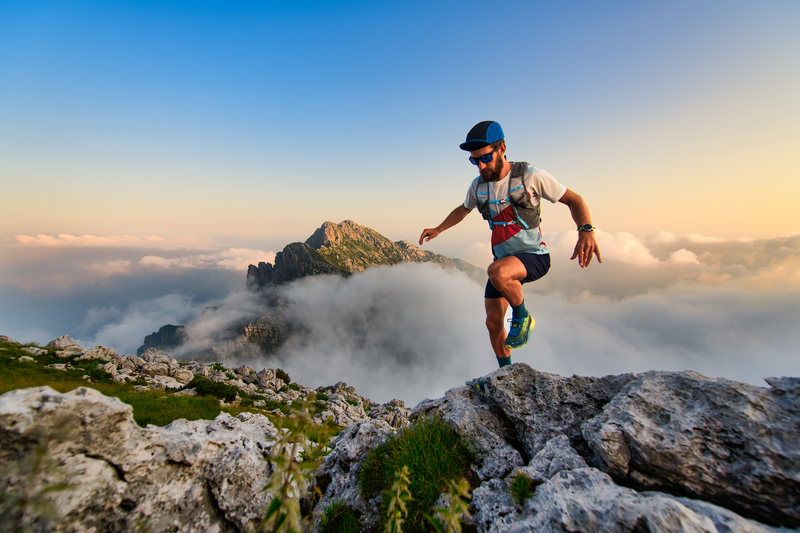
94% of researchers rate our articles as excellent or good
Learn more about the work of our research integrity team to safeguard the quality of each article we publish.
Find out more
ORIGINAL RESEARCH article
Front. Public Health , 30 September 2022
Sec. Infectious Diseases: Epidemiology and Prevention
Volume 10 - 2022 | https://doi.org/10.3389/fpubh.2022.995664
Background: Sleep disturbance including insomnia and sleep duration is associated with an increased risk of infectious. With the ongoing coronavirus disease 2019 (COVID-19) pandemic, it is important to explore potential causal associations of sleep disturbance on COVID-19 susceptibility and hospitalization.
Method: Insomnia and sleep duration were selected as exposure. Outcomes included susceptibility and hospitalization for COVID-19. Two sample mendelian randomization design was used to assess causality between sleep and COVID-19. Inverse variance weighted method was used as main analysis method to combine the ratio estimates for each instrumental variable to obtain the causal effect. Cochran's Q statistic was used to test for global heterogeneity. MR-Egger and weighting median estimator (WME) were used as sensitivity analysis to ensure the stability and reliability of the results. MR-Egger intercept term was used to test the mean pleiotropy. In addition, the direct effects of insomnia and sleep duration on COVID-19 susceptibility and hospitalization were estimated using multivariable mendelian randomization (MVMR).
Results: Univariate MR provided no evidence of a causal associations of insomnia on COVID-19 susceptibility (OR = 1.10, 95% CI:0.95, 1.27; p = 0.21) and hospitalization (OR = 0.61, 95% CI:0.40, 0.92; p = 0.02); as does sleep duration (ORCOIVD − 19susceptibility = 0.93, 95% CI:0.86, 1.01; p = 0.07; ORCOIVD − 19 hospitalization = 1.21, 95% CI: 0.99, 1.47; p = 0.08). MVMR results showed that insomnia may be a risk factor for increased susceptibility to COVID-19 (OR = 1.65, 95% CI: 1.34, 2.05; p <0.001); and sleep duration was also associated with increased COVID-19 susceptibility (OR = 1.31, 95% CI: 1.18, 1.46; p < 0.001).
Conclusion: Insomnia and extreme sleep duration may risk factors for increased COVID-19 susceptibility. Relieving insomnia and maintaining normal sleep duration may be powerful measures to reduce COVID-19 infections.
Up to now, coronavirus disease 2019 (COVID-19) pandemic has caused more than 500 million people infected and more than 6 million people died from COVID-19 in worldwide (WHO, 2021: https://covid19.who.int). To date, there is still no specific therapy for this virus. And there are still outbreaks from time to time around the world. The isolation and treatment measures adopted in response to the COVID-19 have brought a heavy socio-economic burden to global countries. Therefore, exploring and discovering the risk factors of COVID-19, and taking appropriate protective measures for high-risk populations are important primary prevention strategies for current response to COVID-19. Sleep is an important means for relieving fatigue and improving physical function. However, sleep disturbance affect approximately one-quarter of the US population and are still increasing every year (1). Studies have shown that sleep disturbance can adversely affect the risk of infectious and inflammatory diseases (2–4). Sleep disturbances related to COVID-19 have received widespread attention, and studies from different countries have highlighted sleep quality issues in patients with COVID-19 (5–7). Insomnia and sleep duration are common complaints in sleep disturbances, and their association with COVID-19 susceptibility and hospitalization is unclear.
Mendelian randomization (MR), a powerful causal inference method, uses single nucleotide polymorphisms (SNPs) as instrumental variables to estimate the causal effect of an exposure on an outcome (8, 9). It is less affected by confounding and reverse causality than traditional observational studies (10). The core assumptions of MR were shown in Figure 1 (11).
Figure 1. The core assumptions of MR. Z represents the genetic instrument (SNPs), X the exposure (Insomnia, Sleep duration), Y the outcome (COVID-19 Susceptibility, COVID-19 hospitalization) and U denotes the confounders of the relationship of X-Y. Assumptions: 1. Genetic variation is closely related to the exposure of interest, γ ≠ 0; 2. Genetic variation has nothing to do with confounding, φ1 = 0; 3. Genetic variation only affects the outcome through X, φ2 = 0.
This study used univariate MR to explore the potential causal relationship of insomnia, sleep duration on COVID-19 susceptibility and hospitalization, and multivariable Mendelian randomization (MVMR) (12) was used to analyze the effects of insomnia and sleep duration on COVID-19 susceptibility and hospitalization.
In this study, insomnia and sleep duration were selected as exposures. COVID-19 susceptibility and COVID-19 hospitalization were outcomes. Summary-level data on sleep and COVID-19 were obtained from four published genome wide association studies (GWAS). And ethical approval had been obtained for these GWAS (Table 1).
Summary-level data for insomnia were derived from the largest available meta-analysis of GWAS, including unrelated European descent individuals from UK Biobank (UKB, N = 453,379, cases = 345,022, control = 108,357). Self-reported insomnia symptoms were determined by answering questions such as “Do you have trouble falling asleep at night?”, “do you wake up in the middle of the night?” (13).
Genetic association estimates with sleep duration were obtained from the UKB participants of European ancestry (N = 446,118, 55.9% male) (14). The mean self-reported sleep duration was 7.2 h (standard deviation = 1.1 h). Sleep duration was treated as a continuous variable, and assessed by the question: “How many hours do you sleep per day (including naps)?” The answer was responded in hour increments and could only contain integer values.
GWAS for COVID-19 susceptibility and hospitalization were obtained from the COVID-19 Host Genetics Initiative (15). COVID-19 susceptibility was a group of laboratory-confirmed or self-reported COVID-19 cases (N = 32,494) compared to the rest of the population (including all individuals who were not part of the case group, N = 1,316,207). COVID-19 hospitalization was measured based on the group hospitalized for COVID-19 (N = 8,316) vs. the rest of the population (N = 1,549,095). The participants were all of European ancestry, and details are available from the COVID-19 Host Genetics Program homepage (https://www.covid19hg.org/).
As the exposure samples were from the UK Biobank, we excluded subjects from the UK Biobank when selecting outcome samples to avoid bias due to sample overlap in MR analysis.
To avoid potential weak instrumental bias (16), we used genome-wide significance (p < 5 × 10−8) as the threshold for inclusion of SNPs. The instrumental variable strength was assessed using F statistic (17). To further ensure instruments independence, SNPs in linkage disequilibrium (LD) were removed from the instrument variable set (r2 threshold = 0.001, window size = 10,000 kb). The F-statistics corresponding to the SNPs finally included in the MR model were all above 30, indicating that weak instrumental variable bias was unlikely (more details seen in Supplementary material 1).
In univariate MR, Inverse variance weight (IVW) (18) was used as the main analysis method, and global Q test (19) was used to detect the presence of heterogeneity among the effect estimates from individual SNPs. If there was heterogeneity, the random effect IVW model was selected; otherwise, the IVW method of the fixed effect model was selected. In MVMR, insomnia and sleep duration were used as exposures simultaneously to explore their effects on COVID-19 susceptibility and hospitalization.
MR-Egger (20) and weighting median estimator (WME) (21) were used as sensitivity analysis methods. MR-Egger provides a valid test for pleiotropy, and the estimates of the intercept term in MR-Egger can be interpreted as the average pleiotropic effects across SNPs. And the estimator of WME is consistent even when up to 50% of the information comes from invalid SNPs. In addition, to test the reverse causality, we also performed reverse MR analysis.
To account for multiple testing, we employed a Bonferroni-corrected threshold of p < 0.0125 (0.05/4 to correct for two sleep traits in relation to COVID-19 susceptibility and hospitalization). P values between 0.0125 and 0.05 were considered suggestive evidence of causality, requiring confirmation. All statistical analyses were performed using R (version 4.2.0.) software, and univariate MR and MVMR analyses were performed using the TwosampleMR and Mendelian Randomization packages, respectively.
IVW showed that the effect of insomnia on COVID-19 susceptibility was not statistically significant (OR = 1.10, 95% CI: 0.95, 1.27; p = 0.21), the sensitivity analysis method results were consistent with IVW method. In the analysis of the impact of insomnia on COVID-19 hospitalization, IVW method and the sensitivity analysis results showed a suggestive causal association between insomnia and COVID-19 hospitalization (OR = 0.61, 95% CI: 0.40, 0.92; p = 0.02) (Table 2). There was no causal association of sleep duration on COVID-19 susceptibility (OR = 0.93, 95% CI: 0.86, 1.01; p = 0.07). Sleep duration also had no causal effect on COVID-19 hospitalization (OR = 1.21, 95% CI: 0.99, 1.47; p = 0.08), the results of the sensitivity analysis methods were consistent with IVW method (Table 2).
The scatterplot and leave-one-out results of the univariate MR analysis were shown in Figure 2.
Figure 2. The scatterplot and leave-one-out results of the univariate MR analysis. (A) is the scatterplot and leave-one-out results of the effect of insomnia on COVID-19 susceptibility in univariate MR analysis. (B) is the scatterplot and leave-one-out results of the effect of insomnia on COVID-19 hospitalization in univariate MR analysis.
Insomnia and sleep duration were used as exposures to estimate their effects on COVID-19 susceptibility and hospitalization. MVMR results suggested that insomnia may be a risk factor for COVID-19 susceptibility (OR = 1.65, 95% CI: 1.34, 2.05; p < 0.001); Sleep duration also linked to increased COVID-19 susceptibility (OR = 1.31, 95% CI: 1.18, 1.46; p < 0.001). However, we did not find evidence of potential causal relationship of insomnia and sleep duration on COVID-19 hospitalization (ORCOIVD − 19 susceptibility = 0.74, 95% CI: 0.42, 1.30, p = 0.30; ORCOVID − 19 hospitalization=1.04, 95% CI: 0.78, 1.40, p = 0.77) (Table 3).
We explored potential causal associations between insomnia and sleep duration on COVID-19 susceptibility and hospitalization using two sample MR. Univariate MR showed no causal associations of insomnia and sleep duration with COVID-19 susceptibility and hospitalization. MVMR showed that both insomnia and sleep duration were risk factors for increased COVID-19 susceptibility, but there was no causal relationship with COVID-19 hospitalization. We found that univariate MR and MVMR results contradicted, and the reason for this may be that in the insomnia GWAS cohort used in univariate MR, the sleep duration of insomnia patients changed due to insomnia, that means, the sample had selection bias (22, 23). For this reason, the assumption 2 of MR may be violated (Figure 1), thereby the results of univariate MR can be biased. The reverse MR results also further verified this conjecture, reverse MR results showed a causal association between COVID-19 susceptibility and sleep duration (Supplementary Table 1), while insomnia had an impact on sleep duration (Supplementary Table 2). This suggested that sleep duration is a potential collider factor in univariate MR analysis (22), which lead to substantially biased estimates of associations in univariate MR. Notably, research showed that MR results are susceptible to selection bias, and when it is exists, the closer the true estimate is to the null, the easier it is for a reversal to occur (24). While MVMR is not affected by selection bias and collider factors, direct effects of different exposures on outcomes can be obtained (24, 25). Above all, the results of MVMR are more reliable, there is reason to believe that insomnia and sleep duration are risk factors for increased COVID-19 susceptibility. What's more, there was growing evidence that sleep disturbance can adversely affect adaptive immunity in humans. Sleep disturbance can activate the hypothalamic-pituitary-adrenal axis, increase the release of glucocorticoid, and weaken the body's immune response through the following three pathways. First, activation of the glucocorticoid receptor inhibits the transcription of many immune response genes; second, activation of the glucocorticoid receptor induces the transcription of certain anti-inflammatory genes (such as NFKBIA, which encodes IκBα), which interferes with the activation of the transcription factor, resulting in the blockade of the inflammatory cascade; finally, protein-protein interactions interfere with the pro-inflammatory transcription factors NF-κB and AP-1, antagonizing the transcription of inflammatory genes (26). Insomnia independently increases the risk of infectious and inflammatory diseases (27, 28), and Irwin et al. demonstrated that patients with insomnia exhibited a reduction in natural killer cells, which may lead to a decline in the body's natural immunity (29). Furthermore, extreme sleep duration is associated with infectious disease risk, and epidemiological evidence suggested that reduced (e.g., ≤5 hours) and prolonged (e.g., ≥ 9 h) sleep duration was associated with an increased risk of pneumonia (30). The above mechanisms may be the reasons for the increased susceptibility of COVID-19 in patients with insomnia. The sleep disturbance may be related to the confinement, anxiety and other psychosocial factors brought about by COVID-19, rather than COVID-19 itself (31).
Therefore, we suggested that in the future prevention and control of COVID-19, attention should be paid to people with sleep disorders, and taking necessary protective measures will help reduce their COVID-19 susceptibility. Insomnia is a modifiable risk factor, and studies have shown that some relaxation therapies, such as tai chi and yoga, can greatly improve symptoms of insomnia (32, 33). To sum up, carrying out necessary relaxation training in people with insomnia may reduce the risk of COVID-19 infection, alleviate the potential pressure and economic expenditure of COVID-19 epidemic prevention and control, and is expected to become an important primary preventive measure.
This study used two sample MR to explore the causal association between insomnia and sleep duration on COVID-19 susceptibility and hospitalization, compared with traditional observational studies, it is less susceptible to reverse causality and confounding. Furthermore, we found that due to the close biological association between sleep traits, univariate MR is susceptible to selection bias and it is difficult to draw correct conclusions. We suggested when performing univariate MR, it is necessary to fully consider the impact of other traits that are closely related to the exposure of interest on study results, and whether this will cause the violation of the core assumptions of MR. This study assumed a linear association between the exposures of interest and the outcomes, and the nonlinear association still needs to be further explored.
Insomnia and sleep duration are risk factors for increased COVID-19 susceptibility. Relieving insomnia and maintaining normal sleep duration may be a powerful measure to reduce COVID-19 infection.
Summary level data of this article are publicly available, sleep traits can be found at https://sleep.hugeamp.org/dinspector.html?dataset=GWAS_UKBB_eu. COVID-19 susceptibility and hospitalization can be found at https://www.covid19hg.org/.
TW, LP, and JJ designed the whole study. JM acquired and interpreted the data. LP and JJ performed the statistical analysis and drafted the manuscript. All authors read and approved the final manuscript.
This study was supported by the National Natural Science Foundation of China [Grant Numbers 81872715, 82073674, and 82103949], the Basic Research Project of Shanxi Province, China [Grant Number 20210302124186], and the Major Science and Technology Project of Shanxi Province [Grant Numbers 202102130501003 and 202005D121008].
We sincerely acknowledge the original GWAS, UK biobank consortium and COVID-19 host genetics initiative for the collection and management of the large-scale data resources.
The authors declare that the research was conducted in the absence of any commercial or financial relationships that could be construed as a potential conflict of interest.
All claims expressed in this article are solely those of the authors and do not necessarily represent those of their affiliated organizations, or those of the publisher, the editors and the reviewers. Any product that may be evaluated in this article, or claim that may be made by its manufacturer, is not guaranteed or endorsed by the publisher.
The Supplementary Material for this article can be found online at: https://www.frontiersin.org/articles/10.3389/fpubh.2022.995664/full#supplementary-material
1. Rosen RC, Zozula R, Jahn EG, Carson JL. Low rates of recognition of sleep disorders in primary care: comparison of a community-based versus clinical academic setting. Sleep Med. (2001) 2:47–55. doi: 10.1016/S1389-9457(00)00043-5
2. Dew MA, Hoch CC, Buysse DJ, Monk TH, Begley AE, Houck PR. Healthy older adults' sleep predicts all-cause mortality at 4 to 19 years of follow-up. Psychosom Med. (2003) 65:63–73. doi: 10.1097/01.PSY.0000039756.23250.7C
3. Kripke D. Mortality associated with sleep duration and insomnia. Arch Gen Psychiatry. (2002) 59. doi: 10.1001/archpsyc.59.2.131
4. Vgontzas AN, Fernandez-Mendoza J, Liao D, Bixler EO. Insomnia with objective short sleep duration: the most biologically severe phenotype of the disorder. Sleep Med Rev. (2013) 17:241–54. doi: 10.1016/j.smrv.2012.09.005
5. Partinen M. Sleep research in 2020: COVID-19-related sleep disorders. Lancet Neurol. (2021) 20:15–7. doi: 10.1016/S1474-4422(20)30456-7
6. Wang S, Xie L, Xu Y, Yu S, Yao B, Xiang D. Sleep disturbances among medical workers during the outbreak of COVID-2019. Occup Med. (2020) 5:5. doi: 10.1093/occmed/kqaa074
7. Mc A, Ff B, Rt A, Gf B. The enemy who sealed the world: effects quarantine due to the COVID-19 on sleep quality, anxiety, and psychological distress in the Italian population - ScienceDirect. Sleep Med. (2020) 75:12–20. doi: 10.1016/j.sleep.2020.05.011
8. Lawlor DA, Harbord RM, Sterne J, Timpson N, Smith GD. Mendelian randomization: Using genes as instruments for making causal inferences in epidemiology. Stat Med. (2008) 27:1133–63. doi: 10.1002/sim.3034
9. George DS, Shah E. 'Mendelian randomization': can genetic epidemiology contribute to understanding environmental determinants of disease? Int J Epidemiol. (2003) 1:1. doi: 10.1093/ije/dyg070
10. Davies NM, Holmes MV, Smith GD. Reading Mendelian randomisation studies: a guide, glossary, and checklist for clinicians. Bmj-Brit Med J. (2018) 362. doi: 10.1136/bmj.k601
11. Gao X, Wang H, Wang T. Review on correction methods related to the pleiotropic effect in Mendelian randomization. Zhonghua Liu Xing Bing Xue Za Zhi. (2019) 40:360–5.
12. Burgess S, Thompson S G. Multivariable Mendelian randomization: the use of pleiotropic genetic variants to estimate causal effects. Am J Epidemiol. (2015) 181:251–60. doi: 10.1093/aje/kwu283
13. Lane JM, Jones SE, Dashti HS, Wood AR, Aragam KG, Van Hees VT. Biological and clinical insights from genetics of insomnia symptoms. Nat Genet. (2019) 51:387–93. doi: 10.1038/s41588-019-0361-7
14. Dashti HS, Jones SE, Wood AR, Lane JM, Van Hees VT, Wang H. Genome-wide association study identifies genetic loci for self-reported habitual sleep duration supported by accelerometer-derived estimates. Nat Commun. (2019) 10:1100. doi: 10.1038/s41467-019-08917-4
15. THE C-H G I. The COVID-19 Host Genetics Initiative, a global initiative to elucidate the role of host genetic factors in susceptibility and severity of the SARS-CoV-2 virus pandemic. Eur J Human Genet. (2020) 28:715–8. doi: 10.1038/s41431-020-0636-6
16. Davies NM, Scholder SVK, Farbmacher H, Burgess S, Windmeijer F, Smith GD. The many weak instruments problem and Mendelian randomization. Stat Med. (2015) 34:454–68. doi: 10.1002/sim.6358
17. Baum CF, Schaffer ME, Stillman S. Enhanced routines for instrumental variables/generalized method of moments estimation and testing. Boston College Working Papers in Economics. (2007) 7:465–506. doi: 10.1177/1536867X0800700402
18. Burgess S, Butterworth A, Thompson SG. Mendelian randomization analysis with multiple genetic variants using summarized data. Genet Epidemiol. (2013) 37:658–65. doi: 10.1002/gepi.21758
19. Del Greco MF, Minelli C, Sheehanc NA, Thompsonc JR. Detecting pleiotropy in Mendelian randomisation studies with summary data and a continuous outcome. Stat Med. (2015) 34:2926–40. doi: 10.1002/sim.6522
20. Bowden J, Smith GD, Burgess S. Mendelian randomization with invalid instruments: effect estimation and bias detection through Egger regression. Int J Epidemiol. (2015) 44:512–25. doi: 10.1093/ije/dyv080
21. Bowden J, Smith GD, Haycock PC, Burgess S. Consistent estimation in mendelian randomization with some invalid instruments using a weighted median estimator. Genet Epidemiol. (2016) 40:304–14. doi: 10.1002/gepi.21965
22. Munafo MR, Tilling K, Taylor AE, Evans DM, Smith GD. Collider scope: when selection bias can substantially influence observed associations. Int J Epidemiol. (2018) 47:226–35. doi: 10.1093/ije/dyx206
23. Munafo M, Smith GD. Biased estimates in mendelian randomization studies conducted in unrepresentative samples. JAMA Cardiol. (2018) 3:181. doi: 10.1001/jamacardio.2017.4279
24. Schooling CM, Lopez PM, Yang Z, Zhao JV, Yeung SLA, Huang JV. Use of multivariable mendelian randomization to address biases due to competing risk before recruitment. Front Genet. (2021) 11. doi: 10.3389/fgene.2020.610852
25. Sanderson E, Smith GD, Windmeijer F, Bowden J. An examination of multivariable Mendelian randomization in the single-sample and two-sample summary data settings. Int J Epidemiol. (2019) 48:713–27. doi: 10.1093/ije/dyy262
26. Irwin MR, Cole SW. Reciprocal regulation of the neural and innate immune systems. Nat Rev Immunol. (2011) 11:625–32. doi: 10.1038/nri3042
27. Irwin MR. Why sleep is important for health: a psychoneuroimmunology perspective. Annu Rev Psychol. (2015) 66:143–72. doi: 10.1146/annurev-psych-010213-115205
28. Besedovsky L, Lange T, Born J. Sleep and immune function. Pflug Arch Eur J Phy. (2012) 463:121–37. doi: 10.1007/s00424-011-1044-0
29. Irwin M, Clark C, Kennedy B, Christian Gillin J, Ziegler M. Nocturnal catecholamines and immune function in insomniacs, depressed patients, and control subjects. Brain Behav Immun. (2003) 17:365–72. doi: 10.1016/S0889-1591(03)00031-X
30. Shafazand S, A. Prospective Study of Sleep Duration and Pneumonia Risk in Women. Yearbook of Neurology and Neurosurgery. (2012) 2012:149–50. doi: 10.1016/j.yneu.2012.05.042
31. Belleville G, Ouellet MC, Morin CM. Post-traumatic stress among evacuees from the 2016 fort mcmurray wildfires: exploration of psychological and sleep symptoms three months after the evacuation. Int J Env Res Public Health. (2019) 16. doi: 10.3390/ijerph16091604
32. Irwin MR, Olmstead R, Motivala SJ. Improving sleep quality in older adults with moderate sleep complaints: a randomized controlled trial of Tai Chi Chih. Sleep. (2008) 31:1001–8.
Keywords: insomnia, sleep duration, COVID-19, Mendelian randomization, causal inference
Citation: Peng L, Jing J, Ma J, He S, Gao X and Wang T (2022) Insomnia and sleep duration on COVID-19 susceptibility and hospitalization: A Mendelian randomization study. Front. Public Health 10:995664. doi: 10.3389/fpubh.2022.995664
Received: 16 July 2022; Accepted: 07 September 2022;
Published: 30 September 2022.
Edited by:
Hai-Feng Pan, Anhui Medical University, ChinaReviewed by:
Qing Lu, University of Florida, United StatesCopyright © 2022 Peng, Jing, Ma, He, Gao and Wang. This is an open-access article distributed under the terms of the Creative Commons Attribution License (CC BY). The use, distribution or reproduction in other forums is permitted, provided the original author(s) and the copyright owner(s) are credited and that the original publication in this journal is cited, in accordance with accepted academic practice. No use, distribution or reproduction is permitted which does not comply with these terms.
*Correspondence: Tong Wang, dG9uZ3dhbmdAc3htdS5lZHUuY24=
†These authors have contributed equally to this work
Disclaimer: All claims expressed in this article are solely those of the authors and do not necessarily represent those of their affiliated organizations, or those of the publisher, the editors and the reviewers. Any product that may be evaluated in this article or claim that may be made by its manufacturer is not guaranteed or endorsed by the publisher.
Research integrity at Frontiers
Learn more about the work of our research integrity team to safeguard the quality of each article we publish.