, Neutrophil Lymphocyte Ratio, Glasgow Prognostic Score-2) were associated with frailty severity. Only six studies were rated as good quality using assessment ratings. Together, the small number of studies and heterogeneity in frailty assessment limited our ability to draw conclusions from the extant literature. Future research is needed to identify potential target biomarkers of frailty in cancer survivors that may aid in early detection and referral.
Cancer and cancer therapies may contribute to the development of early onset frailty, a geriatric syndrome that is indicative of multi-system decline and often precipitates mortality (1–4). The prevalence of frailty has been reported to range from 8% in adult survivors of childhood cancer to 59 percent in older adult cancer survivors using phenotypic and deficit accumulation frailty measures (5). Sustained or worsening phenotypic frailty measured prior-to post-cancer diagnosis significantly increases the risk of mortality in patients with solid tumors (breast, lung, colorectal, ovarian, and endometrial) (6). Thus, there is an increased need for early identification of patients at risk for developing frailty to aid in timely therapeutic interventions.
Two commonly used, but conceptually distinct constructs of frailty, include: (i) phenotypic frailty, where frailty is a defined and measurable state (e.g., fried frailty phenotype) (7) and (ii) the accumulation of deficits, where frailty is more of a stochastic process, in which random deficits lead to increased vulnerability (8). While phenotypic frailty evaluates signs/symptoms (e.g., weight loss, exhaustion, and weakness) and may exist independent of medically classified conditions as a pre-disability syndrome, deficit accumulation frailty is based on a long checklist of signs/symptoms and medically classified conditions, including disability (9). Phenotypic frailty is most useful if the goal is to define risk factors and mechanisms with a degree of specificity for sub-clinical and clinical frailty because individuals are stratified into distinct risk categories and specific pathways can be identified for prevention and remediation. Stochastic deficit accumulation frailty may be helpful for individual prognostication and targeting shared risk factors or biological mechanisms (10). To encompass the two conceptual definitions, in this review, frailty will be operationalized as both phenotypic frailty (7) and deficit accumulation frailty (8).
Cancer and cancer treatments may accelerate aging which may be measured using correlates or biomarkers representative of hallmarks of aging (e.g., telomere attrition, epigenetic alteration, loss of proteostasis, deregulated nutrient sensing, mitochondrial dysfunction, senescence, and inflammation) and may in turn lead to early frailty states (2, 5, 11). Indeed, several studies report that completion of primary cancer therapy (post-treatment) accelerates biological aging in cancer survivors, as evidenced by increased expression of cytokines (12, 13), senescence-associated p16INK4 (13), and decreased telomere length (14). However, little is known about the association of these biological measures of aging with frailty in cancer survivors with solid tumors. For example, several recent reviews and/or meta-analyses evaluated common frailty biomarkers in older adults, but few included oncologic studies (15–18). The search for sensitive and specific biomarkers of frailty in oncological populations is crucial for early detection of aging-related consequences of cancer and its treatments on cancer survivors (2). Such biomarkers may offer diagnostic and prognostic utility by aiding clinical assessment of frailty signs/symptoms and may help evaluate the effectiveness of interventions designed to mitigate (or potentially reverse) phenotypic and deficit accumulation frailty. Given the heterogeneity in the biology, treatments, and frailty rates (19, 20) between hematologic and solid cancers, this review evaluates biomarkers of frailty specific to cancer survivors with solid tumors.
Potential target biomarkers of frailty may be used to identify cancer survivors at risk for the development of frailty. To fill this gap, this systematic review synthesizes current literature by examining (i) frailty measures and (ii) biomarkers evaluated in association with phenotypic frailty and deficit accumulation in patients with solid tumors across all age groups.
A systematic review was conducted using Preferred Reporting Items for Systematic Reviews and Meta-Analysis (PRISMA) guidelines (21).
Inclusion criteria were: (a) published in the English language, (b) molecular measures that correlate with the aging process (hallmarks of aging) (11): telomere attrition, epigenetic alteration, loss of proteostasis, deregulated nutrient sensing, mitochondrial dysfunction, senescence, and inflammation, (c) evaluated phenotypic frailty or deficit accumulation, and (d) measured an association between the biomarker and phenotypic frailty/deficit accumulation. Studies with non-solid tumors and non-human subjects were excluded.
A medical librarian (D.C.) conducted electronic database searches of PubMed, Web of Science, and Embase databases of publications from the date of inception to December 08, 2021. Frailty was operationalized as both the phenotypic frailty (7) and deficit accumulation frailty (8) consistent with prior reviews on frailty and biomarkers (15, 16). The search terms included: solid tumors (brain, breast, colon, lung, pancreatic, prostate, and ovarian), biomarkers (cytokines, extracellular vesicles, microRNA, mitochondrial DNA, telomere length, cell senescence markers, inflammageing, epigenetic alterations, mitochondrial dysfunction, and stem cell exhaustion), and outcomes (accelerated aging, frailty, functional decline, and deficit accumulation). The complete search strategy with MeSH terms and Boolean operators for each database is detailed in Supplementary Table S1. References from retrieved reviews and Google Scholar were scanned for additional studies using key search terms.
Two reviewers (D.S. and B.P.S.) independently screened titles and abstracts and subsequently full-text articles for study eligibility using the covidence systematic review software (Veritas Health Innovation, Melbourne, Australia). Any incongruencies were resolved upon discussion and consultation with the third researcher (T.S.A.). Two reviewers (D.S. and B.P.S.) completed data abstraction and D.S. reviewed all the final abstracted information. To preserve integrity of the data, the authors kept written communication records of decisions on incongruencies related to data abstraction. Data were extracted using a standardized form for key variables (sample, tumor type, stages, time points, study design, frailty instruments, molecules measured, statistical methods, and key findings).
Risk of bias assessment was conducted using the National Heart, Lung, and Blood Institute quality assessment tool for observational cohort and cross-sectional studies and a tool for case–control studies (22). The tools consist of 12–14 methodological quality items rated as “yes,” “no,” or “other (cannot determine, not reported, not applicable)” (Supplementary Table S2). The questions evaluate the internal validity of each study, considering the potential risk of biases such as information bias, measurement bias, or outcome bias. The greater the bias (higher number of items rated as “no”), the lower the assigned rating. Reviewers (D.S. and B.P.S.) conducted independent quality assessments. Incongruencies were discussed with the third reviewer’s input (T.S.A.) and concordance was reached upon discussion. To grade the overall quality of the studies, the percentage of items free of bias (items rated as “yes”) out of all possible items was calculated. Studies were assigned overall quality ratings according to the following categories: poor (<50%), fair (≥50% and ≤70%), and good (>70%).
Descriptive statistics were calculated (such as mean, range, and standard deviation) for variable age using either the reported mean or median. Where available, data on race/ethnicity (white vs. non-white) and sex (male vs. female) was extracted.
The study selection process is detailed in a PRISMA flow diagram (Figure 1). Briefly, 910 reports were retrieved from the databases. Five additional articles were identified through screening references of relevant reviews and Google Scholar using the search criteria. After removing 19 duplicate reports, search results were uploaded to the covidence software where an additional five reports were identified as duplicates. Two reviewers (D.S. and B.P.S.) independently screened 888 titles and abstracts, of which 844 reports were deemed irrelevant (Supplementary Table S3). Five additional reports were located through Google Scholar and 49 reports were retrieved for full-text review. In total, 14 full-text articles were included in the review. Of the 35 excluded reports, 13 did not measure frailty, 12 were conference abstracts, six were not primary research studies, two were not in human subjects, and two did not measure an association between frailty and biomarkers. Although the study by Falandry and authors (23) did not explicitly use the term “frailty,” the study met the inclusion criteria for measuring “decline in functional reserve” using the geriatric vulnerability score consistent with deficit accumulation definition.
Characteristics of the included studies are presented in Table 1. All 14 studies were observational study designs. Seven studies were longitudinal cohort studies (23, 26–31), six were cross-sectional (32–37), and one study was a case-control design (38). Across the 14 studies, a total of 2,178 participants were included, with the sample size of each study ranging from 20 to 581. The mean age across all studies was 72 years (standard deviation = 7, range: 53–80 years). Thirteen studies reported information on sex and the distribution was 63% female and 37% male. Four studies reported information on race/ethnicity (28, 29, 37, 38), of which 82% of participants were white and 18% non-white.
The most commonly studied solid tumor was breast (n = 6, 43%) (27–29, 32–34), followed by prostate tumor (n = 3, 21%) (26, 36, 38) (Table 1). Stages of cancer varied greatly ranging from stage I to IV (or localized to metastasized) and most studies were initiated at pre-treatment (i.e., at the diagnosis, pre-inclusion, prior to surgery or adjuvant treatments) (n = 11) (23, 27–35, 37) (Table 1). Among studies which included participants on treatment (n = 6, 43%), three studies in prostate cancer (n = 3, 21%) had patients receiving ADT and three studies with participants with breast cancer had patients on adjuvant or neoadjuvant chemotherapy and/or endocrine treatment.
Evaluation of the association between biomarker levels and frailty occurred cross-sectionally in 11 studies (Figure 2). Two studies reported an evaluation of the association between the biomarker and frailty at multiple time points (26, 29). Buigues and authors (26) evaluated the association during treatment (six months or greater on treatment) and at one-year follow-up, notably, authors do not indicate one-year follow-up as post-treatment. Another study (29) evaluated the association of pre-treatment cell counts with pre-treatment frailty scores and an increase in cell counts from pre-treatment to four weeks or six months post-treatment with post-treatment frailty scores. Gilmore and authors (28) evaluated pre-treatment levels of cytokines as predictors of post-treatment frailty, but not at each time point.
Figure 2. Time-points for frailty and biomarker assessments. T-1 = pre-treatment (pre-surgery or post-surgery but pre-adjunctive therapy), T-2 a = during treatment (T-2 b = follow up since treatment beginning but not post-treatment), T-3 = 4 weeks post-treatment, T-4 = 6 months or greater post-treatment, BCa = breast cancer, PCa = prostate cancer, mix = mix solid tumors, Oca = ovarian cancer, CCa = colorectal cancer, B1 = cytokines, cytokine receptors, and acute phase proteins, B2 = molecules from complete blood count, lipid panel, or chemistry panel, B3 = glasgow prognostic score (GPS), B4 = micro RNAs, B5 = telomere length, B6 = metabolomics, F1 = physical frailty phenotype measured by fried frailty phenotype tool, F2 = deficit accumulation or geriatric vulnerability based frailty measured by geriatric assessment (GA) or GA domains (Balducci score, Leuven Oncogeriatric Frailty Score, Comprehensive Geriatric Assessment, Flemish Triage Risk Screening Tool, geriatric vulnerability score, Edmonton Frailty Scale, and Carolina Frailty Index). * = timeline is the same for cancer group with chemotherapy and without. ^control group with history of PCa, post-surgery or radiation therapy. Biomarker levels, frailty scores, and the association was measured between the two, pre-treatment biomarkers were associated with post-treatment frailty scores, biomarker levels and frailty scores were measured but did not evaluate the association between the two.
Frailty measurements varied greatly across the 14 studies. Fried frailty phenotype (FFP) was the most common instrument used (n = 6). The instrument’s prespecified criteria were applied across four studies (26, 31, 36, 38), where “frail” was defined as the presence of three or more components, “pre-frail” with one to two components, and “robust” with zero components (7). However, three studies used a modified version of the FFP, where two reports did not include unintended weight loss (28, 29) and one study replaced unintended weight loss with obesity (38).
Eight studies measured frailty as a deficit accumulation index or geriatric vulnerability scores using the Leuven Oncogeriatric Frailty Score (27, 32, 34), Balducci criteria (27, 30, 32, 34), Flemish Triage Risk Screening Tool (fTRST) (27), G8 (27), geriatric assessment domains (30, 31), the geriatric vulnerability score (23), the Edmonton frailty scale (35), and the Carolina frailty index (37). One study (23) used geriatric assessment domains that also included hypoalbuminemia and lymphopenia as two additional vulnerabilities calculated into the total geriatric vulnerability score. Two studies did not report which domains were assessed in comprehensive geriatric assessment (CGA) (33), Flemish Triage Risk Screening Tool, Balducci, or the G8 (27).
Four studies used multiple deficit accumulation frailty tools (Table 1). Two reports used the Balducci frailty category together with Leuven Oncogeriatric Frailty Score (32, 34); whereas, one study added the Flemish Triage Risk Screening Tool and G8, in addition to Balducci and Leuven Oncogeriatric Frailty Score (27). Although the frailty scores differed based on the instrument applied to either continuous scoring and/or frailty group categories, the authors reported frailty scores and their association with biomarkers across all the tools used (27, 31, 32, 34).
Peripherally circulating blood-based markers were measured across all 14 studies to evaluate their association with frailty. Only one report found no significant association with frailty in any of the markers measured (34). The statistically significant findings (p values < 0.05) identified in this review are presented below and categorized into six categories: cytokines/cytokine receptors and acute phase reactants; complete blood count; Glasgow Prognostic Score; microRNAs; telomere length; and metabolomics (Figure 3).
Figure 3. Potential biomarkers of frailty in solid tumors. IL, interleukin, GA, geriatric assessment, TNF-α, tumor necrosis factor-α, CRP, C-reactive protein, miRNA, micro RNA, GPS, glasgow prognostic score, sTNFR I, soluble TNF receptor I, NLR, neutrophil to lymphocyte ratio, WBC, white blood cells.
Cytokines, cytokine receptors, and acute phase reactants associated with frailty included: interleukin (IL)-6, IL-8, tumor necrosis factor (TNF)-α, soluble TNF receptor I (TNFR I), soluble TNFR II, C-reactive protein (CRP), and fibrinogen (Figure 3). Results are separated by frailty construct and time points for frailty measurements.
Pre-treatment levels of IL-6, TNF-α, and CRP were significantly associated with increased phenotypic frailty in colorectal tumors (31). Of note, while Gilmore and authors (28) measured pre-treatment levels of IL-6, the associations were tested with post-treatment frailty scores, therefore, the results are described below.
Higher levels of IL-6 were associated with higher phenotypic frailty in prostate cancer during androgen deprivation treatment (ADT) (26, 36) and at one-year follow-up (26). However, IL-6 and CRP were not associated with higher phenotypic frailty on six months of ADT in another prostate cancer cohort (38). While Buigues and authors (26) found that higher levels of IL-8 were associated with frailty at inclusion (six months or greater on ADT), IL-8 was no longer associated with frailty at one-year follow-up from inclusion. Similarly, another study (36) reported null findings during treatment, whose cohort had an average of 106 months from diagnosis. The two cohorts reported mixed findings on the association of fibrinogen with phenotypic frailty, where Navarro-Martinez and authors (36) found that higher levels of fibrinogen were associated with frailty, but Buigues and authors (26) reported null findings. Findings were also null for CRP, IL-1β, and TNF-α in these two prostate cancer cohorts (26, 36).
Of note, while Navarro-Martinez and authors (36) also included a non-cancer control group, the adjusted results with posthoc analysis were reported for the ADT group but not the control group, making their comparison challenging. Unadjusted higher levels of CRP, IL-6, and IL-8 were associated with greater frailty in the non-cancer control group.
Pre-treatment levels of IL-6, soluble TNFR I and II were significantly associated with four weeks post-treatment phenotypic frailty in the breast cancer group. Notably, no associations were found with any of the biomarkers in the age-matched non-cancer group (28).
In pre-treatment studies, IL-6 was significantly associated with increased deficit accumulation frailty (Balducci and Leuven Oncogeriatric Frailty Score) in breast cancer (32). In patients with colorectal cancer (31), authors reported increasing trends of IL-6 and CRP across stratified levels of deficit accumulation frailty (geriatric assessment domains) ranging from fit to frail. Authors also found higher levels of TNF-α in frail versus intermediate groups (31). However, no association between IL-6 or TNF-α and greater frailty (Balducci criteria) was found in mixed solid tumors (30).
Five studies investigated the association between markers of complete blood count and frailty (26, 29, 36–38).
At pre-treatment, greater total white blood cell (WBC) count, neutrophils, and neutrophil-lymphocyte ratio (NLR) were associated with phenotypic frailty in patients with breast cancer (29). However, hemoglobin was not associated with frailty in the same cohort (29).
During ADT, Buigues and authors (26) found that a higher lymphocyte count was associated with significant odds of being frail in patients six months or greater on ADT. In contrast, a lower lymphocyte count was associated with frailty progression at a one-year follow-up (26). In another prostate cancer cohort, lower hemoglobin was found in the ADT group compared to the non-ADT control group (38). The authors did not find a significant association with other cell markers. Similarly, total WBC, leukocyte counts, or hemoglobin did not predict frailty states in another study (36).
Pre-to post-treatment increases in WBC, neutrophils, and NLR predicted greater four-week post-treatment frailty in breast cancer, however, none of these markers were significant predictors of six months post-treatment frailty (29). Null findings were reported for hemoglobin or other cell markers in breast cancer post-treatment (29).
One study was found to evaluate complete blood count with deficit accumulation frailty at pre-treatment in mixed tumor types. Authors (37) found that greater NLR was associated with frailty (Carolina Frailty Index), however, they found null findings in total WBC or other cell counts.
Glasgow Prognostic Score (GPS), the ratio between CRP and albumin, was tested as a biomarker of frailty in two studies (30, 35). Both studies included patients with mixed solid tumors in the pre-treatment phase (30, 35) and found GPS 2 (elevated CRP and hypoalbuminemia) to be significantly associated with deficit accumulation frailty (Balducci criteria and Edmonton Frailty Scale).
Two studies evaluated microRNAs (miRNAs) as biomarkers of deficit accumulation frailty (Balducci, Leuven Oncogeriatric Frailty Score, Flemish Triage Risk Screening Tool, G8) in patients with breast cancer (27, 34) at pre-treatment. Dalmasso and authors (27) found that higher miR374a and lower miR-320b levels were associated with lower frailty using the Leuven Oncogeriatric Frailty Score and levels of miR-301a negatively correlated with frailty using Flemish Triage Risk Screening Tool scores. In addition, lower miR-106b, miR-191, miR-320b, and higher miR-374a emerged as independent predictors of deficit accumulation frailty using G8 (27). In comparison, Hatse and authors (34) reported null findings for 15 evaluated miRNAs and deficit accumulation frailty (Balducci, Leuven Oncogeriatric Frailty Scores).
Two studies evaluated the relationship between telomere length and deficit accumulation frailty (Balducci, Leuven Oncogeriatric Frailty Score, geriatric vulnerability score) at pre-treatment (23, 32). In patients with ovarian cancer, shorter telomere length was associated with a geriatric vulnerability score ≥3 (23). However, findings were null in patients with breast cancer (32).
The search yielded only one study that evaluated a metabolomic profile of different amino acids, acylcarnitines, and phospholipids as biomarkers of deficit accumulation frailty (comprehensive geriatric assessment) in patients with breast cancer (33). The authors found greater age-adjusted ß3-methyl-hystidine levels in unfit and frail groups compared to the fit group. Similarly, they found depletion of several sphingolipids and glycerol-phospholipids in unfit and frail groups compared to fit (Table 1).
The risk of bias and quality assessment results are presented in Table 2. Interrater reliability for cross-sectional and cohort studies between the two reviewers was 83 and 67% for the case–control study. Six studies were rated as good (23, 26, 27, 29–31), while the remaining eight were rated as fair. Several areas of potential bias in this body of literature were identified: participant sampling procedures, power analyses, measurement biases, instrumentation, and statistical methods. Most of the cohort studies (12/13) reported selecting participants during the same period and applying inclusion criteria uniformly (23, 26–35, 37). One study selected prostate cancer group undergoing ADT and the control group from nursing home facilities, thus the two groups differed in diagnosis, active treatment, clinical setting and therefore were rated as dissimilar or “no” for the criterion on sampling methodology (question 4) (36).
None of the included studies reported sample size justification through power analysis. Among the observational longitudinal cohorts, four studies (23, 28, 30, 31) measured biomarkers at only one-time points, while reporting longitudinal outcomes such as survival. Thus, these four studies received a “no” rating for the repeated exposure measurement criterion. Among the evaluation of outcome (frailty), over half of the reports either did not use previously validated cut-off scores or modified existing tools without prior validation. All studies were either missing blinding procedures or failed to report them, thus potential risk for bias could not be determined. Only seven studies (23, 26–30, 37) controlled for confounders through multivariate analyses. Lack of multivariate analyses may introduce potential confounding bias in overestimation or underestimation of markers’ impact on frailty. In the single case-control study, the investigators did not provide sample size justification or blinding procedures (38). The investigators also did not specify if concurrent controls were used or if 100% of eligible cases were recruited, thus, it was unclear if participants in the control group were recruited at the same time as cases. Measures of association or effect sizes were not reported or partially reported in seven included studies (28, 31–33, 35, 36, 38; Supplementary Table S4).
In our review evaluating biomarkers of frailty in solid tumors, we identified IL-6, NLR, and GPS 2 as potential biomarkers of frailty found across two or more studies. To our knowledge, this is the first systematic review evaluating existing biomarkers of frailty in patients with solid tumors. While the inclusion criteria included all solid tumors, the search yielded findings in breast, prostate, mixed solid tumors, ovarian, and colorectal cancers with no studies identified in brain, pancreatic, lung, or other solid organ cancers. The included studies used two distinct frailty constructs, phenotypic frailty and deficit accumulation, which are described in prior literature (10, 39, 40). These distinct frailty paradigms make synthetization challenging. We found that biomarkers were most frequently evaluated and associated with phenotypic and deficit accumulation frailty at pre-treatment although associations were found across the cancer continuum.
Inflammatory molecules were most frequently measured and significantly associated with phenotypic and deficit accumulation frailty, on par with prior reviews that evaluated biomarkers of frailty primarily in older individuals with mixed diagnoses (15–18). Cytokines, cytokine receptors, and acute phase reactants were among the most commonly measured, perhaps due to their role as modulators of cell-to-cell communication in inflammatory responses and cancer biology (41, 42).
Five studies reported elevated levels of IL-6, a pleiotropic pro-inflammatory cytokine, in patients with higher phenotypic and deficit accumulation frailty across the breast, prostate, and colorectal tumors (26, 28, 31, 32, 36). Elevated levels of IL-6 have been documented in aging, cancer progression, and the development of cancer cachexia (43). Moreover, IL-6 can be elevated in both acute and chronic immune responses by exerting stimulatory effects on T and B cells and producing acute-phase reactants (44). Included studies reported higher levels of IL-6 associated with phenotypic and deficit accumulation frailty evaluated at pre-treatment, during treatment, and four weeks post-treatment. However, two studies reported null findings: six months on ADT with phenotypic frailty (38) and with pre-treatment deficit accumulation frailty (30). Bylow and authors (38) did not find significance when comparing their ADT group (more frail group) to their non-ADT group (less frail group), which suggested that ADT-associated frailty may not be related to circulating increases in IL-6. Harneshaug and authors (30) found a significant association with pre-treatment deficit accumulation frailty, but the findings were null after adjustment for confounders. That coupled with the absence of multivariate analyses in the studies with positive findings (31, 32), suggests elevated IL-6 may be related to the clinical confounders and analytical adjustments are necessary to parse the relationships. IL-6, as a multifaceted cytokine, has been shown to be elevated in chronic inflammatory states such as aging, cancer, obesity (43, 45) and plays a role in underlying pathology of worsening disease states (18). We hypothesize that elevated levels of IL-6 in worsening frailty may be explained by a greater number of inflammation related symptoms and conditions (18).
IL-8, a pro-inflammatory chemokine, was evaluated in two of the studies (26, 36) and found to serve as a correlate of frailty during treatment (six months or greater on ADT), but not at one-year follow-up (26). In contrast, null findings were reported during treatment in another prostate cancer group (36). Although both studies (26, 36) studied IL-8 and phenotypic frailty during ADT, their discrepant findings may be owed to their analytical methods: namely, post-hoc statistical adjustment versus multivariate regression. Additionally, Navarro-Martinez and authors (36) did not report a list of variables included in the multinomial regression which made it difficult to compare to Buigues and authors (26). Thus, although IL-8 has been postulated to rise during ADT (46), the evidence remains inconclusive and is limited by these two studies with varying methods and small sample sizes (26, 36). A possible explanation for the association between IL-8 and frailty could be that frail individuals may be more susceptible to acute inflammatory response during treatment, which may manifest as reduced physical activity and increased frailty symptomology (2).
TNF-α was evaluated in four reports (26, 30, 31, 36) and found to associate with pre-treatment phenotypic and deficit accumulation frailty in colorectal cancer (31). The associations were null in pre-treatment deficit accumulation in mixed tumors (30) or during treatment with phenotypic frailty in prostate tumors (26, 36). The incongruencies for phenotypic frailty may relate to the heterogeneity in tumor types and time from treatment: pre-treatment (31) versus during treatment (26, 36). Findings were also incongruent for pre-treatment deficit accumulation frailty, where one study (31) found higher levels of TNF-α in the frail group, but another (30) had null findings after adjustment for confounding variables in the multivariate analysis. Importantly, the study by Ronning and authors (31) lacked multivariate adjustments altogether. Soluble TNFR I and II, members of the TNF superfamily, were measured only in one study with post-treatment phenotypic frailty, and findings, albeit significant, are exploratory and thus warrant additional corroborations (28). Thus, the relationships between phenotypic and deficit accumulation frailty severity and TNF-α, soluble TNFR I and II remain unclear.
Consistent with a previous meta-analysis of frailty biomarkers in primarily non-cancer diagnoses of older adults (18), CRP and fibrinogen emerged as correlates of phenotypic and deficit accumulation frailty at pre-treatment (31) and with phenotypic frailty during treatment (36). Importantly, CRP was not significant in three studies of patients with prostate tumors on ADT (26, 36, 38), whereas fibrinogen was not significant in one report (26). The finding by Ronning and authors (31) of elevated pre-treatment CRP in frail groups may correlate with tumor-mediated inflammatory response (47). However, further extrapolation would yield ambiguous conclusions, given the cross-sectional time points and lack of pre-treatment levels for comparison across all four reports. Collectively, findings for IL-6, IL-8, TNF-α, CRP, and fibrinogen suggest that higher levels of pro-inflammatory cytokines and acute phase reactants may play a role in frailty states in patients with solid tumors. Increased levels of inflammation markers may be related to cancer and its treatment effects on frail and pre-frail cancer survivors. Additionally, although we did not restrict the age of the participants for the inclusion criteria in this review, the average age across 14 studies was 72 years. Older age has a linear relationship with low grade chronic inflammation and is subsequently associated with increased comorbidity and higher vulnerability to disease, which may, in turn, be manifested as frailty signs/symptoms such as weakness, decreased physical activity, and exhaustion (16, 18).
Perturbations in neutrophils, lymphocytes, total WBC, and NLR may be related to both tumor promoting and immune suppressive roles associated with poor outcomes in solid tumors (48–52). Across the five studies that evaluated markers of complete blood counts, NLR, a quotient of neutrophil and lymphocyte counts, emerged as a significant predictor of pre-treatment and post-treatment phenotypic frailty in breast cancer (29) and pre-treatment deficit accumulation frailty in mixed tumor types (37). High NLR has been shown to associate with greater phenotypic and deficit accumulation frailty in cancer survivors, patients with cardiovascular disease, and community dwelling older adults (15). Notably, the study by Gilmore and authors (29) found associations between increased NLR, total WBC, neutrophils and frailty scores pre-chemotherapy and four weeks post-chemotherapy; however these markers and frailty scores returned to baseline six months post treatment. We hypothesize the observed elevations in NLR, total WBC, neutrophils and their association with increased frailty symptomology may be related to an acute inflammatory response to cancer pathology and treatment effects.
Higher lymphocyte levels were associated with phenotypic frailty during treatment in patients on ADT six months or greater prior to inclusion; however, when evaluating progression to frailty at one year follow-up, lower lymphocyte levels associated with the likelihood of being frail (26). The discrepancy may relate to the frailty scores at inclusion versus one year follow-up, reflecting the long-term effect of ADT on frailty progression and the potential effect on lymphopoiesis (53). Additionally, decreased physical activity (a component of frailty phenotype) was previously reported to be associated with lower lymphocyte counts, whereas increased physical activity was associated with higher lymphocyte counts. Prior scoping review also documented an association between lower lymphocyte counts in the presence of frailty (15). Lymphocyte counts did not associate with phenotypic frailty pre-or post-treatment in the breast (29) or pre-treatment deficit accumulation in mixed solid tumors (37). The discrepant findings across the three studies may be related to heterogeneity in the types of solid tumors and frailty definitions.
Hemoglobin, a marker of anemia, was evaluated in three studies and was found to be associated with phenotypic frailty in patients with prostate tumors six months on ADT (38). However, this association was not corroborated by the other two reports with phenotypic frailty before and during treatment in neither prostate nor breast tumors (29, 36). The association found by Bylow and authors (38) may relate to the inverse relationship between androgen deprivation treatment and hemoglobin levels, where treatment may cause decline in hemoglobin (53). ADT-related lower hemoglobin (i.e., anemia) has been associated with symptoms such as fatigue and decreased activity (53), thus, it is plausible that lower hemoglobin in the study by Bylow and authors (38) may be related to the exhaustion and decreased physical activity symptoms/components of the phenotypic frailty.
GPS, the ratio between CRP and albumin, has been extensively validated as a biomarker of poor prognosis in cancer (54). GPS includes scores of 0, 1, 2, with scores ≥2 signifying both hypoalbuminemia (<35 g/L) and elevated CRP levels (>10 mg/L) (54). While CRP is a pro-inflammatory molecule, hypoalbuminemia reflects poor nutritional status associated with increased mortality in patients with cancer (55). In this review, two reports found GPS 2 to significantly associate with deficit accumulation frailty at pre-treatment with moderate to excellent specificity (30, 35). Previously, GPS 2 was shown to associate with cancer-related cachexia, weight loss, and poor performance status (54, 56); however, the two reports which evaluated frailty with GPS in the present review did not measure weight loss. Additionally, pronounced inflammatory response induces hypoalbuminemia (57), and the aging process, itself has been linked to lower levels of albumin (58). Because the patients included in the aforementioned reports were >70 years of age with mixed solid tumors, stages, and treatments (30, 35), we hypothesize that GPS 2 (i.e., elevated CRP and hypoalbuminemia) may be related to the physiological processes underlying cancer, aging, and geriatric vulnerabilities which comprised the deficit accumulation frailty scores.
Epigenetic alterations are another hallmark of aging (11) and are causally related to miRNA dysregulations in cancer (59). Among the reports included, two studies evaluated aging-related miRNAs as molecular correlates of pre-treatment deficit accumulation frailty. Dalmasso and authors (27) found an association between higher levels of aging-related miR-320b and higher frailty using the Leuven Oncogeriatric Frailty Score (LOFS) but not with the Balducci score. They also report an inverse relationship with G8 scores and miR-106b, miR-191, and miR320b, suggesting lower levels are associated with higher scores. Given the established link between the miRNAs with aging process (11) and their dysregulation in cancer biology (59), we hypothesize the exploratory findings reported by Dalmasso and colleagues (27) may be related to the older age of participants included (median age > 74 years), cancer biology, and amalgamation of geriatric deficits comprising LOFS and G8. In contrast, a report by Hatse and authors (34) did not find these associations in a smaller cohort of older frail (n = 10) patients with breast cancer. The validation study by Hatse and authors (34) was used as pilot validation cohort and nonsignificant findings in relation to frailty may relate to the smaller sample size. Additional studies are warranted to further extrapolate relationship between aging miRNAs and phenotypic/deficit accumulation frailty phenotypes.
Telomere length also associates with pre-treatment deficit accumulation frailty. Telomeres are nucleoprotein structures located at the chromosomal ends and telomere length attrition is attributed to telomerase deficiency and lack of DNA repair (11, 60). Telomere dysfunction, linked to cell senescence, apoptosis (11), and tissue inflammation, gives rise to diseases with inflammatory components such as cancer (60). While shorter telomere length was associated with greater pre-treatment deficit accumulation frailty in patients with ovarian cancer (23), findings were null in patients with breast cancer (32). This discrepancy may be due to the varying geriatric domains that comprise the geriatric vulnerability score (23), Baducci, and the Leuven Oncogeriatric Frailty Score (32). Given the previously established bidirectional link between inflammation and telomere attrition (61), it is plausible that the shorter telomere length found in the ovarian cancer cohort (23) relates to inflammation and hypoalbuminemia components of GVS. Conversely, shortened telomere length may also relate to differences in stages of cancer: stages I–III in the breast cancer cohort (32) compared to stages III–IV in the ovarian cancer cohort (23). The evidence presented here does not support the extrapolation of the link between shorter telomere length and frailty state in solid tumors. Additional studies investigating telomere capacity as biomarkers of frailty are needed to compare frail versus non-frail cohorts with similar age, disease, and treatment before this finding can be confirmed.
Only one study incorporated a global approach by using metabolomics to investigate a comprehensive profile of amino acids, acylcarnitines, and phospholipids in association with pre-treatment deficit accumulation frailty (33). Metabolomics is a powerful tool that enables researchers to profile endogenous metabolites and metabolic pathways underlying disease (62, 63). Researchers propose that metabolomics may capture the multifactorial frailty profiles (63). Corona and authors (33) found that age-adjusted 3-methylhistidine (3MHis) was elevated and levels of sphingolipids and glycerophospholipids were decreased in frail patients with breast cancer. Higher 3MHis relates to skeletal muscle loss observed with older age (64) in healthy adults, whereas the dysregulation of sphingolipids and glycerophospholipids relates to the progression of metabolic disease (65). A recent study evaluating the metabolomic profile of frailty phenotype in healthy older adults stratified by gender identified modulators of prefrailty phosphatidylglycerol (26:1) and dimethyloxazale for men and threonine, fructose, mannose, dihydroxyphenyl acetic acid, and 2,4-aminobutyric acid for women (66). While the metabolites in the two studies differed, the metabolomics results suggest perturbations in the metabolites may be associated with frailty, but further validation in each solid tumor type is needed.
Interpreting these results requires caution due to several limitations. First, the studies’ frailty instruments measured different constructs of frailty, including phenotypic versus deficit accumulation frailty. Our findings here highlight variations in the constructs, operationalization, and instruments used to assess frailty, of which some were validated. These issues are echoed by findings from previous reviews (40, 67) and a clinician survey (68) of limited validity across instruments and different operationalizations of the frailty concept. Modification of existing tools and lack of validity and reliability support for novel tools collectively threaten the internal and external validity of findings in this body of literature.
Second, great heterogeneity in analysis was found across studies. While some reports incorporated multiple logistic regression, others used bivariate correlations and tests by three groups (e.g., Kruskal-Wallis) to draw associations between the molecular correlates and frailty scores. We found that several studies did not report multiple comparison corrections and adjustments for significant covariates, which would introduce type II error and the potential for multicollinearity. The variation in statistical approach makes it difficult to synthesize findings across studies.
Third, included studies did not report power analyses, although the majority reported smaller sample sizes. This indicates that the evidence is, at this point, largely exploratory and warrants larger corroborative investigations. Moreover, only half the included studies reported measures of association/effect sizes for statistically significant results, which limits our ability to comment on clinically meaningful effect. Future investigations would benefit from reporting effect size calculations to better inform science of biomarker discovery for frailty phenotypes. Fourth, molecule selections were often limited to a few nonspecific markers of inflammation. This reflects the state of science in biomarker development for frailty. Fifth, most of the included studies lacked control groups (i.e., non-cancer or healthy controls), thus it was challenging to determine the strength of association with frailty in the absence of solid tumors and treatments. In addition, IL-6, TNF-α, and CRP are repeatedly found to be elevated in a myriad of conditions linked to inflammation, such as obesity and smoking (45, 69). Therefore, future studies should include these relevant health characteristics as covariates in biomarker discovery studies. Additionally, there was heterogeneity in the type of treatments received among studies during treatment and/or post-treatment. Future studies may benefit from comparing the effects of different treatment types and modalities on frailty profiles and biomarker oscillation. Lastly, current literature lacks stratification by sex, race, and ethnicity, which decreases the generalizability and specificity of the results, and may also hinder our progress in developing targeted interventions.
In summary, IL-6, NLR, and GPS 2 emerged as potential biomarkers of frailty found in two or more of the included studies (Figure 3). Although IL-6 emerged as potential biomarker in five out of seven reports that measured this cytokine, findings remain inconsistent. Findings are inconclusive and were limited by number of reports found for all other measures. Our findings show that the current literature employs varying conceptual definitions of and instruments measuring frailty and that the genesis of frailty in solid tumors may be multifactorial, impacted by time since cancer diagnosis, treatments, and unique biology of individual solid tumors. Our findings highlight a need for further instrument validations and clear conceptual and operational definitions of frailty within the oncology field. Only two reports evaluated associations with biomarkers longitudinally. These two reports found that higher levels of inflammatory markers may serve as predictors of phenotypic frailty four weeks post-treatment (29) or at one year follow-up in patients with prostate cancer on ADT (26), however, further investigations are warranted with longer follow-up times. Post-treatment phenotypic frailty was captured four weeks (28, 29) and six months post-treatment (29), without data for pre-treatment (28) or during treatment (28, 29). The evidence highlights a substantial gap in long-term survivorship and frailty biomarkers evaluated longitudinally from pre-treatment to months and years post-treatment.
Collectively, the reports included in this review suggest that inflammatory pathways related to the proliferation of immune cells at the time of diagnosis and treatment are associated with frailty development and symptomology. Limited reports (one each) also implicate telomere shortening and epigenetic alterations such as perturbations in aging miRNAs as potential correlates of deficit accumulation frailty. Additionally, metabolic pathways underlying deficit accumulation frailty may be of potential value when identifying target biomarkers. Given the paucity of evidence across the diverse set of biomarkers searched, the field of frailty biomarkers in solid tumors is largely underexplored. Future studies will benefit from longitudinal studies with a comprehensive set of biomarkers adjusted for cancer stages, time since diagnosis and treatment, and type of treatment; larger sample sizes, robust control groups, and multiple time points by sex, gender, and race/ethnicity. Such investigations will aid the development of robust biomarker profiles, early identification of cancer survivors at risk for developing frailty, and timely referral to therapeutic interventions.
DS and TA: conceptualization. DS, BP, DC, and TA: data curation and methodology. DS: formal analysis, project administration, and visualization. DS and BP: investigation and validation. TA: supervision. TA and DC: resources. DS, BP, VG, JG, and TA: writing—original draft. DS, BP, VG, DC, JG, and TA: writing—review and editing. All authors contributed to the article and approved the submitted version.
This research was supported by the Intramural Research Program of the National Cancer Institute, National Institutes of Health.
Our research team would like to thank Nancy Touchette, Ph.D., National Institutes of Health Office of Intramural Training & Education writer and editor, for manuscript editing assistance.
The authors declare that the research was conducted in the absence of any commercial or financial relationships that could be construed as a potential conflict of interest.
All claims expressed in this article are solely those of the authors and do not necessarily represent those of their affiliated organizations, or those of the publisher, the editors and the reviewers. Any product that may be evaluated in this article, or claim that may be made by its manufacturer, is not guaranteed or endorsed by the publisher.
The Supplementary material for this article can be found online at: https://www.frontiersin.org/articles/10.3389/fpubh.2023.1171243/full#supplementary-material
1. Hurria, A, Jones, L, and Muss, HB. Cancer treatment as an accelerated aging process: assessment, biomarkers, and interventions. Am Soc Clin Oncol Educ Book. (2016) 35:e516–22. doi: 10.1200/EDBK_156160
2. Guida, JL, Ahles, TA, Belsky, D, Campisi, J, Cohen, HJ, DeGregori, J, et al. Measuring aging and identifying aging phenotypes in cancer survivors. JNCI. (2019) 111:1245–54. doi: 10.1093/jnci/djz136
3. Henderson TONess, KK, and Cohen, HJ. Accelerated aging among cancer survivors: from pediatrics to geriatrics. Am Soc Clin Oncol Educ Book. (2014) 34:e423–30. doi: 10.14694/EdBook_AM.2014.34.e423
4. Hayek, S, Gibson, TM, Leisenring, WM, Guida, JL, Gramatges, MM, Lupo, PJ, et al. Prevalence and predictors of frailty in childhood cancer survivors and siblings: a report from the childhood cancer survivor study. J Clin Oncol. (2020) 38:232–47. doi: 10.1200/JCO.19.01226
5. Ness, KK, and Wogksch, MD. Frailty and aging in cancer survivors. Transl Res. (2020) 221:65–82. doi: 10.1016/j.trsl.2020.03.013
6. Feliciano, EMC, Hohensee, C, Rosko, AE, Anderson, GL, Paskett, ED, Zaslavsky, O, et al. Association of prediagnostic frailty, change in frailty status, and mortality after cancer diagnosis in the women’s health initiative. JAMA Netw Open. (2020) 3:e2016747. doi: 10.1001/jamanetworkopen.2020.16747
7. Fried, LP, Tangen, CM, Walston, J, Newman, AB, Hirsch, C, Gottdiener, J, et al. Frailty in older adults: evidence for a phenotype. J Gerontol Ser A Biol Med Sci. (2001) 56:M146–57. doi: 10.1093/gerona/56.3.m146
8. Song, X, Mitnitski, A, and Rockwood, K. Prevalence and 10-year outcomes of frailty in older adults in relation to deficit accumulation. J Am Geriatr Soc. (2010) 58:681–7. doi: 10.1111/j.1532-5415.2010.02764.x
9. Cesari, M, Gambassi, G, Abellan van Kan, G, and Vellas, B. The frailty phenotype and the frailty index: different instruments for different purposes. Age Ageing. (2014) 43:10–2. doi: 10.1093/ageing/aft160
10. Kuchel, GA. Frailty and resilience as outcome measures in clinical trials and geriatric care: are we getting any closer? J Am Geriatr Soc. (2018) 66:1451–4. doi: 10.1111/jgs.15441
11. López-Otín, C, Blasco, MA, Partridge, L, Serrano, M, and Kroemer, G. The hallmarks of aging. Cells. (2013) 153:1194–217. doi: 10.1016/j.cell.2013.05.039
12. Alfano, CM, Peng, J, Andridge, RR, Lindgren, ME, Povoski, SP, Lipari, AM, et al. Inflammatory cytokines and comorbidity development in breast cancer survivors versus noncancer controls: evidence for accelerated aging? J Clin Oncol. (2017) 35:149–56. doi: 10.1200/JCO.2016.67.1883
13. Sanoff, HK, Deal, AM, Krishnamurthy, J, Torrice, C, Dillon, P, Sorrentino, J, et al. Effect of cytotoxic chemotherapy on markers of molecular age in patients with breast cancer. J Natl Cancer Inst. (2014) 106:1–8. doi: 10.1093/jnci/dju057
14. Lee, J-J, Nam, C-E, Cho, S-H, Park, K-S, Chung, I-J, and Kim, H-J. Telomere length shortening in non-Hodgkin's lymphoma patients undergoing chemotherapy. Ann Hematol. (2003) 82:492–5. doi: 10.1007/s00277-003-0691-4
15. Navarro-Martínez, R, and Cauli, O. Lymphocytes as a biomarker of frailty syndrome: a scoping review. Diseases. (2021) 9:53. doi: 10.3390/diseases9030053
16. Picca, A, Coelho-Junior, HJ, Calvani, R, Marzetti, E, and Vetrano, DL. Biomarkers shared by frailty and sarcopenia in older adults: a systematic review and meta-analysis. Ageing Res Rev. (2022) 73:101530. doi: 10.1016/j.arr.2021.101530
17. Ramakrishnan, P, Alyousefi, N, Abdul-Rahman, P, Kamaruzzaman, S, Chin, A, and Tan, M. A systematic review of studies comparing potential biochemical biomarkers of frailty with frailty assessments. Eur Geriatr Med. (2017) 8:397–407. doi: 10.1016/j.eurger.2017.07.010
18. Soysal, P, Stubbs, B, Lucato, P, Luchini, C, Solmi, M, Peluso, R, et al. Inflammation and frailty in the elderly: a systematic review and meta-analysis. Ageing Res Rev. (2016) 31:1–8. doi: 10.1016/j.arr.2016.08.006
19. Ho, Y-W, Tang, W-R, Chen, S-Y, Lee, S-H, Chen, J-S, Hung, Y-S, et al. Association of frailty and chemotherapy-related adverse outcomes in geriatric patients with cancer: a pilot observational study in Taiwan. Aging. (2021) 13:24192–204. doi: 10.18632/aging.203673
20. Ness, KK, Krull, KR, Jones, KE, Mulrooney, DA, Armstrong, GT, Green, DM, et al. Physiologic frailty as a sign of accelerated aging among adult survivors of childhood cancer: a report from the St Jude Lifetime cohort study. J Clin Oncol. (2013) 31:4496–503. doi: 10.1200/JCO.2013.52.2268
21. Page, MJ, McKenzie, JE, Bossuyt, PM, Boutron, I, Hoffmann, TC, Mulrow, CD, et al. The prisma 2020 statement: an updated guideline for reporting systematic reviews. Int J Surg. (2021) 88:105906. doi: 10.1136/bmj.n71
22. NHLBI. Study Quality Assessment Tools (2021) (cited 2022). Available at: https://www.nhlbi.nih.gov/health-topics/study-quality-assessment-tools.
23. Falandry, C, Horard, B, Bruyas, A, Legouffe, E, Cretin, J, Meunier, J, et al. Telomere length is a prognostic biomarker in elderly advanced ovarian cancer patients: a multicenter Gineco study. Aging. (2015) 7:1066–74. doi: 10.18632/aging.100840
24. Balducci, L, and Extermann, M. Management of the frail person with advanced cancer. Crit Rev Oncol Hematol. (2000) 33:143–8. doi: 10.1016/s1040-8428(99)00063-3
25. Brouwers, B, Hatse, S, Dal Lago, L, Neven, P, Vuylsteke, P, Dalmasso, B, et al. The impact of adjuvant chemotherapy in older breast cancer patients on clinical and biological aging parameters. Oncotarget. (2016) 7:29977–88. doi: 10.18632/oncotarget.8796
26. Buigues, C, Navarro-Martínez, R, Sánchez-Martínez, V, Serrano-Carrascosa, M, Rubio-Briones, J, and Cauli, O. Interleukin-6 and lymphocyte count associated and predicted the progression of frailty syndrome in prostate cancer patients undergoing antiandrogen therapy. Cancers. (2020) 12:1716. doi: 10.3390/cancers12071716
27. Dalmasso, B, Hatse, S, Brouwers, B, Laenen, A, Berben, L, Kenis, C, et al. Age-related micrornas in older breast cancer patients: biomarker potential and evolution during adjuvant chemotherapy. BMC Cancer. (2018) 18:1–15. doi: 10.1186/s12885-018-4920-6
28. Gilmore, N, Kadambi, S, Lei, L, Loh, KP, Mohamed, M, Magnuson, A, et al. Associations of inflammation with frailty in patients with breast cancer aged 50 and over receiving chemotherapy. J Geriatr Oncol. (2020) 11:423–30. doi: 10.1016/j.jgo.2019.04.001
29. Gilmore, N, Mohile, S, Lei, L, Culakova, E, Mohamed, M, Magnuson, A, et al. The longitudinal relationship between immune cell profiles and frailty in patients with breast cancer receiving chemotherapy. Breast Cancer Res. (2021) 23:19–1. doi: 10.1186/s13058-021-01388-w
30. Harneshaug, M, Kirkhus, L, Benth, JŠ, Grønberg, BH, Bergh, S, Whist, JE, et al. Screening for frailty among older patients with cancer using blood biomarkers of inflammation. J Geriatr Oncol. (2019) 10:272–8. doi: 10.1016/j.jgo.2018.07.003
31. Rønning, B, Wyller, TB, Seljeflot, I, Jordhøy, MS, Skovlund, E, Nesbakken, A, et al. Frailty measures, inflammatory biomarkers and post-operative complications in older surgical patients. Age Ageing. (2010) 39:758–61. doi: 10.1093/ageing/afq123
32. Brouwers, B, Dalmasso, B, Hatse, S, Laenen, A, Kenis, C, Swerts, E, et al. Biological aging and frailty markers in breast cancer patients. Aging. (2015) 7:319–33. doi: 10.18632/aging.100745
33. Corona, G, Polesel, J, Fratino, L, Miolo, G, Rizzolio, F, Crivellari, D, et al. Metabolomics biomarkers of frailty in elderly breast cancer patients. J Cell Physiol. (2014) 229:898–902. doi: 10.1002/jcp.24520
34. Hatse, S, Brouwers, B, Dalmasso, B, Laenen, A, Kenis, C, Schöffski, P, et al. Circulating micrornas as easy-to-measure aging biomarkers in older breast cancer patients: correlation with chronological age but not with fitness/frailty status. PLoS One. (2014) 9:e110644. doi: 10.1371/journal.pone.0110644
35. Lealdini, V, Trufelli, DC, da Silva, FBF, Normando, SRC, Camargo, EW, de Matos, LL, et al. Applicability of modified glasgow prognostic score in the assessment of elderly patients with cancer: a pilot study. J Geriatr Oncol. (2015) 6:479–83. doi: 10.1016/j.jgo.2015.09.001
36. Navarro-Martínez, R, Serrano-Carrascosa, M, Buigues, C, Fernández-Garrido, J, Sánchez-Martínez, V, Castelló-Domenech, AB, et al. Frailty syndrome is associated with changes in peripheral inflammatory markers in prostate cancer patients undergoing androgen deprivation therapy. Urol Oncol. (2019) 37:976–87. doi: 10.1016/j.urolonc.2019.08.005
37. Nishijima, TF, Deal, AM, Williams, GR, Guerard, EJ, Nyrop, KA, and Muss, HB. Frailty and inflammatory markers in older adults with cancer. Aging. (2017) 9:650–64. doi: 10.18632/aging.101162
38. Bylow, K, Hemmerich, J, Mohile, SG, Stadler, WM, Sajid, S, and Dale, W. Obese frailty, physical performance deficits, and falls in older men with biochemical recurrence of prostate cancer on androgen deprivation therapy: a case-control study. Urology. (2011) 77:934–40. doi: 10.1016/j.urology.2010.11.024
39. Huisingh-Scheetz, M, and Walston, J. How should older adults with cancer be evaluated for frailty? J Geriatr Oncol. (2017) 8:8–15. doi: 10.1016/j.jgo.2016.06.003
40. Dent, E, Kowal, P, and Hoogendijk, EO. Frailty measurement in research and clinical practice: a review. Eur J Intern Med. (2016) 31:3–10. doi: 10.1016/j.ejim.2016.03.007
41. O’Shea, JJ, Gadina, M, and Siegel, RM. Cytokines and cytokine receptors. Clin Immunol Elsevier. (2019):127–155.e1. doi: 10.1016/B978-0-7020-6896-6.00009-0
42. Pang, WW, Abdul-Rahman, PS, Wan-Ibrahim, WI, and Hashim, OH. Can the acute-phase reactant proteins be used as cancer biomarkers? Int J Biol Markers. (2010) 25:1–11. doi: 10.1177/172460081002500101
43. Brábek, J, Jakubek, M, Vellieux, F, Novotný, J, Kolář, M, Lacina, L, et al. Interleukin-6: molecule in the intersection of cancer, aging and COVID-19. Int J Mol Sci. (2020) 21:7937. doi: 10.3390/ijms21217937
44. Gabay, C. Interleukin-6 and chronic inflammation. Arthritis Res Ther. (2006) 8:S3–6. doi: 10.1186/ar1917
45. Khaodhiar, L, Ling, PR, Blackburn, GL, and Bistrian, BR. Serum levels of interleukin-6 and C-reactive protein correlate with body mass index across the broad range of obesity. J Parenter Enter Nutr. (2004) 28:410–5. doi: 10.1177/0148607104028006410
46. Lopez-Bujanda, ZA, Haffner, MC, Chaimowitz, MG, Chowdhury, N, Venturini, NJ, Patel, RA, et al. Castration-mediated Il-8 promotes myeloid infiltration and prostate cancer progression. Nat Cancer. (2021) 2:803–18. doi: 10.1038/s43018-021-00227-3
47. Hart, PC, Rajab, IM, Alebraheem, M, and Potempa, LA. C-reactive protein and cancer—diagnostic and therapeutic insights. Front Immunol. (2020) 11:595835. doi: 10.3389/fimmu.2020.595835
48. Howard, R, Kanetsky, PA, and Egan, KM. Exploring the prognostic value of the neutrophil-to-lymphocyte ratio in cancer. Sci Rep. (2019) 9:1–10. doi: 10.1038/s41598-019-56218-z
49. Coffelt, SB, Kersten, K, Doornebal, CW, Weiden, J, Vrijland, K, Hau, C-S, et al. Il-17-producing γδ t cells and neutrophils conspire to promote breast cancer metastasis. Nature. (2015) 522:345–8. doi: 10.1038/nature14282
50. Ocana, A, Nieto-Jiménez, C, Pandiella, A, and Templeton, AJ. Neutrophils in cancer: prognostic role and therapeutic strategies. Mol Cancer. (2017) 16:1–7. doi: 10.1186/s12943-017-0707-7
51. Zhao, J, Huang, W, Wu, Y, Luo, Y, Wu, B, Cheng, J, et al. Prognostic role of pretreatment blood lymphocyte count in patients with solid tumors: a systematic review and meta-analysis. Cancer Cell Int. (2020) 20:15–4. doi: 10.1186/s12935-020-1094-5
52. Weng, M, Zhao, W, Yue, Y, Guo, M, Nan, K, Liao, Q, et al. High preoperative white blood cell count determines poor prognosis and is associated with an immunosuppressive microenvironment in colorectal cancer. Front Oncol. (2022) 12:1–15. doi: 10.3389/fonc.2022.943423
53. Grossmann, M, and Zajac, JD. Hematological changes during androgen deprivation therapy. Asian J Androl. (2012) 14:187–92. doi: 10.1038/aja.2011.102
54. McMillan, DC. The systemic inflammation-based glasgow prognostic score: a decade of experience in patients with cancer. Cancer Treat Rev. (2013) 39:534–40. doi: 10.1016/j.ctrv.2012.08.003
55. Ñamendys-Silva, SA, González-Herrera, MO, Texcocano-Becerra, J, and Herrera-Gómez, A. Hypoalbuminemia in critically ill patients with cancer: incidence and mortality. Am J Hosp Palliative Med. (2011) 28:253–7. doi: 10.1177/1049909110384841
56. Dolan, RD, and McMillan, DC. The prevalence of cancer associated systemic inflammation: implications of prognostic studies using the glasgow prognostic score. Crit Rev Oncol Hematol. (2020) 150:102962. doi: 10.1016/j.critrevonc.2020.102962
57. Soeters, PB, Wolfe, RR, and Shenkin, A. Hypoalbuminemia: pathogenesis and clinical significance. J Parenter Enter Nutr. (2019) 43:181–93. doi: 10.1002/jpen.1451
58. Gomi, I, Fukushima, H, Shiraki, M, Miwa, Y, Ando, T, Takai, K, et al. Relationship between serum albumin level and aging in community-dwelling self-supported elderly population. J Nutr Sci Vitaminol. (2007) 53:37–42. doi: 10.3177/jnsv.53.37
59. Suzuki, H, Maruyama, R, Yamamoto, E, and Kai, M. Epigenetic alteration and microrna dysregulation in cancer. Front Genet. (2013) 4:258. doi: 10.3389/fgene.2013.00258
60. Chakravarti, D, LaBella, KA, and DePinho, RA. Telomeres: history, health, and hallmarks of aging. Cells. (2021) 184:306–22. doi: 10.1016/j.cell.2020.12.028
61. Zhang, J, Rane, G, Dai, X, Shanmugam, MK, Arfuso, F, Samy, RP, et al. Aging and the telomere connection: an intimate relationship with inflammation. Ageing Res Rev. (2016) 25:55–69. doi: 10.1016/j.arr.2015.11.006
62. Vinayavekhin, N, Homan, EA, and Saghatelian, A. Exploring disease through metabolomics. ACS Chem Biol. (2010) 5:91–103. doi: 10.1021/cb900271r
63. Picca, A, Coelho-Junior, HJ, Cesari, M, Marini, F, Miccheli, A, Gervasoni, J, et al. The metabolomics side of frailty: toward personalized medicine for the aged. Exp Gerontol. (2019) 126:110692. doi: 10.1016/j.exger.2019.110692
64. Trappe, T, Williams, R, Carrithers, J, Raue, U, Esmarck, B, Kjaer, M, et al. Influence of age and resistance exercise on human skeletal muscle proteolysis: a microdialysis approach. J Physiol. (2004) 554:803–13. doi: 10.1113/jphysiol.2003.051755
65. Rodriguez-Cuenca, S, Pellegrinelli, V, Campbell, M, Oresic, M, and Vidal-Puig, A. Sphingolipids and glycerophospholipids–the “Ying and Yang” of lipotoxicity in metabolic diseases. Prog Lipid Res. (2017) 66:14–29. doi: 10.1016/j.plipres.2017.01.002
66. Pujos-Guillot, E, Pétéra, M, Jacquemin, J, Centeno, D, Lyan, B, Montoliu, I, et al. Identification of pre-frailty sub-phenotypes in elderly using metabolomics. Front Physiol. (2019) 9:1903. doi: 10.3389/fphys.2018.01903
67. Theou, O, Cann, L, Blodgett, J, Wallace, LM, Brothers, TD, and Rockwood, K. Modifications to the frailty phenotype criteria: systematic review of the current literature and investigation of 262 frailty phenotypes in the survey of health, aging, and retirement in Europe. Ageing Res Rev. (2015) 21:78–94. doi: 10.1016/j.arr.2015.04.001
68. Bruyère, O, Buckinx, F, Beaudart, C, Reginster, J-Y, Bauer, J, Cederholm, T, et al. How clinical practitioners assess frailty in their daily practice: an international survey. Aging Clin Exp Res. (2017) 29:905–12. doi: 10.1007/s40520-017-0806-8
Keywords: biomarkers, molecular biomarkers, solid tumors, frailty, deficit accumulation, cancer survivors
Citation: Sass D, Parmelee Streck B, Guedes VA, Cooper D, Guida JL and Armstrong TS (2023) Blood-based biomarkers of frailty in solid tumors: a systematic review. Front. Public Health. 11:1171243. doi: 10.3389/fpubh.2023.1171243
Received: 21 February 2023; Accepted: 07 April 2023;
Published: 04 May 2023.
Edited by:
Junhong Zhou, Harvard Medical School, United StatesReviewed by:
Matheus Uba Chupel, Sunnybrook Research Institute (SRI), CanadaCopyright © 2023 Sass, Parmelee Streck, Guedes, Cooper, Guida and Armstrong. This is an open-access article distributed under the terms of the Creative Commons Attribution License (CC BY). The use, distribution or reproduction in other forums is permitted, provided the original author(s) and the copyright owner(s) are credited and that the original publication in this journal is cited, in accordance with accepted academic practice. No use, distribution or reproduction is permitted which does not comply with these terms.
*Correspondence: Dilorom Sass, ZGlsb3JvbS5zYXNzQGdtYWlsLmNvbQ==; ZGlsaWpvbjA4QGdtYWlsLmNvbQ==
Disclaimer: All claims expressed in this article are solely those of the authors and do not necessarily represent those of their affiliated organizations, or those of the publisher, the editors and the reviewers. Any product that may be evaluated in this article or claim that may be made by its manufacturer is not guaranteed or endorsed by the publisher.
Research integrity at Frontiers
Learn more about the work of our research integrity team to safeguard the quality of each article we publish.