- 1School of Health Management, Harbin Medical University, Harbin, Heilongjiang, China
- 2Department of Nuclear Medicine, The Fourth Hospital of Harbin Medical University, Harbin, Heilongjiang, China
Objective: This study aimed to explore the current state of governance of full population coverage of health insurance in China and its influencing factors to provide empirical references for countries with similar social backgrounds as China.
Methods: A cross-sectional quantitative study was conducted nationwide between 22 January 2020 and 26 January 2020, with descriptive statistics, analysis of variance, and logistic regression models via SPSS 25.0 to analyze the effectiveness and influencing factors of the governance of full population coverage of health insurance in China.
Results: The effectiveness of the governance relating to the total population coverage of health insurance was rated as good by 59% of the survey respondents. According to the statistical results, the governance of the public's ability to participate in insurance (OR = 1.516), the degree of information construction in the medical insurance sector (OR = 2.345), the government's governance capacity (OR = 4.284), and completeness of the government's governance tools (OR = 1.370) were all positively correlated (p < 0.05) on the governance effect of the whole population coverage of health insurance.
Conclusions: The governance of Chinese health insurance relating to the total population coverage is effective. To effectively improve the effectiveness of the governance relating to the total population coverage of health insurance, health insurance information construction, governance capacity, and governance tools should be the focus of governance to further improve the accurate expansion of and increase the coverage of health insurance.
Background
The actualization of health insurance population coverage (HIPC) is one of the biggest challenges facing global health development and is one of the essential health system reforms and development goals advocated by the World Health Organization (WHO) (1). Relevant studies showed that, in 2019, the average effective coverage rate of the global insured population was 60.3% and more than one-third of the world's population still lacked health insurance (2). In the past decade, through its governance of the medical insurance sector, China rapidly increased the average effective coverage rate of its insured population from <30% in 2000 to ~95% in 2010 and has maintained this level to this day (3–6). There is no denying that, as a typical developing country, China has a large population and complex structure where the productivity level is not high, yet, in the shortest possible time, it successfully completed the most expansive HIPC (7, 8). Chinese HIPC governance and its experience, compared with other countries with similar social backgrounds, has received worldwide recognition and high reference value and is worth examining.
Although China has maintained a high level of health insurance population coverage in recent years, still ~80 million people are not covered by health insurance. This phenomenon has continued since 2010. Therefore, to understand the effectiveness of HIPC governance in China in recent past, an in-depth analysis of the factors affecting the efficacy of HIPC governance has become essential for further coverage of the HIPC in China. HIPC governance effectiveness refers to the systematic or singular results produced by the party and government as the core governing body through a series of systematic arrangements and the combined use of composite governance tools and instruments in HIPC. China's efforts in the last decade in the governance of full population coverage of health insurance have led the country to achieve 95% population coverage of health insurance in 2018 alone. However, the special nature of the voluntary principle of participation has impacted the governance of the whole population coverage, calling for the need to focus on the structure of the uninsured population even while acknowledging the increase in the number of people covered by health insurance. Effective governance of health insurance coverage for the entire population should be demonstrated not just by the fact that the majority of the population is insured but also to ensure that the uninsured do not choose to be insured because they are unable to afford health insurance premiums.
Naser Derakhshani (9), Dorjsuren Bayarsaikhan (10), Chhabi L. Ranabhat (11), Gulbiye Yenimahalleli Yasar (12), Ngan Do (13), and other scholars, in a systematic review of the process involved in the HIPC, suggested that social infrastructure, government participation, subject governance capacity, and the choice of instruments and tools were important factors that affect the effectiveness of HIPC governance. A review of the existing studies revealed that most scholars have mainly explored the factors affecting the effectiveness of HIPC governance from the government's perspective. The effectiveness of HIPC governance is also closely related to the governance of individual factors. Studies have shown that the lower affordability of health insurance for the uninsured population is a major factor affecting the achievement of HIPC in most countries such as Vietnam, which has a high uninsured rate of 75% among the poor, and Kenya, which has the highest uninsured rate of 97% among the poor (14–16). The latest data from the China Health and Nutrition Survey (CHNS) also showed that 47% of the uninsured were unable to enroll due to insufficient ability to pay for health insurance, which confirms that it is not only government factors but also individual factors that affect the effectiveness of HIPC governance in China. However, many of the current literature focuses on the influence of specific factors and lacks a comprehensive analysis of HIPC governance from a holistic perspective. Therefore, based on the comprehensive analysis framework of “Government Factors—Governance of Individual Factors,” this study aimed to explore the factors that influence the effectiveness of HIPC governance holistically.
In this study, we aimed to evaluate the governance effectiveness of HIPC in China, comprehensively explore the factors that significantly affect the governance effectiveness of HIPC in China, share important lessons learned from China's move toward HIPC, and provide empirical references for other countries with similar socio-historical backgrounds as China to achieve HIPC in their respective countries in the future.
Materials and methods
Study design
This study used a cross-sectional questionnaire to analyze the effectiveness of HIPC governance in China and the factors influencing it. Before the formal survey, a pre-survey was conducted, and 80 questionnaires were collected using a convenience sampling method to improve the quality of our design and questionnaire. The cross-sectional quantitative study was conducted nationwide between 22 January 2020 and 26 January 2020 using a purposive sampling method through the Questionnaire Star platform, a widely recognized online questionnaire platform in China, influenced by the outbreak of coronavirus (COVID-19). For research fit, the sample population eligible for the study had to meet the following conditions: The survey population had to be familiar with health insurance, be fully aware of the dynamics of health insurance policies, and understand the effects of policy implementation. To ensure the representativeness and validity of the original data, managers and staff of health insurance and its related departments, and academics whose research field is health insurance and its related fields were selected as the respondents of the survey, and their questionnaire results were used as the sample data.
The introductory section of the questionnaire required written informed consent before proceeding further. According to the IP address recorded on the questionnaire, each participant could only answer once. If a questionnaire was completed in 8 min or more, which was the minimum time our team tested to complete the questionnaire, and logically answered two questions, it was judged valid and included in the analysis; otherwise, it was removed. In the end, 2,000 questionnaires were distributed in this study and 1,975 valid questionnaires were returned, with an effective rate of 98.75%.
Data extraction
To measure the governance of HIPC in China, respondents were asked “How effective do you think the governance of universal health coverage is at present?”. The question was evaluated by setting the options to “not at all effective, not very effective, average, relatively effective, very effective.” Using multiple logistic regression, the dependent variable (HIPC governance) was divided into two categories, with 0 indicating not very effective (selecting “not at all effective” and “not very effective”) and 1 indicating effective (selecting “average,” “relatively effective,” “very effective”).
The governance of individual factors included two key areas: governance in the area of participant awareness and governance in the area of participant capacity. In the survey, respondents were asked the following questions to measure the effectiveness of governance in the two areas of participant awareness and participant participation: “What is the level of governance for participant awareness in the HIPC governance process?” (low = 0; high = 1) and “How well is the governance of participation capacity in the HIPC governance process?” (low = 0; high = 1).
The government factor consisted of five major indicators: the degree of clarity of the government's governance functions, the degree of medical insurance information sharing between regions, the degree of information technology construction in the medical insurance sector, the government's governance capacity, and the completeness of governance tools. These factors were assessed through the survey respondents' answers to the following questions, respectively: “Do you think there is clarity in the government's role in the HIPC governance process?” (Unclear = 0; Clarity = 1); “Does your region share health insurance information with other regions?” (not achieved = 0, achieved = 1); “How well do you think China's medical insurance is currently being informalized?”; “How well do you think the government currently governs HIPC in the area of health care?”; and “How complete do you think our HIPC governance tools are?”. The answers to the last three questions ranged from 0 to 5, with higher scores for a higher level of information technology, higher governance capacity, and better completeness of governance tools. In multiple logistic regression, the three dependent variables were scored as 1–2 for low-level, poor capacity, and incomplete groups, 3 for medium-level, general capacity, and general completeness groups, and 4–5 for high-level, high capacity, and completeness groups.
Variable selection
Control variable
HIPC governance effectiveness, as a subjective evaluation, is influenced by personal characteristics such as personal preference and temperament. Considering the representativeness of the sample, this study selected individual characteristic variables, controlling for gender, age, education, profession, and type of unit. The specific variable assignments are shown in Table 1.
Explaining variable
In this study, HIPC governance was used as the dependent variable. The explanatory variables mainly included (1) governance of individual factors, including governance of the population's awareness of insurance participation and governance of the population's ability to participate in insurance; and (2) government factors, which included the degree of clarity of the government's governance function, the degree of medical insurance information sharing between regions, the degree of information technology construction in the medical insurance sector, the government's governance capacity, and the completeness of governance tools. The specific distribution of variables is shown in Table 1.
Statistical analysis
Logistic regression model
Logistic regression is a generalized linear regression analysis that uses a logit function to fit data to predict the probability of an event occurring or to screen for joint markers or dependent variables, with the probability and independent variable model usually being an S-shaped curve. Since the dependent variables used in this study were binary (0 and 1), the binary logistic regression model, which is mainly used to describe an optimal mapping relationship between the independent variables and the response variables with dichotomous nature, was used. The expression of the logistic regression mathematical model was as follows (17–19):
where pi = probability {yi = y1|Xi}, β0 is the y intercept, β′ is the vector of slope parameters, yi is the first ordered level of y, and Xi is the vector of explanatory variables.
In the binary logistic regression equation above, the response variable was the log dominance ratio (log) for Y = 1. The meaning of the regression coefficient can be understood as the change in the log dominance ratio of the dependent variable that may be induced by a unit change in the independent variable, i.e., the parameter in the regression equation that indicates the magnitude of the effect of the independent variable on the dependent variable. As the logarithmic function is more convenient, faster, and easier to interpret, it was chosen as the model for statistical analysis in this study to determine the factors affecting the effectiveness of HIPC governance.
Data analysis
All statistical analyses were performed using SPSS 26.0, with confidence intervals at 95%, and bilateral statistical significance was based on a p-value of <0.05. In the first step, descriptive statistical analysis was used to describe participant characteristics and HIPC governance effects; in the second step, chi-square independence tests were used to analyze differences in categorical variables; and in the third step, multi-factor logistic regression analysis was performed to identify significant factors influencing HIPC governance effects and to control for possible confounding variables.
Results
The characteristics of the sample
Among the control variables, men accounted for 32.05%, and women for 67.95% of respondents; the respondents were all adults, with a majority aged 44 years and below (66.73%). The lowest level of education among the group was bachelor's degree (56.10%), indicating that the education level of the respondents was in line with the actual situation of the nine-year compulsory education implemented in China. Most of the survey respondents' majors were in medical insurance-related disciplines, accounting for 53.6% of respondents; their job types were mainly in the medical insurance sector, accounting for 81.1% of respondents, indicating that most of the survey respondents' job types were directly related to medical insurance.
Single-factor analysis of the governance effect of full population coverage of medical insurance
The results of the survey showed that 59% of the respondents had a good knowledge of the governance efficiency of the HIPC. The governance effect of HIPC and its influencing factors are detailed in Table 1. The HIPC had achieved remarkable results since the government began governing health insurance coverage for the entire population. Refer to Figure 1 for details.
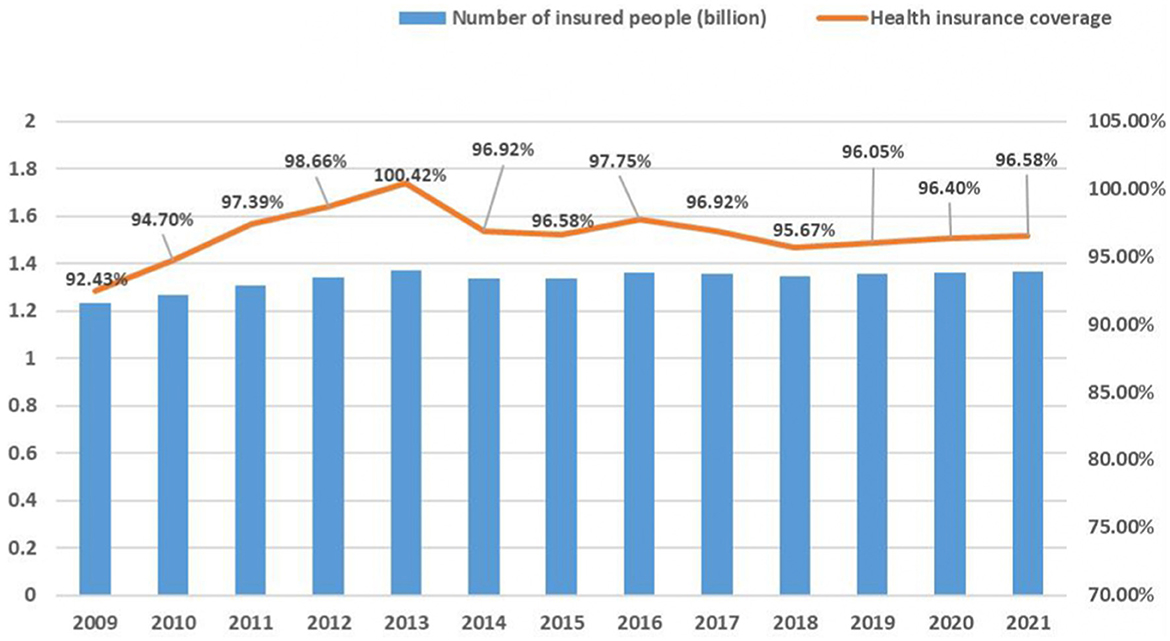
Figure 1. Trend of health insurance coverage in China from 2009 to the present. In 2013, due to the duplication of statistics on the number of participants in the New Rural Cooperative and urban medical insurance, the total number of people covered by the national medical insurance was high, and if duplicate statistics were excluded, the participation rate would still be above 95%.
Table 2 illustrates the estimation results controlling for individual background characteristics with government factors and governance of individual factors as the main explanatory variables. The results showed that all individual factors have a significant effect (p < 0.05) on the effectiveness of HIPC governance, and all the governing factors are significantly associated with the efficacy of HIPC governance (p < 0.05), except for inter-regional health insurance information sharing. For details, refer to Table 2.
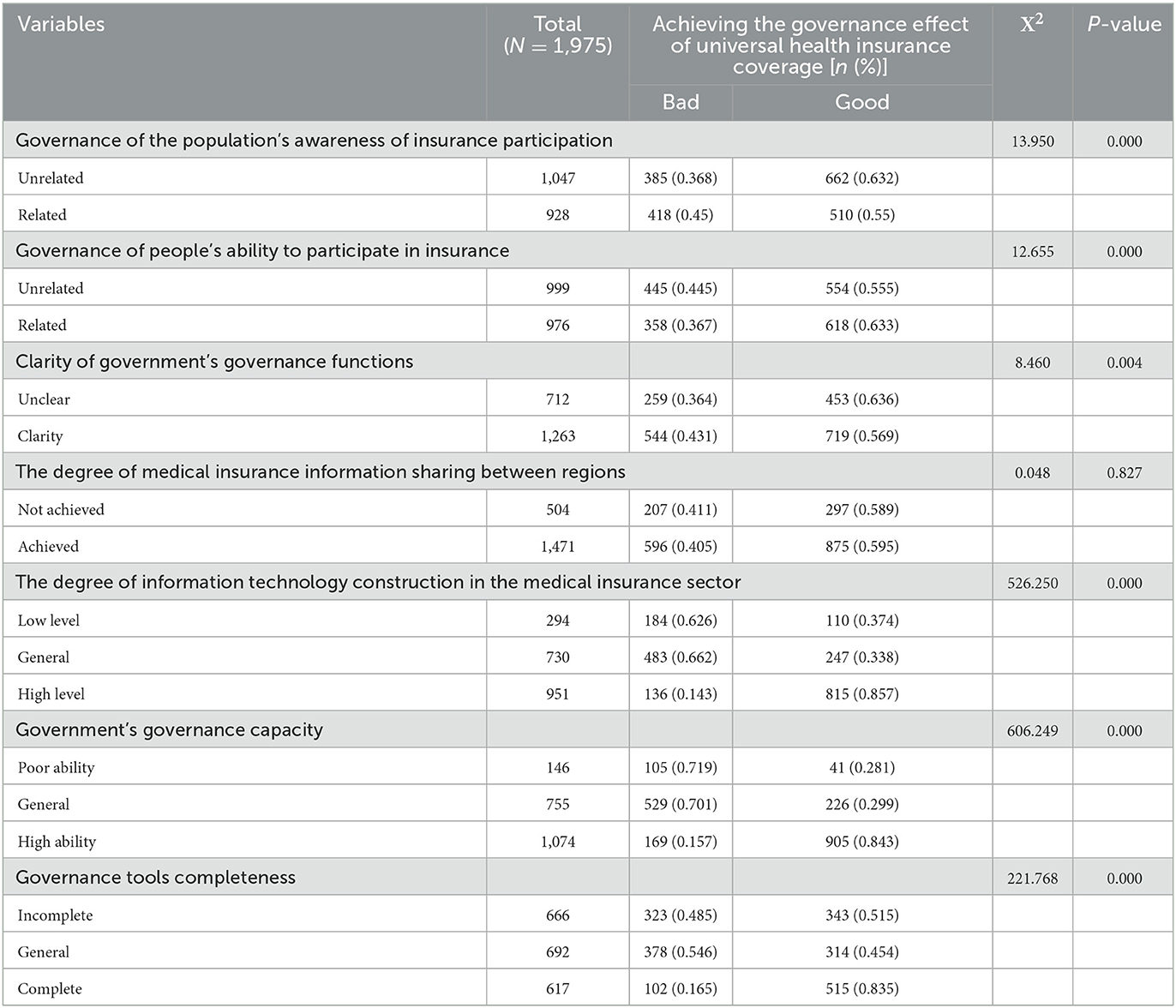
Table 2. Sample characteristics and univariate analysis of the effect of governance on health insurance coverage for the entire population.
Multivariate logistic regression analysis of the governance effects of full population coverage of medical insurance
Based on the results of the single-factor analysis, this study assigned the statistically significant influencing factors and the effect of governance of China's health insurance coverage for the whole population in turn. The study substituted them into the logistic regression equation for multi-factor analysis; the results are shown in Table 3 in detail. The effect of targeting the governance of the population's ability to participate and the governance of the health insurance coverage across the population is positively affected at a significant level of 5%. Other indicators positively affect the effect of governance of China's health insurance total population coverage, among which the degree of information construction of the health insurance sector, government's governance ability, and governance tools positively affect at a 5% significant level, that is, the higher the degree of information construction of health insurance sector, the higher the government's governance ability; and the more complete the governance tools and instruments, the better the effect of governance of China's health insurance total population coverage.
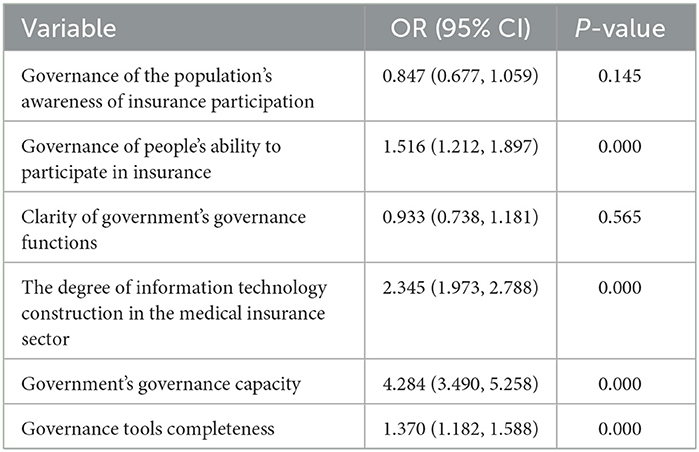
Table 3. The logistic regression analysis of factors influencing the effectiveness of governance for full population coverage of medical insurance (n = 1,975).
Sensitivity analysis
This study conducted a sensitivity analysis by changing the outcome classification of the independent or dependent variables, which may have an impact on the significant results of the explanatory variables. Therefore, in this study, the results of the independent variables were first set to 3 categories, i.e., 1 = not very effective (choice of “not at all effective” and “not very effective”), 2 = average (choice of “average”) and 3 = effective (choice of “relatively effective” and “very effective”), the results of the explanatory variables were statistically analyzed according to the 3 categories, and the results of the adjusted model were unchanged (Table 4). Subsequently, the independent variables were analyzed by the two classification results, the explanatory variables were analyzed by the five classification results of the original data, and the statistical steps were repeated. The adjusted model results were generally consistent with the statistical analysis of the previous data, again indicating that the results of this study were stable and reliable (Table 5).
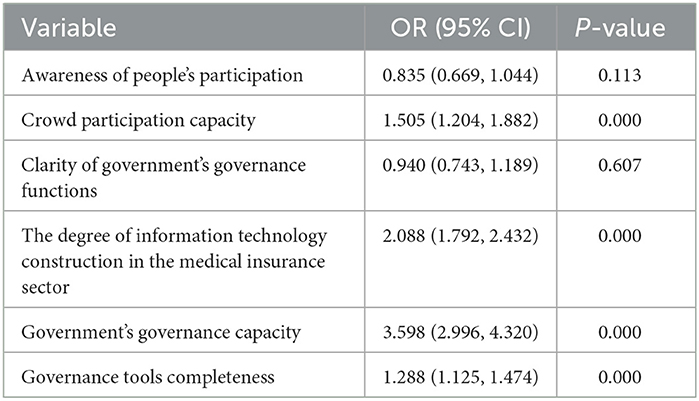
Table 4. Classification results according to the explaining variable of the original data—logistic regression analysis (n = 1,975).
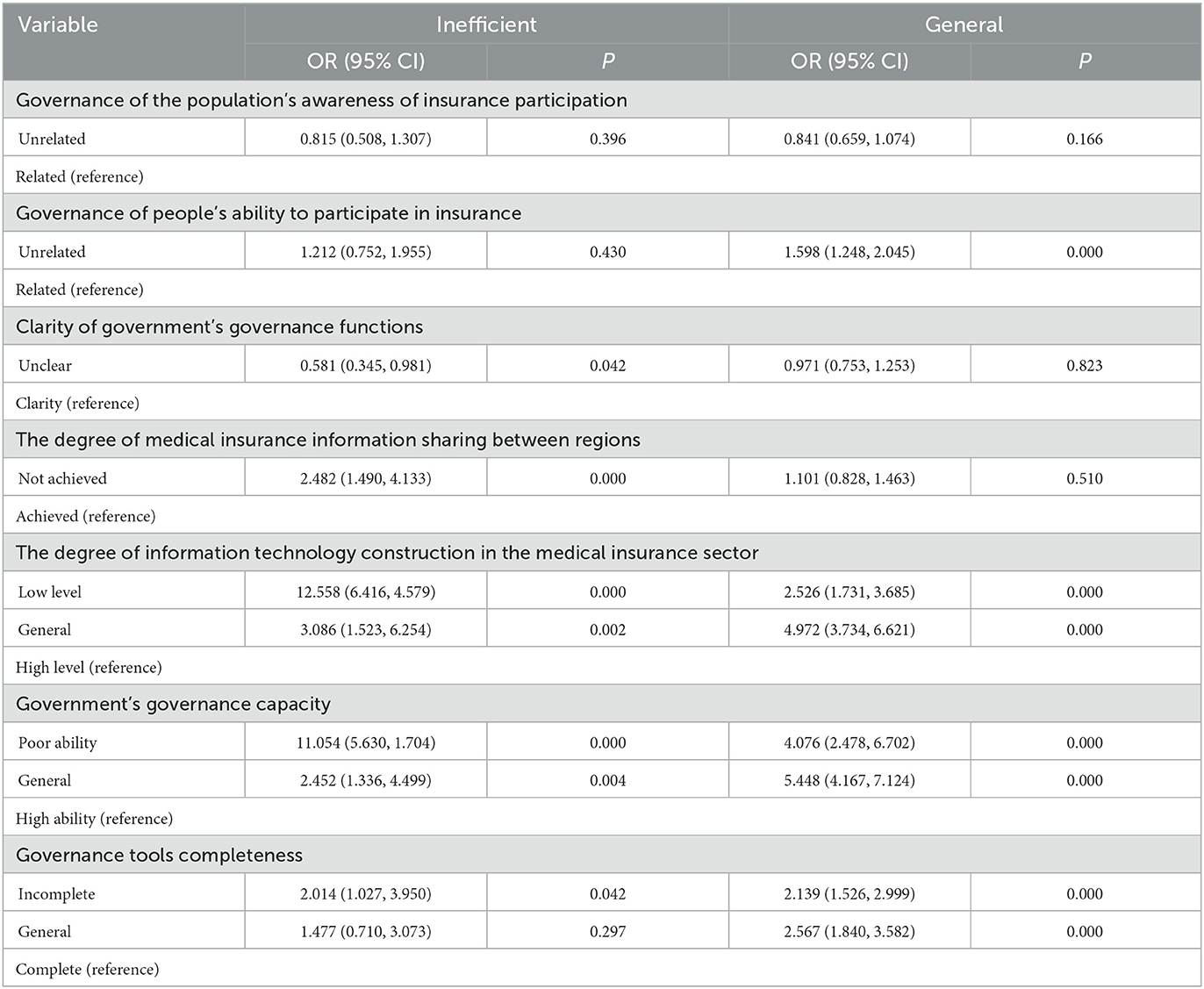
Table 5. Analysis of results classified according to explaining variable 3—logistic regression analysis (n = 1,975).
Discussion
This study aimed to investigate the factors that influence the effectiveness of the governance of health insurance total population coverage. The results of the study showed that factors such as the governance of the population's ability to participate in insurance, the degree of information technology construction in the medical insurance sector, government's governance capacity, and governance tools completeness significantly affect the effectiveness of the governance of health insurance coverage for the whole population.
This study found that governance of the population's ability to participate in insurance was positively correlated with the impact of achieving governance effectiveness in HIPC. Studies have shown that nearly half of China's uninsured population is uninsured due to their low participation ability. This group offers a high willingness to participate, resulting in an unbalanced phenomenon of high demand for health insurance and adverse selection of actual participation (20–22). Studies have shown that, in other countries, the uninsured are more likely to be uninsured due to their low capacity to participate (23, 24). China noticed this phenomenon early in the process of HIPC promotion and has enhanced the public's ability to participate in health insurance, especially for the poor, through diversified means such as health insurance poverty alleviation policies, medical assistance underwriting policies, and continuous lowering of health insurance contribution thresholds, effectively bringing people with low participation ability into the scope of health insurance, making China's HIPC governance achieve good results (21, 25). According to official data, since China launched medical insurance to help the poor and established a multi-level medical insurance system, 230 million people in poverty have been insured, and the coverage of the poor has stabilized at over 99.9% (26). Therefore, China should adhere to the policy guidelines such as “health insurance for the poor” and continue the evolution of the governance measures for the population's ability to participate in the insurance and continue to consolidate the achievements of “universal health insurance, Everyone who can be guaranteed is guaranteed” in China.
Second, the degree of information technology construction in the medical insurance sector significantly impacts the effectiveness of achieving full population coverage of medical insurance governance. Tomasz Janowski's research also shows that the development of information technology and digitalization affects governance goals (27). Countries such as Brazil have effectively promoted HIPC by adopting a unified medical insurance information system platform (28). Currently, China's medical insurance information technology is in its infancy. The medical insurance information system cannot share information with other related departments, making it difficult to effectively and timely screen uninsured people and bring them into the medical insurance coverage from a technical level (29–31). Meanwhile, medical insurance information systems are usually oriented toward regional health insurance services (31), and there are barriers to information systems between different medical insurance systems. This results in the inability to share information on public participation, the increased probability of biased targeting of uninsured people by health insurance departments, and the increased difficulty of full population coverage of medical insurance, which in turn affects the effectiveness of the governance of full population coverage of medical insurance in the country. Therefore, China should hasten the construction of a unified national health insurance information platform, break the barriers between regional health insurance information systems, actively promote the integration and docking of health insurance information systems with information systems of other relevant departments, rely on blockchain and other technologies to achieve inter-regional and inter-departmental sharing of insurance information, establish an identification system for uninsured people, and achieve accurate targeting of uninsured people through big data matching and other means.
Our findings showed that the size of the government's governance capacity and the completeness of its governance tools directly correlates with the effectiveness of the governance of the whole population coverage of health insurance in China. Studies by related scholars also emphasize that the government's governance capacity and the completeness of governance tools are paramount to enhancing the effectiveness of HIPC governance (32–34). Relevant studies have shown that governance in the public sphere, central government institutions, and governance capacity are critical prerequisites for effective governance (35). China has also gradually recognized this in HIPC governance and established the National Health Insurance Administration in 2018 to strengthen the top-level design of HIPC and rationalize the health insurance management system. The former Health and Planning Commission was responsible for the new agricultural cooperation. The former Ministry of Human Resources and Social Security was responsible for the medical insurance of urban residents and urban workers; three kinds of medical security were unified under the management of the Medical Insurance Bureau. From an institutional perspective, it solved the long-standing state of HIPC governance of nine dragons, effectively circumvented the dead ends in HIPC governance, and provided a solid and robust organizational guarantee for HIPC governance in China. In addition, China also provided a good platform and legal support for HIPC governance in China by building a medical insurance information and business coding platform, continuously improving its medical insurance legal system and other diversified governance tools. Its efforts in augmenting its governance capacity and governance tools have effectively alleviated China's HIPC governance dilemma and laid a solid foundation for it to achieve more effective HIPC governance. In recent years, the new pneumonia epidemic has accelerated the process of building digital health insurance in China. In addition, the integration of modern information technology such as blockchain, the Internet of Things, and big data with the health insurance governance system has become the mainstream trend to enhance governance capacity and improve governance tools in China. However, it is still in its initial stage of development and needs to be explored to enhance digital governance capacity and improve digital governance tools. Therefore, China should continue to strengthen its macro system construction and planning, plan for the development of governance capacity and tools, respond positively to the needs of HIPC governance, build a digital governance network for health insurance, strengthen modern information technology, and provide strong institutional and technical support for the development of health insurance governance capacity and tools.
Conclusions
In this study's survey, 59% of respondents believe that HIPC governance is effective. China has effectively solved the problem of low-participation ability and people's participation through medical insurance poverty alleviation, medical assistance underwriting policy, and lowering the threshold of medical insurance payment. It has also actualized the precise expansion of medical insurance coverage for the uninsured, resulting in its HIPC governance achieving good results. In addition, in realizing HIPC governance, accelerating the construction of health insurance information systems, promoting the modernization of governance capacity, and expanding good governance tools that can effectively promote the accurate expansion of health insurance population coverage, which is an effective path to realizing efficient HIPC governance.
Limitations
Although this study contributes to the understanding of HIPC governance in China and the factors influencing it, there are still some limitations that need to be noted. China's HIPC governance is the focus of China's current healthcare development, and it has gradually entered a period of rapid development with the continuous improvement of the healthcare governance system, and because the data used in this study is a cross-sectional study for the whole country, it cannot show a longitudinal analysis of the effectiveness of China's health care governance; therefore, the findings of this study may show certain stage characteristics.
Data availability statement
The data analyzed in this study is subject to the following licenses/restrictions: The datasets generated during and/or analyzed during the current study are not publicly available due to protection of privacy of respondents, but are available from the corresponding author on reasonable request. Requests to access these datasets should be directed to a2FuZ3poZW5nQGhyYm11LmVkdS5jbg==.
Ethics statement
The studies involving human participants were reviewed and approved by Ethics Committee of Harbin Medical University. The patients/participants provided their written informed consent to participate in this study.
Author contributions
TZ and WM conducted and planned the study and designed the methodology. TZ and HZ drafted the manuscript. TZ and JX conducted a feasibility analysis and helped conceptualize the project. QS and JL conducted the literature search and data visualization. FD and JH proofread and revised the article's language. FC and ZK revised the manuscript and gave critical feedback. ZK and GT conducted quality control and review of the manuscripts. TZ, WM, HZ, and JX contributed to the concept, design, research, data analysis, and drafting of the article. All authors have read and approved the manuscript for publication.
Funding
This study was funded by the National Natural Science Foundation of China (72074064 and 71573068), China Postdoctoral Science Foundation (2019M650068), China Postdoctoral Fund Special Grant Program (2018T110319), and the National Social Science Fund of China (19AZD013). The funding body did not influence the study design, data collection, data analysis, data interpretation, or writing of the manuscript.
Conflict of interest
The authors declare that the research was conducted in the absence of any commercial or financial relationships that could be construed as a potential conflict of interest.
Publisher's note
All claims expressed in this article are solely those of the authors and do not necessarily represent those of their affiliated organizations, or those of the publisher, the editors and the reviewers. Any product that may be evaluated in this article, or claim that may be made by its manufacturer, is not guaranteed or endorsed by the publisher.
Abbreviations
HIPC, Health insurance population coverage.
References
1. WHO. Address to Sixtieth World Health Assembly. WHO (2007). Available online at: https://www.who.int/director-general/speeches/detail/address-to-sixtieth-world-health-assembly (accessed May 20, 2022).
2. Kisa A, Kisa S, Collaborators GS. Global, regional, and national burden of stroke and its risk factors, 1990–2019: a systematic analysis for the Global Burden of Disease Study 2019. Lancet Neurol. (2021) 20:795–820. doi: 10.1016/S1474-4422(21)00252-0
3. Jing L, Chen R, Jing L, Qiao Y, Lou J, Xu J, et al. Development and enrolee satisfaction with basic medical insurance in China: a systematic review and stratified cluster sampling survey. Int J Health Plann Manage. (2017) 32:285–98. doi: 10.1002/hpm.2430
4. Ma M, Li Y, Wang N, Wu Q, Shan L, Jiao M, et al. Does the medical insurance system really achieved the effect of poverty alleviation for the middle-aged and elderly people in China? Characteristics of vulnerable groups and failure links. BMC Public Health. (2020) 20:435. doi: 10.1186/s12889-020-08554-3
5. Ma M, Tian W, Kang J, Li Y, Xia Q, Wang N, et al. Does the medical insurance system play a real role in reducing catastrophic economic burden in elderly patients with cardiovascular disease in China? Implication for accurately targeting vulnerable characteristics. Glob Health. (2021) 17:36. doi: 10.1186/s12992-021-00683-7
6. National Healthcare Security Administration. Statistical Snapshot of the Development of Medicare in 2021 (2022). Available online at: http://www.nhsa.gov.cn/art/2022/3/4/art_7_7927.html
7. Meng Q, Xu L. Monitoring and Evaluating Progress towards Universal Health Coverage in China. PLoS Med. (2014) 11:e1001694. doi: 10.1371/journal.pmed.1001694
8. Mao W, Tang Y, Tran T, Pender M, Khanh PN, Tang S. Advancing universal health coverage in China and Vietnam: lessons for other countries. BMC Public Health. (2020) 20:1791. doi: 10.1186/s12889-020-09925-6
9. Derakhshani N, Maleki M, Pourasghari H, Azami-Aghdash S. The influential factors for achieving universal health coverage in Iran: a multimethod study. Bmc Health Serv Res. (2021) 21:724. doi: 10.1186/s12913-021-06673-0
10. Bayarsaikhan D Kwon S and Chimeddagva D. Social health insurance development in Mongolia: Opportunities and challenges in moving towards Universal Health Coverage. Int Soc Secur Rev. 68:93–113. doi: 10.1111/issr.12089
11. Ranabhat CL, Jakovljevic M, Dhimal M, Kim C-B. Structural factors responsible for universal health coverage in low- and middle-income countries: results from 118 countries. Front Public Health. (2020) 7:414. doi: 10.3389/fpubh.2019.00414
12. Yasar GY, Ugurluoglu E. Can Turkey's general health insurance system achieve universal coverage? Int J Health Plann Manage. (2011) 26:282–95. doi: 10.1002/hpm.1079
13. Do N, Oh J, Lee JS. Moving toward universal coverage of health insurance in Vietnam: barriers, facilitating factors, and lessons from Korea. J Korean Med Sci. (2014) 29:919–25. doi: 10.3346/jkms.2014.29.7.919
14. Kazungu JS, Barasa EW. Examining levels, distribution and correlates of health insurance coverage in Kenya. Trop Med Int Health. (2017) 22:1175–85. doi: 10.1111/tmi.12912
15. Nguyen TD, Wilson A. Coverage of health insurance among the near-poor in rural Vietnam and associated factors. Int J Public Health. (2017) 62:63–73. doi: 10.1007/s00038-016-0911-z
16. Lee DC, Liang H, Shi L. The convergence of racial and income disparities in health insurance coverage in the United States. Int J Equity Health. (2021) 20:96. doi: 10.1186/s12939-021-01436-z
17. Dissanayake S, Lu J. Analysis of severity of young driver crashes: sequential binary logistic regression modeling. Transp Res Rec J Transp Res Board. (2002) 1784:108–14. doi: 10.3141/1784-14
18. Lombardo L, Cama M, Conoscenti C, Märker M, Rotigliano E. Binary logistic regression versus stochastic gradient boosted decision trees in assessing landslide susceptibility for multiple-occurring landslide events: application to the 2009 storm event in Messina (Sicily, southern Italy). Nat Hazards. (2015) 79:1621–48. doi: 10.1007/s11069-015-1915-3
19. Harrell, FE. Regression Modeling Strategies: With Applications to Linear Models, Logistic and Ordinal Regression, and Survival Analysis. Cham: Springer International Publishing (2015). doi: 10.1007/978-3-319-19425-7
20. Zheng L, Hu R, Dong Z, Hao Y. Comparing the needs and utilization of health services between urban residents and rural-to-urban migrants in China from 2012 to 2016. BMC Health Serv Res. (2018) 18:717. doi: 10.1186/s12913-018-3522-y
21. Qin L, Pan S, Wang C, Jiang Z. Adverse selection in China's new rural cooperative medical scheme. China Agric Econ Rev. (2012) 4:69–83. doi: 10.1108/17561371211196784
22. Meng Y, Zhang X, Han J. The impact of medical insurance programmes on the health of the insured: evidence from China. Int J Health Plann Manage. (2020) 35:718–34. doi: 10.1002/hpm.2931
23. Amaya JL, Ruiz F, Trujillo AJ, Buttorff C. Identifying barriers to move to better health coverage: preferences for health insurance benefits among the rural poor population in La Guajira, Colombia. Int J Health Plann Manage. (2016) 31:126–38. doi: 10.1002/hpm.2268
24. Tetteh J, Ayanore MA, Ayanore M, N Kugbey, Fusheini A, Ayanore AA. Health insurance coverage, type of payment for health insurance, and reasons for not being insured under the National Health Insurance Scheme in Ghana. Health Econ Rev. (2019) 9:39. doi: 10.1186/s13561-019-0255-5
25. Chen C, Pan J. The effect of the health poverty alleviation project on financial risk protection for rural residents: evidence from Chishui City, China. Int J Equity Health. (2019) 18:79. doi: 10.1186/s12939-019-0982-6
26. National Healthcare Security Administration. Questions and Answers on the “14th Five-Year Plan” for Universal Health Coverage (2021). Available online at: http://www.nhsa.gov.cn/art/2021/9/30/art_38_6144.html
27. Janowski T. Implementing sustainable development goals with digital government – aspiration-capacity gap. Gov Inf Q. (2016) 33:603–13. doi: 10.1016/j.giq.2016.12.001
28. Massuda A, Hone T, Leles FAG, de Castro MC, Atun R. The Brazilian health system at crossroads: progress, crisis and resilience. BMJ Glob Health. (2018) 3:e000829. doi: 10.1136/bmjgh-2018-000829
29. Tang C, Wu L. Problems and countermeasures of medical protection system for rural poor patients in the context of health poverty alleviation. Med Soc. (2019) 32:118–22. doi: 10.13723/j.yxysh.2019.09.029
30. Li Y, Lu C, Liu Y. Medical Insurance information systems in china: mixed methods study. JMIR Med Inf. (2020) 8:e18780. doi: 10.2196/18780
31. Yoon K, Park S, Choi S, Lee M. A proposal for public health information system-based health promotion services. Processes. (2020) 8:338. doi: 10.3390/pr8030338
32. Bump JB. The long road to universal health coverage: historical analysis of early decisions in Germany, the United Kingdom, and the United States. Health Syst Reform. (2015) 1:28–38. doi: 10.4161/23288604.2014.991211
33. Fryatt R, Bennett S, Soucat A. Health sector governance: should we be investing more? BMJ Glob Health. (2017) 2:e000343. doi: 10.1136/bmjgh-2017-000343
34. Acharya KK. The capacity of local governments in Nepal: from government to governance and governability? Asia Pac J Public Adm. (2018) 40:186–97. doi: 10.1080/23276665.2018.1525842
Keywords: medical insurance, population coverage, medical insurance governance, governance effect, influence factors
Citation: Zhang T, Zhang H, Miao W, Xu J, Shi Q, Liu J, Deng F, He J, Chen F, Kang Z, Wu Q and Tian G (2023) What has affected the governance effect of the whole population coverage of medical insurance in China in the past decade? Lessons for other countries. Front. Public Health 11:1079173. doi: 10.3389/fpubh.2023.1079173
Received: 07 November 2022; Accepted: 02 March 2023;
Published: 30 March 2023.
Edited by:
Sabina Baraković, University of Sarajevo, Bosnia and HerzegovinaReviewed by:
Petra Maresova, University of Hradec Králové, CzechiaJunli Zhu, Capital Medical University, China
Copyright © 2023 Zhang, Zhang, Miao, Xu, Shi, Liu, Deng, He, Chen, Kang, Wu and Tian. This is an open-access article distributed under the terms of the Creative Commons Attribution License (CC BY). The use, distribution or reproduction in other forums is permitted, provided the original author(s) and the copyright owner(s) are credited and that the original publication in this journal is cited, in accordance with accepted academic practice. No use, distribution or reproduction is permitted which does not comply with these terms.
*Correspondence: Zheng Kang, a2FuZ3poZW5nQGhyYm11LmVkdS5jbg==
†These authors share first authorship
‡ORCID: Zheng Kang orcid.org/0000-0001-6074-611X