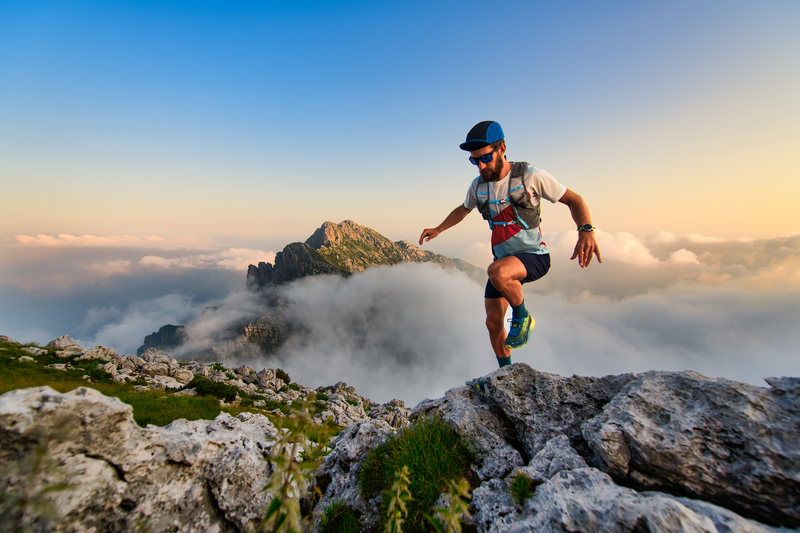
95% of researchers rate our articles as excellent or good
Learn more about the work of our research integrity team to safeguard the quality of each article we publish.
Find out more
ORIGINAL RESEARCH article
Front. Public Health , 09 March 2023
Sec. Children and Health
Volume 11 - 2023 | https://doi.org/10.3389/fpubh.2023.1137933
This article is part of the Research Topic Assessing Health-related Quality of Life of Children View all 23 articles
Background: The adverse effects of 2.5-μm particulate matter (PM2.5) exposure on public health have become an increasing concern worldwide. However, epidemiological findings on the effects of PM2.5-bound metals on children's respiratory health are limited and inconsistent because PM2.5 is a complicated mixture.
Objectives: Given the vulnerability of children's respiratory system, aim to pediatric respiratory health, this study evaluated the potential sources, health risks, and acute health effects of ambient PM2.5-bound metals among children in Guangzhou, China from January 2017 to December 2019.
Methods: Potential sources of PM2.5-bound metals were detected using positive matrix factorization (PMF). A health risk assessment was conducted to investigate the inhalation risk of PM2.5-bound metals in children. The associations between PM2.5-bound metals and pediatric respiratory outpatient visits were examined with a quasi-Poisson generalized additive model (GAM).
Results: During 2017–2019, the daily mean concentrations of PM2.5 was 53.39 μg/m3, and the daily mean concentrations of PM2.5-bound metals range 0.03 ng/m3 [thorium (Th) and beryllium (Be)] from to 396.40 ng/m3 [iron (Fe)]. PM2.5-bound metals were mainly contributed by motor vehicles and street dust. PM2.5-bound arsenic (As), cadmium (Cd), cobalt (Co), chromium (Cr)(VI), nickel (Ni), and lead (Pb) were found to pose a carcinogenic risk (CR). A quasi-Poisson GAM was constructed that showed there were significant associations between PM2.5 concentrations and pediatric outpatient visits for respiratory diseases. PM2.5 was significantly associated with pediatric outpatient visits for respiratory diseases. Moreover, with a 10 μg/m3 increase in Ni, Cr(VI), Ni, and As concentrations, the corresponding pediatric outpatient visits for respiratory diseases increased by 2.89% (95% CI: 2.28–3.50%), acute upper respiratory infections (AURIs) increased by 2.74% (2.13–3.35%), influenza and pneumonia (FLU&PN) increased by 23.36% (20.09–26.72%), and acute lower respiratory infections (ALRIs) increased by 16.86% (15.16–18.60%), respectively.
Conclusions: Our findings showed that PM2.5 and PM2.5-bound As, Cd, Co, Cr(VI), Ni, and Pb had adverse effects on pediatric respiratory health during the study period. New strategies are required to decrease the production of PM2.5 and PM2.5-bound metals by motor vehicles and to reduce levels of street dust to reduce children's exposure to these pollutants and thereby increase child health.
The International Agency for Research on Cancer (IARC) defines outdoor particulate matter (PM) as a Group 1 carcinogen in humans (1). Exposure to fine particulate matter (PM2.5; particulate matter with an aerodynamic diameter of ≤2.5 μm) has significantly increased the burden of disease globally, mainly by increasing the risk of diabetes, cardiovascular diseases, poor respiratory health, and lung cancer (2, 3). In China, PM2.5 exposure is one of the top four risk factors for death from stroke, ischemic heart disease, chronic obstructive pulmonary disease (COPD), and lung cancer (2).
The small size and large specific surface area of PM2.5 means that it can easily bind toxic compounds, including metals. Among these, PM2.5-bound metals are key contributors to PM2.5 toxicity and can cause severe adverse health effects. PM2.5-bound metals can enter the body by ingestion, dermal contact, or by inhalation, which allows them to affect cardiopulmonary function and distribute to organs through the blood circulation (4). Several epidemiological studies have found that exposure to PM2.5-bound nickel (Ni), vanadium (V), lead (Pb), and zinc (Zn) can increase cardiopulmonary diseases, and that exposure to PM2.5-bound arsenic (As), cobalt (Co), Ni, manganese (Mn), and chromium (Cr) can trigger the production of reactive oxygen species and thereby cause respiratory inflammation (5–9). However, owing to on the inconsistency of PM2.5-bound metals, there is a limited epidemiological understanding of their effects on respiratory health (10–13). Therefore, it is critical to investigate the health effects of PM2.5-bound metals further.
A better understanding of the sources of PM2.5-bound metals and the identification of those metals that are most harmful to children's health would assist decision-makers to develop air pollution regulations and provide directions for basic research. Positive matrix factorization (PMF) is an analytical method that has been widely employed to determine the contributions of various sources of PM2.5 to air pollution and is recommended by the United States Environmental Protection Agency (USEPA) (14, 15). Moreover, health risk assessments are recommended by the USEPA and the National Health Commission of the People's Republic of China (16, 17) for identifying heavy metals that pose carcinogenic and non-carcinogenic risks to humans via inhalation (8). A generalized additive model (GAM) with a quasi-Poisson link can be used for time series analysis of the short-term association between ambient air pollution and outpatient visits, as it enables control for long-term trends, day of the week (DOW), temperature, and humidity (18). Thus, we used this model to determine the most significant associations between PM2.5-bound metals and respiratory diseases in children.
Guangzhou is the third-largest city in China and is one of the top four first-tier cities in the country. Due to rapid social and economic development, air pollution condition in Guangzhou has become more serious. Present study on the sources of PM2.5-bound metals in Guangzhou mainly focused on total PM, and the results show that the main sources are traffic emissions, soil dust, and biomass burning (19). However, since the distribution spectrum of metal is specific to different sources, it is of public health importance to study the sources of PM2.5-bound metals and to discover the effects on the population for better regulation of different sources in Guangzhou. In addition, many studies have demonstrated that children are more vulnerable than adults to the adverse effects of air pollution for many reasons. These include the fact that children breathe twice as quickly as adults, children's lungs are still growing, and children spend more time outside than adults (20, 21). Hence, comprehensive studies are needed to determine the characteristics of air pollution and the relationship between metal-containing pollution and respiratory diseases in children.
The major objectives of this study were to (1) use PMF to determine the sources of PM2.5 and PM2.5-bound metals air pollution and estimate their contribution to overall PM2.5 and PM2.5-bound metals air pollution (as a ratio); (2) identify the non-carcinogenic and carcinogenic risks (CR) posed by PM2.5-bound metals to children; and (3) use a GAM to investigate the short-term relationships between air concentrations of PM2.5-bound metals and daily pediatric outpatient visits for respiratory diseases.
Guangzhou (22°26′-23°56′ N, 112°57′-114°3′ E) is located in the south of China and is the primary economic and cultural city in the Pearl River Delta. It covers an area of 7,434 km2 and had a residential population of 8,970,000 in 2017. Due to its location, Guangzhou is an influential port and transportation hub for Guangdong province. Guangzhou has a humid, warm, tropical/subtropical climate, so its weather is hot and humid in summer and mild and dry in winter. The annual average temperature is 22°C, and the annual average humidity is 80%. Yuexiu district is one of the “four old districts” in Guangzhou and from 2017 to 2019 was ranked 10th out of the 11 districts of Guangzhou in terms of air pollution, and Liwan district ranked 11th. Yuexiu district also has the largest population of all districts in Guangzhou, with children younger than 18 years accounting for 14.41% of its population.
Data from January 1, 2017 to December 31, 2019 were obtained from Guangzhou Yuexiu District Children's Hospital, which is one of the two specialized children's hospitals in Guangzhou. During the study period, individuals who visited the respiratory pediatric department of this hospital and were diagnosed with respiratory disease [International Statistical Classification of Diseases and Related Health Problems 10th revision (ICD-10) codes: J00–J99 and R04–R9.3] were identified. Specifically, patients who over 18 years of age would be excluded, and the respiratory diseases would be further divided into three kind of specific diseases: acute upper respiratory infections (AURIs, ICD-10 codes J00–J06), influenza and pneumonia (FLU&PN, J09–J18), and acute lower respiratory infections (ALRIs, J20–J22).
We collected 24-h mean concentrations of PM2.5 and 26 metals from the air monitoring station of the Guangdong Environment Monitoring Center in Yuexiu district for 265 days. The 26 metals were silver (Ag), aluminum (Al), As, barium (Ba), beryllium (Be), bismuth (Bi), Cd, Co, chromium (Cr), copper (Cu), iron (Fe), mercury (Hg), lithium (Li), Ni, Mn, molybdenum (Mo), Pb, antimony (Sb), selenium (Se), tin (Sn), strontium (Sr), thorium (Th), thallium (Tl), uranium (U), V, and Zn. The samples were obtained approximately in the middle of every month, for an average of approximately 10 days, from 2017 to 2019. The air pollution monitor was located approximately 10–20 m above ground level. It was regularly maintained in accordance with the standard operating procedures described in the China National Quality Control Assurance Plan. We also obtained the daily 24-h mean PM2.5 mass from 2017 to 2019 (674 days).
The daily meteorological data of temperature (°C) and relative humidity (%) in the study period were obtained from the Guangdong Meteorological Service.
We used EPA PMF 5.0 to quantify the source contributions of PM2.5-bound metals. The number of sources was calculated based on Cattell's scree test. The mathematical expression of PMF can be written as follows (Equation 1):
where Xij is the concentration of metal j in sample i; p is the number of pollution sources; Gik is the factor contribution of source p to sample i; Fkj is the factor concentration of pollutant j from source p; and Eij is the residual.
Gik and Fkj can be calculated by minimizing the objective function Q (Equation 2), as follows:
where Uij is the uncertainty in the concentration of each PM2.5-bound metal component.
Finally, because we did not know the detection limit for the measurement method, we calculated the uncertainty as follows (Equation 3) (22):
where cj is the relative uncertainty for high values of the measured parameter, and SD is the standard deviation of Xij.
To assess the health risk of inhaled PM2.5-bound metals in children, we adopted the model recommended by the standards of both the USEPA and the National Health Commission of the People's Republic of China. According to previous research (8, 23), Al, As, Ba, Cd, Co, Cr(VI), Mn, Ni, Se, and V are considered to be non-carcinogenic, while As, Cd, Co, Cr(VI), Ni, and Pb are considered to be both carcinogenic and non-carcinogenic. Daily respiratory tract exposure concentrations were estimated by Equation (4), as follows:
where EC is the average daily concentration of metal exposure through inhalation (μg/m3); C is the concentration of metals in PM2.5 (μg/m3); ET is the exposure time (24 h/d for children), EF is the exposure time (365 d/year for children), ED is the exposure duration (18 years for children); ASF is the age sensitivity factor (3 for children); and AT is the average contact time (18 years × 365 d/year × 24 h/d for non-carcinogenic risk and 70 years × 365 d/year × 24 h/d for carcinogenic risk).
The carcinogenic and non-carcinogenic risks were further estimated based on EC. The non-carcinogenic risk level was calculated by Equation (5), as follows:
where HQ is the hazard quotient, which represents the non-carcinogenic risk for PM2.5-bound metals, and the RfC is the maximum daily reference concentration (Table 1), above which there will be a non-carcinogenic risk over a child's lifetime (mg/m3). HQ < 1 indicates that there will be no adverse health effects, while HQ > 1 indicates that non-carcinogenic effects are possible.
Table 1. Summary of non-carcinogenic and carcinogenic risks from exposure to PM2.5-bound metals via inhalation in children.
Carcinogenic risk (CR), which represents the risk of developing cancer due to exposure to PM2.5-bound metals, was calculated by Equation (6), as follows:
where IUR is the inhalation unit risk (m3/μg). CR < 1 × 10−6 indicates a negligible carcinogenic risk in children; CR = 1 × 10−6-1 × 10−4 indicates a possible carcinogenic risk in children; and CR > 10−4 indicates a high carcinogenic risk in children and therefore attention is needed.
We used two-stage Poisson regression in a GAM to examine the associations between pediatric outpatient visits for respiratory diseases and daily PM2.5 and PM2.5-bound metal concentrations, respectively. This model complies with the recommendations of the National Health Commission of the People's Republic of China (17). Due to missing data—the lack of daily concentration data for PM2.5-bound metals—we followed previous studies by computing the ratio of the monthly mean concentration of PM2.5-bound metals to the daily PM2.5 concentration (13, 24). We controlled for seasonality and long-term trends in the model by using penalized smoothing splines with six degrees of freedom (df ) per year. We also included an indicator variable for the DOW and the daily mean temperature and relative humidity, each with three df. Consistent with a previous study (25), we calculated the effect of PM2.5 exposure with a lag of 0–7 days and a moving average of lag days 01–07, in addition to the effect of PM2.5-bound metal exposure with a lag of 0 days.
In the first stage, we fitted a time series analysis of daily pediatric outpatient visits for respiratory disease and daily PM2.5 concentration (Equation 7). In the second stage, we used the PM2.5 concentration, the ratio between the monthly mean concentration of the PM2.5-bound metal and the daily PM2.5 concentration, and the number of daily pediatric outpatient visits for respiratory diseases in Equation (8). These two equations are presented below:
where E(Yt) is the expected number of daily pediatric outpatient visits for respiratory diseases on day t; β0–β3 are the regression coefficients, where β0–β2 represent the main effects of PM2.5 and the ratio of the monthly mean concentration of PM2.5-bound metals to the daily PM2.5 concentration and β3 is the interaction term; Zt is the daily PM2.5 concentration; s is the penalized smoothing spline function; df are the degrees of freedom; Xt is the meteorological factor, which includes daily mean temperature and relative humidity, DOW is the day of week, which was used to adjust for the DOW effect; and a is the intercept. We reported the results as the percent change in excess risk (ER) with a 95% confidence interval (CI) for every 10 μg/m3 increase in PM2.5 concentration or for every interquartile range (IQR) increase in the ratio of the monthly mean PM2.5-bound metal concentration to daily PM2.5 concentration.
All analyses were conducted using the mgcv package in R (version 4.0.2) software. P < 0.05 was considered statistically significant.
Table 2 shows the means and standard deviations of PM2.5 concentrations, PM2.5-bound metal concentrations, and pediatric outpatient visits for respiratory disease from 2017 to 2019. During the study period, there were 1,707,346 pediatric outpatient visits for respiratory diseases, ranging from 176 to 3,046 visits per day. During the 265-day sampling period, the daily mean concentrations of PM2.5, Cd, As, Cr, and Hg were 53.39 μg/m3, 1.22, 6.05, 3.22, and 0.06 ng/m3, respectively. Based on the secondary and primary concentration limits in GB 3085-2012 and stipulated by the World Health Organization, 19.60%, 69.00%, and 89.10% of the days exceeded the PM2.5 concentration limit (75.00, 35.00, and 25.00 μg/m3), and 1.10%, 35.80%, and 100% of the days exceeded the Cd, As, and Cr concentration limits, respectively. Cr(VI) accounted for 16.66% of the total Cr concentration (26).
Table 2. Daily means and standard deviations of PM2.5 concentration, PM2.5-bound metal concentrations, and pediatric respiratory outpatient visits from 2017 to 2019.
As shown in Figure 1, the PM2.5 concentrations were higher in winter and lower in summer. The time series analysis required PM2.5 concentration information on consecutive dates; however, there were incomplete data for the 24-h mean PM2.5 concentrations for the 26 metals (data from only 265 days were available). However, the trends in the PM2.5 concentrations on these 265 days and the trends in the PM2.5 concentrations over the entire study period were similar. Therefore, we used the continuously monitored PM2.5 data to fit the incomplete PM2.5 concentration data by correlation and linear regression, and the results showed a strong correlation (r = 0.74, P < 0.01) and were significant (β = 0.97, P < 0.05).
Figure 1. Trends in the complete and incomplete PM2.5 concentration (μg/m3) on the sampling dates in Yuexiu district and a comparison with World Health Organization and GB 3085-2012 standards. The three lines represent PM2.5 concentration limits in GB 3085-2012 (75.00 and 35.00 μg/m3) and World Health Organization (25.00 μg/m3).
We used PMF to analyze the sources of PM2.5-bound metals. First, Cattle's scree test was performed to determine the number of principal components. Next, based on the source spectrum database in China (15, 27, 28), EPA PMF 5.0 was employed to quantify PM2.5-bound metal sources. Based on the Kaiser criterion in the Cattle's scree test, we retained four principal factors as pollution sources. Figure 2 presents the PMF results from 2017 to 2019. These results reveal that the six potential sources chosen by the model were street dust, motor vehicles, ships and heavy oil, secondary nitrates, boiler combustion, and aerospace manufacturing.
Figure 2. Positive matrix factorization (PMF) analysis of the distribution of sources of PM2.5-bound metal from 2017 to 2019. Red dot means percentage ratio of each kind of species, blue bar means concentration of each species.
Among these six potential sources, street dust was characterized by high concentrations of Al, Cr, and Fe; motor vehicles were characterized by high concentrations of Pb and Zn; ships and heavy oil were characterized by high concentrations of Ni and V; secondary nitrates were characterized by high concentrations of sulfate (), nitrate (), and ammonium (); boiler combustion was characterized by high concentrations of As; and aerospace manufacturing was characterized by high concentrations of Be.
The source contributions to PM2.5-bound metals from 2017 to 2019 are shown in Figure 3. The highest contributions in the 3 years were from motor vehicles and boiler combustion (2017), motor vehicles and street dust (2018), and street dust (2019).
Table 1 shows the exposure parameters and non-carcinogenic risk assessment results for exposure to PM2.5-bound Al, As, Ba, Cd, Co, Cr(VI), Mn, Ni, Se, and V, and the CRs for exposure to PM2.5-bound As, Cd, Co, Cr(VI), Ni, and Pb. Only Mn posed a non-carcinogenic health risk, and thus the other PM2.5-bound metals were not included in the time series analysis. The mean CRs of As, Cd, Co, Cr(VI), Ni, and Pb exceeded the acceptable risk level (1 × 10−6); therefore, the relatively high exposures of children to these PM2.5-bound metals are of concern.
Figure 4 shows the PM2.5-associated ER for total pediatric outpatient visits for diseases and pediatric outpatient visits for three respiratory diseases. The lag structure of changes in pediatric outpatient visits for respiratory diseases was associated with a 10 μg/m3 increase in PM2.5 daily concentration. PM2.5 concentration significantly affected the number of daily pediatric outpatient visits due to AURIs, FLU&PN, and ALRIs.
Figure 4. Excess risk and 95% CIs for the percentage increase in outpatient visits for respiratory diseases per 10 μg/m3 increase in the concentration of PM2.5. CI, confidence interval, the black dot indicates the mean excess risk, the black vertical line corresponds to 95%CI, and the vertical dotted line at 0 represents the reference value.
Regarding the lag effect, lag3 was specifically associated with a 1.49% (95% CI: 1.39–1.59%) increase in daily pediatric outpatient visits for respiratory diseases per 10 μg/m3 increase in PM2.5 concentrations. Regarding pediatric outpatient visits for the three causes of respiratory diseases, the highest lag effects were a 3.04% (95% CI: 2.59–3.49%) increase in FLU&PN at lag0, a 2.18% (95% CI: 2.04–2.31%) increase in AURIs at lag0, and a 1.36% (95% CI: 1.13–1.59%) increase in ALRIs at lag5.
In terms of the cumulative lag effect, the strongest effect was at lag07 for all but FLU&PN—the FLU&PN daily outpatient visits reached a peak at lag05. In terms of total pediatric outpatient visits for respiratory diseases, each 10 μg/m3 increase in the concentration of PM2.5 was associated with a 3.18% (95% CI: 3.01–3.35%) increase in visits. FLU&PN visits were most affected, with an increase of 4.84% (95% CI: 4.20–5.49%), which was consistent with the results of the lagged effects model.
We included As, Cd, Co, Cr(VI), Mn, Ni, and Pb in the GAM because these metals were found in the risk assessment to have carcinogenic and non-carcinogenic risks in children. Next, as mentioned, the ratio of the monthly mean concentration of PM2.5-bound metals to the daily PM2.5 concentration was calculated to compensate for the absence of daily metal information. The ER for respiratory disease outpatient visits is given per IQR increase in the monthly mean ratio of metal concentration to PM2.5 concentration.
Figure 5 shows the changes in total pediatric outpatient visits for respiratory diseases and pediatric outpatient visits for the three respiratory diseases associated with an IQR increase in the monthly mean metal-to-PM2.5 concentration ratio. The increase in monthly mean Ni concentration/PM2.5 concentration had the greatest effect, increasing total pediatric outpatient visits for respiratory diseases by 2.89% (95% CI: 2.28–3.50%) and for daily pediatric outpatient visits for FLU&PN by 23.36% (95% CI: 20.09–26.72%). An IQR increase in the monthly mean Cr(VI) and As concentrations/PM2.5 concentration had the greatest effects on daily pediatric outpatient visits for AURIs and ALRIs, respectively.
Figure 5. Percentage change (ER and 95% CI) in pediatric outpatient visits for various respiratory diseases per IQR increase in the concentrations of As, Cd, Co, Cr(VI), Mn, Ni, and Pb in PM2.5 at time lag0. CI, confidence interval, black dot indicates the mean excess risk, the black vertical line corresponds to 95%CI, and the vertical dotted line at 0 represents the reference value.
The exposure–response relationship curves in Figure 6 indicate that the daily concentrations of PM2.5 and PM2.5-bound metals were associated with total daily pediatric outpatient visits for respiratory diseases at lag0 (Figure 6). The relationships between exposure to PM2.5, As, Cd, Co, Cr(VI), Mn, and total daily pediatric outpatient visits for respiratory diseases show an “N” shape, suggesting that lower concentrations of PM2.5 and these six metals increased the total daily pediatric outpatient visits for respiratory diseases. The exposure–response curve for Ni shows a “~” trend, indicating that as Ni exposure increased, the total daily pediatric outpatient visits for respiratory diseases initially decreased, then rose, and then finally decreased.
Figure 6. Exposure–response relationships between the concentrations of ambient PM2.5 and PM2.5-bound metals and total pediatric outpatient visits for respiratory diseases at lag0. CI, confidence interval, dotted line represents confidence interval; solid line represents log relative risk.
This study was conducted in three stages. In the first stage, we investigated the pollution characteristics of PM2.5-bound metals through PMF source analysis. In the second stage, we identified metals that pose a potential carcinogenic risk and non-carcinogenic risk for children. In the third stage, we used GAM to analyze the associations between ambient PM2.5 and PM2.5-bound metals and the risk of daily pediatric outpatient visits for respiratory diseases in Yuexiu district, Guangzhou, China, from 2017 to 2019. During the study period, the daily mean PM2.5 concentration was 53.39 μg/m3 in Yuexiu district; by comparison, a similar study found a concentration of 50.30 μg/m3 in Panyu district, Guangzhou (29). Yuexiu district has poor air quality and severe ambient pollution. The PM2.5 concentration is typically higher in spring and winter and lower in summer and autumn; these changes might be related to the decrease in temperature and relative humidity in spring and winter, in addition to firecracker burning that takes place during the Spring Festival in China (30).
The PMF source analysis results showed that the primary sources of PM2.5-bound metals were motor vehicles and present in street dust; secondary sources were boiler combustion and ships and heavy oil. These results are consistent with previous studies that found that PM2.5-bound metals originated mostly from vehicle exhaust and boiler combustion in Zhuhai (28) and Guangzhou (27). According to the source spectrum database in China (15, 27, 28), Al, Cr, and Fe are mainly found in street dust, Pb and Zn are mainly emitted by motor vehicles, Ni and V are mainly produced by ship fuel and heavy oil combustion, and As is mainly produced by boiler combustion.
The health risk assessment outcomes showed that PM2.5-bound As, Cd, Co, Cr(VI), Mn, Ni, and Pb posed a risk to children. Only Mn had a non-carcinogenic risk, a finding that is consistent with a similar study (9). In the carcinogenic risk assessment, As, Cd, Co, Cr(VI), Ni, and Pb were found to be potentially carcinogenic in children, with a CR order of Cr(VI) > As > Co > Cd > Ni > Pb. This is consistent with the results of a previous study in Yuexiu district (As > Cd) (9). At present, the IARC classifies As, Cd, and Cr(VI) as “I” (Group 1 carcinogens) and Ni as “IIB” (Group 2B carcinogens); hence, more attention should be given to the health effects of As, Cd, Co, Cr(VI), Mn, Ni, and Pb exposure.
We found significant associations between PM2.5 concentrations and pediatric outpatient visits for various respiratory diseases. We also found that the effect of PM2.5 concentrations on pediatric outpatient visits for various respiratory diseases was cumulative. Regarding the lag effect, we observed that the PM2.5 concentration had a significant positive effect on total pediatric outpatient visits for respiratory diseases at lag3 (1.49%; 95% CI: 1.39–1.59%). This result is similar to that in a previous study in the same city. However, it is a stronger association than has been found in a different city (25, 31). Regarding the cumulative lag effect, the effect of PM2.5 concentration on pediatric outpatient visits for various respiratory diseases increased with the number of cumulative lag days. The most significant effect was for lag07, where each 10 μg/m3 increase in PM2.5 concentration was associated with a 3.18% (95% CI: 3.01–3.35%) increase in total daily pediatric outpatient visits for respiratory diseases. This finding is similar to those of previous studies (25, 31).
Regarding pediatric respiratory outpatient visits for AURIs, FLU&PN, and ALRIs, the ER was most significant at lag0 for AURIs and FLU&PN. The ER increase slowed as cumulative lag days increased. A few studies have examined the relationship between outpatient visits for different respiratory diseases and PM2.5, and most have also only found relationships between PM2.5 concentrations and AURIs and FLU&PN (25, 32, 33). Therefore, further exploration of the relevant mechanisms and epidemiological evidence is necessary. Our analysis revealed that As, Cd, Co, Cr(VI), Mn, Ni, and Pb concentrations were associated with pediatric outpatient visits for respiratory diseases. In a similar analysis, Valdés et al. (13) found that Ni and Cr concentrations were significantly associated with higher respiratory mortality. In line with our findings, a study in Canada (6) reported associations between respiratory hospitalizations and Sulfur (S) and Cu, and Mn exposure, and a study in Xian (11) found a significant association between Ni exposure and respiratory mortality.
The mechanisms by which inhaled As, Cd, Co, Cr(VI), Mn, Ni, and Pb adversely affect children's respiratory health have been widely studied (34–39). As is known to cause lung cancer by disrupting immune function and inhibiting immune function-related genes, and as exposure is also associated with non-malignant respiratory diseases (e.g., acute respiratory infections and symptoms of decreased lung function) (36). Low levels of Cd exposure can lead to lung function alterations that can cause pulmonary fibrosis, emphysema, and lung tumors, and Cd toxicity increases oxidative stress that depletes protein-bound glutathione and sulfhydryl groups. Although Cd can enter the body through inhalation, the current study shows that high Cd exposure mainly arises through food intake and smoking. This suggests that particular attention should be given to preventing children being exposed to secondhand smoke (35). Chronic inhalation of Co compounds can lead to respiratory tumors, and Co exposure often leads to interstitial lung disease (34). Inhalation is the primary route of exposure to Cr and can lead to lung cancer, chromosomal damage, asthma, cough, acute bronchitis, and pulmonary edema (35). Exposure to low concentrations of Mn can result in adverse health effects on respiratory organs; Mn is also an immunotoxin and is cytotoxic to lung macrophages, thus making children susceptible to small airway bronchial injury (37). Ni exposure can increase respiratory disease morbidity and mortality, and it promotes the expression of inflammatory factors, contributing to airway inflammation (39). Pb exposure downregulates interleukin-13 expression and can increase the risk of asthma-related immunomodulatory abnormalities in preschool children (38).
Children are a population group that is sensitive to air pollution because they are in a period of growth and development. In addition, children have a relatively rapid respiratory rate and are more vulnerable to respiratory diseases such as asthma, cough, and respiratory inflammation. Studies have shown that respiratory diseases have become the most frequent cause of childhood outpatient visits for illness, and these diseases are a primary medical problem adversely affecting the health and growth of children (40).
Thus, the government should strengthen the supervision and control of three sources of pollution—waste incineration, metal smelting, and traffic—in addition to improving purification measures and rationalizing the separation of traffic flow to relieve traffic pressure. In addition, parents can lower the risk of respiratory diseases in their children by reducing their children's exposure to PM2.5, As, Cd, Co, Cr(VI), Mn, Ni, and Pb. This can be achieved by parents not bringing their children to certain areas and not exposing them to secondhand smoke. Finally, parents should also ensure that their children have adequate physical exercise to strengthen their immunity.
This study has several strengths. First, to the extent of our knowledge, it is the first study to use a comprehensive and systematic approach to quantify the associations between exposure to PM2.5-bound metals and pediatric outpatient visits for respiratory disease. Second, it examined the total pediatric outpatient visits for respiratory diseases, AURIs, FLU&PN, and ALRIs, while previous studies have investigated only some of these conditions. Third, to obtain reliable results, it used a quasi-Poisson GAM with adjustments for lag effects, DOW, temperature, and humidity.
The limitations of our study should also be noted. First, we included clinical data from only one hospital, which cannot accurately represent all of the pediatric outpatient visits in Yuexiu district. Second, the air pollution exposure concentration we used was obtained from regional air pollution monitoring data, and thus there may have been some errors in the exposure measurement. Third, a certain amount of error was introduced by compensating for the lack of daily data on metal concentrations by calculating the monthly mean metal concentration to daily PM2.5 concentration ratio. Few studies have been conducted in this way; therefore, precise comparisons with previous studies were not possible. Nevertheless, relative changes were examined.
Our findings suggest that PM2.5 and PM2.5-bound As, Cd, Co, Cr(VI), Ni, and Pb pose potential health risks to children in Guangzhou, China, and can contribute to respiratory disease in this population. Government departments can lower children's risk of respiratory diseases by introducing measures that decrease the production of these pollutants by motor vehicles and reduce the amount of street dust.
The original contributions presented in the study are included in the article/supplementary material, further inquiries can be directed to the corresponding author.
QY designed the study and supervised the research, including funding, text review, and overall quality assurance and control. YZ and SC were helped with the formulation of research methods, software analysis and interpretation of the results, and wrote the original draft of the text. YC and JL were helped with the investigation and review of the data and assisted in the preparation of the original draft of the text. BX and TS were assisted in the implementation of research, data management and investigation, and supervision. All authors contributed to the article and approved the submitted version.
This work was supported by the National Natural Science Foundation of China (Grant Number: 81773385 to QY), Natural Science Foundation of Guangdong Province (Grant Numbers: 2019A1515011298 and 2022A1515010727 to QY), and Key scientific research projects of Guangdong Provincial Department of Education (Grant Number: 2022ZDZX2047 to QY).
The authors declare that the research was conducted in the absence of any commercial or financial relationships that could be construed as a potential conflict of interest.
All claims expressed in this article are solely those of the authors and do not necessarily represent those of their affiliated organizations, or those of the publisher, the editors and the reviewers. Any product that may be evaluated in this article, or claim that may be made by its manufacturer, is not guaranteed or endorsed by the publisher.
1. Cancer IAfRo. IARC Monographs on the Evaluation of Carcinogenic Risks to Humans. Lyon: International Agency for Research on Cancer (2013, 2016). Available online at: https://monographs.iarc.who.int/list-of-classifications
2. Bu X, Xie Z, Liu J, Wei L, Wang X, Chen M, et al. Global PM2.5-attributable health burden from 1990 to 2017: estimates from the Global Burden of disease study 2017. Environ Res. (2021) 197:111123. doi: 10.1016/j.envres.2021.111123
3. Zhang Q, Meng X, Shi S, Kan L, Chen R, Kan H. Overview of particulate air pollution and human health in China: evidence, challenges, and opportunities. Innovation (Camb). (2022) 3:100312. doi: 10.1016/j.xinn.2022.100312
4. Zhang L, Jin X, Johnson AC, Giesy JP. Hazard posed by metals and As in PM25 in air of five megacities in the Beijing-Tianjin-Hebei region of China during APEC. Environ Sci Pollut Res Int. (2016) 23:17603–12. doi: 10.1007/s11356-016-6863-2
5. Zhao Y, Wang S, Lang L, Huang C, Ma W, Lin H. Ambient fine and coarse particulate matter pollution and respiratory morbidity in Dongguan, China. Environ Pollut. (2017) 222:126–31. doi: 10.1016/j.envpol.2016.12.070
6. Korsiak J, Lavigne E, You H, Pollitt K, Kulka R, Hatzopoulou M, et al. Air pollution and pediatric respiratory hospitalizations: effect modification by particle constituents and oxidative potential. Am J Respir Crit Care Med. (2022) 206:1370–8. doi: 10.1164/rccm.202205-0896OC
7. Chang Q, Liu S, Chen Z, Zu B, Zhang H. Association between air pollutants and outpatient and emergency hospital visits for childhood asthma in Shenyang city of China. Int J Biometeorol. (2020) 64:1539–48. doi: 10.1007/s00484-020-01934-9
8. Wang YS, Chang LC, Chang FJ. Explore regional PM25 features and compositions causing health effects in Taiwan. Environ Manage. (2021) 67:176–91. doi: 10.1007/s00267-020-01391-5
9. Chen R, Jia B, Tian Y, Feng Y. Source-specific health risk assessment of PM(25)-bound heavy metals based on high time-resolved measurement in a Chinese megacity: insights into seasonal and diurnal variations. Ecotoxicol Environ Saf. (2021) 216:112167. doi: 10.1016/j.ecoenv.2021.112167
10. Achilleos S, Kioumourtzoglou MA, Wu CD, Schwartz JD, Koutrakis P, Papatheodorou SI. Acute effects of fine particulate matter constituents on mortality: a systematic review and meta-regression analysis. Environ Int. (2017) 109:89–100. doi: 10.1016/j.envint.2017.09.010
11. Cao J, Xu H, Xu Q, Chen B, Kan H. Fine particulate matter constituents and cardiopulmonary mortality in a heavily polluted Chinese city. Environ Health Perspect. (2012) 120:373–8. doi: 10.1289/ehp.1103671
12. Hwang SH, Lee JY Yi SM, Kim H. Associations of particulate matter and its components with emergency room visits for cardiovascular and respiratory diseases. PLoS ONE. (2017) 12:e0183224. doi: 10.1371/journal.pone.0183224
13. Valdés A, Zanobetti A, Halonen JI, Cifuentes L, Morata D, Schwartz J. Elemental concentrations of ambient particles and cause specific mortality in Santiago, Chile: a time series study. Environ Health. (2012) 11:82. doi: 10.1186/1476-069X-11-82
14. US EPA. Positive Matrix Factorization Model for Environmental Data Analyses. (2022). Available online at: https://www.epa.gov/air-research/positive-matrix-factorization-model-environmental-data-analyses
15. Ho WY, Tseng KH, Liou ML, Chan CC, Wang CH. Application of positive matrix factorization in the identification of the sources of PM2.5 in Taipei city. Int J Environ Res Public Health. (2018). 15:1305. doi: 10.3390/ijerph15071305
16. US EPA. Risk Assessment. (2022). Available online at: https://www.epa.gov/risk
17. China NHCotPsRo. Technical Specifications for Health Risk Assessment of Ambient Air Pollution. WS/T 666-2019. Beijing: Standards Press of China (2019).
18. Kan H, London SJ, Chen G, Zhang Y, Song G, Zhao N, et al. Differentiating the effects of fine and coarse particles on daily mortality in Shanghai, China. Environ Int. (2007) 33:376–84. doi: 10.1016/j.envint.2006.12.001
19. Yang M, Zeng HX, Wang XF, Hakkarainen H, Leskinen A, Komppula M, et al. Sources, chemical components, and toxicological responses of size segregated urban air PM samples in high air pollution season in Guangzhou, China. Sci Total Environ. (2023) 865:161092. doi: 10.1016/j.scitotenv.2022.161092
20. Friedrich MJ. UNICEF reports on the impact of air pollution on children. JAMA. (2017) 317:246. doi: 10.1001/jama.2016.19034
21. Saadeh R, Klaunig J. Child's development and respiratory system toxicity. J Environ Anal Toxicol. (2014) 4:5. doi: 10.4172/2161-0525.1000233
22. Brown SG, Eberly S, Paatero P, Norris GA. Methods for estimating uncertainty in PMF solutions: examples with ambient air and water quality data and guidance on reporting PMF results. Sci Total Environ. (2015). 518–519:626–35. doi: 10.1016/j.scitotenv.2015.01.022
23. Xiao SH, Cai MJ, Li X, Huang Z, Wang J, Zhu QD, et al. [Characterization and health risk assessment of heavy metals in PM2.5 in Xiamen port]. Huan Jing Ke Xue. (2022) 43:3404–15 (Chinese). doi: 10.13227/j.hjkx.202110163
24. Mostofsky E, Schwartz J, Coull BA, Koutrakis P, Mittleman MA. Modeling the association between particle constituents of air pollution and health outcomes. Am J Epidemiol. (2012) 176:317–26. doi: 10.1093/aje/kws018
25. Li Y, Li C, Liu J, Meng C, Xu C, Liu Z, et al. An association between PM(25) and pediatric respiratory outpatient visits in four Chinese cities. Chemosphere. (2021) 280:130843. doi: 10.1016/j.chemosphere.2021.130843
26. Brown RJC, Van Aswegen S, Webb WR, Goddard SL. UK concentrations of chromium and chromium (VI), measured as water soluble chromium, in PM10. Atmos Environ. (2014) 99:385–91. doi: 10.1016/j.atmosenv.2014.10.008
27. Wang X, Xinhui BI, Sheng G, Jiamo FU. Chemical composition and sources of PM10 and PM2.5 aerosols in Guangzhou, China. Environ Monit Assess. (2006). 119:425–30. doi: 10.1007/s10661-005-9034-3
28. Liang Z, Zhao X, Chen J, Gao L, Zhu A, Wang Z, et al. Seasonal characteristics of chemical compositions and sources identification of PM2.5 in Zhuhai, China. Environ Geochem Health. (2018) 41:715–28. doi: 10.1007/s10653-018-0164-2
29. Min L, Yan-hong G, Ling-chuan G, Xiao-wen L, Jing Y. Pollution characteristics of atmospheric PM(2.5) metal components in two urban areas of Guangzhou. J Environ Occup. (2016). 33. doi: 10.16241/j.cnki.1001-5914.2016.05.011
30. Zhao JP, Xu Y, Zhang FW, Chen JS. Atmospheric pollution characteristic during fireworks burning time in spring festival in Quanzhou suburb. Huan Jing Ke Xue. (2011) 32:1224–30. doi: 10.13227/j.hjkx.2011.05.020
31. Liu J, Li Y, Li J, Liu Y, Tao N, Song W, et al. Association between ambient PM(25) and children's hospital admissions for respiratory diseases in Jinan, China. Environ Sci Pollut Res Int. (2019) 26:24112–20. doi: 10.1007/s11356-019-05644-7
32. Xiao D, Guo W, Xu D, Chen J, Liang Z, Zhang X. Three exposure metrics for fine particulate matter associated with outpatient visits for acute lower respiratory infection among children in Guangzhou, China. Front Public Health. (2022) 10:876496. doi: 10.3389/fpubh.2022.876496
33. Liang Z, Meng Q, Yang Q, Chen N, You C. Size-specific particulate matter associated with acute lower respiratory infection outpatient visits in children: a counterfactual analysis in Guangzhou, China. Front Public Health. (2021) 9:789542. doi: 10.3389/fpubh.2021.789542
34. Adams TN, Butt YM, Batra K, Glazer CS. Cobalt related interstitial lung disease. Respir Med. (2017) 129:91–7. doi: 10.1016/j.rmed.2017.06.008
35. Osman MA, Yang F, Massey IY. Exposure routes and health effects of heavy metals on children. BioMetals. (2019) 32:563–73. doi: 10.1007/s10534-019-00193-5
36. Sanchez TR, Perzanowski M, Graziano JH. Inorganic arsenic and respiratory health, from early life exposure to sex-specific effects: a systematic review. Environ Res. (2016) 147:537–55. doi: 10.1016/j.envres.2016.02.009
37. McRae N, Gennings C, Rivera Rivera N, Tamayo-Ortiz M, Pantic I, Amarasiriwardena C, et al. Association between prenatal metal exposure and adverse respiratory symptoms in childhood. Environ Res. (2022) 205:112448. doi: 10.1016/j.envres.2021.112448
38. Zeng Z, Xu X, Zhu Y, Wang Q, Zhang Y, Huo X. Pb and Cd exposure linked with Il-10 and Il-13 gene polymorphisms in asthma risk relevant immunomodulation in children. Chemosphere. (2022) 294:133656. doi: 10.1016/j.chemosphere.2022.133656
39. Gray DL, Wallace LA, Brinkman MC, Buehler SS, La Londe C. Respiratory and cardiovascular effects of metals in ambient particulate matter: a critical review. Rev Environ Contam Toxicol. (2015) 234:135–203. doi: 10.1007/978-3-319-10638-0_3
Keywords: PM2.5-bound metals, source identification, children, health risk, time-series study
Citation: Zheng Y, Chen S, Chen Y, Li J, Xu B, Shi T and Yang Q (2023) Association between PM2.5-bound metals and pediatric respiratory health in Guangzhou: An ecological study investigating source, health risk, and effect. Front. Public Health 11:1137933. doi: 10.3389/fpubh.2023.1137933
Received: 05 January 2023; Accepted: 20 February 2023;
Published: 09 March 2023.
Edited by:
Jing Wu, Tianjin University, ChinaReviewed by:
Arlette Setiawan, Padjadjaran University, IndonesiaCopyright © 2023 Zheng, Chen, Chen, Li, Xu, Shi and Yang. This is an open-access article distributed under the terms of the Creative Commons Attribution License (CC BY). The use, distribution or reproduction in other forums is permitted, provided the original author(s) and the copyright owner(s) are credited and that the original publication in this journal is cited, in accordance with accepted academic practice. No use, distribution or reproduction is permitted which does not comply with these terms.
*Correspondence: Qiaoyuan Yang, cWlhb3l1YW5feWFuZ0BnemhtdS5lZHUuY24=
†These authors have contributed equally to this work
Disclaimer: All claims expressed in this article are solely those of the authors and do not necessarily represent those of their affiliated organizations, or those of the publisher, the editors and the reviewers. Any product that may be evaluated in this article or claim that may be made by its manufacturer is not guaranteed or endorsed by the publisher.
Research integrity at Frontiers
Learn more about the work of our research integrity team to safeguard the quality of each article we publish.